Click through the PLOS taxonomy to find articles in your field.
For more information about PLOS Subject Areas, click here .
Loading metrics
Open Access
Peer-reviewed
Research Article
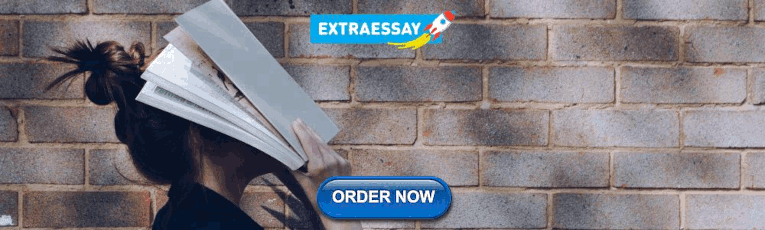
Review of guidance papers on regression modeling in statistical series of medical journals
Roles Data curation, Formal analysis, Investigation, Methodology, Software, Visualization, Writing – original draft, Writing – review & editing
* E-mail: [email protected] (CW); [email protected] (GR)
Affiliations Institute of Biometry and Clinical Epidemiology, Corporate Member of Freie Universität Berlin and Humboldt-Universität zu Berlin, Charité—Universitätsmedizin Berlin, Berlin, Germany, Center for Medical Statistics, Informatics and Intelligent Systems, Section for Clinical Biometrics, Medical University of Vienna, Vienna, Austria

Roles Data curation, Formal analysis, Investigation, Methodology, Writing – review & editing
Affiliations Institute of Biometry and Clinical Epidemiology, Corporate Member of Freie Universität Berlin and Humboldt-Universität zu Berlin, Charité—Universitätsmedizin Berlin, Berlin, Germany, School of Business and Economics, Emmy Noether Group in Statistics and Data Science, Humboldt-Universität zu Berlin, Berlin, Germany
Roles Data curation, Formal analysis, Investigation, Writing – review & editing
Affiliation Institute of Biometry and Clinical Epidemiology, Corporate Member of Freie Universität Berlin and Humboldt-Universität zu Berlin, Charité—Universitätsmedizin Berlin, Berlin, Germany
Roles Validation, Writing – review & editing
Affiliation School of Business and Economics, Emmy Noether Group in Statistics and Data Science, Humboldt-Universität zu Berlin, Berlin, Germany
Roles Conceptualization, Funding acquisition, Project administration, Resources, Supervision, Writing – review & editing
Affiliation Faculty of Medicine and Medical Center, Institute of Medical Biometry and Statistics, University of Freiburg, Freiburg, Germany
Affiliation Department of Biomedical Data Sciences, Leiden University Medical Center, Leiden, The Netherlands
Roles Conceptualization, Funding acquisition, Investigation, Supervision, Validation, Writing – review & editing
Affiliation Center for Medical Statistics, Informatics and Intelligent Systems, Section for Clinical Biometrics, Medical University of Vienna, Vienna, Austria
Roles Conceptualization, Funding acquisition, Investigation, Methodology, Project administration, Resources, Supervision, Validation, Writing – original draft, Writing – review & editing
¶ Membership of the topic group 2 of the STRATOS initiative is listed in the Acknowledgments.
- Christine Wallisch,
- Paul Bach,
- Lorena Hafermann,
- Nadja Klein,
- Willi Sauerbrei,
- Ewout W. Steyerberg,
- Georg Heinze,
- Geraldine Rauch,
- on behalf of topic group 2 of the STRATOS initiative
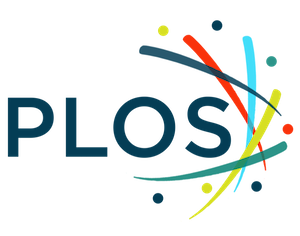
- Published: January 24, 2022
- https://doi.org/10.1371/journal.pone.0262918
- Reader Comments
Although regression models play a central role in the analysis of medical research projects, there still exist many misconceptions on various aspects of modeling leading to faulty analyses. Indeed, the rapidly developing statistical methodology and its recent advances in regression modeling do not seem to be adequately reflected in many medical publications. This problem of knowledge transfer from statistical research to application was identified by some medical journals, which have published series of statistical tutorials and (shorter) papers mainly addressing medical researchers. The aim of this review was to assess the current level of knowledge with regard to regression modeling contained in such statistical papers. We searched for target series by a request to international statistical experts. We identified 23 series including 57 topic-relevant articles. Within each article, two independent raters analyzed the content by investigating 44 predefined aspects on regression modeling. We assessed to what extent the aspects were explained and if examples, software advices, and recommendations for or against specific methods were given. Most series (21/23) included at least one article on multivariable regression. Logistic regression was the most frequently described regression type (19/23), followed by linear regression (18/23), Cox regression and survival models (12/23) and Poisson regression (3/23). Most general aspects on regression modeling, e.g. model assumptions, reporting and interpretation of regression results, were covered. We did not find many misconceptions or misleading recommendations, but we identified relevant gaps, in particular with respect to addressing nonlinear effects of continuous predictors, model specification and variable selection. Specific recommendations on software were rarely given. Statistical guidance should be developed for nonlinear effects, model specification and variable selection to better support medical researchers who perform or interpret regression analyses.
Citation: Wallisch C, Bach P, Hafermann L, Klein N, Sauerbrei W, Steyerberg EW, et al. (2022) Review of guidance papers on regression modeling in statistical series of medical journals. PLoS ONE 17(1): e0262918. https://doi.org/10.1371/journal.pone.0262918
Editor: Tim Mathes, Witten/Herdecke University, GERMANY
Received: June 28, 2021; Accepted: January 8, 2022; Published: January 24, 2022
Copyright: © 2022 Wallisch et al. This is an open access article distributed under the terms of the Creative Commons Attribution License , which permits unrestricted use, distribution, and reproduction in any medium, provided the original author and source are credited.
Data Availability: The data was collected within the review and is available as supporting information S6.
Funding: CW: I-4739-B Austrian Science Fund, https://www.fwf.ac.at/en/ LH: RA 2347/8-1, German Research Foundation, https://www.dfg.de/en/ WS: SA 580/10-1, German Research Foundation, https://www.dfg.de/en/ All funders had no role in study design, data collection and analysis, decision to publish, or preparation of the manuscript.
Competing interests: The authors have declared that no competing interests exist.
Introduction
Knowledge transfer from the rapidly growing body of methodological research in statistics to application in medical research does not always work as it should [ 1 ]. Possible reasons for this problem are the lack of guidance and that not all statistical analyses are conducted by statistical experts but often by medical researchers who may or may not have a solid statistical background. Applied researchers cannot be aware of all statistical pitfalls and the most recent developments in statistical methodology. Keeping up is already challenging for a professional biostatistical researcher, who is often restricted to an area of main interest. Moreover, articles on statistical methodology are often written in a rather technical style making knowledge transfer even more difficult. Therefore, there is a need for statistical guidance documents and tutorials written in more informal language, explaining difficult concepts intuitively and with illustrative educative examples. The international STRengthening Analytical Thinking for Observational Studies (STRATOS) initiative ( http://stratos-initiative.org ) aims to provide accessible and accurate guidance documents for relevant topics in the design and analysis of observational studies [ 1 ]. Guidance is intended for applied statisticians and other medical researchers with varying levels of statistical education, experience and interest. Some medical journals are aware of this situation and regularly publish isolated statistical tutorials and shorter articles or even whole series of articles with the intention to provide some methodological guidance to their readership. Such articles and series can have a high visibility among medical researchers. Although some of the articles are short notes or rather introductory texts, we will use the phrase ‘statistical tutorial’ for all articles in our review.
Regression modeling plays a central role in the analysis of many medical studies, in particular, of observational studies. More specifically, regression model building involves aspects such as selection of a model type that matches the type of outcome variable, selection of explanatory variables to include in a model, choosing an adequate coding of the variables, deciding on how flexibly the association of continuous variables with the outcome should be modeled, planning and performing model diagnostics, model validation and model revision, reporting of a model and describing how well differences in the outcome can be explained by differences in the covariates. Some of the choices made during model building will strongly depend on the aim of modeling. Shmueli (2010) [ 2 ] distinguished between three conceptual modeling approaches: descriptive, predictive and explanatory modeling. In practice these aims are still often not well clarified, leading to confusion about which specific approach is useful in a modeling problem at hand. This confusion, and an ever-growing body of literature in regression modeling may explain why a common state-of-the-art is still difficult to define [ 3 ]. However, not all studies require an analysis with the most advanced techniques and there is the need for guidance for researchers without a strong background in statistical methodology, who might be “medical students or residents, or epidemiologists who completed only a few basic courses in applied statistics” according to the definition of level-1 researchers by the STRATOS initiative [ 1 ].
If suitable guidance for level-1 researchers in peer-reviewed journals was available, many misconceptions about regression model building could be avoided [ 4 – 6 ]. The researchers need to be informed about methods that are easily implemented, and they need to know about strengths and weaknesses of common approaches [ 3 ]. Suitable guidance should also point to possible pitfalls, elaborate on dos and don’ts in regression analyses, and provide software recommendations and understandable code for different methods and aspects. In this review, we focused on low-dimensional regression models where the sample size exceeds the number of candidate predictors. Moreover, we will not specifically address the field of causal inference, which goes beyond classical regression modeling.
So far, it is unclear what aspects of regression modeling have already been well-covered by related tutorials and where gaps still exist. Furthermore, suitable tutorial papers may be published but they are unknown to (nearly all) clinicians and therefore widely ignored in their analyses.
The objective of this review was to provide an evidence-based information basis assessing the extent to which regression modeling has been covered by series of statistical tutorials published in medical journals. Specifically, we sought to define a catalogue of important aspects on regression modeling, to identify series of statistical tutorials in medical journals, and to evaluate which aspects were treated in the identified articles and at which level of sophistication. Thereby, we put an intended focus on the choice of the regression model type, on variable selection and for continuous variables on the functional form. Furthermore, this paper will provide an overview, which helps to inform a broad audience of medical researchers about the availability of suitable papers written in English.
The remainder of this review is organized as follows: In the next section, the review protocol is described. Subsequently, we summarize the results of the review by means of descriptive measures. Finally, we discuss implications of our results suggesting potential topics for future tutorials or entire series.
Material and methods
The protocol of this review describing the detailed design was already published by Bach et al. (2020) [ 7 ]. In here, we summarize its main characteristics.
Eligibility criteria
First, we identified series of statistical tutorials and papers published in medical journals with a target audience mainly or exclusively consisting of medical researchers or practitioners. Second, we searched for topic-relevant articles on regression modeling within these series. Journals with a target audience of pure theoretical, methodological or statistical focus were not considered. We included medical journals if they were available in English language since this implies high international impact and broad visibility. Moreover, the series had to comprise at least five or more articles including at least one topic-relevant article. We focused on statistical series only since we believed that entire series have higher impact and visibility than isolated articles.
Sources of information & search strategy
After conducting a pilot study for a systematic search for series of statistical tutorials, we had to adapt our search strategy since sensitive keywords to identify statistical series could not be found. Therefore, we consulted more than 20 members of the STRATOS initiative via email in spring 2018 for suggestions on statistical series addressing medical researchers. We also asked them to forward this request to colleagues, which resembles snowball sampling [ 8 , 9 ]. This call was repeated at two international STRATOS meetings in summer 2018 and in 2019. The search was closed on June 30 st , 2019. Our approach also included elements of respondent-driven sampling [ 10 ] by offering collaboration and co-authorship in case of relevant contribution to the review. In addition, we included several series that were additionally proposed by a reviewer during the peer-review process of this manuscript, and which were published by the end of June, 2019 to be consistent with the original request.
Data management & selection process
The list of all resulting statistical series suggested is available as S1 File .
Two independent raters selected relevant statistical series from the pool of candidate series by applying the inclusion criteria outlined above.
An article within a series was considered to be topic-relevant if the title included one of the following keywords: regression , linear , logistic , Cox , survival , Poisson , multivariable , multivariate , or if the title suggested that the main topic of the article was statistical regression modeling . Both raters decided on the topic-relevance of an article independently and resolved discrepancies by discussion. To facilitate the selection of relevant statistical series, we designed a report form called inclusion form ( S2 File ).
Data collection process
After the identification of relevant series and topic-relevant articles, a content analysis was performed on all topic-relevant articles using an article content form ( S3 File ). The article content form was filled-in for every identified topic-relevant article by the two raters independently and again discrepancies were resolved by discussion. The results of completed article content forms were copied into a data base for further quantitative analysis.
In total 44 aspects of regression modeling were examined in the article content form ( S3 File ), which were related to four areas: type of regression model , general aspects of regression modeling , functional form of continuous predictors , and selection of variables . The 44 aspects cover topics of different complexity. Some aspects can be considered basic, others are more advanced. This was also commented in the S3 File for orientation. We mainly focused on predictive and descriptive models and did not consider particular aspects attributed to ethological models.
For each aspect, we evaluated whether it was mentioned at all, and if yes, the extent of explanation (short = one sentence only / medium = more than one sentence to one paragraph / long = more than one paragraph) [ 7 ]. We recorded whether examples and software commands were provided, and if recommendations or warnings were given with respect to each aspect. A box for comments provided space to note recommendations, warnings and other issues. In the article content form, it was also possible to add further aspects to each area. A manual for raters was created to support an objective evaluation of the aspects ( S4 File ).
Summary measures & synthesis of results
This review was designed as an explorative study and uses descriptive statistics to summarize results. We calculated absolute and relative frequencies to analyze the 44 statistical aspects. We used stacked bar charts to describe the ordinal variable extent of explanation for each aspect. To structure the analysis, we grouped the aspects into the afore mentioned areas: type of regression model , general aspects of regression modeling , determination of functional form for continuous predictors and selection of variables .
We conducted the above analyses both article-wise and series-wise. In the article-wise analysis, each article was considered individually. For the series-wise analysis, the results from all articles in a series were pooled and each series was considered as the unit of observation. This means, if an aspect was explained in at least one article, this also counted for the entire series.
Risk of bias
The risk of bias by missing a series was addressed extensively in the protocol of this study [ 7 , 11 , 12 ]. Moreover, bias could result from the inclusion criterion of series, which was the requirement of at least five articles in a series. This may have led to a less representative set of series. We set this inclusion criterion to identify highly visible series. Bias could also result from the specific choice of aspects of regression modeling to be screened. We tried to minimize this bias by the possibility for free text entries that could later be combined into additional aspects.
This review has been written according to the PRISMA reporting guideline [ 13 , 14 ], compare S1 Checklist . This review does not include patients or humans. The data that were collected within the review are available in S1 Data .
Selection of series and articles
The initial query revealed 47 series of statistical tutorials ( Fig 1 and S1 File ). Out of these 47 series, two series were not published in a medical journal and five series did not target an audience with low statistical knowledge. Therefore, these series were excluded. Five and ten series were excluded because they were not written in English or they did not comprise at least five articles, respectively. Further, we excluded three series because they did not contain any topic-relevant article. The list of the series and the reason for each excluded series is found in S1 File . Finally, we included 23 series with 57 topic-relevant articles.
- PPT PowerPoint slide
- PNG larger image
- TIFF original image
https://doi.org/10.1371/journal.pone.0262918.g001
Characteristics of the series
Each series contained between one to nine topic-relevant articles (two on average, Table 1 ). The variability of the average number of article pages per series illustrates that the extent of the articles was very different (1 to 10.3 pages). Whereas the series Statistics Notes in the BMJ typically used a single page to discuss a topic, hence pointing only to the most relevant issues, there were longer papers with a length of up to 16 pages [ 15 , 16 ]. The series in the BMJ is also the one spanning over the longest time period (1994–2018). Beside of the series in the BMJ , only the Archives of Disease in Childhood and the Nutrition series started publishing papers already in the last century. Fig 2 shows that most series were published only during a short period, perhaps paralleling terms of office of an Editor.
https://doi.org/10.1371/journal.pone.0262918.g002
We considered 44 aspects, see S3 File .
https://doi.org/10.1371/journal.pone.0262918.t001
The most informative series with respect to our pre-specified list of aspects was published in Revista Española de Cardiologia , which mentioned 35 aspects in two articles on regression modeling ( Table 1 ). Similarly, Circulation and Archives of Disease in Childhood covered 31 and 30 aspects in three article each. The number of articles and the years of publication varied across the series ( Fig 2 ). Some series comprised only five articles whereas Statistics Notes of the BMJ published 68 short articles, which was very successful with some articles that were cited about 2000 times. Almost all series covered multivariable regression in at least one article. The range of regression types varied across series. Most statistical series were published with the intention to improve the knowledge of their readership about how to apply appropriate methodology in data analyses and how to critically appraise published research [ 17 – 19 ].
Characteristics of articles
The top three articles that covered the highest number of aspects (27 to 34 out of 44 aspects) on six to seven pages were published in Revista Española de Cardiologia , Deutsches Ärzteblatt International , and in European Journal of Cardio-Thoracic Surgery [ 20 – 22 ]. The article of Nuñez et al. [ 22 ] published in Revista Española de Cardiologia covered the most popular regression types (linear, logistic and Cox regression) and explained not only general aspect but also gave insights into non-linear modeling and variable selection. Schneider et al. [ 20 ] covered all regression types that we considered in our review in their publication in Deutsches Ärzteblatt International . The top-ranked article in European Journal of Cardio-Thoracic Surgery [ 21 ] particularly focused on the development and validation of prediction models.
Explanation of aspects in the series
Almost all statistical series included at least one article that mentioned or explained multivariable regression ( Table 1 ). Logistic regression was the most frequently described regression type in 19 out of 23 series (83%), followed by linear regression (78%). Cox regression/survival model (including proportional hazards regression) was mentioned in twelve series (52%) and was less extensively described than linear and logistic regression. Poisson regression was covered by three series (13%). Each of the considered general aspects of regression modeling were mentioned in at least four series (17%) ( Fig 3 ) except for random effect models , which were treated in only one series (4%). Interpretation of regression coefficients , model assumptions , and different purposes of regression mode were covered in 19 series (83%). The aspect different purposes of regression models comprised at least one statement in an article concerning purposes of regression models, which could be identified by keywords like prediction, description, explanation, etiology, or confounding. More than one sentence was used for the explanation of different purposes in 15 series (65%). In 18 series (78%), reporting of regression results and regression diagnostics were described, which was done extensively in most series. Aspects like treatment of binary covariates , missing values , measurement error , and adjusted coefficient of determination were rather infrequently mentioned and found in four to seven series each (25–30%).
Extent of explanation of general aspects of regression modeling in statistical series: One sentence only (light grey), more than one sentence to one paragraph (grey) and more than one paragraph (black).
https://doi.org/10.1371/journal.pone.0262918.g003
At least one aspect of functional forms of continuous predictors , was mentioned in 17 series (74%), but details were hardly ever given ( Fig 4 ). The possibility of non-linear relation and non-linear transformations were raised in 16 (70%) and eleven series (48%), respectively. Dichotomization of continuous covariates was found in eight series (35%) and it was extensively discussed in two (9%). More advanced techniques like the use of splines or fractional polynomials were mentioned in some series but detailed information for splines was not provided. Generalized additive models were never mentioned.
Extent of explanation of aspects of functional forms of continuous predictors in statistical series: One sentence only (light grey), more than one sentence to one paragraph (grey) and more than one paragraph (black).
https://doi.org/10.1371/journal.pone.0262918.g004
Selection of variables was mentioned in 15 series (65%) and described extensively in ten series (43%) ( Fig 5 ). However, specific variable selection methods were rarely described in detail. Backward elimination , selection based on background knowledge , forward selection , and stepwise selection were the most frequently described selection methods in seven to eleven series (30–48%). Univariate screening , which is still popular in medical research, was only described in three series (13%) in up to one paragraph. Other aspects of variable selection were hardly ever mentioned. Selection based on AIC/BIC , relating to best subset selection or stepwise selection based on these information criteria, and the choice of the significance level were found in 2 series only (9%). Relative frequencies of aspects mentioned in articles are detailed in Figs 1 – 3 in S5 File .
Extent of explanation of aspects of selection of variables in statistical series: One sentence only (light grey), more than one sentence to one paragraph (grey) and more than one paragraph (black).
https://doi.org/10.1371/journal.pone.0262918.g005
We found general recommendations for software in nine articles of nine different series. Authors mentioned R, Nanostat, GLIM package, SAS and SPSS [ 75 – 78 ]. SAS as well as R were recommended in three articles. In only one article the authors referred to a specific package in R. Detailed code examples were provided in two articles only [ 16 , 58 ]. In the article of Curran-Everett [ 58 ], the R script file was provided as appendix and in the article of Obuchowski [ 16 ], code chunks were included throughout the text directly showing how to derive the reported results. In all, software recommendations were rare and mostly not detailed.
Recommendations and warnings in the series
Recommendations and warnings were given on many aspects of our list. All statements are listed in S5 File : Table 1 and some frequent statements across articles are summarized below.
Statements on general aspects
We found numerous recommendations and warnings on general aspects as described in the following. Concerning data preparation, some authors recommended to impute missing values in multivariable models, e.g. by multiple imputation [ 20 – 22 , 31 ]. Steyerberg et al. [ 31 ] and Grant et al. [ 21 ] discouraged from using a complete case analysis to handle missing values. As an aspect of model development, number of observations/events per variable was a disputed topic in several articles [ 79 – 81 ]. In seven articles, we found explicit recommendations for the number of observations (in linear models) or the events per variable (in logistic and Cox/survival models), varying between at least ten to 20 observations/events per variable [ 16 , 20 , 22 , 25 , 31 , 33 , 55 ]. Several recommendations and warnings were given on model assumptions and model diagnostics . Many series authors recommended to check assumptions graphically [ 24 , 27 , 44 , 58 , 72 ] and they warned that models may be inappropriate if the assumptions are not met [ 20 , 24 , 31 , 33 , 52 , 55 , 56 , 62 ]. In the context of Cox proportional hazards model, authors especially mentioned the proportional hazards assumption [ 24 , 44 , 49 , 56 , 62 ]. Concerning reporting of results, some authors warned to not confuse odds ratios with relative risks or hazard ratios [ 25 , 44 , 59 ]. Several warnings could also be found on reporting performance of a model. Most authors did not recommend to report the coefficient of determination R 2 [ 20 , 27 , 51 , 61 ] and indicated that the pitfall of R 2 is that its value increases with increasing number of covariates in the model [ 15 ]. Schneider et al. [ 20 ] and Richardson et al. [ 61 ] recommended to use the adjusted coefficient of determination instead. We also found many recommendations and statements about model validation for prediction models. Authors of the evaluated articles recommended cross-validation or bootstrap validation instead of split sample validation if internal validation is performed [ 21 , 22 , 31 , 70 , 72 ]. It was also suggested that internal validation is not sufficient for the model to be used in clinical practice and an external validation should be executed as well [ 21 ]. In several articles, we found that authors warned about applying the Hosmer-Lemeshow test because of potential pitfalls [ 31 , 60 , 61 ]. For reporting regression results , in two articles the guideline for Transparent Reporting of multivariable prediction models for Individual Prognosis or Diagnosis (TRIPOD) was mentioned [ 21 , 71 , 82 ].
Statements on functional form of continuous predictors
Dichotomization of continuous predictors is an aspect of functional forms of continuous predictors that was frequently discussed. Many authors argued against categorization of continuous variables because it may lead to loss of power, to increased risk of false positive results, to underestimation of variation, and to concealment of non-linearities [ 21 , 26 , 31 , 69 ]. However, other authors advised to categorize continuous variables if the relation to the outcome is non-linear [ 24 , 25 , 59 ].
Statements on variable selection
We also found recommendations in favor of or against specific variable selection methods. Four articles explicitly recommended to take advantage of background knowledge to select variables [ 15 , 20 , 48 , 59 ]. Univariate screening was advised against by one article [ 19 ]. Comparing stepwise selection methods, Grant et al. [ 21 ] preferred backward elimination over forward selection. Authors warned about consequences of stepwise methods such as unstable selection and overfitting [ 21 , 31 ]. It was also pointed out that selected models must be interpreted with greatest caution and implications should be checked on new data [ 28 , 53 ].
Methodological gaps in the series
This descriptive analysis of contents gives rise to some observations on important gaps and possibly misleading recommendations. First, we found that one general type of regression models, Poisson regression, was not treated in most series. This omission is probably due to the fact that Poisson regression is less frequently applied in medical research because most outcomes are binary or time-to-event and, therefore, logistic and Cox regression are more frequent. Second, several series introduced the possibility of non-linear relations of continuous covariates with the outcome. However, only few statements on how to deal with non-linearities by specifying flexible functional forms in multiple regression were available. Third, we did not find very detailed information on advantages and disadvantages of data-driven variable selection methods in any of the series. Finally, tutorials on statistical software and on specific code examples were hardly found in the reviewed series.
Misleading recommendations in the series
Quality assessment of recommendations would have been controversial and we did not intend doing it. Nevertheless, here we mention two issues that we consider as severely misleading. Although univariate screening as a method for variable selection was never recommended in any of the series, one article showed an example with the application of this procedure to pre-filter the explanatory variables based on their associations with the outcome variable [ 47 ]. It is known since long that univariate screening should be avoided because it has the potential to wrongly reject important variables [ 83 ]. In another article it was suggested that a model can be considered robust if results from both backward elimination and forward selection agree [ 20 ]. Such agreement does not support robustness of stepwise methods: relying on agreement is a poor strategy [ 84 , 85 ].
Series and articles recommended to read
Depending on the aim of the planned study, as well as the focus and knowledge level of the reader, different series and articles might be recommended. The series in Circulation comprised three papers about multiple linear and logistic regression [ 24 – 26 ], which provide basics and describe many essential aspects of univariable and multivariable regression modeling. For more advanced researchers, we recommend the article of Nuñ ez et al. in Revista Española de Cardiologia [ 22 ], which gives a quick overview of aspects and existing methods including functional forms and variable selection. The Nature Methods series published short articles focusing on few, specific aspects of regression modeling [ 34 – 42 ]. This series might be of interest if one likes to spent more time on learning about regression modeling. If someone is especially interested in prediction models, we recommend a concise publication in the European Heart Journal [ 31 ], which provides details on model development and validation for predictive purposes. For the same topic we can also recommend the paper by Grant et al. [ 21 ]. We consider all series and articles recommended in this paragraph as suitable reading for medical researchers but this does not imply that we agree to all explanations, statements and aspects discussed.
Summary and consequences for future work
This review summarizes the knowledge about regression modeling that is transferred through statistical tutorials published in medical journals. A total of 23 series with 57 topic-relevant articles were identified and evaluated for coverage of 44 aspects of regression modeling. We found that almost all aspects of regression modeling were at least mentioned in any of the series. Several aspects of regression modeling, in particular most general aspects, were covered. However, detailed descriptions and explanations of non-linear relations and variable selection in multivariable models were lacking. Only few papers provided suitable methods and software guidance for analysts with a relatively weak statistical background and limited practical experience as recommended by the STRATOS initiative [ 1 ]. However, we confess that currently there is no agreement on state of the art methodology [ 3 ].
Nevertheless, readers of statistical tutorials should not only be informed about the possibility of non-linear relations of continuous predictors with the outcome but they should also be given a brief overview about which methods are generally available and may be suitable. This could be achieved by tutorials that introduce readers to methods like fractional polynomials or splines, explaining similarities and differences between these approaches, e.g., by comparative, commented analyses of realistic data sets. Such documents could also show how alternative analyses (considering/ignoring potential non-linearities) may result in conflicting results and explain the reasons for such discrepancies.
Detailed tutorials on variable selection could aim at describing the mechanism of different variable selection methods, which can easily be applied with standard statistical software, and should state in what situations variable selection methods are needed and could be used. For example, if sufficient background knowledge is available, prefiltering or even the selection of variables should be based on this information rather than using data-driven methods on the entire data set. Such tutorials should provide comparisons and interpretation of the results of various variable selection methods and suggest adequate methods for different data settings.
Generally, the articles also lacked details on software to perform statistical analysis and usually did not provide code chunks, descriptions of specific functions, an appendix with commented code or references to software packages. Future work should also focus on filling this gap by recommendations of software as well as providing well commented and documented code for different statistical methods in a format that is accessible by non-experts. We recommend that software, packages and functions therein to apply certain methods should be reported in every statistical tutorial article. The respective code to derive analysis results could be provided in an appendix or directly in the manuscript text, if not too lengthy. Any provided code in the appendix should be well-structured and lavishly commented referring to the particular method and describing all defined parameter settings. This will encourage medical researchers to increase the reproducibility of their research by also publishing their statistical code, e.g., in electronic appendices to their publications. For example, worked examples with openly accessible data sets and commented code allowing fully reproducible results have a high potential to guide researchers in their own statistical tasks. On the contrary, we discourage from using point-and-click software programs, which sometimes output far more analysis results than requested. Users may pick inadequate methods or report wrong results inadvertently, which could debilitate their research work.
Generally, our review may stimulate the development of targeted gap-filling guidance and tutorial papers in the field of regression modeling, which should support medical researchers in several ways: 1) by explaining how to interpret published results correctly, 2) by guiding them how to critically appraise the methodology used in a published article, 3) by enabling them to plan, perform basic statistical analyses and report results in a proper way and 4) by helping them to identify situations in which the advice of a statistical expert is required. In S3 File : CRF article screening we commented which aspects should usually be addressed by an expert and which aspects are considered basic.
Strengths and limitations
According to our knowledge this is the first review on series of statistical tutorials in the medical field with the focus on regression modeling. Our review followed a pre-specified and published protocol to which many experienced researchers in the field of applied regression modeling contributed. One aspect of this contribution was the collection of series of statistical tutorials that could not be identified by common keyword searches.
We standardized the selection process by designing an inclusion checklist for series of statistical tutorials and by providing a manual for the content form with which we extracted the actual information of the article and series. Another strength is that the data collection process was performed objectively since each article was analyzed by two out of three independent raters. Discrepancies were discussed among all three of them to find a consent. This procedure avoided that single opinions were transferred to the output of this review. This review is informative for many clinical colleagues who are interested in statistical issues in regression modeling and search for suitable literature.
This review also has limitations. An automated, systematic search was not possible because series could not be identified by common keywords neither on the series’ title level nor on the article’s title level. Thus, not all available series may have been found. To enrich our initial query, we also searched on certain journals’ webpages and requested our expert panel from the STRATOS initiative to complement our list with other series they were aware of. We also included series that were suggested by one reviewer during the peer-review procedure of this manuscript. This selection strategy may impose a bias towards higher-quality journals since series of less prestigious journals might not be known to the experts. However, the higher-quality journals can be considered as the primary source of information for researchers seeking advice on statistical methodology.
We considered only series with at least five articles. This boundary is of course to a certain extend arbitrary. It was motivated by the fact that we intended to do analyses on the series level, which is only reasonable if a series covers an adequate number of articles. We also assumed that larger series are more visible and well-known to researchers.
We also might have missed or excluded some important aspects of regression modeling in our catalogue. The catalogue of aspects was developed and discussed by several experienced researchers of the STRATOS initiative working in the field of regression modeling. After submission of the protocol paper some more aspects were added on request of its reviewers [ 7 ]. However, further important aspects such as meta-regression, diagnostic models, causal inference, reproducibility or open data and open software code were not addressed. We encourage researchers to repeat similar reviews on these related fields.
A third limitation is that we only searched for series whereas there might be other educational papers on regression modeling that were published as single articles. However, we believe that the average visibility of an entire series and thereby its educational impact is much higher than for isolated articles. This does not negate that there could be excellent isolated articles, which can have a high impact for training medical researchers. While working on the final version of this paper we became aware of the series Big-data Clinical Trial Column in the Annals of Translational Medicine . Until 1 January 2019 they had published 36 papers and the series would have been eligible for our review. Obviously, we might have overseen further series, but it is unlikely that it has a larger effect on the results of our review.
Moreover, there are many introductory textbooks, educational workshops and online video tutorials, some of them with excellent quality, which were not considered here. A detailed review of such sources clearly was out of our scope.
Despite many series of statistical tutorials being available to guide medical researchers on various aspects of regression modeling, several methodological gaps still persist, specifically on addressing nonlinear effects, model specification and variable selection. Furthermore, papers are published in a large number of different journals and are therefore likely unknown to many medical researchers. This review fills the latter gap, but many more steps are needed to improve the quality and interpretation of medical research. More detailed statistical guidance and tutorials with a low technical level on regression modeling and other topics are needed to better support medical researchers who perform or interpret regression analyses.
Supporting information
S1 checklist. prisma reporting guideline..
https://doi.org/10.1371/journal.pone.0262918.s001
S1 File. List of candidate series for potential inclusion in the review.
https://doi.org/10.1371/journal.pone.0262918.s002
S2 File. Case report form–series inclusion.
https://doi.org/10.1371/journal.pone.0262918.s003
S3 File. Case report form–article screening.
https://doi.org/10.1371/journal.pone.0262918.s004
S4 File. Manual for the article screening sheet.
https://doi.org/10.1371/journal.pone.0262918.s005
S5 File. Supplementary figures and tables.
https://doi.org/10.1371/journal.pone.0262918.s006
S1 Data. Collected data.
https://doi.org/10.1371/journal.pone.0262918.s007
Acknowledgments
When this article was written, topic group 2 of STRATOS consisted of the following members: Georg Heinze (Co-chair, [email protected] ), Medical University of Vienna, Austria; Willi Sauerbrei (co-chair, [email protected] ), University of Freiburg, Germany; Aris Perperoglou (co-chair, [email protected] ), AstraZeneca, London, Great Britain; Michal Abrahamowicz, Royal Victoria Hospital, Montreal, Canada; Heiko Becher, Medical University Center Hamburg, Eppendorf, Hamburg, Germany; Harald Binder, University of Freiburg, Germany; Daniela Dunkler, Medical University of Vienna, Austria; Rolf Groenwold, Leiden University, Leiden, Netherlands; Frank Harrell, Vanderbilt University School of Medicine, Nashville TN, USA; Nadja Klein, Humboldt Universität, Berlin, Germany; Geraldine Rauch, Charité–Universitätsmedizin Berlin, Germany; Patrick Royston, University College London, Great Britain; Matthias Schmid, University of Bonn, Germany.
We thank Edith Motschall (Freiburg) for her important support in the pilot study where we tried to define keywords for identifying statistical series within medical journals. We thank several members of the STRATOS initiative for proposing a high number of candidate series and we thank Frank Konietschke for English language editing in our protocol.
- View Article
- PubMed/NCBI
- Google Scholar
- 75. R Core Team. R: A Language and Environment for Statistical Computing. Vienna, Austria: R Foundation for Statistical Computing; 2021.
- 77. SAS Institute Inc. The SAS system for Windows. Release 9.4. Cary, NC: SAS Institute Inc.; 2021.
- 78. IBM Corporation. IBM SPSS Statistics for Windows, Version 27.0. Armonk, NY: IBM Corporation; 2020.
IEEE Account
- Change Username/Password
- Update Address
Purchase Details
- Payment Options
- Order History
- View Purchased Documents
Profile Information
- Communications Preferences
- Profession and Education
- Technical Interests
- US & Canada: +1 800 678 4333
- Worldwide: +1 732 981 0060
- Contact & Support
- About IEEE Xplore
- Accessibility
- Terms of Use
- Nondiscrimination Policy
- Privacy & Opting Out of Cookies
A not-for-profit organization, IEEE is the world's largest technical professional organization dedicated to advancing technology for the benefit of humanity. © Copyright 2024 IEEE - All rights reserved. Use of this web site signifies your agreement to the terms and conditions.
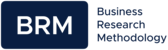
Regression Analysis
Regression analysis is a quantitative research method which is used when the study involves modelling and analysing several variables, where the relationship includes a dependent variable and one or more independent variables. In simple terms, regression analysis is a quantitative method used to test the nature of relationships between a dependent variable and one or more independent variables.
The basic form of regression models includes unknown parameters (β), independent variables (X), and the dependent variable (Y).
Regression model, basically, specifies the relation of dependent variable (Y) to a function combination of independent variables (X) and unknown parameters (β)
Y ≈ f (X, β)
Regression equation can be used to predict the values of ‘y’, if the value of ‘x’ is given, and both ‘y’ and ‘x’ are the two sets of measures of a sample size of ‘n’. The formulae for regression equation would be
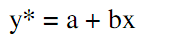
Do not be intimidated by visual complexity of correlation and regression formulae above. You don’t have to apply the formula manually, and correlation and regression analyses can be run with the application of popular analytical software such as Microsoft Excel, Microsoft Access, SPSS and others.
Linear regression analysis is based on the following set of assumptions:
1. Assumption of linearity . There is a linear relationship between dependent and independent variables.
2. Assumption of homoscedasticity . Data values for dependent and independent variables have equal variances.
3. Assumption of absence of collinearity or multicollinearity . There is no correlation between two or more independent variables.
4. Assumption of normal distribution . The data for the independent variables and dependent variable are normally distributed
My e-book, The Ultimate Guide to Writing a Dissertation in Business Studies: a step by step assistance offers practical assistance to complete a dissertation with minimum or no stress. The e-book covers all stages of writing a dissertation starting from the selection to the research area to submitting the completed version of the work within the deadline. John Dudovskiy
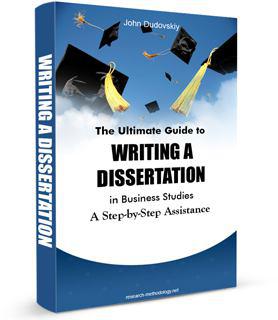
Robust Regression Analysis in Analyzing Financial Performance of Public Sector Banks: A Case Study of India
- Published: 01 July 2022
- Volume 11 , pages 677–691, ( 2024 )
Cite this article
- Asif Pervez 1 &
- Irfan Ali ORCID: orcid.org/0000-0002-1790-5450 2
5173 Accesses
3 Citations
Explore all metrics
Regression analysis is a statistical method to analyze financial data, commonly using the least square regression technique. The regression analysis has significance for all the fields of study, and almost all the fields apply least square regression methods for data analysis. However, the ordinary least square regression technique can give misleading and wrong results in the presence of outliers and influential observations in the data. Robust estimation is a statistical method to analyze such financial data with outliers. It is an alternative method for the least square regression for such data. It is necessary to elaborate on the applications of the robust regression model in analyzing real-world financial data that do not fulfil the assumptions of most statistical methods of data analysis to get reliable results. Public sector banks are the backbone of the Indian financial system. The present study analyzed the financial performance of public sector banks in India by applying a robust linear regression technique for more reliable outcomes. Twenty-one public sector banks were selected to study based on the importance of public sector banks in India.
Similar content being viewed by others
A Robust Version of the FGLS Estimator for Panel Data
Rise of the Partial Least Squares Structural Equation Modeling: An Application in Banking
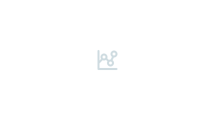
GCC banks liquidity and financial performance: does the type of financial system matter?
Avoid common mistakes on your manuscript.
1 Introduction
Analyses of real-world financial and economic data are a great challenge due to their high volatility, vastness and other economic and political factors. Recently, the covid-19 pandemic has also caused a drastic change in the financial data [ 1 ]. Data science is the study of analysing these large volumes of data by employing various modern methods of data analyses like complex machine learning algorithms to get meaningful information which helps in efficient and timely decision making. Several methods can be employed to analyze the financial data in this context. Optimization based Data Mining mainly focuses on Multiple Criteria Programming, and Support Vector Machines also have applications in various including finance [ 2 , 3 ]. Data mining can also be used to analyze enormous amounts of information and datasets, which can help business organizations to solve various managerial problems, manage risks, and find opportunities [ 4 ]. A hybrid intelligent model was proposed for forecasting stock market prediction based on news using the psycholinguistic variables [ 5 ].
Regression estimation is an important statistical method used in analyzing financial data, which often applies the Ordinary Least Square (OLS) method of regression to analyze such data. However, the necessary assumption in regression analysis that as normality, homoscedasticity, multicollinearity etc., presence of outliers and influential observations makes the least square regression analysis vulnerable to such data and may give misleading and wrong results. The robust alternatives, such as Least Median Squares estimators (LMS), Least Trimmed Squares estimators (LTS), and M estimators, can tolerate up to a certain level of contaminations [ 6 ]. The significance of the predictors changes significantly due to the removal of outliers from the data [ 7 ]. Real-world financial data, which is generally collected for a continuous period, shows a correlation that makes the errors in the regression model interdependent and rejects the assumption of independent errors. It may give an inflated R-square and erroneous significance level of the regression model. The assumption of normality is also essential for most statistical data analysis methods.
The deviation in financial data because of changes in financial policies and commercial cycles gives rise to outliers in the data. In cases when economic factors vary greatly, robust regression methods represent an acceptable and useful analytic tool [ 8 ]. Ordinary least-squares (OLS) estimators for a linear model are very sensitive to unusual values in the design space [ 9 ]. These are the observations that are not fitted to the pattern developed for the majority of observations in the data. According to many statisticians, robust estimation is required to analyze the data with outliers. Therefore, it is necessary to employ such regression analysis, which is not influenced by such data and gives unbiased results in financial data analysis. Robust regression is recommended to get more precise financial data analysis results. The robust regression is a good substitution for the least square regression for these data.
The study aims to elaborate on the applications of the robust regression model in analyzing real-world financial data, which does not fulfil the assumptions of most of the statistical methods of data analysis.
2 Scope of the Study
The present study analyses India's public sector banks’ financial performance by applying a robust linear regression technique. Twenty-one public sector banks were selected to study based on the importance of public sector banks in India. The study was conducted for a period from 2004–05 to 2017–18. The study is confined to analyzing the performance of public sector banks operating in India with the help of robust regression analysis.
3 Data and Methodology
The present study is descriptive as well as analytical in nature. Research is the systematic method of pronouncing the problem, formulating the hypotheses, collecting and analyzing the data and concluding the results.
3.1 Data Source and sample
The present study is mainly based on secondary data on selected parameters of the public sector banks operating in India. The Reserve Bank of India database has been used as the main source of secondary data for extracting data on selected parameters of the public sector from 2005 to 2018. Twenty-one public sector banks that were operating in India from 2005 to 2018 and whose data were available for all the selected parameters were selected for the present study.
3.2 Regression analysis
Regression analysis is an important statistical technique for estimating the impact of independent variables on a dependent variable in the field of finance and economics, among others. A general equation for the regression model is:
where Y is the dependent variable, ε is the vector of true residuals n × 1, and X is n × p in the design matrix. The estimate of β is \(\hat{\beta }\) .
The least-squares method is generally used to estimate parameters in regression analysis based on certain assumptions like the normality of errors ( ε ∼ N (0, δ 2). This method is susceptible to outliers and can provide misleading results if the assumption of normality is not fulfilled or with the presence of outliers as outliers in the data drag the least square fit towards itself. Outliers in the data are influential to the least square method of regression. A diagnostic approach can be used to identify and remove it from the data. However, a robust regression method can detect outliers even in complex data and give efficient results.
3.3 Robust Linear Regression Methods
There are various robust regression techniques. The first step in this respect came from Edge verst (1887), who proposed the least absolute deviation (LAD) by minimizing the sum of absolute residuals instead of minimizing the sum of squared residuals.
Another popular approach is M-estimator by Huber [ 10 ]. The M-estimator replaces the squared residuals by a function of the residuals \(r_{i}^{2}\) . Huber Gave the asymptotic theory of estimating a location parameter for contaminated normal distribution and exhibits asymptotically most robust estimators among all translation, invariant estimators.
M-estimate of β is
where \(\rho \left( . \right)\) a robust loss is function and \(\hat{\sigma }\) is an error scale estimate.
The Least Median Square estimates by Siegel [ 11 ] are found by minimizing the median of the squared residuals. Repeated median estimates maintain the high 50% breakdown value, unlike many generalizations of the univariate median. It can resist the effects of outliers even when they comprise nearly half of the data. Repeated median estimates are unbiased and Fisher consistent for bivariate linear regression with symmetric errors.
There are some other robust procedures, LTS estimates by Rousseeuw [ 12 ].
where \(r_{i} (\beta )^{2} \le . . . \le r_{q} (\beta )^{2}\) are ordered squared residuals, \({\text{q }} = \, \left[ {{\text{n}}\left( {{1} - \alpha } \right) \, + { 1}} \right],\) and α is proportion of trimming.
Other robust estimators include S-estimator and MM-estimators. S-estimates by Rousseeuw and Yohai, [ 13 ]
where, \(r_{1} \left( \beta \right) = y_{i} - x_{i}^{T} \beta\) .
Yohai [ 14 ] proposed a class of robust estimates for the linear model called MM-estimates, one of the most commonly employed robust regression techniques. These estimates are efficient when the errors have a normal distribution and a breakdown point of 0.5. In a three-stage procedure, an initial regression estimate is computed in the first stage, not necessarily efficient, but consistent robust with a high breakdown point. It is followed by the computation of an M-estimate of the scale of the errors with the help of residuals based on the initial estimate in the second stage. At last, an M-estimate of the regression parameters is computed based on a proper redescending psi-function in the third stage. A convergent iterative numerical algorithm is given. Then a comparison is made of asymptotic biases under contamination of optimal bounded influence estimates and MM-estimates.
The R-estimate proposed by Jackel [ 15 ] minimizes the sum of some scores of the ranked residuals.
where Ri is the rank of the ith residual ri, and an is a monotone score function that satisfies:
Gervini and Yohai (2002) proposed a new class of robust regression methods called robust and efficient weighted least squares estimator (REWLSE). The weights are adaptively computed using the empirical distribution of the residuals of an initial robust estimator.
A large value of \(\left| {r_{i} } \right|\) would suggest that \(\left( {x_{i } , y_{i} } \right)\) is an outlier.
She and Owen [ 16 ] proposed a new class of robust regression, the mean shift model for linear regression. One mean shift parameter was added for each n data point, and regularization was applied to favour a sparse vector of mean shift parameters. Thresholding (denoted by Θ) based iterative procedure for outlier detection (Θ–IPOD) was introduced. They proposed a method with one tuning parameter to identify outliers and estimate regression coefficients. A general framework for the inclusion of case-specific parameters in regularization problems, describing the impact on the effective loss for a variety of regression and classification problems is as:
where, \({\text{y}} = \left( {{\text{y}}_{{1}} , \ldots ,{\text{ y}}_{{\text{n}}} } \right)^{{\text{T}}} ,{\text{ x}} = \left( {{\text{x}}_{{1}} , \ldots \,,{\text{ x}}_{{\text{n}}} } \right)^{{\text{T}}} = \gamma (\gamma_{{1}} , \ldots \,,\gamma_{{\text{n}}} )^{{\text{T}}}\) , and the mean shift parameter i is non-zero when the ith observation is an outlier and zero.
3.4 Selected variables for the study
Table 1 highlights various financial parameters used in the study to analyse the impact of Basel norms on the financial performance of public sector Banks in India. These variables have been classified as Bank performance variables, independents variables, Bank Specific variables, and macroeconomic variables.
3.5 Framework of the Study
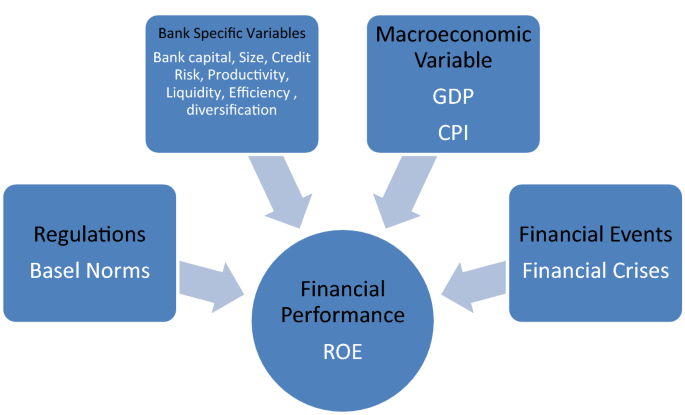
Source: Author’s Own
3.6 Robust Regression Model
The study analyzes the performance of Public sector banks under a set of variables, including bank-specific, bank regulation, macroeconomic variables and financial events. For this purpose, the following models were developed was used based on previous literature.
Here, the subscript i indicates cross-sectional dimension across the selected banks, t is for years, and ε indicates random error term. Pit denotes the financial performance of banks (ROE). β 1 is the constant term. LNA is the log of total assets (bank size), NNPANA is for credit risk, while CAR is the capital adequacy ratio (Bank capital). LATA is the liquidity measured by the liquid assets to total assets ratio. OPEXTA is management efficiency, PPE is Productivity, while NONIITI is for income diversification. GDP is used for economic growth, while CPI is for inflation. B1, B2 and B3 are dummy variables used for Three Basel Eras.
4 Results of Empirical Analysis
4.1 descriptive analysis.
Table 2 highlights the descriptive statistics of selected study variables. The mean ROE is 4.31%. Its maximum & minimum values are 46.63% and 1.09%, respectively. The mean value of the Capital Adequacy Ratio (CAR) during the study period was 12.21%. The mean value for NNPANA measures for banks’ credit risk in the study is 2.99%. The average value of Non-Interest Income to Total Income (NIITI), a measure of business diversification, is 11.28%. It can be seen from Table 2 that the average value of Operating expenses to total assets (OPETA), a measure of inefficiency used in the study, is 2.19%. The table shows the mean value of LATA, the measure used for liquidity is 8.45%. Profit per employee (PPE), a measure of productivity, has an average value of 0.57.
4.2 Correlation Analysis
Pearson’s correlation coefficients between the study variables in Table 3 indicate the degree of association between the variables. The problem of multicollinearity exists if the correlation coefficient is greater than 0.80. There exists no severe problem of multicollinearity between the dependent variables. ROE is positively associated with PPE, CAR, NNPANA, CPI, LNA, and NONIITI while negatively associated with GDP, LATA, and OPEXTA.
4.3 Robust Regression Analysis
Robust regression analysis was conducted to analyze the impact of Basel Norms on the financial performance of public sector banks in India. The empirical results of robust regression analysis have been stated in Table 4 .
5 Results and Discussion
Tables 4 give the empirical findings of the study indicating that the impact of bank size (LNA) and Bank risk (NNPANA) on the bank performance (ROE) of public sector banks in India were the same except for models 4 and 7. The findings show that bank size had no significance for the public sector banks' performance across all the seven models. Bank Risk had negatively and significantly impacted ROE of public sector banks in India during the study period confirming that increasing non-performing assets had negatively impacted the profitability of public sector banks. Capital adequacy ratio (CAR) shows a negative and significant impact in models (2, 4, 6 and 7). Higher bank capital relates to low return to shareholders. Basel I norms had positively and significantly impacted ROE of public sector banks, while Basel II & III had negatively and significantly affected the public sector banks’ performance implying that more stringent policies of Basel II and Basel III had adverse effects on public sector banks’ performance. Cost inefficiency had not impacted ROE during the study period. Liquidity (LATA) had an inverse, and significant relationship with bank performance across all the seven models except models 3 and 6, which indicates that’s banks have more return on equity for shareholders by lending more and maintaining lower liquid assets. In all the seven models, Labour productivity (PPE) and income diversification (NONIITI) had a positive and significant relationship with bank performance (ROE), implying that higher labour productivity and diversified income lead to higher profit for shareholders. Beyond the expectations, financial crises were positively related to ROE. GDP growth rate had no significant impact on ROE of public sector banks while CPI affected the bank performance positively as indicated in all models except models 6 and 7, where it shows a negative impact on ROE.
It can be concluded from the findings that bank size had no significance on the public sector banks’ performance during the study period. Bank Risk had negatively and significantly impacted the bank performance of public sector banks in India during the study period confirming that increasing non-performing assets had negatively impacted the profitability of public sector banks. Some models positively and significantly impact the capital adequacy ratio (CAR). Higher bank capital relates to higher bank profitability. Cost inefficiency had not impacted bank performance during the study period. It was found that the measures of liquidity (LATA) had an inverse and significant relationship with bank performance in most of the models, which indicates that banks earn more by lending more and maintaining lower liquid assets. However, Cost inefficiency had positively impacted Net Interest Margin during the study period. It was found that the measures of liquidity (LATA) across all the seven models had no significant relationship with the Net Interest Margin of public sector banks. In almost all the models, Labour productivity (PPE) and income diversification (NONIITI) had a positive and significant relationship with bank performance, implying that higher labour productivity and diversified income lead to higher profit for the banks. Income diversification (NONIITI) had a negative and significant relationship with the net interest margin of public sector banks, implying that diversified income is related to the lower NIM of banks. Surprisingly, financial crises positively correlated with bank performance in some models. Financial crises have negatively impacted the NIM of public sector banks in India.
6 Implications of the Study
The robust regression offers an efficient and more realistic analysis of financial data by eliminating or reducing the impact of outliers and influential observations. Robust regression can be helpful to the researchers dealing with financial and economic data for getting more reliable and efficient results in financial data analysis.
Banks are suggested to develop information technology and human resource skills and should adopt an advanced database management system to implement Basel III. Banks should also change their business model to comply with Basel III requirements cost-efficiently. Implementation of Basel III would require more capital. The government is suggested to propose a plan for disinvestment in public sector banks. To reduce the burden of additional capital requirements for banks, RBI can also reduce the Capital adequacy ratio for public sector banks.
7 Direction for Future Research
Robust regression analysis can be performed on financial data with outliers and influential observations. It can be used to analyse financial data related to the capital market, banking sector, insurance, mutual funds etc. Robust regression can be applied when the financial data are suspicious of heteroscedasticity. Robust estimation can be considered when the leasdt square method is inefficient and can give a biased outcome. The present study applied the M-estimator method of robust regression, other methods can also be applied, and a study based on a comparison of different methods can be conducted.
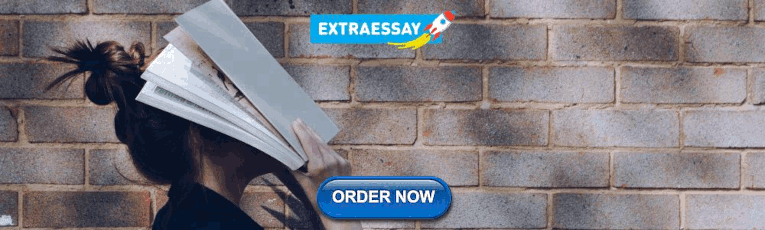
Availability of data and materials
All data and materials are included in the submission.
Code Availability
Zavadzki T, de Pauli S, Kleina M, Bonat WH (2020) Comparing artificial neural network architectures for brazilian stock market prediction. Ann Data Sci 7:613–628
Article Google Scholar
Tien JM (2017) Internet of things, real-time decision making, and artificial intelligence. Ann Data Sci 4(2):149–178
Shi Y (2022) Advances in big data analytics: theory, algorithm and practice. Springer, Singapore
Book Google Scholar
Olson DL, Shi Y (2007) Introduction to business data mining. McGraw-Hill/Irwin, New York
Google Scholar
Kumar BS, Ravi V, Miglani R (2021) Predicting Indian stock market using the psycho-linguistic features of financial news. Ann Data Sci 8:517–558
Muthukrishnan R et al (2017) Robust regression procedure for model fitting with application to image analysis. Int J Statistics Syst 12(1):79–92
Khan DM (2021) Applications of robust regression techniques: an econometric approach. Math Probl Eng 2021:9
Blatna D (2015) Application of Robust Regression Methods in An Analysis of The European Countries’ Share Of Renewable Energy In Gross Final Energy Consumption, 18th international scientific conference on application of mathematics and statistics in economics, 2–6 septembet, 2015.
Yu, C. & Yao, W. (2014). Robust linear regression: Review and comparison, arxiv, pp. 1–38. Available at: arXiv:1404.6274 . Accessed on 10 Dec 2022
Huber PJ (1964) Robust version of a location parameter. Ann Math Stat 36:1753–1758
Siegel AF (1982) Robust regression using repeated medians. Biometrika 69:242–244
Rousseeuw, P. J.(1983). Multivariate Estimation with High Breakdown Point. Research Report No. 192, Center for Statistics and Operations research, VUB Brussels.
Rousseeuw PJ, Yohai VJ (1984) Robust regression by means of s-estimators robust and nonlinear time series. In: Franke J, Hardle W, Martin RD (eds) Lectures Notes in Statistics, vol 26. Springer, New York, pp 256–272
Yohai VJ (1987) High breakdown-point and high efficiency robust estimates for regression. Ann Stat 15:642–656
Jackel LA (1972) Estimating regression Coe_cients by minimizing the dispersion of the residuals. Ann Mathematical Statistics 5:1449–1458
She Y, Owen AB (2011) Outlier Detection Using Nonconvex Penalized regression. J Am Stat Assoc 106:626–639
Chen YK, Shen CH, Kao L, Yeh CY (2018) Bank liquidity risk and performance. Rev Pac Basin Financial Mark Policies 21(01):1850007
Yüksel S, Mukhtarov S, Mammadov E, Özsarı M (2018) Determinants of profitability in the banking sector: an analysis of post-soviet countries. Economies 6(3):41
Kohlscheen E, Murcia Pabón A, Contreras JA (2018) Determinants of bank profitability in emerging markets. ERN: Other Emerging Markets Economics: Macroeconomic Issues & Challenges, BIS working paper no. 686
Căpraru B, Ihnatov I (2015) Determinants of bank’s profitability in EU15. Ann Alexandru Ioan Cuza Univ-Econ 62(1):93–101
Rahman MM, Hamid MK, Khan MAM (2015) Determinants of bank profitability: Empirical evidence from Bangladesh. Int j bus manag 10(8):135
Albulescu CT (2015) Banks’ profitability and financial soundness indicators: a macro-level investigation in emerging countries. Procedia economics and finance 23(2015):203–209
Menicucci E, Paolucci G (2016) The determinants of bank profitability: empirical evidence from European banking sector. J financial report Account. https://doi.org/10.1108/JFRA-05-2015-0060
Alexiou C, Sofoklis V (2009) Determinants of bank profitability: Evidence from the Greek banking sector. Econ Ann 54(182):93–118
Alhassan AL, Tetteh ML, Brobbey FO (2016) Market power, efficiency and bank profitability: evidence from Ghana. Econ Chang Restruct 49(1):71–93
Bougatef K (2017) Determinants of bank profitability in Tunisia: does corruption matter? J Money Laund Control. https://doi.org/10.1108/JMLC-10-2015-0044
Brahmaiah B (2018) Factors influencing profitability of banks in India. Theor Econ Lett 8(14):3046
Bouzgarrou H, Jouida S, Louhichi W (2018) Bank profitability during and before the financial crisis: domestic versus foreign banks. Res Int Bus Finance 44:26–39
Sufian F, Habibullah MS (2009) Determinants of bank profitability in a developing economy: empirical evidence from Bangladesh. J Bus Econ Manag 10(3):207–217
Petria N, Capraru B, Ihnatov I (2015) Determinants of banks’ profitability: evidence from EU 27 banking systems. Procedia econ finance 20(15):518–524
Athanasoglou PP, Delis MD, Staikouras CK (2006) Determinants of bank profitability in the South Eastern European region. Working Papers 47, Bank of Greece
Al-Jafari MK, Alchami M (2014) Determinants of bank profitability: Evidence from Syria. J Appl Finance Bank 4(1):17
Islam MS, Nishiyama SI (2016) The determinants of bank profitability: dynamic panel evidence from South Asian countries. J Appl Finance Bank 6(3):77
Salike N, Ao B (2018) Determinants of bank’s profitability: role of poor asset quality in Asia. China Finance Rev Int. https://doi.org/10.1108/CFRI-10-2016-0118
Majumder MTH, Li X (2018) Bank risk and performance in an emerging market setting: the case of Bangladesh. J Econ, Finance Admin Sci. https://doi.org/10.1108/JEFAS-07-2017-0084
Naifar N (2010) The determinants of bank performance: an analysis of theory and practice in the case of an emerging market. Int J Bus Environ 3(4):460–470
Tan Y, Floros C (2012) Bank profitability and inflation: the case of China. J Econ Stud. https://doi.org/10.1108/01443581211274610
Mirzaei A, Mirzaei Z (2011) Bank-specific and macroeconomic determinants of profitability in middle eastern banking. Iran Econ Rev 15(29):101–128
Ćurak M, Poposki K, Pepur S (2012) Profitability determinants of the Macedonian banking sector in changing environment. Procedia Soc Behav Sci 44(2012):406–416
Sufian F, Chong RR (2008) Determinants of bank profitability in a developing economy: empirical evidence from Philippines. Asian Acad Manag J Account Fin 4(2):91–112
Abdullah MN, Parvez K, Ayreen S (2014) Bank specific, industry specific and macroeconomic determinants of commercial bank profitability: a case of Bangladesh. World 4(3):82–96
Tan Y, Floros C (2012) Bank profitability and GDP growth in China: a note. J Chin Econ Bus Stud 10(3):267–273
Sufian F (2012) Determinants of bank profitability in developing economies: empirical evidence from the South Asian banking sectors. Contemp South Asia 20(3):375–399
Samad A (2015) Determinants bank profitability: empirical evidence from Bangladesh commercial banks. Int j financial res 6(3):173–179
Reddy KS (2011) Determinants of commercial banks profitability in India: a dynamic panel data model approach. Pakistan J Appl Econ 21(1&2):15–36
Kosmidou K, Tanna S, Pasiouras F (2005) Determinants of profitability of domestic UK commercial banks: panel evidence from the period 1995–2022. Money Macro and Finance Res Group Conf 45:1–27
Sarpong-Kumankoma E, Abor J, Aboagye AQQ, Amidu M (2018) Freedom, competition and bank profitability in Sub-Saharan Africa. J Financial Regul Compliance. https://doi.org/10.1108/JFRC-12-2017-0107
Goddard J, Molyneux P, Wilson JO (2004) The profitability of European banks: a cross-sectional and dynamic panel analysis. Manch Sch 72(3):363–381
Yameen M, Pervez A (2016) Impact of liquidity, solvency and efficiency on profitability of steel authority of India Limited. Int J Account Res 42(3968):1–10
Ali MA, Pervez A, Bansal R, Khan MA (2022) Analyzing performance of banks in India: robust regression analysis approach. Discret Dyn Nat Soc 2022:1–9
Taqi M, Ajmal M, Pervez A (2016) Impact of capital structure on profitability of selected trading companies of India. Oman chapter Arabian J Bus Manag Rev 34(3956):1–16
Momeni M, Nayeri MD, Ghayoumi AF, Ghorbani H (2010) Robust regression and its application in financial data analysis. World Academy of Science, Engineering and Technology. Int J Soc Behav Educ Econ Bus Indust Eng 4:2173–2178
Download references
This work has not been funded by any agency.
Author information
Authors and affiliations.
Centre for Distance and Online Education, Jamia Millia Islamia, New Delhi, India
Asif Pervez
Department of Statistics & Operations Research, Aligarh Muslim University, Aligarh, India
You can also search for this author in PubMed Google Scholar
Contributions
Dr Asif Pervez: main author; Dr Irfan Ali: Corresponding Author.
Corresponding author
Correspondence to Irfan Ali .
Ethics declarations
Conflict of interest.
The authors declare that they have no conflict of interest.
Ethical statement
We hereby declare that this manuscript is the result of our creation with the reviewers’ comments. Except for the quoted contents. This manuscript does not contain any research achievements that have been published or written by other individuals or groups. The legal responsibility of this statement shall be borne by us.
Additional information
Publisher's note.
Springer Nature remains neutral with regard to jurisdictional claims in published maps and institutional affiliations.
Rights and permissions
Reprints and permissions
About this article
Pervez, A., Ali, I. Robust Regression Analysis in Analyzing Financial Performance of Public Sector Banks: A Case Study of India. Ann. Data. Sci. 11 , 677–691 (2024). https://doi.org/10.1007/s40745-022-00427-3
Download citation
Received : 06 January 2022
Revised : 07 June 2022
Accepted : 10 June 2022
Published : 01 July 2022
Issue Date : April 2024
DOI : https://doi.org/10.1007/s40745-022-00427-3
Share this article
Anyone you share the following link with will be able to read this content:
Sorry, a shareable link is not currently available for this article.
Provided by the Springer Nature SharedIt content-sharing initiative
- Financial data
- Financial analysis
- Bank performance
- Robust regression analysis
- Robust estimation
- Find a journal
- Publish with us
- Track your research
A Study on Multiple Linear Regression Analysis
- December 2013
- Procedia - Social and Behavioral Sciences 106:234–240
- 106:234–240
- CC BY-NC-ND 3.0

- Sakarya University

Discover the world's research
- 25+ million members
- 160+ million publication pages
- 2.3+ billion citations
- J ENVIRON MANAGE

- Hongxun Xiang
- Boleng Zhai
- Lingling Chen
- Weixiang Ma
- Shuang Liang

- Nyigit Wudi Amini

- Erna Setijaningrum
- Hardayani Haruno
- Decky Oktaviansyah

- Chayanat Buathongkhue
- Kritsana Sureeya

- Francis G. Phi
- Jungeun Kim
- Ruidong Zhao
- Ranhong Bie

- Linda S. Fidell
- Ş Büyüköztürk
- Recruit researchers
- Join for free
- Login Email Tip: Most researchers use their institutional email address as their ResearchGate login Password Forgot password? Keep me logged in Log in or Continue with Google Welcome back! Please log in. Email · Hint Tip: Most researchers use their institutional email address as their ResearchGate login Password Forgot password? Keep me logged in Log in or Continue with Google No account? Sign up

An official website of the United States government
The .gov means it’s official. Federal government websites often end in .gov or .mil. Before sharing sensitive information, make sure you’re on a federal government site.
The site is secure. The https:// ensures that you are connecting to the official website and that any information you provide is encrypted and transmitted securely.
- Publications
- Account settings
Preview improvements coming to the PMC website in October 2024. Learn More or Try it out now .
- Advanced Search
- Journal List
- Cardiopulm Phys Ther J
- v.20(3); 2009 Sep
Regression Analysis for Prediction: Understanding the Process
Phillip b palmer.
1 Hardin-Simmons University, Department of Physical Therapy, Abilene, TX
Dennis G O'Connell
2 Hardin-Simmons University, Department of Physical Therapy, Abilene, TX
Research related to cardiorespiratory fitness often uses regression analysis in order to predict cardiorespiratory status or future outcomes. Reading these studies can be tedious and difficult unless the reader has a thorough understanding of the processes used in the analysis. This feature seeks to “simplify” the process of regression analysis for prediction in order to help readers understand this type of study more easily. Examples of the use of this statistical technique are provided in order to facilitate better understanding.
INTRODUCTION
Graded, maximal exercise tests that directly measure maximum oxygen consumption (VO 2 max) are impractical in most physical therapy clinics because they require expensive equipment and personnel trained to administer the tests. Performing these tests in the clinic may also require medical supervision; as a result researchers have sought to develop exercise and non-exercise models that would allow clinicians to predict VO 2 max without having to perform direct measurement of oxygen uptake. In most cases, the investigators utilize regression analysis to develop their prediction models.
Regression analysis is a statistical technique for determining the relationship between a single dependent (criterion) variable and one or more independent (predictor) variables. The analysis yields a predicted value for the criterion resulting from a linear combination of the predictors. According to Pedhazur, 15 regression analysis has 2 uses in scientific literature: prediction, including classification, and explanation. The following provides a brief review of the use of regression analysis for prediction. Specific emphasis is given to the selection of the predictor variables (assessing model efficiency and accuracy) and cross-validation (assessing model stability). The discussion is not intended to be exhaustive. For a more thorough explanation of regression analysis, the reader is encouraged to consult one of many books written about this statistical technique (eg, Fox; 5 Kleinbaum, Kupper, & Muller; 12 Pedhazur; 15 and Weisberg 16 ). Examples of the use of regression analysis for prediction are drawn from a study by Bradshaw et al. 3 In this study, the researchers' stated purpose was to develop an equation for prediction of cardiorespiratory fitness (CRF) based on non-exercise (N-EX) data.
SELECTING THE CRITERION (OUTCOME MEASURE)
The first step in regression analysis is to determine the criterion variable. Pedhazur 15 suggests that the criterion have acceptable measurement qualities (ie, reliability and validity). Bradshaw et al 3 used VO 2 max as the criterion of choice for their model and measured it using a maximum graded exercise test (GXT) developed by George. 6 George 6 indicated that his protocol for testing compared favorably with the Bruce protocol in terms of predictive ability and had good test-retest reliability ( ICC = .98 –.99). The American College of Sports Medicine indicates that measurement of VO 2 max is the “gold standard” for measuring cardiorespiratory fitness. 1 These facts support that the criterion selected by Bradshaw et al 3 was appropriate and meets the requirements for acceptable reliability and validity.
SELECTING THE PREDICTORS: MODEL EFFICIENCY
Once the criterion has been selected, predictor variables should be identified (model selection). The aim of model selection is to minimize the number of predictors which account for the maximum variance in the criterion. 15 In other words, the most efficient model maximizes the value of the coefficient of determination ( R 2 ). This coefficient estimates the amount of variance in the criterion score accounted for by a linear combination of the predictor variables. The higher the value is for R 2 , the less error or unexplained variance and, therefore, the better prediction. R 2 is dependent on the multiple correlation coefficient ( R ), which describes the relationship between the observed and predicted criterion scores. If there is no difference between the predicted and observed scores, R equals 1.00. This represents a perfect prediction with no error and no unexplained variance ( R 2 = 1.00). When R equals 0.00, there is no relationship between the predictor(s) and the criterion and no variance in scores has been explained ( R 2 = 0.00). The chosen variables cannot predict the criterion. The goal of model selection is, as stated previously, to develop a model that results in the highest estimated value for R 2 .
According to Pedhazur, 15 the value of R is often overestimated. The reasons for this are beyond the scope of this discussion; however, the degree of overestimation is affected by sample size. The larger the ratio is between the number of predictors and subjects, the larger the overestimation. To account for this, sample sizes should be large and there should be 15 to 30 subjects per predictor. 11 , 15 Of course, the most effective way to determine optimal sample size is through statistical power analysis. 11 , 15
Another method of determining the best model for prediction is to test the significance of adding one or more variables to the model using the partial F-test . This process, which is further discussed by Kleinbaum, Kupper, and Muller, 12 allows for exclusion of predictors that do not contribute significantly to the prediction, allowing determination of the most efficient model of prediction. In general, the partial F-test is similar to the F-test used in analysis of variance. It assesses the statistical significance of the difference between values for R 2 derived from 2 or more prediction models using a subset of the variables from the original equation. For example, Bradshaw et al 3 indicated that all variables contributed significantly to their prediction. Though the researchers do not detail the procedure used, it is highly likely that different models were tested, excluding one or more variables, and the resulting values for R 2 assessed for statistical difference.
Although the techniques discussed above are useful in determining the most efficient model for prediction, theory must be considered in choosing the appropriate variables. Previous research should be examined and predictors selected for which a relationship between the criterion and predictors has been established. 12 , 15
It is clear that Bradshaw et al 3 relied on theory and previous research to determine the variables to use in their prediction equation. The 5 variables they chose for inclusion–gender, age, body mass index (BMI), perceived functional ability (PFA), and physical activity rating (PAR)–had been shown in previous studies to contribute to the prediction of VO 2 max (eg, Heil et al; 8 George, Stone, & Burkett 7 ). These 5 predictors accounted for 87% ( R = .93, R 2 = .87 ) of the variance in the predicted values for VO 2 max. Based on a ratio of 1:20 (predictor:sample size), this estimate of R , and thus R 2 , is not likely to be overestimated. The researchers used changes in the value of R 2 to determine whether to include or exclude these or other variables. They reported that removal of perceived functional ability (PFA) as a variable resulted in a decrease in R from .93 to .89. Without this variable, the remaining 4 predictors would account for only 79% of the variance in VO 2 max. The investigators did note that each predictor variable contributed significantly ( p < .05 ) to the prediction of VO 2 max (see above discussion related to the partial F-test).
ASSESSING ACCURACY OF THE PREDICTION
Assessing accuracy of the model is best accomplished by analyzing the standard error of estimate ( SEE ) and the percentage that the SEE represents of the predicted mean ( SEE % ). The SEE represents the degree to which the predicted scores vary from the observed scores on the criterion measure, similar to the standard deviation used in other statistical procedures. According to Jackson, 10 lower values of the SEE indicate greater accuracy in prediction. Comparison of the SEE for different models using the same sample allows for determination of the most accurate model to use for prediction. SEE % is calculated by dividing the SEE by the mean of the criterion ( SEE /mean criterion) and can be used to compare different models derived from different samples.
Bradshaw et al 3 report a SEE of 3.44 mL·kg −1 ·min −1 (approximately 1 MET) using all 5 variables in the equation (gender, age, BMI, PFA, PA-R). When the PFA variable is removed from the model, leaving only 4 variables for the prediction (gender, age, BMI, PA-R), the SEE increases to 4.20 mL·kg −1 ·min −1 . The increase in the error term indicates that the model excluding PFA is less accurate in predicting VO 2 max. This is confirmed by the decrease in the value for R (see discussion above). The researchers compare their model of prediction with that of George, Stone, and Burkett, 7 indicating that their model is as accurate. It is not advisable to compare models based on the SEE if the data were collected from different samples as they were in these 2 studies. That type of comparison should be made using SEE %. Bradshaw and colleagues 3 report SEE % for their model (8.62%), but do not report values from other models in making comparisons.
Some advocate the use of statistics derived from the predicted residual sum of squares ( PRESS ) as a means of selecting predictors. 2 , 4 , 16 These statistics are used more often in cross-validation of models and will be discussed in greater detail later.
ASSESSING STABILITY OF THE MODEL FOR PREDICTION
Once the most efficient and accurate model for prediction has been determined, it is prudent that the model be assessed for stability. A model, or equation, is said to be “stable” if it can be applied to different samples from the same population without losing the accuracy of the prediction. This is accomplished through cross-validation of the model. Cross-validation determines how well the prediction model developed using one sample performs in another sample from the same population. Several methods can be employed for cross-validation, including the use of 2 independent samples, split samples, and PRESS -related statistics developed from the same sample.
Using 2 independent samples involves random selection of 2 groups from the same population. One group becomes the “training” or “exploratory” group used for establishing the model of prediction. 5 The second group, the “confirmatory” or “validatory” group is used to assess the model for stability. The researcher compares R 2 values from the 2 groups and assessment of “shrinkage,” the difference between the two values for R 2 , is used as an indicator of model stability. There is no rule of thumb for interpreting the differences, but Kleinbaum, Kupper, and Muller 12 suggest that “shrinkage” values of less than 0.10 indicate a stable model. While preferable, the use of independent samples is rarely used due to cost considerations.
A similar technique of cross-validation uses split samples. Once the sample has been selected from the population, it is randomly divided into 2 subgroups. One subgroup becomes the “exploratory” group and the other is used as the “validatory” group. Again, values for R 2 are compared and model stability is assessed by calculating “shrinkage.”
Holiday, Ballard, and McKeown 9 advocate the use of PRESS-related statistics for cross-validation of regression models as a means of dealing with the problems of data-splitting. The PRESS method is a jackknife analysis that is used to address the issue of estimate bias associated with the use of small sample sizes. 13 In general, a jackknife analysis calculates the desired test statistic multiple times with individual cases omitted from the calculations. In the case of the PRESS method, residuals, or the differences between the actual values of the criterion for each individual and the predicted value using the formula derived with the individual's data removed from the prediction, are calculated. The PRESS statistic is the sum of the squares of the residuals derived from these calculations and is similar to the sum of squares for the error (SS error ) used in analysis of variance (ANOVA). Myers 14 discusses the use of the PRESS statistic and describes in detail how it is calculated. The reader is referred to this text and the article by Holiday, Ballard, and McKeown 9 for additional information.
Once determined, the PRESS statistic can be used to calculate a modified form of R 2 and the SEE . R 2 PRESS is calculated using the following formula: R 2 PRESS = 1 – [ PRESS / SS total ], where SS total equals the sum of squares for the original regression equation. 14 Standard error of the estimate for PRESS ( SEE PRESS ) is calculated as follows: SEE PRESS =, where n equals the number of individual cases. 14 The smaller the difference between the 2 values for R 2 and SEE , the more stable the model for prediction. Bradshaw et al 3 used this technique in their investigation. They reported a value for R 2 PRESS of .83, a decrease of .04 from R 2 for their prediction model. Using the standard set by Kleinbaum, Kupper, and Muller, 12 the model developed by these researchers would appear to have stability, meaning it could be used for prediction in samples from the same population. This is further supported by the small difference between the SEE and the SEE PRESS , 3.44 and 3.63 mL·kg −1 ·min −1 , respectively.
COMPARING TWO DIFFERENT PREDICTION MODELS
A comparison of 2 different models for prediction may help to clarify the use of regression analysis in prediction. Table Table1 1 presents data from 2 studies and will be used in the following discussion.
Comparison of Two Non-exercise Models for Predicting CRF
Variables | Heil et al = 374 | Bradshaw et al = 100 |
---|---|---|
Intercept | 36.580 | 48.073 |
Gender (male = 1, female = 0) | 3.706 | 6.178 |
Age (years) | 0.558 | −0.246 |
Age | −7.81 E-3 | |
Percent body fat | −0.541 | |
Body mass index (kg-m ) | −0.619 | |
Activity code (0-7) | 1.347 | |
Physical activity rating (0–10) | 0.671 | |
Perceived functional abilty | 0.712 | |
) | ||
.88 (.77) | .93 (.87) | |
4.90·mL–kg ·min | 3.44 mL·kg min | |
12.7% | 8.6% |
As noted above, the first step is to select an appropriate criterion, or outcome measure. Bradshaw et al 3 selected VO 2 max as their criterion for measuring cardiorespiratory fitness. Heil et al 8 used VO 2 peak. These 2 measures are often considered to be the same, however, VO 2 peak assumes that conditions for measuring maximum oxygen consumption were not met. 17 It would be optimal to compare models based on the same criterion, but that is not essential, especially since both criteria measure cardiorespiratory fitness in much the same way.
The second step involves selection of variables for prediction. As can be seen in Table Table1, 1 , both groups of investigators selected 5 variables to use in their model. The 5 variables selected by Bradshaw et al 3 provide a better prediction based on the values for R 2 (.87 and .77), indicating that their model accounts for more variance (87% versus 77%) in the prediction than the model of Heil et al. 8 It should also be noted that the SEE calculated in the Bradshaw 3 model (3.44 mL·kg −1 ·min −1 ) is less than that reported by Heil et al 8 (4.90 mL·kg −1 ·min −1 ). Remember, however, that comparison of the SEE should only be made when both models are developed using samples from the same population. Comparing predictions developed from different populations can be accomplished using the SEE% . Review of values for the SEE% in Table Table1 1 would seem to indicate that the model developed by Bradshaw et al 3 is more accurate because the percentage of the mean value for VO 2 max represented by error is less than that reported by Heil et al. 8 In summary, the Bradshaw 3 model would appear to be more efficient, accounting for more variance in the prediction using the same number of variables. It would also appear to be more accurate based on comparison of the SEE% .
The 2 models cannot be compared based on stability of the models. Each set of researchers used different methods for cross-validation. Both models, however, appear to be relatively stable based on the data presented. A clinician can assume that either model would perform fairly well when applied to samples from the same populations as those used by the investigators.
The purpose of this brief review has been to demystify regression analysis for prediction by explaining it in simple terms and to demonstrate its use. When reviewing research articles in which regression analysis has been used for prediction, physical therapists should ensure that the: (1) criterion chosen for the study is appropriate and meets the standards for reliability and validity, (2) processes used by the investigators to assess both model efficiency and accuracy are appropriate, 3) predictors selected for use in the model are reasonable based on theory or previous research, and 4) investigators assessed model stability through a process of cross-validation, providing the opportunity for others to utilize the prediction model in different samples drawn from the same population.
Subscribe to the PwC Newsletter
Join the community, add a new evaluation result row.
2129 papers with code • 0 benchmarks • 14 datasets
Benchmarks Add a Result

Most implemented papers
Model-agnostic meta-learning for fast adaptation of deep networks.
We propose an algorithm for meta-learning that is model-agnostic, in the sense that it is compatible with any model trained with gradient descent and applicable to a variety of different learning problems, including classification, regression, and reinforcement learning.
Weight Uncertainty in Neural Networks
We introduce a new, efficient, principled and backpropagation-compatible algorithm for learning a probability distribution on the weights of a neural network, called Bayes by Backprop.
Dropout as a Bayesian Approximation: Representing Model Uncertainty in Deep Learning
yaringal/DropoutUncertaintyExps • 6 Jun 2015
In comparison, Bayesian models offer a mathematically grounded framework to reason about model uncertainty, but usually come with a prohibitive computational cost.
Implicit Quantile Networks for Distributional Reinforcement Learning
In this work, we build on recent advances in distributional reinforcement learning to give a generally applicable, flexible, and state-of-the-art distributional variant of DQN.
Distance-IoU Loss: Faster and Better Learning for Bounding Box Regression

By incorporating DIoU and CIoU losses into state-of-the-art object detection algorithms, e. g., YOLO v3, SSD and Faster RCNN, we achieve notable performance gains in terms of not only IoU metric but also GIoU metric.
SQuAD: 100,000+ Questions for Machine Comprehension of Text
worksheets/0xd53d03a4 • EMNLP 2016
We present the Stanford Question Answering Dataset (SQuAD), a new reading comprehension dataset consisting of 100, 000+ questions posed by crowdworkers on a set of Wikipedia articles, where the answer to each question is a segment of text from the corresponding reading passage.
Distributional Reinforcement Learning with Quantile Regression
In this paper, we build on recent work advocating a distributional approach to reinforcement learning in which the distribution over returns is modeled explicitly instead of only estimating the mean.
Multi-Task Learning Using Uncertainty to Weigh Losses for Scene Geometry and Semantics
yaringal/multi-task-learning-example • CVPR 2018
Numerous deep learning applications benefit from multi-task learning with multiple regression and classification objectives.
Bayesian regression and Bitcoin
panditanvita/BTCpredictor • 6 Oct 2014
In this paper, we discuss the method of Bayesian regression and its efficacy for predicting price variation of Bitcoin, a recently popularized virtual, cryptographic currency.
Tensor Regression
Tensors, as high dimensional extensions of vectors, are considered as natural representations of high dimensional data.
Thank you for visiting nature.com. You are using a browser version with limited support for CSS. To obtain the best experience, we recommend you use a more up to date browser (or turn off compatibility mode in Internet Explorer). In the meantime, to ensure continued support, we are displaying the site without styles and JavaScript.
- View all journals
- Explore content
- About the journal
- Publish with us
- Sign up for alerts
- Open access
- Published: 18 June 2024
A matched case-control analysis of autonomous vs human-driven vehicle accidents
- Mohamed Abdel-Aty 1 &
- Shengxuan Ding ORCID: orcid.org/0009-0006-7690-9546 1
Nature Communications volume 15 , Article number: 4931 ( 2024 ) Cite this article
9272 Accesses
978 Altmetric
Metrics details
- Civil engineering
- Decision making
Despite the recent advancements that Autonomous Vehicles have shown in their potential to improve safety and operation, considering differences between Autonomous Vehicles and Human-Driven Vehicles in accidents remain unidentified due to the scarcity of real-world Autonomous Vehicles accident data. We investigated the difference in accident occurrence between Autonomous Vehicles’ levels and Human-Driven Vehicles by utilizing 2100 Advanced Driving Systems and Advanced Driver Assistance Systems and 35,113 Human-Driven Vehicles accident data. A matched case-control design was conducted to investigate the differential characteristics involving Autonomous’ versus Human-Driven Vehicles’ accidents. The analysis suggests that accidents of vehicles equipped with Advanced Driving Systems generally have a lower chance of occurring than Human-Driven Vehicles in most of the similar accident scenarios. However, accidents involving Advanced Driving Systems occur more frequently than Human-Driven Vehicle accidents under dawn/dusk or turning conditions, which is 5.25 and 1.98 times higher, respectively. Our research reveals the accident risk disparities between Autonomous Vehicles and Human-Driven Vehicles, informing future development in Autonomous technology and safety enhancements.
Similar content being viewed by others
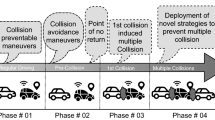
Multiple vehicle cooperation and collision avoidance in automated vehicles: survey and an AI-enabled conceptual framework
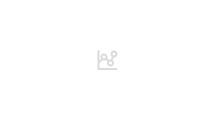
The ironies of autonomy
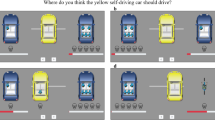
The risk ethics of autonomous vehicles: an empirical approach
Introduction.
Automation of systems has been experiencing rapid development and has brought about a revolution in the transportation industry. The introduction of Autonomous Vehicles (AV) technology has made the vision of a safe transportation system with effortless driving seem attainable. It is anticipated that the automation of systems will significantly reduce the number of accidents, as human errors contribute up to 90% of accidents 1 . While smart transportation has showcased several benefits, these emerging technologies have also exhibited drawbacks, particularly regarding safety risks. Accidents of on-road testing have already been documented in limited testing data 2 .
According to findings from the RAND corporation 3 , a mere advancement in safety features in the initial release of AVs could yield significant life-saving results. The research suggests that if AVs were to be introduced with an average safety level ten percent higher than that of the typical human driver, approximately 600,000 fatalities could be averted in the United States over a span of 35 years. Nevertheless, it is crucial to acknowledge that between 2015 and 2022, there was a yearly rise in both the annual miles traveled by autonomous vehicles (AVMT) and the number of AV accidents on public roads in California, with the exception of a decline in 2020 attributable probably to the COVID-19 pandemic 4 . AV testing on California public roads is permitted by the California Department of Motor Vehicles (CADMV) 5 . Up until June 2023, we have identified and enriched with 598 Advanced Driving Systems (ADS (SAE Level 4)) accidents in California (AVOID dataset) 6 . These reports provide details about AV accidents and disengagements, which happen when the vehicle switches from autonomous mode due to technological issues or when the test driver or operator assumes manual control for safety purposes 7 . The detailed information included in these reports revealed various factors related to AV accidents. We further collected the corresponding reports of Human-Driven Vehicles (HDV) accidents to contrast the differences between AV and matched HDV accidents.
There are many potential benefits of AVs on traffic safety, such as a reduction in human error, reduced fatigue, and distraction. ADAS functions including Electronic Stability Control (ESC), Anti-Lock Braking (ABS), and Information and Communication Technology aids in ongoing driving tasks to prevent accidents 8 , 9 , 10 . For example, Tesla Autopilot consistently maintains a closer distance to the lane center than human drivers 11 . Additionally, Level 3 or higher levels of automation can further enhance traffic by reducing accidents 12 , 13 , improving mobility for the disabled and elderly, and minimizing traffic collisions through efficient driving and the reduction of human errors 14 . Koopman and Wagner highlighted the importance of understanding how AVs will interact with human drivers, pedestrians, and other road users 15 . Compared with HDV, accident avoidance features of AVs can reduce accidents and fatalities caused by distracted driving or human error by helping control the vehicle and alerting drivers to potential dangers 16 , 17 .
However, there are also some possible safety challenges that need to be addressed in AVs. Penmetsa et al. 18 identified several safety challenges that need to be addressed in the development of AV, such as the need for reliable sensing and perception, robust decision-making algorithms, and fail-safe mechanisms 18 . Kalra and Paddock presented a statistical methodology with 382 accidents per 100 million miles based on binomial and Poisson distributions for estimating the required mileage to establish the reliability of AVs, it shows that AVs would have to be driven hundreds of millions of miles and sometimes hundreds of billions of miles to demonstrate their reliability in terms of fatalities and injuries 19 . Some studies also have concentrated on analyzing the accident severity involving different types of AV 20 . Ding et al. 6 collected 1280 cases to compare factors related to injury severity between the ADAS and ADS accidents by random parameter multinomial logit models. Subject vehicle’s contact area, road and environment, and pre-accident movement, significantly impact accidents injury severity. ADS-equipped vehicles in work zones have a higher probability of being involved in minor and moderate/severe injury accidents 21 .
The comparison of safety performance between AV and HDV is a topic of debate, with conflicting viewpoints 22 . On one side, numerous studies support the view that AVs are generally safer than HDVs 23 . For instance, Dixit et al. 24 analyzed the statistical distribution of reports on 69 manual disengagements and accidents, they compared the accident rates of Google self-driving cars to those of human drivers and found that fatal accidents involving Google cars were lower than that of HDV, no fatalities occurred as compared to 1 death for every 108 million miles in California 24 . Additionally, from 2009 to 2015 in Mountain View, California, Google cars demonstrated a significantly lower rate of police-reportable accidents per million vehicle miles traveled (VMT) compared to human drivers (2.19 versus 6.06) 25 . On the other hand, some research challenges this view, suggesting that the safety of AVs may not always exceed that of HDVs. Schoettle and Sivak uncovered that AVs have a higher rate of accidents per million miles traveled compared to HDVs in limited (and generally less demanding) conditions (e.g., avoiding snowy areas) 26 . Favarò et al. 27 found that rear-end accidents were the most frequent type of AV accidents, with AVs being hit from behind by conventional vehicles at a rate twice that of rear-end accidents than rear-end “fender-benders” for conventional vehicles in California 2013 27 .
These studies offered significant insight into the factors that contribute to AV and HDV accidents, while earlier studies may not consider sufficient factors of road environment, accident outcome, pre-accident condition, and accident type for accident analysis due to a lack of matched AV and HDV data for identifying the characteristics of AV accidents and how they differ from regular HDV accidents 28 , 29 , 30 . The number of accidents of the relevant studies and their automation level are shown in Fig. 1 .
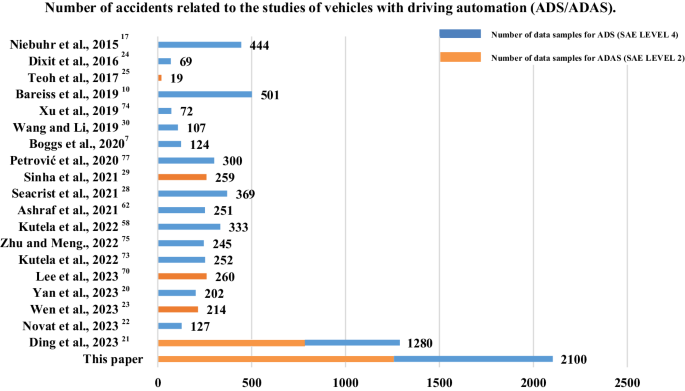
The blue line shows the number of ADS data samples, and the orange line shows the number of ADAS data samples used in related studies.
In this work, we examined a dataset comprising both AV and HDV accidents. The dataset utilized in this study was compiled from various sources and encompassed information on accident types, road and environmental conditions, pre-accident vehicle movements, and accident outcomes. We use a matched case-control logistic regression model. We further add the National Highway Traffic Safety Administration (NHTSA) AV database comprising an additional 495 ADS and 1001 Advanced driver assistance systems (ADAS (SAE Level 2)) accidents 31 . These findings illuminate the factors that contribute to accidents involving AVs vs. HDVs.
General trends in the full accident data
We first present general trends and comparisons between AV and HDV accidents of the full dataset. Figure 2a–d displays the distribution of factors affecting AV (2100), including SAE level 4 ADS (1099), level 2 ADAS (1001) and HDV accidents (35,133), respectively. Notably, vehicles make up 80% of participants in AV accidents, with pedestrians accounting for 3%. In contrast, for HDVs, pedestrians constitute 15% and vehicles 63% of accident participants, as depicted in Fig. 2a, b . When examining the outcomes of accidents, both AVs and HDVs lead to either no injuries or minor injuries occurring in 94%.
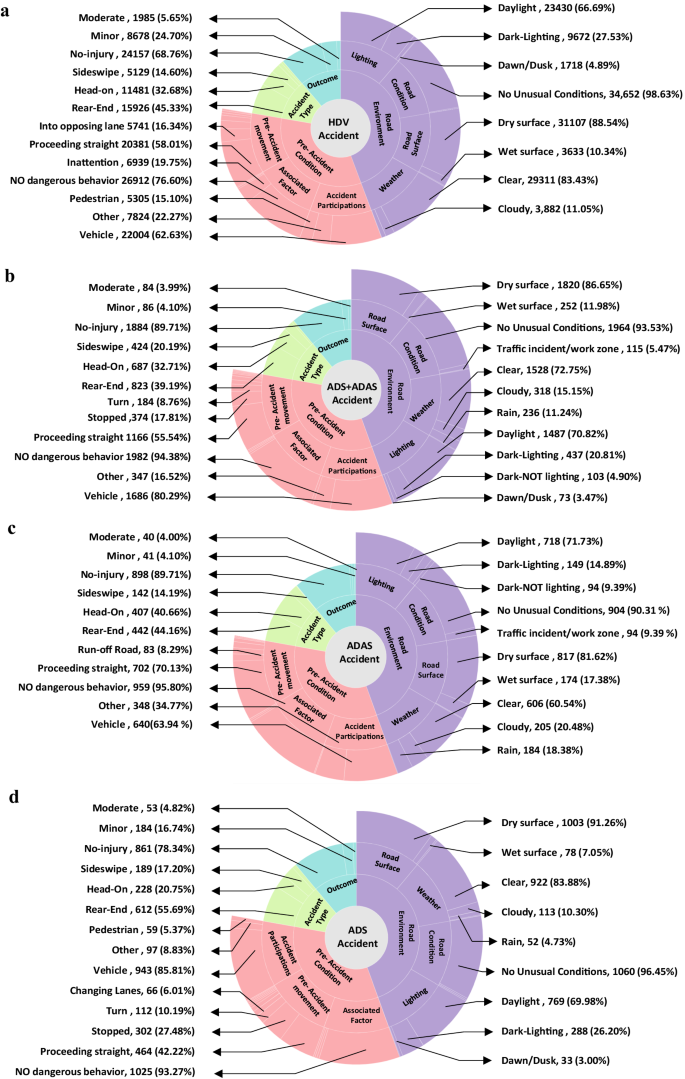
a HDV accidents with a sample of 35,133. b SAE level 4 ADS + SAE level 2 ADAS accidents with a sample of 2100. c SAE level 2 ADAS accidents with a sample of 1001. d SAE level 4 ADS accidents with a sample of 1099.
Significant disparities between AV and HDV accidents can be seen in work zones, traffic events, and pre-accident movements such as slowing down, proceeding straight, and moving into opposing lanes, with AVs exhibiting higher accident rates. For both AVs and HDVs, the most frequent pre-accident movement is proceeding straight. It is observed that 56% of AV accidents and 58% of HDV accidents occur under this specific condition. About 5% of AV accidents take place in locations impacted by previous traffic events or work zones, where normal traffic is disrupted by earlier incidents like disabled vehicles or spilled cargo. In comparison, just 1.3% of HDV accidents occur in similar settings. Analyzing the pre-accident scenario, a distinctive observation is that only 1.8% of AV accidents are attributed to inattention or poor driving behavior, in contrast to a much higher 19.8% for HDVs.
Evaluating environmental factors, the majority of accidents involving both AVs and HDVs tend to happen under clear weather conditions. Notably, accidents involving HDVs occur slightly more frequently under these conditions, at a rate of 83%, compared to 73% for AVs. However, AVs are more commonly involved in accidents during rainy conditions, accounting for 11% of such accidents, compared to HDVs which experience these conditions in only 5% of accidents. Dawn or dusk conditions experience 3.5% of AV accidents, which is lower than the 4.9% rate for HDVs.
In terms of accident type, rear-end accidents constitute a majority for both AVs and HDVs. Furthermore, we determine the accident type associated with AV and HDV based on the accident angle. Our data indicates that for HDVs, the rear-end stands at 45% (other vehicles hit HDV), while head-on accidents (HDV hit other vehicles) occur at a rate of 33%. In contrast, AVs have a slightly lower rear-end accidents (other vehicles hit AV) rate of 39%, but a similar head-on accidents (AV hit other vehicles) rate of 33%. This suggests that while AVs have a marginally lower percentage of being rear-ended compared to HDVs, their percentage of head-on accidents is almost the same.
Figure 3 describes two conditions related to ADS rear-end accidents: a: HDV has hit an ADS from behind (252) and b: an ADS has hit an HDV from behind (67). The left side of the diagram starts with two conditions of HDV hit ADS or ADS hit HDV. The middle section shows the movement of vehicle: Moving or Stopping. The right side categorizes the severity of the accidents: Minor, Moderate and Major. The numbers indicated in each section of the diagram correspond to the total count of each specific category. The width of each link connecting the sections of the diagrams represents the proportion of scenarios that fall into the subsequent category. For example, In Fig. 3a , which details accidents where a HDV hit an ADS, we see that for the accidents where the ADS was moving (middle section), 62 cases (57%) involved ADS in autonomous mode while 46 cases (43%) involved ADS in conventional mode (left section). Among the 108 accidents categorized under the ‘moving condition’, there were 2 cases (2%) that resulted in major injuries, 18 cases (17%) that led to moderate injuries, and the remaining 88 cases (81%) involved minor injuries.
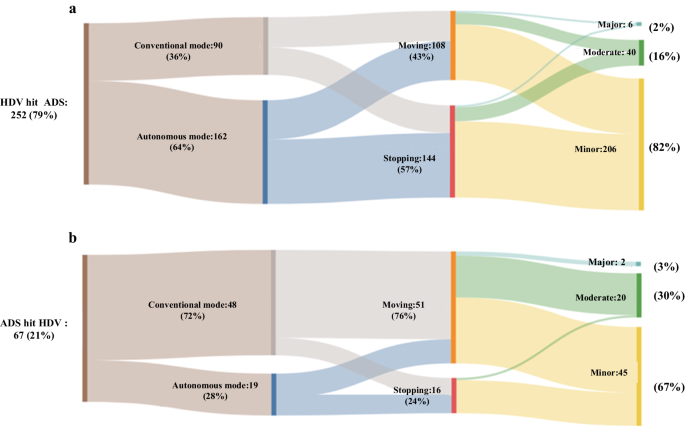
a Rear-end accidents that HDV hit an ADS from behind with a sample of 252. b Rear-end accidents that ADS hit an HDV from behind with a sample of 67.
The analysis reveals that 79% of rear-end accidents involve HDV hitting ADS, while 21% of rear-end accidents involve ADS hitting HDV. When the ADS hit HDV in conventional mode, most of the ADS are moving. We may conclude that compared with the autonomous mode, human drivers may not react as quickly or may not notice the object in time to take appropriate action. In terms of accident severity, 206 of accidents (82%) occur as minor injury when HDV hit ADS. This percentage is 67% when the ADS hit HDV. It is important to note that a majority of moderate and major accidents involving an ADS hitting an HDV occur when both vehicles are moving in the conventional mode. Notably, in cases where HDVs hit ADS, 64% of ADS are operating in autonomous mode. Conversely, when ADS are responsible for hitting HDVs, 72% of ADS are operating in the conventional mode. According to Dixit et al. in 2016, 56.1% of disengagements were attributed to system failures, 26.57% were initiated by the driver, and 9.89% were related to road infrastructure issues 24 . This observation suggests that conventional mode occurred more frequently than autonomous mode where ADS hit the HDV. This may be attributed to the advance autonomous mode of ADS. Autonomous mode uses advanced algorithms to detect and avoid obstacles and other vehicles in the path of the vehicle 32 .
We also contrast the ADS vs the ADAS, accidents related to ADAS and ADS display differences across various conditions, as shown in Fig. 2c, d . Regarding weather and road conditions, ADAS has 23.34% fewer accident number in clear skies but a 13.65% higher in rain compared to ADS. For road conditions, ADAS accidents experience a 7.48% higher accident number in traffic events or work zones and 10.33% higher accident number on wet roads than ADS. Analyzing pre-accident movements, ADAS indicate a 27.91% higher accident number for proceeding straight, while reporting 3.0% fewer turning accident numbers than ADS. In terms of accident types, ADAS is 3.0% higher accident number than ADS in broadside accidents and lags by 5.4% in sideswipe accidents. From an injury outcome perspective, ADAS accidents present a 11.37% higher accident number of no-injury but a 2.1% lower number in fatal injuries against ADS. To enhance the understanding of pre-accident speeds, heatmaps that visually represent these speed patterns for ADS and ADAS vehicles are shown in Fig. 4 . This chart offers a detailed comparison of how speeds vary across different days of the week and at various times of the day. This trend can be attributed to the fact that ADAS is primarily designed for highway use, leading to a higher average pre-accident speed when compared to ADS vehicles, which are designed for a complex urban driving scenario.
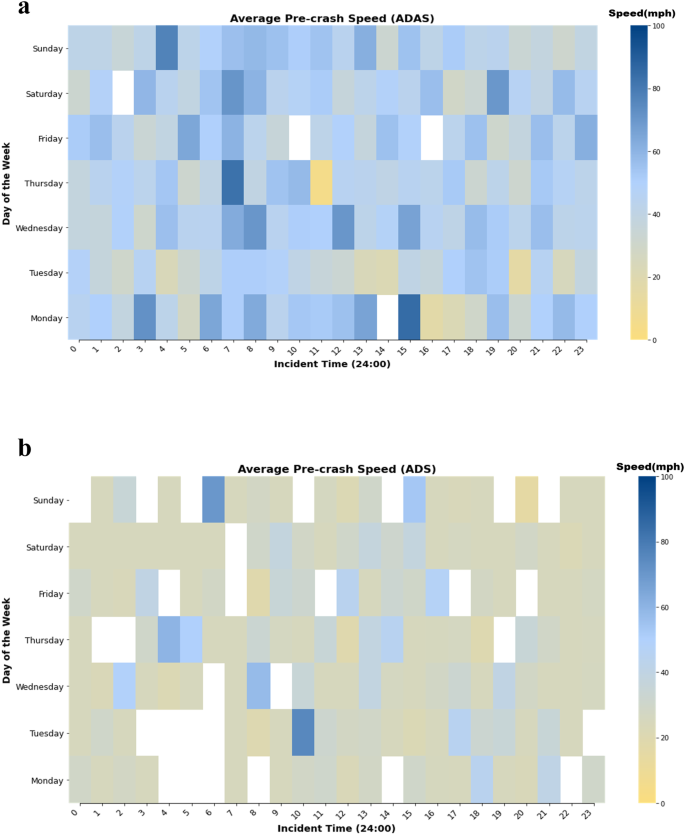
a ADAS Average Pre-accident Speed Heatmap. b ADS Average Pre-accident Speed Heatmap.
We have also analyzed the full data to identify the influence of roadway elements and factors related to time using a random parameter logit model 33 . Only a single random parameter “Day of the week” demonstrated a significant effect. Upon analyzing the model, we found that the dawn/dusk and turn conditions exhibit positive coefficients that are statistically significant at a 95% confidence level. This indicates a higher odd of an AV accident occurrence when these variables are significant in the random parameter logit model. Furthermore, we discovered that several variables demonstrate high significance and exhibit negative coefficients, suggesting a reduced likelihood of an accident when these factors are significant. These variables include the rain conditions, rear-end conditions, broadside conditions (a broadside condition is a car accident that occurs when the front of one vehicle slams into the side of another vehicle), moderate severity, proceeding straight, run-off road, backing, and entering traffic lane.
Findings of road, environment, and accident type
Based on the results of the matched case control logistic regression model, compared with HDV, the odds of an ADS accident occurring in rainy weather are 0.335 times. This indicates a lower likelihood of an ADS accident in rainy weather compared to an HDV accident. RADAR is capable of detecting objects at distances exceeding 150 m, even in adverse weather conditions such as fog or rain 34 . In contrast, human drivers may only be able to perceive objects up to approximately 10 meters away under similar circumstances 35 . Although adverse weather can increase the likelihood of potential failures or loss of sensors 36 , 37 , 38 , recent innovations in visual algorithms, coupled with the combined use of cameras, LIDAR, GNSS, and RADAR sensors 39 , 40 , are crafted to recognize pedestrians and vehicles under varying weather scenarios, such as cloudiness, snow, rain, and darkness 41 , 42 . This offers solutions to the challenges associated with driving in less-than-ideal conditions. In contrast, human drivers may have difficulties seeing through heavy rain or fog, leading to a delay in detecting potential hazards or reacting appropriately 40 .
Interestingly, the dawn/dusk odds ratio indicates a 5.250 higher probability of ADS accident than HDV accident. This could be attributed to the sensors and cameras used by AVs may not be able to quickly adapt to changes in lighting conditions, which could affect their ability to detect obstacles, pedestrians, and other vehicles 39 , 43 . At dawn and dusk, for instance, the sun’s shadows and reflections may confuse sensors, making it hard for them to distinguish between objects and identify potential hazards. Furthermore, the fluctuating light conditions can impact the accuracy of object detection and recognition algorithms used by AVs, which can result in false positives or negatives 35 , 44 .
Accident types related findings for ADS and HDVs are worth noting. Compared to HDV accidents, AVs experience relatively lower risks in rear-end and broadside accidents (0.457 times and 0.171 times, respectively). This finding indicates that AVs may detect and react to potential rear-end and sideswipe accident situations much faster than humans can. This is because they are equipped with advanced sensors and software that can quickly analyze the surrounding environment and make decisions based on the data received 45 . In addition, the kinematic method used by ACC system keeps track of and regulates the distance between vehicles, alerting drivers if this space becomes smaller than the safe limit, especially at highways 46 . By ensuring that vehicles keep a consistent speed and distance between vehicles, thus effectively reduces the risk of rear-end accidents 47 . Compared with ADS, HDVs tend to display greater velocity differences at larger spacing ranges 48 , a factor that significantly contributes to a higher incidence of rear-end and sideswipe accidents 49 .
Findings of pre-accident conditions and accident outcomes
In terms of pre-accident conditions, most of the pre-accident movements made by ADS reduce the probability of accidents from the results of the matched case control logistic regression, except for turning, which increases the likelihood of an accident by 1.988 times compared to HDVs. One possible reason is a lack of situational awareness. Situational awareness of AVs can be defined as the ability of these vehicles to perceive essential elements in their surroundings, understand the importance of these elements, and anticipate their future state or changes 50 . The complexity of turning in autonomous driving scenarios arises from three primary challenges: choosing the appropriate lane (target lane selection), devising and computing a safe and efficient path (trajectory planning and calculation), and executing the turn while adjusting to dynamic conditions (vehicle controlling and tracking) 51 . AVs rely on sensors and algorithms to perceive their surroundings and make driving decisions 45 . However, these systems may not detect all obstacles and hazards, particularly in complex and dynamic driving scenarios like turning at intersections 52 , 53 . It is a significant challenge to generate sufficient information and achieve comprehensive detection of the surrounding environment from a single independent source due to limited sensor ranges and limited coverage of the environment by sensors in AV 45 , 54 . Additionally, some AVs are programmed to follow predefined rules and scenarios, which may not encompass every possible driving situation 55 , 56 , 57 . The modifications of scenarios can present difficulty for AVs in perceiving and responding to them, thereby raising the risk of an accident 58 . Moreover, multiple oncoming HDVs and the complexity of such driving scenarios are a considerable challenge for AVs such as unprotected left turns at intersections 59 . These situations are complicated by factors like limited priority and variation in trajectories 60 . AVs tend to be overcautious (such as having a longer startup delay during the turning at intersections) 61 , which can lead to rear-end or sideswipe accidents with HDVs 62 . Furthermore, multi-interactions caused by mixed flows aggravate uncertainties in detection, such as the superposition of distance and angle measurement error 63 . Conversely, HDVs can adapt and modify their speed more seamlessly than AVs, highlighting the limitations of AVs in comparison to the adeptness of experienced drivers 64 . And AVs face difficulties with executing lane changes or turning in heavy traffic and lack psychological insight 65 , 66 . In addition, HDVs can predict pedestrian movements and exercise caution based on their driving experience, whereas AVs may struggle with recognizing pedestrians’ intentions, potentially leading to emergency braking or accidents due to a lack of understanding of social cues and psychological reasoning 65 , 67 , 68 .
ADS accidents are less likely to occur than HDV accidents in situations such as proceeding straight, run-off road (a vehicle leaves the designated roadway and travels onto an area that is not intended for regular traffic) and entering traffic lane conditions (a vehicle transitioning from a stationary or parked position to enter a traffic lane and become physically present within the flow of traffic). When considering the proceeding straight condition, it was found that AV accident resulted in a 0.299 lower probability of an HDV accident. Remarkably, ADS accident risk is 0.021 times as high as that of an HDV accident in run-off road condition, which can be explained by the faster reaction time of AVs 24 . AVs can detect these situations and apply corrective actions, such as adjusting the speed or steering angle 69 , 70 , more quickly and accurately than a human driver 71 , 72 . The result of matched-case control model revealed a significant correlation between the entering traffic lane condition of ADS accident, the risk of which is 0.267 times as high as HDV accident. According to the results of the matched case-control logistic regression, the impact of backing is noteworthy, which shows that the ADS is less likely to be affected than the HDV. According to the analysis, the model using accidents of AVs showed a decreased probability of accidents for moderate and fatal severity in comparison to HDV.
A comprehensive examination was performed using a dataset of AV and HDV accidents in this study. A total of 2100 AV accidents and 35,133 HDV accident records were collected, which accurately reflected the accident details. The analysis first dealt the whole available data that includes both ADS and ADAS (SAE levels 4 and 2, respectively) using general descriptive statistics, percentages, and a random parameter logit model (not shown in the paper for brevity and since results are almost consistent with the other matched model). The analysis considered four categories of variables, including accident type, road and environment, pre-accident movement, and accident outcomes.
The accident data of AV and HDV were compared using the matched case-control logistic regression. The impact of different variables on the potential of accidents in AV vs HDV was conducted using a matched case-control logistic regression model. Based on the model estimation results, it can be concluded that ADS in general are safer than HDVs in most accident scenarios for their object detection and avoidance, precision control, and better decision-making.
However, the odds ratio of an ADS accident happening under dawn/dusk or turning conditions is 5.250 and 1.988 times higher, respectively, than the probability of an HDV accident occurring under the same conditions. The possible reasons might be a lack of situational awareness in complex driving scenarios and limited driving experience of AVs 21 . Improving the safety of ADS under dawn/dusk or turning conditions necessitates a holistic approach that involves advanced sensors, robust algorithms, and smart design considerations. Key strategies include enhancing weather and lighting sensors, implementing redundancy measures, and integrating sensor data effectively. By focusing on these aspects, the safety of ADS can be significantly enhanced in challenging scenarios.
Compared with current studies that only focus on AV accidents 73 , 74 , 75 or analyze AV and HDV with limited samples 4 , 76 , 77 , this paper has analyzed the factors that contribute to AV in comparison to HDV accidents through the analysis of real-world accidents and multi-source data. Furthermore, this research encompassed both AV and HDV accidents, instead of solely concentrating on different levels of AV accidents without considering a comparison with HDV accidents. Moreover, this study addresses the issue of unbalanced data between AV and HDV accidents by employing a matched case-control study design. One of the constraints of this study is analyzing the detailed levels of AV and the specific activated ADAS or AV system in an accident. Understanding and modeling different classifications of AV versus HDV accidents can be challenging and may require more data. It would also be crucial in the future to incorporate data about right-of-way at intersections, encompassing yield signs, stop signs, priority signals, and traffic lights, to enhance the comparative analysis between AV and HDV. Future research could also benefit from consulting a group of AV experts to identify and report on the factors contributing to safety differences between HDVs and AVs. Reporting their responses could provide qualitative depth to the research findings.
Data preparation
The full AV data set includes 2100 (ADS + ADAS) accidents based on AVOID 6 (CADMV and NHTSA’s AV accident databases 31 ). Supplementary Table 3 presents the general descriptive statistics of the databases, and the description and explanation for the variables are given in Supplementary Table 4 . It provides a summary of the characteristics of the available variables that are classified into four major categories in the final data. The categories of variables include road and environmental characteristics (such as weather, road condition, road surface, and lighting conditions), pre-accident conditions (including vehicle manufacturers, AV driving modes, and pre-accident vehicle movement status), accident type, and accident outcome (accident severity), as shown in Supplementary Table 3 . Among this information, the day of week, time of day and road location are typical confounders relevant to the traffic accident risk 78 . To be specific, the risk of traffic accidents can vary by the day of the week and time of day due to differences in traffic volume and driver behavior (e.g., commuters mostly in peak periods). In addition, the road location can affect the risk of traffic accidents by influencing traffic volume, speed limits, and the presence of other risk factors such as road design factors, intersections, pedestrians, and bicyclists.
The second group of accidents data comprised information of HDV, which was gathered from the Statewide Integrated Traffic Records System (SWITRS) 79 . This format and structure of data can be matched with the AV data, we collected 35,113 cases of HDV accidents according to the year of AV accidents as the first step. The distributions of accident types (head-on, sideswipe, rear-end, broadside) for vehicle categories (HDV and ADS) are shown in Fig. 5 , which visually illustrates the frequency and proportion of each accident type in the respective locations by vehicle categories. For HDV accidents, intersections are the primary locations (significantly higher than other HDV accident location types with F = 5.1043 and p = 0.0166), where 61.5% of HDV intersection accidents are rear-end, making it the most common type. Urban streets are the second most common scenario, with head-on accounting for 48.0% of HDV street accidents. Conversely, ADS accidents occur more frequently on urban streets (significantly higher than other ADS accident location types with F = 10.4982 and p = 0.0011), where 45.6% of ADS street accidents were head-on. Accidents at intersections are the second most common for ADS, with rear-end making up 53.8% of these ADS intersection accidents.
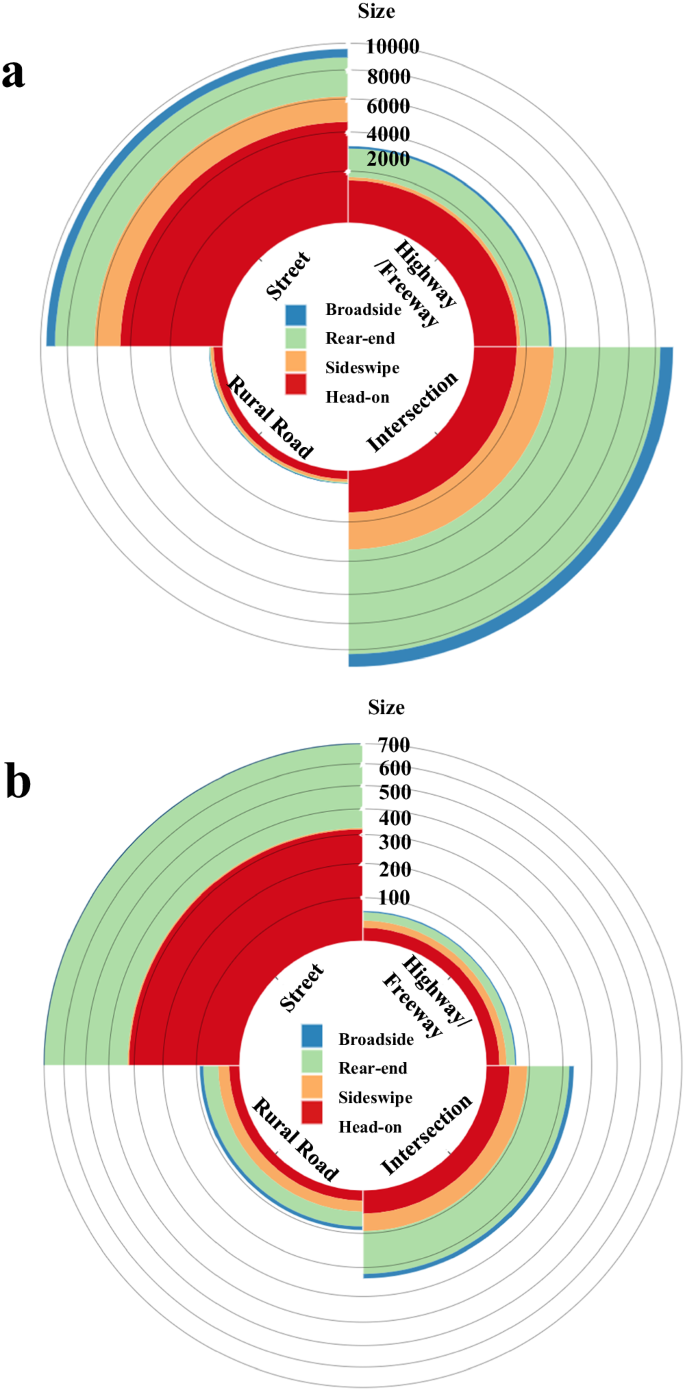
a HDV accidents with a sample of 35,113. b ADS accidents with a sample of 1099. The distributions of accident types (head-on, sideswipe, rear-end, broadside) for vehicle categories (HDV and ADS) illustrate the frequency and proportion of each accident type in the respective locations by vehicle categories.
A matched case-control logistic regression model
A matched case-control study is an observational study that involves comparing individuals who have a specific health outcome or disease (the cases) with individuals who do not have the health outcome or disease (the controls) 80 . The study design involves selecting cases and controls based on their exposure to a particular risk factor or characteristic, and then comparing the frequency of that exposure between the two groups. In the context of this paper, a matched case-control study could be used to investigate the relationship between accident-related risk factors 81 , 82 . Cases would be AVs involved in accidents, and controls would be HDVs involved in accidents.
A matched control study has been designed to investigate the impact of various factors on the likelihood of accidents in two specific scenarios: AV and HDV.
Conditional logistic regression is a variant of logistic regression that specifically tackles the issue of stratification within matched case-control studies 83 . In this research, there are N strata denoted by \(i={{{{\mathrm{1,2}}}}},\ldots \ldots,N\) . Each stratum has one AV accident case sample and k HDV accident control samples denoted by \(j={{{{\mathrm{1,2}}}}},\ldots \ldots,k\) . The conditional likelihood for the \({i\; th}\) strata depends on the probability of the total number of cases (AV accident case samples) and controls ( k HDV accident control samples) recorded in the stratum 84 . \({P}_{{ri}}\) ( \({x}_{{ji}}\) ) refers to the probability of the \({j\; th}\) samples in the \({i\; th}\) stratum is a AV accident where \({x}_{{ij}}=(\,{x}_{1{ij}},\,{x}_{2{ij}},\,{\ldots \ldots,\, x}_{{pij}})\) is determined by a vector of variables \((\,{x}_{1},\,{x}_{2},\,{\ldots \ldots,\, x}_{p})\) . A logistic regression model with linear parameters is employed to estimate the likelihood of an accident, as described by Abdel-Aty et al. 85 :
The controlled variables used to create strata are reflected in the intercept term. To incorporate the impact of stratification in the analysis, it is possible to construct a conditional log-likelihood. This log-likelihood function comprises multiple terms, each representing the conditional probability of an accident occurring within a specific stratum 86 . The following equation presents the formula for the conditional likelihood function, as stated by Abdel-Aty et al. 85 :
The coefficients’ estimates in Eq. ( 1 ) are identical to the maximum likelihood function values in Eq. ( 2 ). These estimates are log-odds ratios that can provide an approximation of the relative risk of an accident and are also referred to as hazard ratios (i.e., the ratio of odds for accident occurrence versus non-occurrence). The hazard ratio is calculated by raising the exponential value to the coefficient’s power. For a dummy variable, the odds ratio is a statistic defined as the ratio of the odds of the case. The odds ratio can be written as
where, \(Z\) represents the vector of explanatory variables excluding \({x}_{k}\) . \({\beta }_{k}\) is estimated coefficient for \({x}_{k}\) .
Matched case control study for ADS accidents
Our aim is to explore the differential characteristics of accidents involving AVs and HDVs, rather than comparing accidents and non-accidents. Direct comparisons between AV and HDV accidents are still not viable as the difference in exposure and number of vehicles of both types is extremely unbalanced. We incorporated Annual Average Daily Traffic (AADT) data of various road types from the California Traffic Census Program 87 , which is provided in the supplementary methods. HDVs show a higher incidence of accidents on highways, intersections, and streets, particularly on highways. For rural roads, HDVs and AVs exhibit almost similar accident rates. Across all road types, HDVs consistently record significantly higher accident figures than AVs. To examine the impact of exogenous variables on accident risk for different vehicle types, we conducted a matched case-control logistic regression model for AV (ADS) and HDV accidents. The coordinates of accidents are extracted by Google Map API, and then the type of road is identified to conduct matched case-control logistic regression. The distribution of AV accidents over various situations differs from the distribution of HDV accidents is concluded from the matched case-control study.
To overcome this challenge of variables that confound the relationship between risk factors and traffic accident outcomes, the first principle is to match cases and controls at the same location. In the case of a location that does not have enough controls, similar locations within a radius of 5 miles for intersections and urban segments were used, and the day of the week and time of day were controlled. We assumed that cases and controls were under similar traffic patterns based on the controlled time and space. Aside from intersections and streets, the location of each stratum for AV and HDV accidents is on the same highways and expressways. In addition, the same road type for each stratum is controlled to ensure similar geometric design. As the manual override and conventional modes of ADAS closely resemble HDV, we only focus on ADS cases from CA for the matched case-control study. Furthermore, some cases were removed due to difficulties in obtaining or imputing precise road types for matched case-control logistic regression.
We organized the data into N strata according to the occurrence of AV accidents. Each stratum consisted of one case and k corresponding controls. To ensure consistency across strata, we employed a matched case-control logistic regression by adjusting the number of control samples and assessed the resulting estimates for each model. Samples generally refer to the groups of accidents selected for comparison within each stratum. Case samples are specific accidents who have the outcome that is the focus of the study. Control samples are accidents who do not have the specific outcome being studied. In this study, ‘AV accident case sample’ consists of AV accidents within each stratum, and the ‘HDV accident control samples’ consist of the HDV accidents within the same stratum. The method begins by utilizing an initial equal proportion of AV accident case samples to HDV accident control samples (1:1) and progressively increasing the ratio (1:3, 1:5, 1:7, 1:9…) until the coefficients between consecutive models exhibit no significant change. From Fig. 6 , it is evident that there are no notable disparities between the models employing sample ratios of 1:5 and 1:6. As a result, we opt for the 1:5 ratio for our analysis. To further support our decision, we evaluate the improvement in log-likelihood across the models, aligning with our hypothesis of selecting an AV accident case sample to HDV-accident control sample ratio of 1:5.
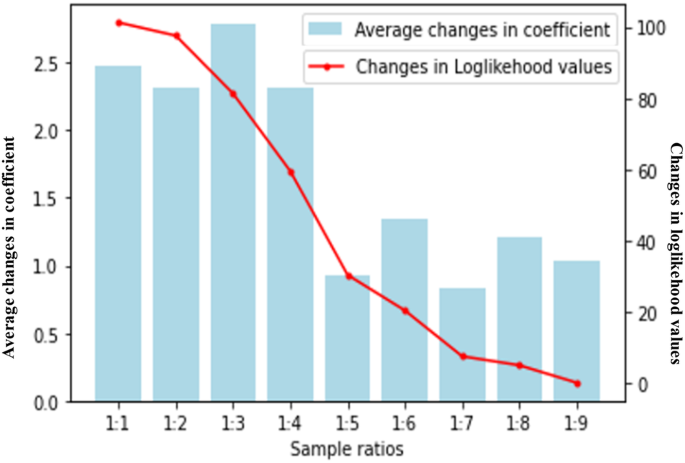
The blue column line indicates the average coefficient changes (left y-axis), and the red line shows the changes in loglikelihood values (right y-axis).
As a result, 548 ADS accident accidents were applied for the matched case-control design and are discussed in this paper. The sample of the data is shown in Fig. 7 . The estimation results and 95% confidence intervals of the odds ratio are presented in Table 1 , which was generated using the survival package in R programming 88 . A total of 11 significant variables were identified by combining road and environment, accident type, accident outcomes, and pre-accident conditions during the estimation process.
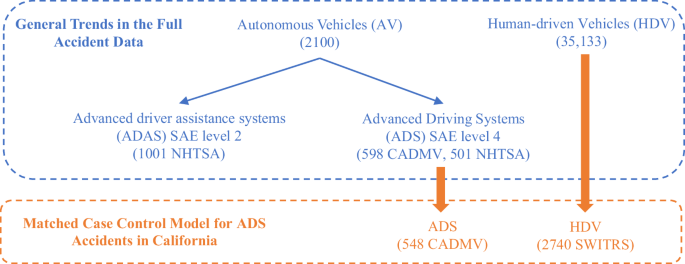
The blue fonts indicate general accident trends, while the orange fonts represent data for the matched case control model of ADS accidents in California. The HDV data is sourced from SWITRS 79 . The ADS (SAE Level 4) data is sourced from CADMV 5 and NHTSA 31 , while the ADAS (SAE Level 2) data is sourced from NHTSA 31 .
Data availability
The Human Driven-Vehicle (HDV) accidents dataset that we used to is publicly available at https://www.chp.ca.gov/programs-services/services-information/switrs-internet-statewide-integrated-traffic-records-system . The Autonomous Vehicle (AV) accidents dataset is available at https://github.com/UCF-SST-Lab/AVOID-Autonomous-Vehicle-Operation-Incident-Dataset/tree/main/asset . The Annual Average Daily Traffic (AADT) data of various road types from the California Traffic Census Program is available at https://dot.ca.gov/programs/traffic-operations/census . Source data for figures are provided with this paper. All other data used in this study are available from the corresponding authors upon request. Source data are provided with this paper.
Code availability
The codes for data validation and processing are available on Zenodo with a ( https://doi.org/10.5281/zenodo.11081206 ). The quick tutorial and README file are also included in the repository for reference. Python scripts for geospatial data processing are prepared with the OSMnX package and offered in the repository, which can be referred to in the file Address2OSM.ipynb under the folder of code. All other code used in this study are available from the corresponding authors upon request.
Fleetwood, J. Public health, ethics, and autonomous vehicles. Am. J. Public Health 107 , 532–537 (2017).
Article PubMed PubMed Central Google Scholar
Lee, D. & Hess, D. J. Regulations for on-road testing of connected and automated vehicles: Assessing the potential for global safety harmonization. Transp. Res. Part A 136 , 85–98 (2020).
Google Scholar
Zhang, L. Cruise’s Safety Record Over 1 Million Driverless Miles , https://getcruise.com/news/blog/2023/cruises-safety-record-over-one-million-driverless-miles (2023).
Liu, Q., Wang, X., Wu, X., Glaser, Y. & He, L. Crash comparison of autonomous and conventional vehicles using pre-crash scenario typology. Accid. Anal. Prev. 159 , 106281 (2021).
Article PubMed Google Scholar
DMV, C. Autonomous vehicle collision reports, https://www.dmv.ca.gov/portal/vehicle-industry-services/autonomous-vehicles/autonomous-vehicle-collision-reports/ (2023).
Zheng, O. et al. AVOID: Autonomous Vehicle Operation Incident Dataset Across the Globe. Preprint at https://arxiv.org/abs/2303.12889 (2023).
Boggs, A. M., Wali, B. & Khattak, A. J. Exploratory analysis of automated vehicle crashes in California: A text analytics & hierarchical Bayesian heterogeneity-based approach. Accid. Anal. Prev. 135 , 105354 (2020).
Scanlon, J. M. et al. Waymo simulated driving behavior in reconstructed fatal crashes within an autonomous vehicle operating domain. Accident. Anal. Prev. 163 , 106454 (2021).
Article Google Scholar
Ahangarnejad, A. H., Radmehr, A. & Ahmadian, M. A review of vehicle active safety control methods: From antilock brakes to semiautonomy. J. Vib. Control 27 , 1683–1712 (2021).
Article MathSciNet Google Scholar
Bareiss, M., Scanlon, J., Sherony, R. & Gabler, H. C. Crash and injury prevention estimates for intersection driver assistance systems in left turn across path/opposite direction crashes in the United States. Traffic Inj. Prev. 20 , S133–S138 (2019).
Gordon, T. J. & Lidberg, M. Automated driving and autonomous functions on road vehicles. Vehicle System Dynamics 53 , 958–994 (2015).
Milakis, D., Van Arem, B. & Van Wee, B. Policy and society related implications of automated driving: A review of literature and directions for future research. J. Intell. Transp. Syst. 21 , 324–348 (2017).
Yue, L., Abdel-Aty, M., Wu, Y. & Wang, L. Assessment of the safety benefits of vehicles’ advanced driver assistance, connectivity and low level automation systems. Accident . Anal. Prev. 117 , 55–64 (2018).
Chan, C.-Y. Advancements, prospects, and impacts of automated driving systems. Int. J. Transp. Sci. Technol. 6 , 208–216 (2017).
Koopman, P. & Wagner, M. Autonomous vehicle safety: An interdisciplinary challenge. IEEE Intell. Transp. Syst. Mag. 9 , 90–96 (2017).
Harper, C. D., Hendrickson, C. T. & Samaras, C. Cost and benefit estimates of partially-automated vehicle collision avoidance technologies. Accid. Anal. Prev. 95 , 104–115 (2016).
Niebuhr, T., Junge, M. & Achmus, S. Expanding pedestrian injury risk to the body region level: how to model passive safety systems in pedestrian injury risk functions. Traffic Inj. Prev. 16 , 519–531 (2015).
Penmetsa, P., Sheinidashtegol, P., Musaev, A., Adanu, E. K. & Hudnall, M. Effects of the autonomous vehicle crashes on public perception of the technology. IATSS Res. 45 , 485–492 (2021).
Kalra, N. & Paddock, S. M. Driving to safety: How many miles of driving would it take to demonstrate autonomous vehicle reliability? Transp. Res. Part A 94 , 182–193 (2016).
Yan, S., Huang, C. & He, D. A comparison of patterns and contributing factors of ADAS and ADS involved crashes. J. Transp. Saf. Sec. 15 , 1–28 (2023).
Ding, S. et al. Exploratory Analysis of the Crash Severity between Vehicular Automation (SAE L2-5) with Multi-Source Data. Preprint at https://arxiv.org/abs/2303.17788 (2023).
Norris, N., Emmanuel, K., Boniphace, K. & Angela, E. K. A comparative study of collision types between automated and conventional vehicles using Bayesian probabilistic inferences. J. Saf. Res. 84 , 251–260 (2023).
Wen, X., Huang, C., Jian, S. & He, D. Analysis of discretionary lane-changing behaviours of autonomous vehicles based on real-world data. Transportmetrica A: Transport Science 19 , 1–24 (2023).
Dixit, V. V., Chand, S. & Nair, D. J. Autonomous vehicles: disengagements, accidents and reaction times. PLoS One 11 , e0168054 (2016).
Teoh, E. R. & Kidd, D. G. Rage against the machine? Google’s self-driving cars versus human drivers. J. Saf. Res. 63 , 57–60 (2017).
Schoettle, B. & Sivak, M. A preliminary analysis of real-world crashes involving self-driving vehicles (University of Michigan Transportation Research Institute, 2015).
Favarò, F. M., Nader, N., Eurich, S. O., Tripp, M. & Varadaraju, N. Examining accident reports involving autonomous vehicles in California. PLoS One 12 , e0184952 (2017).
Seacrist, T. et al. In-depth analysis of crash contributing factors and potential ADAS interventions among at-risk drivers using the SHRP 2 naturalistic driving study. Traffic Inj. Prev. 22 , S68–S73 (2021).
Sinha, A., Vu, V., Chand, S., Wijayaratna, K. & Dixit, V. A crash injury model involving autonomous vehicle: Investigating of crash and disengagement reports. Sustainability 13 , 7938 (2021).
Wang, S. & Li, Z. Exploring the mechanism of crashes with automated vehicles using statistical modeling approaches. PloS One 14 , e0214550 (2019).
Article CAS PubMed PubMed Central Google Scholar
NHTSA. Standing General Order on Crash Reporting , https://www.nhtsa.gov/laws-regulations/standing-general-order-crash-reporting#data (2023).
Ahangar, M. N., Ahmed, Q. Z., Khan, F. A. & Hafeez, M. A survey of autonomous vehicles: Enabling communication technologies and challenges. Sensors 21 , 706 (2021).
Article ADS PubMed PubMed Central Google Scholar
Yuan, R., Ding, S., Fang, Z., Gu, X., & Xiang, Q. Investigating the spatial heterogeneity of factors influencing speeding-related crash severities using correlated random parameter order models with heterogeneity-in-means. Transp. Lett. 15 , 1−13 (2023).
Sun, Z., Bebis, G. & Miller, R. On-road vehicle detection: A review. IEEE Trans. Pattern Anal. Mach. Intell. 28 , 694–711 (2006).
Zang, S. et al. The impact of adverse weather conditions on autonomous vehicles: How rain, snow, fog, and hail affect the performance of a self-driving car. IEEE Vehicular Technol. Mag. 14 , 103–111 (2019).
Gehrig, S., Reznitskii, M., Schneider, N., Franke, U. & Weickert, J. Priors for stereo vision under adverse weather conditions. In Proceedings of the IEEE International Conference on Computer Vision Workshops . 238–245 (2013).
Cui, Z., Yang, S.-W. & Tsai, H.-M. A vision-based hierarchical framework for autonomous frontvehicle taillights detection and signal recognition. In 2015 IEEE 18th International Conference on Intelligent Transportation Systems . 931–937 (IEEE, 2015).
Hnewa, M. & Radha, H. Object detection under rainy conditions for autonomous vehicles: A review of state-of-the-art and emerging techniques. IEEE Signal Process. Mag. 38 , 53–67 (2020).
Vargas, J., Alsweiss, S., Toker, O., Razdan, R. & Santos, J. An Overview of Autonomous Vehicles Sensors and Their Vulnerability to Weather Conditions. Sensors 21 , 5397 (2021).
Van Brummelen, J., O’Brien, M., Gruyer, D. & Najjaran, H. Autonomous vehicle perception: The technology of today and tomorrow. Transp. Res. Part C 89 , 384–406 (2018).
Radecki, P., Campbell, M. & Matzen, K. All weather perception: Joint data association, tracking, and classification for autonomous ground vehicles. Preprint at https://arxiv.org/abs/1605.02196 (2016).
Filgueira, A., González-Jorge, H., Lagüela, S., Díaz-Vilariño, L. & Arias, P. Quantifying the influence of rain in LiDAR performance. Measurement 95 , 143–148 (2017).
Article ADS Google Scholar
Parekh, D. et al. A review on autonomous vehicles: Progress, methods and challenges. Electronics 11 , 2162 (2022).
Khatab, E., Onsy, A., Varley, M. & Abouelfarag, A. Vulnerable objects detection for autonomous driving: A review. Integration 78 , 36–48 (2021).
Yeong, D. J., Velasco-Hernandez, G., Barry, J. & Walsh, J. Sensor and sensor fusion technology in autonomous vehicles: A review. Sensors 21 , 2140 (2021).
Alotibi, F. & Abdelhakim, M. Anomaly detection for cooperative adaptive cruise control in autonomous vehicles using statistical learning and kinematic model. IEEE Trans. Intell. Transp. Syst. 22 , 3468–3478 (2020).
Li, Y. et al. Evaluation of the impacts of cooperative adaptive cruise control on reducing rear-end collision risks on freeways. Accid. Anal. Prev. 98 , 87–95 (2017).
Article ADS PubMed Google Scholar
Adewale, A. & Lee, C. Prediction of car-following behavior of autonomous vehicle and human-driven vehicle based on drivers’ memory and cooperation with lead vehicle. Transp. Res. Record. , https://doi.org/10.1177/03611981231195051 (2023).
Li, Y., Wu, D., Lee, J., Yang, M. & Shi, Y. Analysis of the transition condition of rear-end collisions using time-to-collision index and vehicle trajectory data. Accid. Anal. Prev. 144 , 105676 (2020).
Endsley, M. R. Toward a theory of situation awareness in dynamic systems. Hum. factors 37 , 32–64 (1995).
Ding, Z., Sun, C., Zhou, M., Liu, Z. & Wu, C. Intersection vehicle turning control for fully autonomous driving scenarios. Sensors 21 , 3995 (2021).
Levin, M. W. & Boyles, S. D. Intersection auctions and reservation-based control in dynamic traffic assignment. Transp. Res. Rec. 2497 , 35–44 (2015).
Haris, M. & Hou, J. Obstacle detection and safely navigate the autonomous vehicle from unexpected obstacles on the driving lane. Sensors 20 , 4719 (2020).
Bhavsar, P., Das, P., Paugh, M., Dey, K. & Chowdhury, M. Risk analysis of autonomous vehicles in mixed traffic streams. Transp. Res. Rec. 2625 , 51–61 (2017).
Fagnant, D. J. & Kockelman, K. Preparing a nation for autonomous vehicles: opportunities, barriers and policy recommendations. Transp. Res. Part A 77 , 167–181 (2015).
Zhang, Q. et al. A systematic framework to identify violations of scenario-dependent driving rules in autonomous vehicle software. Proc. ACM Meas. Anal. Comput. Syst. 5 , 1–25 (2021).
CAS Google Scholar
Riedmaier, S., Ponn, T., Ludwig, D., Schick, B. & Diermeyer, F. Survey on scenario-based safety assessment of automated vehicles. IEEE Access 8 , 87456–87477 (2020).
Kutela, B., Avelar, R. E. & Bansal, P. Modeling automated vehicle crashes with a focus on vehicle at-fault, collision type, and injury outcome. J. Transp. Eng. Part A 148 , 04022024 (2022).
Zhou, D., Ma, Z., Zhang, X. & Sun, J. Autonomous vehicles’ intended cooperative motion planning for unprotected turning at intersections. IET Intell. Transp. Syst. 16 , 1058–1073 (2022).
Wael, K. M. A., Miho, A., Hideki, N. & Dang Minh, T. Stochastic approach for modeling the effects of intersection geometry on turning vehicle paths. Transp. Res. Part C. 32 , 179–192 (2013).
Noh, S. Decision-making framework for autonomous driving at road intersections: Safeguarding against collision, overly conservative behavior, and violation vehicles. IEEE Trans. Ind. Electron. 66 , 3275–3286 (2018).
Ashraf, M. T., Dey, K., Mishra, S. & Rahman, M. T. Extracting rules from autonomous-vehicle-involved crashes by applying decision tree and association rule methods. Transp. Res. Rec. 2675 , 522–533 (2021).
Zhou, D., Ma, Z. & Sun, J. Autonomous Vehicles’ Turning Motion Planning for Conflict Areas at Mixed-Flow Intersections. IEEE Trans. Intell. Veh. 5 , 204–216 (2020).
Grahn, H., Kujala, T., Silvennoinen, J., Leppänen, A. & Saariluoma, P. Expert drivers’ prospective thinking-aloud to enhance automated driving technologies–Investigating uncertainty and anticipation in traffic. Accid. Anal. Prev. 146 , 105717 (2020).
Lake, B. M., Ullman, T. D., Tenenbaum, J. B. & Gershman, S. J. Building machines that learn and think like people. Behav. Brain Sci. 40 , e253 (2017).
Zhang, Y., Wang, W., Zhou, X., Wang, Q. & Sun, X. Tactical-level explanation is not enough: Effect of explaining AV’s lane-changing decisions on drivers’ decision-making, trust, and emotional experience. Int. J. Hum. Comput. Interact. 39 , 1438–1454 (2023).
Rasouli, A. & Tsotsos, J. K. Autonomous vehicles that interact with pedestrians: A survey of theory and practice. IEEE Trans. Intell. Transp. Syst. 21 , 900–918 (2019).
Schwarting, W., Pierson, A., Alonso-Mora, J., Karaman, S. & Rus, D. Social behavior for autonomous vehicles. Proc. Natl Acad. Sci. 116 , 24972–24978 (2019).
Article ADS MathSciNet CAS PubMed PubMed Central Google Scholar
Raouf, I. et al. Sensor-based prognostic health management of advanced driver assistance system for autonomous vehicles: A recent survey. Mathematics 10 , 3233 (2022).
Lee, S., Arvin, R. & Khattak, A. J. Advancing investigation of automated vehicle crashes using text analytics of crash narratives and Bayesian analysis. Accid. Anal. Prev. 181 , 106932 (2023).
Cui, J., Sabaliauskaite, G., Liew, L. S., Zhou, F. & Zhang, B. Collaborative analysis framework of safety and security for autonomous vehicles. IEEE Access 7 , 148672–148683 (2019).
Sun, X., Cao, S. & Tang, P. Shaping driver-vehicle interaction in autonomous vehicles: How the new in-vehicle systems match the human needs. Appl. Ergonomics 90 , 103238 (2021).
Kutela, B., Das, S. & Dadashova, B. Mining patterns of autonomous vehicle crashes involving vulnerable road users to understand the associated factors. Accid. Anal. Prev. 165 , 106473 (2022).
Xu, C., Ding, Z., Wang, C. & Li, Z. Statistical analysis of the patterns and characteristics of connected and autonomous vehicle involved crashes. J. Saf. Res. 71 , 41–47 (2019).
Zhu, S. & Meng, Q. What can we learn from autonomous vehicle collision data on crash severity? A cost-sensitive CART approach. Accid. Anal. Prev. 174 , 106769 (2022).
Cascetta, E., Carteni, A. & Di Francesco, L. Do autonomous vehicles drive like humans? A Turing approach and an application to SAE automation Level 2 cars. Transp. Res. Part C. 134 , 103499 (2022).
Petrović, Đ., Mijailović, R. & Pešić, D. Traffic accidents with autonomous vehicles: type of collisions, manoeuvres and errors of conventional vehicles’ drivers. Transp. Res. Proc. 45 , 161–168 (2020).
Gross, F. & Jovanis, P. P. Estimation of the safety effectiveness of lane and shoulder width: Case-control approach. J. Transp. Eng. 133 , 362–369 (2007).
SWITRS. Statewide Integrated Traffic Records System , https://iswitrs.chp.ca.gov/Reports/jsp/index.jsp (2023).
Ingram, D., Sanders, K., Kolybaba, M. & Lopez, D. Case-control study of phyto-oestrogens and breast cancer. Lancet 350 , 990–994 (1997).
Article CAS PubMed Google Scholar
Abdel-Aty, M. A., Hassan, H. M., Ahmed, M. & Al-Ghamdi, A. S. Real-time prediction of visibility related crashes. Transp. Res. part C 24 , 288–298 (2012).
Ahmed, M. M., Abdel-Aty, M. & Yu, R. Bayesian updating approach for real-time safety evaluation with automatic vehicle identification data. Transp. Res. Rec. 2280 , 60–67 (2012).
Rahman, M. M. & Lamsal, B. P. Ultrasound‐assisted extraction and modification of plant‐based proteins: Impact on physicochemical, functional, and nutritional properties. Compr. Rev. Food Sci. Food Saf. 20 , 1457–1480 (2021).
Rahman, R., Bhowmik, T., Eluru, N. & Hasan, S. Assessing the crash risks of evacuation: A matched case-control approach applied over data collected during Hurricane Irma. Accid. Anal. Prev. 159 , 106260 (2021).
Abdel-Aty, M., Uddin, N., Pande, A., Abdalla, M. F. & Hsia, L. Predicting freeway crashes from loop detector data by matched case-control logistic regression. Transp. Res. Rec. 1897 , 88–95 (2004).
Peck, R. C., Gebers, M. A., Voas, R. B. & Romano, E. The relationship between blood alcohol concentration (BAC), age, and crash risk. J. Saf. Res. 39 , 311–319 (2008).
Program, T. C. Traffic Census Program , https://dot.ca.gov/programs/traffic-operations/census (2024).
Therneau, T. M., Lunley, T., Atkinson, E. & Crowson, C. survival: Survival analysis. https://cran.r-project.org/web/packages/survival/index.html (2024).
Download references
Acknowledgements
The authors wish to thank Dr. Ou Zheng for his role in creating the AVOID data used in this study.
Author information
Authors and affiliations.
Smart and Safe Transportation Lab (SST), Department of Civil, Environmental and Construction Engineering, University of Central Florida, 12800 Pegasus Dr, Orlando, FL, 32816, USA
Mohamed Abdel-Aty & Shengxuan Ding
You can also search for this author in PubMed Google Scholar
Contributions
M.A.A. and S.D. conceived the study. M.A.A. and S.D. wrote the manuscript. M.A.A. and S.D. estimated the models and conducted the analysis. M.A.A. supervised the analysis and edited the manuscript.
Corresponding author
Correspondence to Shengxuan Ding .
Ethics declarations
Competing interests.
The authors declare no competing interests.
Peer review
Peer review information.
Nature Communications thanks Yee Mun Lee and the other, anonymous, reviewer(s) for their contribution to the peer review of this work. A peer review file is available.
Additional information
Publisher’s note Springer Nature remains neutral with regard to jurisdictional claims in published maps and institutional affiliations.
Supplementary information
Supplementary information, peer review file, source data, source data, rights and permissions.
Open Access This article is licensed under a Creative Commons Attribution 4.0 International License, which permits use, sharing, adaptation, distribution and reproduction in any medium or format, as long as you give appropriate credit to the original author(s) and the source, provide a link to the Creative Commons licence, and indicate if changes were made. The images or other third party material in this article are included in the article’s Creative Commons licence, unless indicated otherwise in a credit line to the material. If material is not included in the article’s Creative Commons licence and your intended use is not permitted by statutory regulation or exceeds the permitted use, you will need to obtain permission directly from the copyright holder. To view a copy of this licence, visit http://creativecommons.org/licenses/by/4.0/ .
Reprints and permissions
About this article
Cite this article.
Abdel-Aty, M., Ding, S. A matched case-control analysis of autonomous vs human-driven vehicle accidents. Nat Commun 15 , 4931 (2024). https://doi.org/10.1038/s41467-024-48526-4
Download citation
Received : 30 September 2023
Accepted : 02 May 2024
Published : 18 June 2024
DOI : https://doi.org/10.1038/s41467-024-48526-4
Share this article
Anyone you share the following link with will be able to read this content:
Sorry, a shareable link is not currently available for this article.
Provided by the Springer Nature SharedIt content-sharing initiative
By submitting a comment you agree to abide by our Terms and Community Guidelines . If you find something abusive or that does not comply with our terms or guidelines please flag it as inappropriate.
Quick links
- Explore articles by subject
- Guide to authors
- Editorial policies
Sign up for the Nature Briefing newsletter — what matters in science, free to your inbox daily.

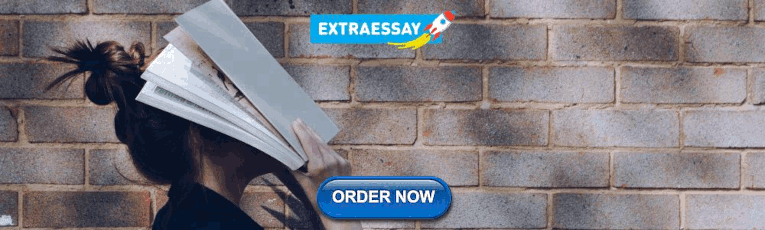
IMAGES
VIDEO
COMMENTS
7.1 Introduction. Regression analysis is one of the most fr equently used tools in market resear ch. In its. simplest form, regression analys is allows market researchers to analyze rela tionships ...
The paper depended on logistic regression model because the dependent variable is nominal. ... and determine the research variables and regression equations in the model. Starting from the ...
Abstract. Although regression models play a central role in the analysis of medical research projects, there still exist many misconceptions on various aspects of modeling leading to faulty analyses. Indeed, the rapidly developing statistical methodology and its recent advances in regression modeling do not seem to be adequately reflected in ...
Machine learning models have been able to have an excellent position in this field and provide admirable results. This paper examines and compares various regression models and machine learning algorithms. The selected techniques include multiple linear regression (MLR), ridge regression (RR), least absolute shrinkage and selection operator ...
Linear regression is used to study the linear relationship between a dependent variable Y (blood pressure) and one or more independent variables X (age, weight, sex). The dependent variable Y must be continuous, while the independent variables may be either continuous (age), binary (sex), or categorical (social status).
A quantitative research approach was applied, and correlation analysis, multiple regression analysis and structural equation modelling were used to analyse data.Main findings: The study ...
The significance and value of regression coefficients and R 2 for a model with both regression coefficients positive, E(W|H,J) = 0.7H + 0.08J - 46.5 + ε. The format of the figure is the same as ...
These questions can in principle be answered by multiple linear regression analysis. In the multiple linear regression model, Y has normal distribution with mean. The model parameters β 0 + β 1 + +β ρ and σ must be estimated from data. β 0 = intercept. β 1 β ρ = regression coefficients.
Although regression models play a central role in the analysis of medical research projects, there still exist many misconceptions on various aspects of modeling leading to faulty analyses. Indeed, the rapidly developing statistical methodology and its recent advances in regression modeling do not seem to be adequately reflected in many medical publications. This problem of knowledge transfer ...
In a linear regression model, the dependent variable must be continuous ... Logistic regression in medical research. Anesth Analg. 2021;132:365-6. Article Google Scholar
Linear Regression is a method for modelling a relationship between a dependent variable and independent variables. ... $. We can also define the problem in probabilistic terms as a generalized linear model (GLM) where the pdf is a Gaussian distribution, and then perform maximum likelihood estimation to estimate $\hat{\beta}$. ... Stay informed ...
In this study, data for multilinear regression analysis is occur from Sakarya University Education Faculty student's lesson (measurement and evaluation, educational psychology, program development, counseling and instructional techniques) scores and their 2012- KPSS score. Assumptions of multilinear regression analysis- normality, linearity, no ...
In both cases, we still use the term 'linear' because we assume that the response variable is directly related to a linear combination of the explanatory variables. The equation for multiple linear regression has the same form as that for simple linear regression but has more terms: = 0 +. 1 +. 2 + ⋯ +.
Linear regression refers to the mathematical technique of fitting given data to a function of a certain type. It is best known for fitting straight lines. In this paper, we explain the theory behind linear regression and illustrate this technique with a real world data set. This data relates the earnings of a food truck and the population size of the city where the food truck sells its food.
Least squares linear regression is one of the oldest and widely used data analysis tools. Although the theoretical analysis of the ordinary least squares (OLS) estimator is as old, several fundamental questions are yet to be answered. Suppose regression observations $(X_1,Y_1),\\ldots,(X_n,Y_n)\\in\\mathbb{R}^d\\times\\mathbb{R}$ (not necessarily independent) are available. Some of the ...
Regression analysis is a quantitative research method which is used when the study involves modelling and analysing several variables, where the relationship includes a dependent variable and one or more independent variables. In simple terms, regression analysis is a quantitative method used to test the nature of relationships between a dependent variable and one or more independent variables.
Regression analysis is a statistical method to analyze financial data, commonly using the least square regression technique. The regression analysis has significance for all the fields of study, and almost all the fields apply least square regression methods for data analysis. However, the ordinary least square regression technique can give misleading and wrong results in the presence of ...
Using the linear regression model, we found a compound correlation coefficient R of 0.463 and a coefficient of determination R² of 0.214, which implies that income satisfaction explains 21.4% of ...
Abstract—In this paper, market values of the football players in the forward positions are estimated using multiple. linear regression by including the physical and performance factors in 2017-2018 season. Players from 4 major. leagues of Europe are examined, and by applying Breusch - Pagan test for homoscedasticity, a reasonable regression.
Home environment and reading achievement research has been largely dominated by a focus on early reading acquisition, while research on the relationship between home environments and reading success with preadolescents (Grades 4-6) has been largely overlooked. There are other limitations as well. Clarke and Kurtz-Costes (1997) argued that prior ...
Regression analysis is a statistical technique for determining the relationship between a single dependent (criterion) variable and one or more independent (predictor) variables. The analysis yields a predicted value for the criterion resulting from a linear combination of the predictors. According to Pedhazur, 15 regression analysis has 2 uses ...
The Biometrical Journal publishes papers on statistical methods and their applications to life sciences, ... CS is the slope term in a logistic regression model, where the linear predictor is regressed on the binary outcome. ... This article has earned an open data badge "Reproducible Research" for making publicly available the code ...
Linear regression models. Linear regression models describe the relationship between a dependent variable and one or more independent variables. The goal is to find the best fit straight line that minimizes the sum of squared residuals of the linear regression model. The least squares method is the most common method used to fit a regression line.
Model-Agnostic Meta-Learning for Fast Adaptation of Deep Networks. cbfinn/maml • • ICML 2017 We propose an algorithm for meta-learning that is model-agnostic, in the sense that it is compatible with any model trained with gradient descent and applicable to a variety of different learning problems, including classification, regression, and reinforcement learning.
Based on the results of the matched case control logistic regression model, compared with HDV, the odds of an ADS accident occurring in rainy weather are 0.335 times.