- Overview Architecture Enterprise Models Business Area Models Data Warehouse Models
- Industry Models Communications & Media CDMA Wireless GSM / 3G / 4G / 5G Wireless Internet & Data Services Landline Communications Radio Broadcasting Television Broadcasting Financial Services Brokerage Card Services Commercial Banking Corporate & Investment Banking Credit Unions Mortgage Services Mutual Funds & Fund Management Payment Services Retail Banking Govt & Information Services Credit Reporting Services Government Services IT Services National Postal Services Smart Cities High Tech Products Computers High Tech Components Medical Systems Mobile Wireless Devices Networking Equipment Semiconductors Software Manufacturing Apparel & Footwear Automotive Products Aviation Products Consumer Packaged Goods (CPG) Beauty Products Cleaning Products Food & Beverage Products Home Healthcare Products Durable Medical Equipment Heavy Vehicle Manufacturing Luxury Goods Pharmaceuticals Tire Manufacturing Insurance & Healthcare Healthcare Insurance Healthcare Providers Life Insurance & Annuities Property & Casualty Insurance Petroleum, Chemicals & Mining Agricultural Chemicals Coal Mining Copper Mining Industrial Chemicals Iron Ore Mining Oil Sands Mining Oil & Gas - Upstream Natural Gas Processing & Fractionation Oil & Gas - Midstream Petroleum Refining Oil & Gas - Downstream Polypropylene Production Methanol Production Retailing & Distribution Apparel & Footwear Beauty Products Building Materials Grocery Jewelry Optical Products Pharmacy Transportation, Travel & Leisure Airlines Car Rental Services Freight & Logistics Gaming & Betting Hotel Services Lotteries Parcel & Mail Delivery Quick Service Restaurants Restaurant Services Travel Services Utilities Gas & Electric Utilities Water Utilities
- Solution Models Energy & Utilities Desalination - Multi-Effect Distillation (MED) ** --> Desalination - Multi-Stage Flash Distillation (MSF) Desalination - Reverse Osmosis (RO) Electricity Generation - Biomass Electricity Generation - Coal Electricity Generation - Gas & Oil Electricity Generation - Hydro Electricity Generation - Nuclear Electricity Generation - Solar Electricity Generation - Wind Energy & Commodity Trading Financial Services Basel III STP / Securities Back Office Healthcare & Life Sciences ePedigree Genomics R&D and Clinical Trials
- Business Area Models Accounting & Financial Reporting Bookings / Billings / Backlog Budget Business Metrics Channel Contract Customer Service Geography Human Resources Individual Customer Individual Customer Credit & Collections Inventory Legal Entity Customer Legal Entity Customer Credit & Collections Manufacturing / Shop Floor Control Marketing & Advertising Order Party Products & Services Property & Equipment Purchasing Training & Education
- Downloads Data Model Datasheet Data Model Product Brochure --> ADRM Named to Banking CIO Outlook Top 10 The Best-Practice Vertical-Industry Enterprise Model
- About Company Partners Events News --> FAQs Contact
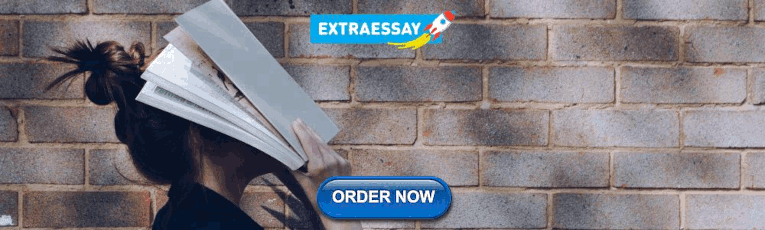
Industry Data Model
Upstream oil & gas.
The upstream segment of oil and gas industry encompasses exploration and production (E&P) activities related to exploring for, recovery and production of crude oil, natural gas, and natural gas liquids (NGLs).
ADRM Software's Upstream Oil & Gas data model set consists of Enterprise , Business Area , and Data Warehouse logical data models that address the information requirements of upstream oil and gas companies engaged in any or all of the three primary upstream functions:
- Exploration
The primary emphasis of exploration relates to wells: determining appropriate locations, depths and drilling and management techniques. Exploration also involves the operator obtaining leases and permission to drill from the owners of onshore or offshore property and/or drilling rights in areas considered prospective to contain oil and/or gas
Drilling involves the creation of a borehole in the ground to become an oil or gas well. Wells can be simple or extremely complex requiring considerable planning, resources and risk as with 'deviated wells' that emanate from original or 'mother' wells. Drilling work is typically done by contractors and services companies with as many as 30-40 different service contractors providing expertise and services.
Production is bringing oil and gas to the surface via one or more techniques that might include:
- Horizontal drilling
- Hydraulic fracturing
Oil and gas which is produced and brought to the surface is either temporarily stored locally or immediately offloaded into midstream infrastructure or transportation options such as trucks or railcars. Upstream oil and gas activities entail high risk and are information and capital intensive. Upstream oil and gas activities are also highly regulated in terms of pricing, taxation, drilling rights and reserve calculations. The primary participants are the major oil companies, national oil companies (NOC's), Independents, and oilfield services companies.
Many upstream oil and gas organizations are also involved in trading activities, often for the purpose of hedging their anticipated future production, and may therefore be interested to learn more about ADRM's Energy & Commodity Trading Solution Model .

- Vision & Strategy
- Board of Directors
- Board Nominations Committee
- PPDM Association ByLaws
- Conflict of Interest Policy
- CPDA Exam Accommodations and Provisions
- PPDM Access Policy
- PPDM Anti-Corruption and Bribery Policy
- PPDM Association Sanction Policy
- PPDM Competition Policy (Anti-Trust)
- PPDM Membership Agreement and IP Policy
- PPDM Privacy Policy
- PPDM Services and Website Terms and Conditions
- PPDM Voting Policy
- The PPDM Way
- PPDM Overview Videos
- Regional Leadership Teams
- Editorial Committee
- Council of Chairs
Banff Protocol
Data objects.
- Hydraulic Fracturing
- Reference Values List Committee
- Rules Committee
What is Seismic?
- Compliance with Teeth
What is a Facility
- What is a Well Milestone
- Competency-Based Learning
- Professional Development Committee
- Education Advisory Committee
- Certification Committee
- Upcoming Events
- Sponsorship & Exhibiting
- Advertising
- Abstract Submissions
- Past Events
- Industry Events Guidelines
- Data Model Compliance
- PPDM 3.9 Data Model
- PPDM 3.8 Data Model
- PPDM Lite 1.1 Data Model
- Sample Data Sets
- Architecture Terminology Glossary
- Reference Values List
- Taxonomy Dimensions Info
- US Well Number Standard
- Canadian Well Identification System Standard
- Global Framework for Well Identification
Well Status & Classification
- What is a Completion?
- What is a Well Application
- WITSML Mapping
- Who Should Certify
- How to Apply
- How to Prepare
- Credential Registry
- Credential Maintenance
- Sample Questions
- CPDA Resources
- CPDA Spotlight
- Ethical Integrity Policy
- Private Training Request
- Job Descriptions
- Job Families Grid
- Competency Framework
- Job Competency Mapping Tool
- Job Market Survey
- Job Market Survey Report
- Training Catalogue
- Certification Committee Information
- Professional Development Committee Information
- Volunteer Now
- Annual Reports
- Technical Publications
- Editorial Guidelines
- Foundations Index
- Useful Resources
- PPDM Future
- Well Numbering
- Data Management What is it?
- Data Management as a Career
- Data Examiner
- Marketplace Spot Reservation
- How to Become a Volunteer
- Volunteer Opportunities
- Volunteer Resources
- Volunteer Recognition
- Tracking your Volunteer Hours
- Award Winners
- Testimonials
IEDS (International Energy Data Standards)
The ppdm association defines and creates standards to help oil and gas companies manage exploration and production data..
The members of the PPDM Association provide critical input and help to set the strategic direction for the organization. Under member direction, the PPDM Association has come to represent much more than the world’s leading petroleum data model. Our organization has also been instrumental in developing and introducing oil and gas data management standards that are built around business rules and best practices.
PPDM - WITSML Mapping
Reference values, taxonomy dimensions, well identification, what is a completion, what is a well, ppdm data model compliance.
- Share on Facebook
- Share on Twitter
- Share on LinkedIn
- More options
- StumbleUpon
- Choosing the data use cases that are important to the business
- Unlocking the key data assets that power those use cases
- Governing the data and updating the architecture that makes the data assets accessible

A Ninety-Day Plan to Build a Data and Digital Strategy

Digital Acceleration Is Just a Dream Without a New Approach to Tech

Is Your Company Gaining Momentum in Data?
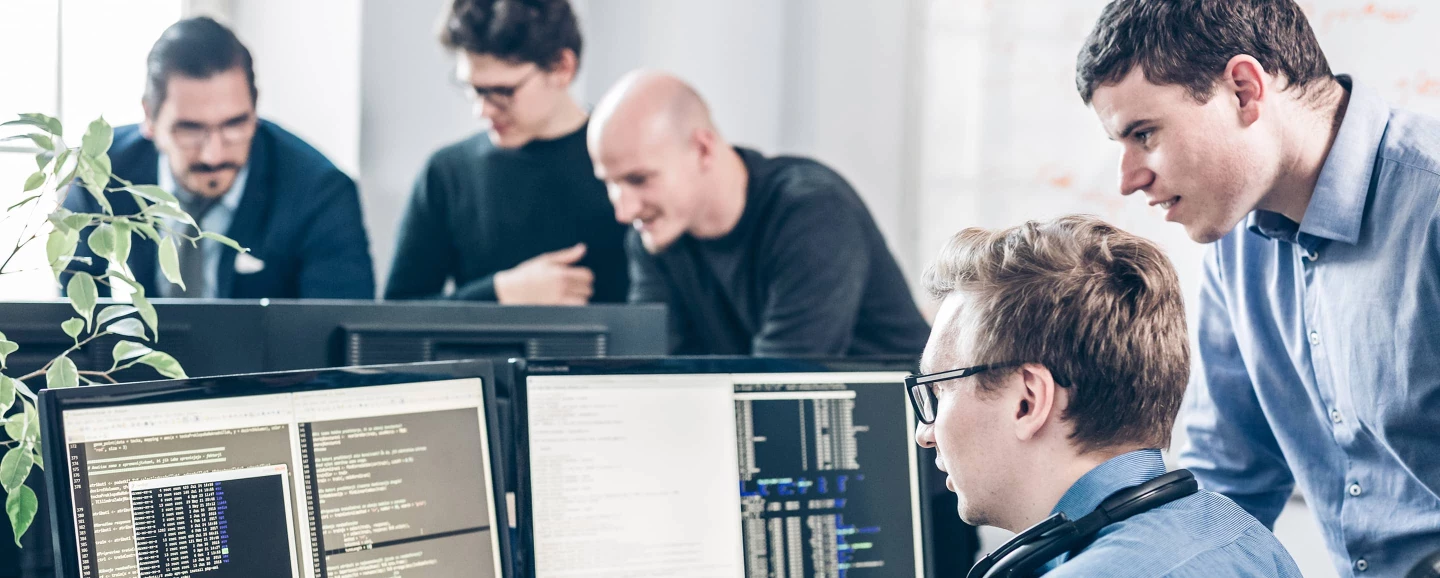
Data and Digital Platform
/ article, harnessing the power of data in oil and gas.
By Håvard Holmås , Marie-Hélène Ben Samoun , Sylvain Santamarta , Lucas Quarta , and Johan Stockmann
Key Takeaways
As the world strives for a sustainable future, no industry is more in the crosshairs than oil and gas. Every new project is scrutinized and more difficult to get underway than the next, so it’s critical that O&G companies maximize value from existing operations. One clear opportunity to manage this feat is to do more with the mountains of data that O&G companies have at their disposal by applying emerging analytics and AI capabilities to unlock new sources of value and cost savings.
Harnessing data to improve efficiencies and unlock value requires transformative change and close coordination between the business side and IT—two groups that do not always work together well. Focusing on data and digital innovation as well as on cross-functional collaboration could also help attract young, tech-savvy talent looking for an innovative and inspiring work environment.
Finding the Value
To align the business and IT, we recommend that both sides jointly define a North Star for their data and digital architecture. Key to this exercise is the creation of a data trinity : the data use cases that are important to the business, the data assets that enable those use cases, and the architecture that makes the data assets accessible. Proper prioritization of high-value use cases and data projects shapes the architecture modernization initiatives necessary to implement them.
This is a complex exercise, but turning to data and AI to unlock value is critical and real examples from the O&G industry do exist. Moreover, BCG analysis shows that investors recognize data as a rich vein of potential profits and cost savings. For example, HSBC has stated that “the opportunity for the digital oilfield to have a major impact across most parts of the value chain is significant.” Barclays estimates that digital could lower the cost of production by more than $3 per barrel, amounting to about $150 billion in value. And the World Economic Forum estimates that “operations optimization” could unlock as much as $275 billion in value, with 90% of it coming from optimizing drilling and production and from leveraging the data from end-to-end connected assets to feed advanced analytics algorithms.
Subscribe to our Digital, Technology, and Data E-Alert.
Choosing data use cases.
The first step is selecting the data use cases that are most important to the business. High-value use cases cover a range of value pools and methods that address many of the challenges that O&G companies face today, such as the following:
- Applying AI based on existing data (such as in exploration, reservoir modeling, or drilling activities) to improve exploration success rates, maintain asset yields, or reduce cost
- Improving workforce effectiveness and reducing risks with, for example, remote and unmanned operations and monitoring or better resource planning and scheduling
- Reducing carbon footprints (such as by optimizing refining or transportation)
- Leveraging industry networks by, for example, collaborating online for engineering, procurement, or construction
- Streamlining labor-intensive processes, whether in administration (such as by using e-procurement) or smart automation of order processing
What these use cases all have in common is that they depend on data from a variety of processes—many of them AI enabled. Mastering that data gives O&G companies a “right to win” in today’s marketplace. Recently, we worked on cases that illustrate this well:
- A Middle Eastern company combining geological and engineering data into an optimized, automated drilling sequence that avoids collisions with existing wellbores
- A major European O&G company using an AI engine to optimize the production schedule based on a broad variety of data, maximizing returns from the company’s production assets
- A major integrated oil company leveraging smart maintenance with a combination of equipment, operations, and maintenance data, drastically reducing maintenance budgets and downtime
Successful implementation of data-driven use cases requires reimagining the end-to-end value chain with data and digital technologies. Creating a joint business and IT view of the value chain shows where key business capabilities reside and helps clarify which use cases are enabled by those capabilities. Developing strong, reusable, and shared capabilities helps launch successful use cases.
Unlocking Key Data Assets
Based on this initial assessment, companies must identify the specific data assets needed to fuel their use cases. (See Exhibit 1.) Success ultimately depends on unlocking existing data assets along the O&G value chain and then applying analytics and AI within a consistent, robust, and scalable architecture.
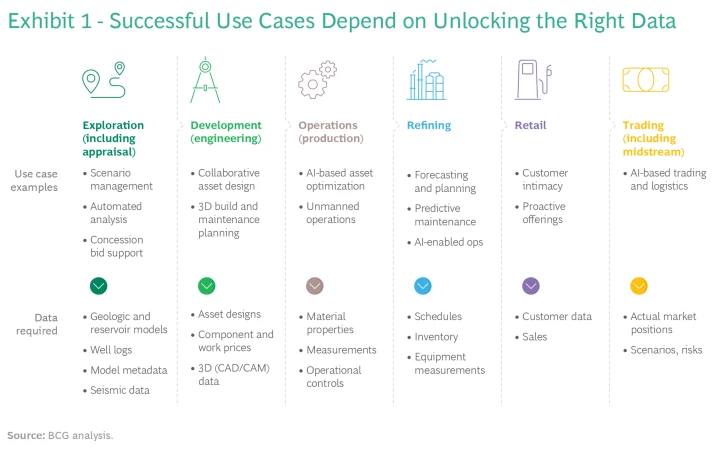
Then—and this is critical—companies need to plan the rollout of use cases so that, to the extent possible, the first wave leverages and improves data assets in a way that will benefit subsequent use cases, accelerating their deployment and business impact over time.
To unlock their data assets, companies must manage eight data challenges that are largely specific to the industry:
- Variety of Data . O&G data ranges from the very technical (such as drilling, log, seismic, lab, and sensor data) to complex models (reservoir models and wellbore shapes, for example) to 3D engineering designs to logistics (regarding people and equipment as well as hydrocarbons). All of this on top of the business’s “normal” transactional or master data.
- Proprietary or Inaccessible Formats. Data comes in proprietary sensor- or system-defined formats (such as 3D geological and reservoir models) that require conversion and might not be accessible through direct means. Similarly, engineering design data tends to be quite specific to the software that generated it.
- Dataset Sizes and Volumes . Datasets tend to be huge—up to petabytes for a single seismic survey. This stretches the capabilities of even cloud service providers.
- Scarcity and Interpretation. Technical data is often limited, incomplete, and even inconsistent, making it subject to interpretation. There is not always a “single source of truth.”
- Stakeholder Requirements . Governments, regulators, subcontractors, and joint venture partners all provide and use data. Often they have specific contractual commitments (such as export constraints and confidentiality guidelines) regarding what they can share and how. These requirements must be properly managed.
- Ambiguous Definitions. Internal and external organizations apply different data definitions and standards, impeding reuse and sharing of datasets. Uniform definitions are the goal, but reliable metadata to facilitate interpretation is the second-best option.
- Organizational Complexity . Typical O&G companies have decentralized structures with local joint ventures. The latter often have IT priorities that compete with central IT directives. If not resolved, these competing priorities could compromise data ownership and the ability to use data.
- Location and Geography . Data is often generated and used in locations with little bandwidth, preventing O&G companies from using cloud technology effectively and requiring smart replication methods.
Governing the Data and Updating the Architecture
These challenges require a mature approach to data governance covering the life cycle of different data types and addressing the constraints and complexities intrinsic to each one. Governance should be based on a system-agnostic view built on a single, company-wide categorization of all data into “data domains.” The level of detail may be different for different companies, reflecting variations in organizational levels and functional ownership. Data domains are the basis for any initiative regarding data definition, data quality, data access, or data-specific business rules, including concerns such as data privacy and confidentiality. Exhibit 2 shows an illustrative subset of a data domain map.
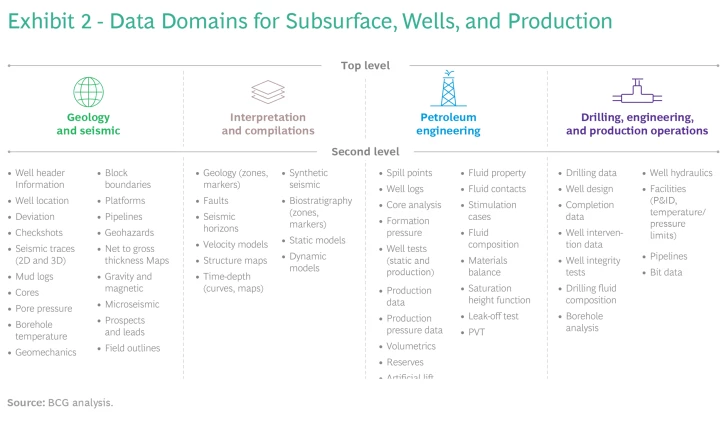
Next, O&G companies must move from a monolithic to a modular technology stack in order to free up their data and take advantage of new technologies. A data and digital platform lowers costs and improves speed and agility by decoupling front-end capabilities from data, liberating data from core transactional systems, and enabling smart-business engines with machine-learning and AI capabilities.
With this goal of a decoupled architecture in mind, O&G companies need to build a reference architecture to access and use the data assets. As with the data use cases, companies must prioritize and sequence their architecture modernization initiatives so that the first wave of improvements provides a foundation for the upgrades necessary to enable future use cases. Ultimately, O&G leaders must build a data platform that can scale horizontally to leverage the data sources in a consistent way across a variety of cases and potentially complex local IT landscapes. Horizontal scaling combined with a multicloud approach is necessary in order to cope with the huge amount of data and to allow geographically dispersed teams to collaborate effectively.
Successful Implementation
The final challenge is to get the execution right. Digital is, to a large degree, a change management effort. As a rule of thumb, typically 10% of success depends on architecture, 20% on data, and 70% on change management. There must be an integrated, multidisciplinary effort across digital app development, data governance, and architecture and operating model changes. To realize value at scale, digital needs to enable fundamental changes in how people work and how decisions are made in new end-to-end workflows. Starting with a few powerful lighthouse use cases—with well-defined value potential and a high degree of management support—is a tested way to move forward and ensure organizational learning and buildup of momentum. This, in the end, is what ensures that value is actually delivered.
O&G companies are currently reaping large profits from their operations, but it’s important that they innovate to stay relevant and competitive in a rapidly changing economic environment. They will need to become much more data driven, leveraging data and AI to drive high-value use cases. But successfully leveraging data is a complex effort that cannot be done by IT alone. It is a major change to how companies work. Setting up the basic data governance, defining a new data and digital architecture, and driving the first use case pilots require significant time, effort, and commitment.

Partner and Associate Director

Managing Director & Partner

Managing Director & Senior Partner

Partner and Associate Director, Data and Digital Platform
ABOUT BOSTON CONSULTING GROUP
Boston Consulting Group partners with leaders in business and society to tackle their most important challenges and capture their greatest opportunities. BCG was the pioneer in business strategy when it was founded in 1963. Today, we work closely with clients to embrace a transformational approach aimed at benefiting all stakeholders—empowering organizations to grow, build sustainable competitive advantage, and drive positive societal impact.
Our diverse, global teams bring deep industry and functional expertise and a range of perspectives that question the status quo and spark change. BCG delivers solutions through leading-edge management consulting, technology and design, and corporate and digital ventures. We work in a uniquely collaborative model across the firm and throughout all levels of the client organization, fueled by the goal of helping our clients thrive and enabling them to make the world a better place.
© Boston Consulting Group 2024. All rights reserved.
For information or permission to reprint, please contact BCG at [email protected] . To find the latest BCG content and register to receive e-alerts on this topic or others, please visit bcg.com . Follow Boston Consulting Group on Facebook and X (formerly Twitter) .
What Is Next
Read more insights from BCG’s teams of experts.
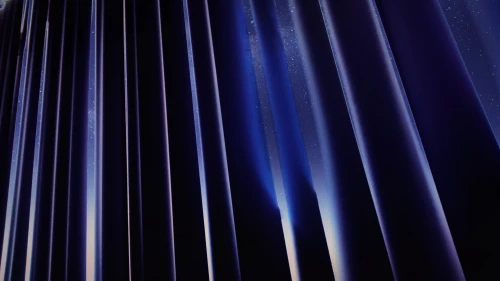
Use next-generation IT architectures to build digital-ready tech functions.
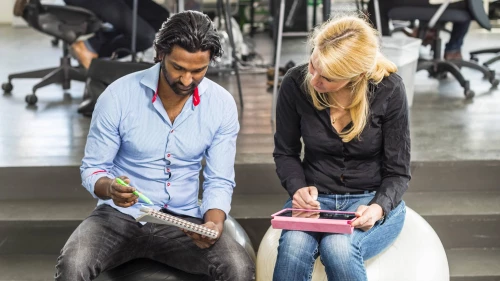
To swiftly achieve the goals of a transformation, the business and IT sides must jointly match the business strategy to the company’s data assets and architecture.
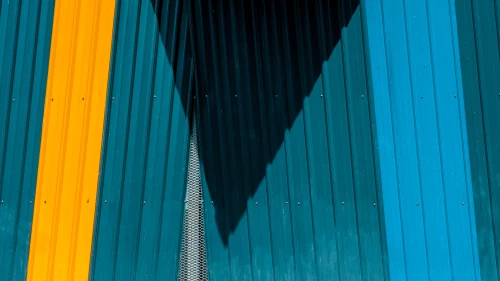
To accelerate a digital and IT transformation and build value faster, focus on business outcomes and decouple the digital transformation from core system replacement.
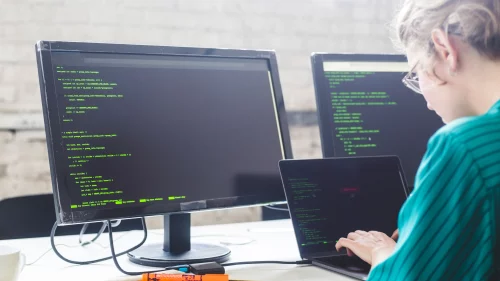
Data is increasingly valuable, yet some organizations lack the capabilities to capitalize on it. A key first step is setting realistic ambitions to improve data maturity.
Petroleum Data Analytics - Engineering Application of Artificial Intelligence & Machine Learning
Disciplines: Completions | Production and Operations | Reservoir
Course Description
Artificial Intelligence and Machine Learning is revolutionizing many industries. This technology is becoming an important point of competitive differentiation in the upstream oil and gas industry. Since optimization of production and enhanced recovery is the important issue for the petroleum industry, companies are realizing that reality and actual field measurements play much more important role in success of decision making than traditional assumptions, interpretations, and preconceived notions.
“Data” representing the actual field measurements, can provide much needed insight. Petroleum Data Analytics provides a set of tools and techniques for both conventional and unconventional resources to extract patterns and trends from data and construct predictive models to assist decision making and optimization. The most important part of Engineering Application of Artificial Intelligence and Machine Learning in the Petroleum Industry is the realistic and scientific understanding of this technology, its differences with traditional statistics and its non-engineering applications. This short course covers all such details to generate the interest in becoming Petroleum Data Analytics Engineers.
- Artificial Neural Networks (Deep Learning)
- Fuzzy Set Theory
- Evolutionary Computation
- Engineering & Non-Engineering Problem Solving using AI&ML
- Traditional Statistics versus AI&ML
- Data-Driven Reservoir Modeling - Top-Down Modeling (TDM)
- Actual Case Studies
- Multiple Case Studies
Learning Level
Intermediate to Advanced
Course Length
Petroleum Data Analytics is fairly new. A handful of domain experts have dedicated extensive amounts of time and effort to develop and present the next generation of tools that incorporate these technologies in the petroleum industry. Unfortunately, hypes, buzz words, and marketing ploys around data analytics have overwhelmed the petroleum industry in the past few years, specifically in the United States and most recently throughout the world. Many with little to no understanding and knowledge of the physics and the geology and other with very superficial understanding of AI and Machine Learning have been marketing these hypes.
This course will demonstrate the power of Artificial Intelligence and Machine Learning and the difference this technology can make for informed decision making when it comes to accomplishing important short-term, mid-term and long-term objectives. This course will also show how to distinguish between realistic application of AI and Machine Learning versus marketing ploys.
Who Attends
This course is designed for geo-scientists, engineers, and managers. Specifically, those involved with geology, drilling, reservoir, completion, and production in operating and service companies. In general, those involved in planning, completion, and operation in assets are the main target audience.
0.8 CEUs (Continuing Education Units) are awarded for this 1-day course.
Cancellation Policy
All cancellations must be received no later than 14 days prior to the course start date. Cancellations made after the 14-day window will not be refunded. Refunds will not be given due to no show situations.
Training sessions attached to SPE conferences and workshops follow the cancellation policies stated on the event information page. Please check that page for specific cancellation information.
SPE reserves the right to cancel or re-schedule courses at will. Notification of changes will be made as quickly as possible; please keep this in mind when arranging travel, as SPE is not responsible for any fees charged for cancelling or changing travel arrangements.
We reserve the right to substitute course instructors as necessary.
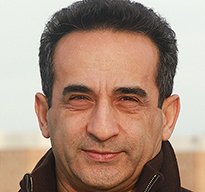
Dr. Shahab D. Mohaghegh , a pioneer in the application of Artificial Intelligence and Machine Learning in the Exploration and Production industry, is Professor of Petroleum and Natural Gas Engineering at West Virginia University and the president and CEO of Intelligent Solutions, Inc. (ISI). He is the director of WVU-LEADS (Laboratory for Engineering Application of Data Science).
Including more than 30 years of research and development in the petroleum engineering application of Artificial Intelligence and Machine Learning, he has authored three books (Shale Analytics – Data Driven Reservoir Modeling – Application of Data-Driven Analytics for the Geological Storage of CO2), more than 200 technical papers and carried out more than 60 projects for independents, NOCs and IOCs. He is a SPE Distinguished Lecturer (2007 and 2020) and has been featured four times as the Distinguished Author in SPE’s Journal of Petroleum Technology (JPT 2000 and 2005). He is the founder of SPE’s Technical Section dedicated to AI and machine learning (Petroleum Data-Driven Analytics, 2011).
He has been honored by the U.S. Secretary of Energy for his AI-based technical contribution in the aftermath of the Deepwater Horizon (Macondo) incident in the Gulf of Mexico (2011) and was a member of U.S. Secretary of Energy’s Technical Advisory Committee on Unconventional Resources in two administrations (2008-2014). He represented the United States in the International Standard Organization (ISO) on Carbon Capture and Storage technical committee (2014-2016).
Other courses by this instructor
Numerical Reservoir Simulation is a “Bottom-Up” Reservoir Modeling, while AI-based Reservoir Simulation is a “Top-Down” Reservoir Modeling. AI-based Reservoir Simulation is NOT a “Hybrid Model” through incorporation of the realistic, engineering applic...
Disciplines: Data Science and Engineering Analytics | Reservoir
Engineering application of Artificial Intelligence & Machine Learning will significantly address Climate Change in the next several decades. The main reason of positive and important contribution of Artificial Intelligence to Climate Change has muc...
Disciplines: Data Science and Engineering Analytics | Production and Operations | Reservoir
Data-Driven Reservoir Modeling (Reservoir Analytics) is defined as the application of Artificial Intelligence and Machine Learning in fluid flow through porous media. Data-Driven Reservoir Modeling (Reservoir Analytics) is the manifestation of the digi...
Disciplines: Completions | Data Science and Engineering Analytics | Drilling | Production and Operations | Reservoir
It is very important to learn what are the main characteristics and requirements in applying Artificial Intelligence and Machine Learning to solve Engineering related problems. To learn and apply Science and Engineering, Homo Sapiens must take a seriou...
Disciplines: Data Science and Engineering Analytics
Combining petroleum engineering domain expertise with computer programming using "Python" as the most popular coding language for data science, artificial intelligence and machine learning, this course enables petroleum engineering professionals to bui...
Disciplines: Completions | Data Science and Engineering Analytics | Drilling | Health, Safety, Environment, and Sustainability | Production and Operations | Projects, Facilities, and Construction | Reservoir
Data-driven analytics is becoming an important point of competitive differentiation in the upstream oil and gas industry. When it comes to production from shale, companies are realizing that they possess a vast source of important facts and information...
Smart Proxy Modeling is the application of Artificial Intelligence and Machine Learning in Numerical Simulation. Smart Proxy Modeling has already been successfully applied to Numerical Reservoir Simulation and Computational Fluid Dynamic. Details of Sm...
Petroleum Data Analytics is the application of Artificial Intelligence and Machine Learning in the oil and gas industry. Future of our industry will be highly influenced by Petroleum Data Analytics. Engineering-domain experts who become highly skilled ...
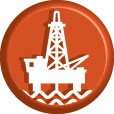
Real-time data streaming supports drilling automation, moving decision roles to centralized facilities for increased safety, lower costs and more eyes on the data.
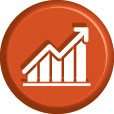
Preserve data integrity during collection to ensure that business-critical data analysis and processing always uses trustworthy data.
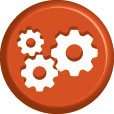
The upstream industry is rapidly transitioning to digital; industry-sourced data standards play a critical role in the success of this endeavor.
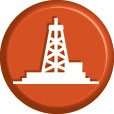
WITSML™ drilling and wellsite data standard, includes all the downhole data, MWD / LWD, mud logging, perforation, fracing and other stimulation data for a comprehensive oversight of drilling operations in real time.
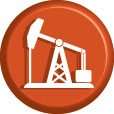
PRODML™ production, fluid analysis and field monitoring data standard, covers all the data needed to report production volumes, describe production facilities, PVT, WFT, DAS and DTS data.
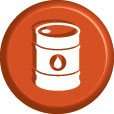
RESQML™ reservoir and structural interpretation data standard, allows for the comprehensive transfer between applications of a reservoir model, geocellular model, properties and structural information. It can transfer the whole data, or incremental modifications.
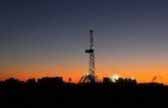
Modern E&P requires operators to work with a wide variety of partners and providers; our data exchange standards support collaboration, multi-vendor remote operations and automation. The open environment accelerates the integration of innovative technologies.
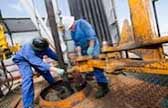
Service providers are being asked to work together on customer-focused solutions. Standards facilitate the reliable flow of data across many systems, minimizing headaches and accelerating deployment.
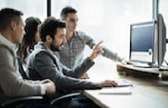
Developing innovative digital products and software systems is made easier and more cost-effective using standard data interfaces to connect to integration platforms or other data ports.
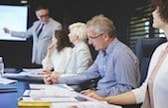
Data standards greatly simplify the collection and archiving of the technical data that is mandated by government bodies. The data is needed to support the exploration, development and production of a country’s hydrocarbon assets.
© Copyright 2022 | Energistics Consortium | All Rights Reserved
- Case Studies
- Product News
- Compliance Corner
Data Modeling in the Oil & Gas Industry
The Oil and Gas Industry faces a number of new challenges in the coming years. From the obvious challenge of managing a finite resource to ever-changing environmental regulations and the consequent competitive pressures, it’s easy to understand why industry leaders are continually striving to find ways to optimize and improve operational efficiency.
The new digital landscape of the industry has led to a proliferation of data, with greater frequency, at every stage of the business lifecycle. This abundance of data has led to new ideas about how this data can best be managed to maximize its value. The standards introduced by PPDM 3.8 include 53 subject areas intended to give executives a model for a Master Data Management solution.
A data management model is necessary largely because of the separate data sets that must be monitored – such as location data, well data and production data – which have traditionally been grouped and viewed separately. Now, research is showing that the proliferation of these information “silos” and the lack of a unified view can lead to a number of undesirable consequences, including:
- Project delays
- Escalating costs
- Lack of regulatory compliance
- Inability to utilize full production capacity (missed revenue opportunities)
If an integrated data management system can increase efficiency and productivity, improve safety, ensure regulatory compliance, and reduce costs, it becomes obvious that data integration is well worth the investment.
Data Modeling Tools
The OPC Foundation first introduced the Unified Architecture specification in 2006, taking a major step toward a cross-platform service-oriented architecture for process control. The OPC UA information model provides a logical framework for an integrated data management system. Additionally, other software tools have been engineered to facilitate the transition toward a more integrated model.
Recently, SCADA (Supervisory Control and Data Acquisition) and HMI (Human Machine Interface) software has made progress toward allowing a fully integrated data visualization system that aligns with the needs and expectations of the modern oil and gas industry. Some of the ways that SCADA has embraced the notion of integration include:
- OPC UA Compatibility Many HMI/SCADA developers have embraced OPC Unified Architecture, giving their software the ability to communicate with hundreds of different devices. The enhanced security and multi-platform support of the latest OPC specification helps facilitate an integrated information management system.
- Data Modeling Although data modeling in an HMI/SCADA system is still quite rare, some innovative developers have developed software with integration in mind. Finding a powerful HMI/SCADA system that incorporates the concept of data modeling is an essential step toward creating a fully integrated data management system.
- Mobile Device Support Not quite as rare as data modeling, but still not fully embraced, several software developers or ISVs have added mobile device support to their product offerings. As the oil and gas industries begin transitioning toward a unified system of data visualization, the benefits of mobility become more apparent.
Using the right SCADA system can significantly reduce unplanned downtime or unexpected delays, while simultaneously improving efficiency and safety.
B-Scada’s Status Enterprise HMI/SCADA software provides a perfect example of how a modern SCADA system can truly embrace the modern, data-driven industrial landscape. Learn more at http://scada.com .
- Reference Manager
- Simple TEXT file
People also looked at
Original research article, data governance facilitate digital transformation of oil and gas industry.
- 1 School of Economics and Management, China University of Petroleum, Qingdao, China
- 2 Research Institute of Petroleum Exploration and Development, Beijing, China
Data gradually becomes a critical concern for the strategic resources in enterprises. Massive amounts of data with different types involved in the oil and gas industry can be obtained through IoT (Internet of Things) devices, supported by Blockchain technology with characteristics of immutability and credibility in distributed storage. Hence, credible data will be rationally used in Big Data analysis and Artificial Intelligence implementation. The global mutual trust protocol of Blockchain technology is the bridge among IoT devices, Big Data analysis and Artificial Intelligence implementation, and enables effective coordination of participation of multiple parties and ultimately break isolated island of information. Data governance is the prerequisite for data sharing. As a basic project, Data Sharing breaks data islands and business barriers, improving Data Governance capabilities in Digital Transformation and promoting high-quality development of the oil and gas industry) From the perspective of Digital Transformation, this paper reviews the current situation and problems of Data Governance by evaluating the Data Governance ability of oil and gas enterprises. Furthermore, a proposal is put forward to apply Blockchain technology to improve the Data Governance system and capabilities as the overall program of the oil and gas industry Data Governance system.
Recently the digital economy has embarked on a major era of transformation. The in-depth integration of industrial technology and information technology innovates production organization and operation methods, triggering industrial resolution and traditional industrial upgrading with new industries, new formats and new models continuing to emerge. As a traditional industry, the oil and gas industry faces a new situation of the energy revolution to be accelerated. Digital technologies, such as Cloud Computing, Internet of Things (IoT), 5G, Big Data, Artificial Intelligence and Blockchain, should be adopted effectively to drive business model restructuring, management model reform, business model innovation and core competence enhancement to achieve industrial transformation and upgrading with value growth. At the same time, only when oil and gas companies systematically and thoroughly redefine their business, including all aspects of the organization, process, business model and employee capabilities, can Digital Transformation succeed.
In 2013, Tallon considered data to be a special asset ( Tallon, 2013 ). With the advent of the Big Data era, “data as an asset” has become the core trend of the industry. The definition of data assets in the “Data Asset Management Practice White Paper 4.0”: What is owned or controlled by an enterprise includes not only the internal data of the enterprise, but also the external data obtained by the enterprise through purchase, which can bring future economic benefits to the enterprise, or data resources recorded electronically. The core of Digital Transformation is data sharing, and data sharing requires Data Governance as the foundation. Therefore, Data Governance has become an important basic project related to Digital Transformation and high-quality development.
Urgent Requirements for Data Governance in Digital Transformation of Oil and Gas Industry
The core of digital transformation is to achieve full data sharing.
With the rapid development of Informatization, Big Data, and Intelligence, people usually compare cash flow to the blood of an enterprise. Data is an information record that identifies various resources and activities of an enterprise, with each datum as a neuron of an enterprise. Data has become a significant strategic resource that enterprises and society pay attention to as an important carrier of production, operation and management activities with highlights from all people. If data cannot be fully shared and used efficiently, other sharing will be impossible, and Digital Transformation will not be realized. For the oil and gas industry, data sharing has huge space, great potential and great value, including the business data such as internal enterprise planning, procurement, production, sales, and finance, and the oil and gas value chain data such as production, refining, transportation, sales, storage and marketing, as well as other data from all aspects of data on changes in external market supply and demand, price adjustments, and policy environment. Through full sharing of these data, the value of data assets is comprehensively improved, and business operations and decision-making methods are transformed from traditional manual statistical calculation and empirical analysis to accurate analysis based on systems, data and models, which effectively supports the optimal allocation of resources, efficient market operation forecasts, and Scientific connection of production, refining, transportation, sales, storage, and trade links to maximize the company’s overall benefits ( Liu, 2019 ; Dai, 2020 ).
Data sharing embodies three aspects of value in the oil industry. Firstly, it contributes to oil exploration and development management, realizing the most scientific decision-making and efficient productivity. Then, data sharing could analyze the trend and potential demand of oil resource consumers. Thus it encourages the industry to innovate pragmatically and develop potential markets steadily. For instance, data analysis and detection systems are set up in gas and petrol stations to record every customer demand in the country. It could formulate timely service and new product suggestions to retain high-quality customers and expand the market. Finally, data sharing could monitor the production and safety of oil fields through network detection. After all, intelligent detection is more efficient and safer than manual detection.
The Premise and Foundation of Data Sharing is Scientific and Effective Data Governance
As information technology continues to deepen application integration and innovation, various applications have gradually penetrated all walks of life. The authority, authenticity, validity, and compliance of data with business requirements are critical to cross-business data analysis. Data management with the unified criterium and quality will be the institutional guarantees for sharing applications among various businesses. Bhansali also elaborated on the value of Data Governance in the book, believing that the norms of Data Governance can help organizations manage data more effectively, reduce the cost of information use, improve the effectiveness of law compliance and control, and promote the generation of high-quality data ( Bhansali, 2013 ). Only through Data Governance can the high quality of data be improved. The main purpose of Data Governance is to improve the effectiveness of data sharing applications and work around how data is transformed into assets. Trope, etc. all believe that Data Governance should be integrated with various business departments ( Trope and Power, 2005 ), not just the IT department. At present, the business information systems of oil and gas companies have multiple data collections, repeated data entry, inconsistent data standards, and low data quality, which severely restrict the level of data sharing and application of oil and gas companies and hinder the company’s Digital Transformation ( Dai, 2020 ).
Technology platforms have inconsistent specifications, no reuse of components, insufficient adaptability, and are difficult to integrate and share.
Data is scattered and need to find everywhere, inconsistent standards, duplicate entries and inconsistent data.
System applications are management-oriented, independent applications and few all-in-one applications.
The following phenomena exist in system construction: slow response to business needs、long construction cycle、some functions overlap、high system maintenance costs. As shown in Figure 1 , the realization path of consistency, integrity, authenticity and validity of business data is the implementation of Data Governance.
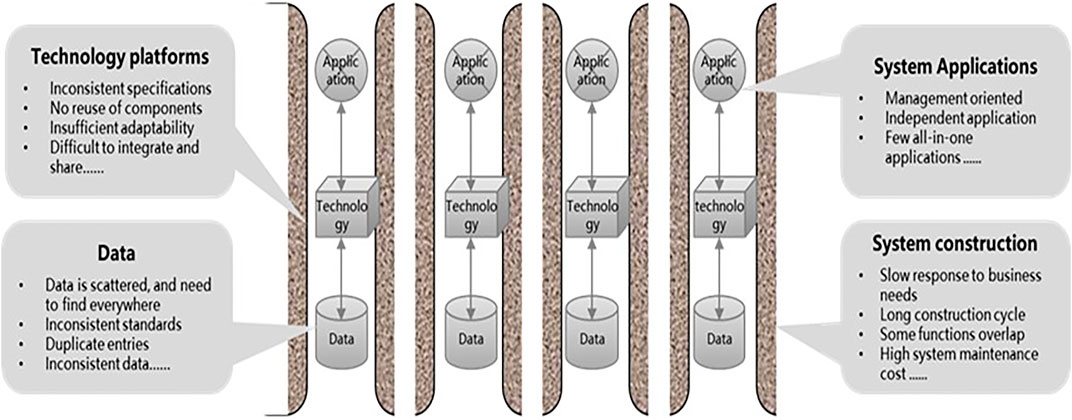
FIGURE 1 . Current system status of data sharing.
Data governance helps to identify the current situation and problems of data management. Unify the enterprise business system’s data governance system, data structure, and data standards to form a company-level data resource directory. Guided by the concept of data co-construction, sharing and co-governance, Data Governance advocates data management of standard, quality, security and sharing. It guarantees the operation of organization, system and process. It is also crucial to organize relevant parties to carry out Data Governance activities together, promote all parties to build the company’s Data Governance system jointly, and improve Data Governance capabilities. Data governance activities should be divided into front-line information systems and data warehouses, embedded in the whole life cycle of the system, jointly manage and highlight data problems, improve the quality of data resources from the source. In this way, all parties involved could share high-quality Data Governance achievements and create a new enterprise Data Governance model of “co-construction, co-management and sharing”.
The Connotation of Data Governance in the Oil and Gas Industry and the Key Issues to be Addressed
The connotation of data governance in the oil and gas industry.
Informatic defines Data Governance as “the functional alignment and definition of processes, policies, standards, technologies and people across the organization to manage data as a corporate asset, enabling the availability of accurate, consistent, secure and timely data and controlled growth to make better business decisions, reduce risk and improve business processes”.
The essence of Data Governance in the oil and gas industry is to treat data as an asset of the company, and to develop a set of systems for the “collection, storage, management and application” of data across the organization, including both decision-making and executive levels, and both business and IT departments. Data governance is a long-term process that integrates business and IT throughout the business process.
All data sources are generated by its business front-end transaction process, and those who generate data and input data. In other words, all data depends on the business, and it is meaningless to discuss data governance and business separately. Therefore, the analysis of data sources and their business distribution is the foundation of Data Governance.
Key Issues in Data Governance at Oil and Gas Industry
The dynamic and static data of oil and gas field enterprises are massive. Taking the unified upstream system as an example, the data management mode segmented from A1 to An has accumulated massive data resources, including exploration and production technology data management system, oil and gas water well production data management system, oil recovery and surface engineering operation management system, engineering technology production operation management system, exploration and production scheduling command system, oil and gas production IoT system, engineering technology IoT system, etc. It has built nine major types of data asset libraries, covering nearly 450,000 exploration and evaluation development wells, managing more than 1.08 million logging data body files, 600 oil and gas reservoirs, more than 7,000 work areas of seismic data, more than 3.67 million documents, etc., and storing more than 1.7 petabytes of data in total. As shown in Figure 2 , with such a huge amount of data and the continuous growth of data diversity, “data island” are growing, and data quality varies, greatly hindering the digital transformation process, and Data Governance is urgently needed to address the following issues.
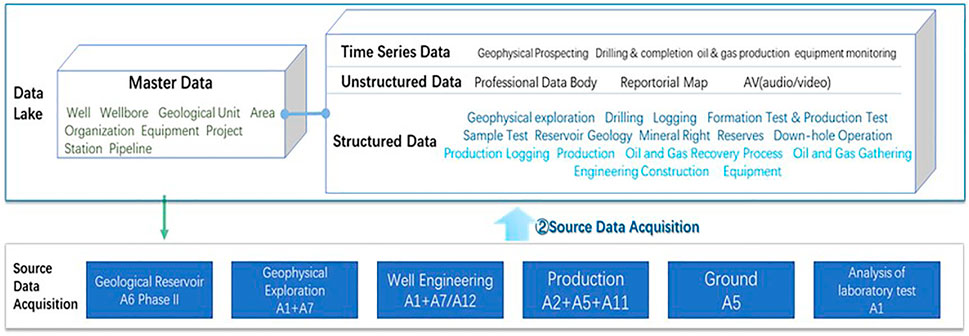
FIGURE 2 . Source collection of exploration and development data.
The First question lies in the management mode of compartmentalization that caused a large number of “data island”. At present, oil and gas field enterprises contain several compartmentalized departments or systems, each of which has its own data, and the data of each department or system is often stored separately, forming its own system. The raw data of the same study may exist in multiple systems, multiple databases, and even in the computers of various researchers. It cannot ensure the authority of the raw data in each study, affecting the accuracy of the research results and failing to guide E&P research and production effectively. The core problem of such data is that there are many databases, platforms and isolated applications, leading to the serious problem of “data island”. Firstly the data standards are not uniform, and the data patterns do not match. There is no unified, timely updated and shared oil and gas well geological database and engineering foundation database, which is easy to cause data conflicts and data redundancy, and there is no consulting service company with compatibility and neutrality to classify and secondary development, which is later applied to data sharing, mining and analysis. Secondly, the specifications of the technical platform are inconsistent. The components are not reusable, have insufficient adaptability, and are difficult to integrate and share. Thirdly the number of system applications is large, and there are many management-oriented independent applications but few integrated applications. Fourthly, system construction is slow in response to business needs with a long construction cycle, duplication of some functions, and high system maintenance costs ( Li et al., 2019 ).
There are two main direct negative effects brought by data silos: First, investment waste. Geological data has multiple interpretations, and the results need to be verified according to the back-end works, with continuously revised and optimized geological model and parameters should be to gradually approach the real underground situation. If the back-end engineering construction results cannot be fed back to the geological department timely, the reservoir model will not be corrected in time. If some ideas that have been proved to be wrong by predecessors are tried again by others, low-level mistakes will be repeated continuously, which resulting in extreme waste.
The second is reduced efficiency. The multi-solution of geological knowledge also causes different disciplines and different teams to choose inconsistent parameter parameters when working on the same reservoir, which makes the research results lack uniformity and comparability. Different disciplines or teams often repeat simple and low-level data collection, data screening and understanding and digestion after taking over the same target work, which seriously affects work efficiency.
The second issue is data quality. It is necessary to verify the authenticity and integrity of the data, because of the inconsistency of data collection methods, statistical methods and control measures over the past 60 years. In particular, the current IoT in the old upstream oil fields is not fully covered, and the degree of automated data collection is not yet high, which affects the validity and accuracy of data. Moreover, data comes from various sources and involves multiple participating subjects in their life cycle. Issues such as whether data are authentically generated, data tampering, and inconsistent standards and types of data from multiple sources can affect data quality and, thus, the data decision outcomes of data users. Therefore, Data Governance needs to support the traceability of big data throughout its lifecycle.
Goals and Directions of Digital Transformation in the Oil and Gas Industry
As a traditional industry, the oil and gas industry faces an opportunity of accelerated energy revolution and energy transformation. It requires to make effective use of digital technologies (such as cloud computing, Internet of Things, 5G, big data, artificial intelligence) to promote business model reconstruction, management model reform, business model innovation and core competency enhancement, so as to achieve industrial transformation, upgrading and value growth. At the same time, digital transformation can only succeed if oil and gas companies systematically and thoroughly redefine their business, including all aspects of the organization, processes, business models and employee capabilities.
Overall Objectives
It is necessary to use automatic sensing to collect real-time operation data in the oil and gas industry chain, use comprehensive interconnection to widely obtain internal and external data, and use digital technology to optimize business execution and operational efficiency continuously. We will build a closed-loop system for the integration and interaction between the physical oil and gas enterprise and the digital twin, and promote the two-way connection between the physical business and the digital world, so as to form an internal and external connection, sharing and collaboration mechanisms, and finally realize cost reduction and efficiency increase, collaboration and sharing, continuous innovation, risk pre-control and intelligent decision-making, and continuously improve the productivity of all employees and the ability to create assets.
Main Directions
It is important to integrate digital technology into the products, services and processes of the oil and gas industry chain, thus promoting changes in the company’s development philosophy, working model, operation and management, scientific and technological research and development and management system mechanism to build new capabilities such as intelligent production, networked collaboration and personalized services and create new business models, new production methods and new industrial ecologies driven by users, data and innovation.
Internet of Things, Blockchain Technology in Data Governance
Data integrity, accuracy, consistency and timeliness guaranteed by iot technologies.
The oil and gas industry is characterized by high asset density, long operating distances and dangerous production environments. Establishing an industrial IoT can effectively streamline processes, enrich decision-making tools and improve operational efficiency. The IoT of the oil and gas industry usually consists of three layers: sensing, transmission, and application. The sensing layer consists of sensors and radio identification frequency devices (RIFD) installed in each key production link, which is used to collect data information in oil and gas exploration, development and production in real-time. The transmission layer is composed of networks such as the Internet and mobile communication networks, which are responsible for data transmission. And the application layer refers to the system management platform, which realizes the analysis and processing of oil and gas data. It establishes a comprehensive, interconnected information network among reservoirs, wellbores, individual wells, field stations, pipeline networks, equipment and personnel, realizes the comprehensive sensing capability of the oil and gas exploration, development and production management system, and provides a basic platform for intelligent production and decision management of oil and gas systems.
The IoT technology enables a unified automatic and standardized collection of data sources through data quality control tools. These tools guarantee data quality by building a high-quality data mode, allowing collected data to directly flow into the lake to realize the standardized docking of data lake and sensors. In this way, redundant data like repeated and multiple collections are avoidable. So that the data quality control tools could not only ensure the integrity, accuracy, consistency, and timeliness of data acquisition, enrichment, conversion, storage, and application.
IoT collects production data formed “four unification”. Unification of data entrance - is a uniform data acquisition interface that allows automatic production data collection and guarantees uniqueness and timeliness. Unification of the data model—provides developing and producing functions and facilitates data sharing. Unification of data quality control - equips by combining big data, artificial intelligence algorithm judgment and manual sampling to ensure accuracy, and improves efficiency while reducing the labour intensity and workload of data entry personnel. Unification of data services—provides reliable data services for unified construction systems and personalized oilfield systems through cloud platforms to ensure data consistency and integrity.
The IoT system construction of oil and gas fields has created significant economic and social benefits in optimizing the production process and organizational structure, improving production efficiency and management level, and reducing labour intensity (especially for front-line workers) and production costs and safety risk. It has generated a cumulative direct economic benefit of 1.16 billion yuan and cut 24660 in the number of front-line workers.
The Changning shale gas field, which is the first in China in terms of daily shale gas production, is a typical case of successful digital transformation empowered by Data Governance with IoT technology as a grip, As shown in Figure 3 .
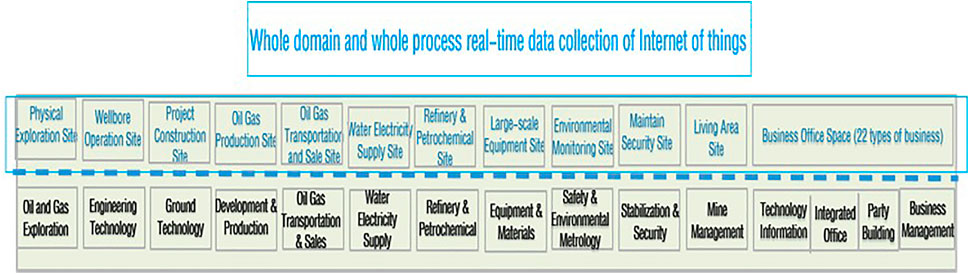
FIGURE 3 . Whole domain and whole process real-time data collection of Internet of things.
IoT technology supports a lot in oil and gas production process management. It realizes real-time data collection, transmission and monitoring of oil and gas production sites, including wells, workshops, stations and processing plants. Besides, it promotes the transformation of production methods—optimizes production processes, management processes, and organization and improves production efficiency and management level. Furthermore, IoT technology energizes intelligent analysis of production devices, electrical facilities, energy consumption and self-control equipment during the production process. The production process realizes intelligent application of data in four fields, covering intelligent analysis of production devices, intelligent analysis of power facilities, intelligent analysis of energy consumption correlation, and intelligent analysis of self-control equipment, to realize lean and safe production with high timeliness and considerable economy. Timeliness is reflected in the automatic data collection at the minute level and early warning at the hourly level. The economy is reflected in the timely detection of abnormalities in plant conditions, accurate prediction of the probability of accidents and events, scientific evaluation of the health of key plants and reduction of plant daily management costs by more than 20%.
Elimination of “Data Island” by Blockchain Technology to Ensure True and Safe Data Sharing
Blockchain integrates cryptography and distributed database technologies, which can effectively and securely solve the problem of data sharing across organizations and eliminate the phenomenon of “data island”. With its decentralized, open, transparent, and tamper-evident characteristics, Blockchain is compatible with the transparency requirement of Big Data value realization and can overcome the authenticity problem of current Data Governance, providing a new solution for Data Governance.
Blockchain technology can be used during data circulation to strengthen data quality control and ensure data traceability and immutability. The distributed storage can be used to realize data sharing, break “data island”, and promote integrated data integration.
Data decision-making permeates all aspects of production and operation. Due to the involvement of multiple stakeholders, there are problems such as data tampering, data falsification, and differences in the types and standard rules of data from different sources in the process of storage, processing and sharing, which will affect the quality of decision-making data. Therefore, data users need to audit the decision data. Blockchain, as a decentralized distributed database, enables data storage and processing that supports auditing. In addition, by building a decentralized distributed database system among different interest subjects based on Blockchain, data is quickly broadcasted to each interest subject through the whole network, ensuring the authenticity and timeliness of data sharing and circulation.
Each node in the Blockchain network stores data, and once the data is stored in the Blockchain, it will not be tampered with or lost. Even if there are problems such as communication failures and deliberate attacks, the correctness of the data storage can still be guaranteed, and the data users can audit it. In addition, storing data in the Blockchain also supports the audibility of the data treatment process and results.
As a decentralized distributed database, Blockchain supports historical status query of data to confirm whether the current data status is correct. For a traditional database management system, the current data state is stored and maintained in the database, and only the information such as the data processing process is stored in the database log for failure recovery, and does not support the historical state query of data.
Blockchain technology allows data to be stored and shared more conveniently and safely, which is of great significance in the oil and gas field with high data integrity requirements. Blockchain technology has strong practicality for data protection and management. By building a Blockchain-based data storage and sharing platform, the data can be safely stored on the chain, but all data can be kept interrelated and connected with each other. As shown in Figure 4 , in the data storage sharing platform, relevant business organizations will join the data storage sharing platform as nodes in the Blockchain system to realize the utilization and sharing of data resources. At the same time, the distributed storage, consensus mechanism and smart contracts in Blockchain technology provide guarantees for data management, safe storage and sharing and data users can audit decision data and perform analysis and make decisions on trusted data ( He et al., 2017 ; Zhao et al., 2018 ; Fu, 2019 ; Gong et al., 2019 ).
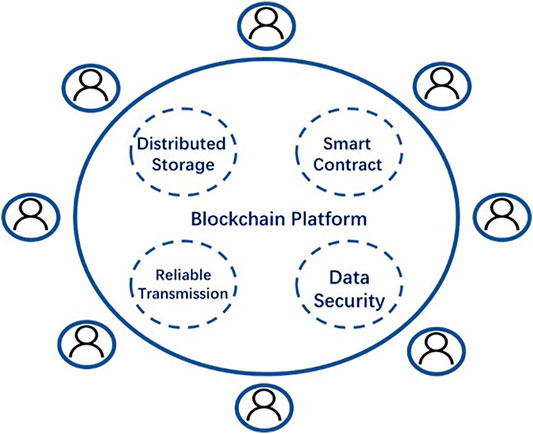
FIGURE 4 . Blockchain-based data storage sharing platform.
For problems such as inconsistent types and standard rules for data from different sources, uniform data types and standard rules can be developed based on the Blockchain and smart contracts. Smart contracts will be stored and synchronized in various nodes of the Blockchain, and the Blockchain will automatically execute validation based on the code on the smart contracts. Since the execution process of smart contracts is open and transparent, the execution process and execution results are audible, which can improve the efficiency of multi-source data sharing and there is no single point of failure.
At present, in * oilfield, Blockchain technology supports various E&P business fields of shale gas to store the structured raw data. These data cover 12 categories: geophysical exploration, drilling, mud logging, well logging, oil testing, analysis and testing, reserve management, reservoir geology, oil recovery technology, borehole operation, oil-gas gathering and transportation and surface construction, as shown in Table 1 .
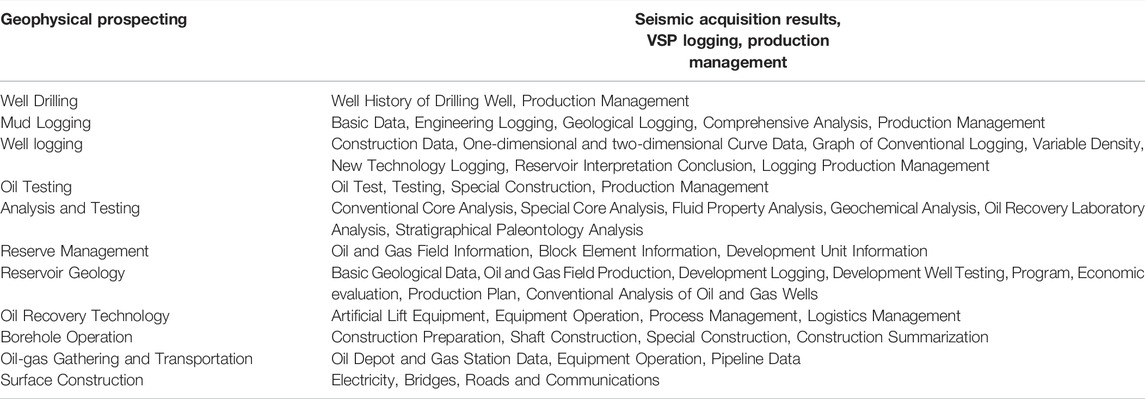
TABLE 1 . Blockchain data storage item of shale gas.
On account of the authenticity data before uploading the chain, a Blockchain-based data management platform is established to record the site data and operation information of reservoir exploration, oil and gas extraction and other aspects in real-time and accurately. In addition, this platform digitizes real-time and data sharing of site supervision, significantly improves data security, site supervision effectiveness, and production risk control. It also optimizes the construction process and supervision measures and takes full advantage of Blockchain. The advantages of Blockchain technology will drive the oil and gas industry towards digital business innovation and provide a guarantee for safe and efficient production and cost reduction and efficiency in the oil and gas sector.
The shale gas master Data Governance based on Blockchain is the core task to realize centralized management and sharing of shale gas data. Master data refers to relatively static, core and high-value data shared by multiple application systems across the enterprise, also known as enterprise benchmark data. Master data can create and maintain the consistency, completeness, relevance and accuracy of data of each business and system in the related fields.
Master data scope determination: the use of shale gas business data in each business system is sorted out, and then analyzed by the Data Governance expert group in conjunction with actual shale gas data use and shale gas characteristic business to form consistent, complete and accurate company-wide core business data of shale gas.
Master data standard specification: based on Blockchain and Smart Contracts, unified data types and standard rules can be established for master data naming specification of shale gas, model standard specification, coding specification, master data association relationship and master data accuracy and integrity business logic rules to develop a quality management in the whole life cycle process.
The Data Governance technical team can load the confirmed authoritative master data information from the temporary environment to the Blockchain through the ETL tool, and distribute the master data information of shale gas to all business systems. So far, the integrity, uniqueness, standardization, consistency and correlation of shale gas master data of all company business systems were realized; the data-sharing mechanism was realized and improved, thus helping the oilfield reduce cost and improve efficiency through data analysis and reuse.
The Overall Plan for a Data Governance System
At present, the oil and gas industry has many problems in the process of data generation, processing, and application due to many reasons. It is necessary to accelerate the construction of the Data Governance system from a group lever, including the clarification of the centralized Data Governance department, the data management of standardization, metadata and master data and regular evaluation of data maturity of governance capabilities. It is necessary to strengthen the dynamic data collection of the entire industry chain and establish a data collection, transmission and aggregation system covering the entire business chain. In order to improve Data Governance capabilities comprehensively, we should adopt systematic thinking and systematic solutions, and implement policies from the concept, organization, management, and technology dimensions. A comprehensive Data Governance system could provide high-quality data foundation guarantees for the Digital Transformation of the oil and gas industry.
Most foreign scholars have proposed a Data Governance framework system during their research. For example, the draft framework model proposed by Wende defined various decision-making fields and corresponding role divisions ( Wende, 2007 ). The most significant contribution of this framework model is to help organizations build data quality responsibilities. The decision domains and roles it proposed can be used as the structural configuration of Data Governance, but there is still a lack of use verification under different organizational scenarios. Among them, the most influential is the decision domain model of Data Governance proposed by Khatri and Brown ( Khatri and Brown, 2010 ). Their framework includes five decision domains: data criteria, quality, metadata, access, and life cycle, and expounds the type and scope of decision domains. This model proposed different centralized, decentralized and shared decision-making power levels in the same organizational decision-making domain. At the same time, it provides a common framework of standard terms, which makes it widely adopted in subsequent studies.
Combined with foreign design concepts and the characteristics of the oil and gas industry ( Fisher, 2008 ), the author summarizes the Data Governance system elements as follows. Under the data co-construction, sharing and co-governance guidelines, the Data Governance system concentrates on data standards, quality, security and sharing, and guarantees the operation of organizations, institutions and processes. Supported by the Data Governance platform, this system innovates the data integration analysis and sharing exchange mechanism. So that, Blockchain technology is able to promote the construction of Data Governance systems and governance capabilities in the oil and gas industry. *Oil field regional data lake as shown in Figure 5 .
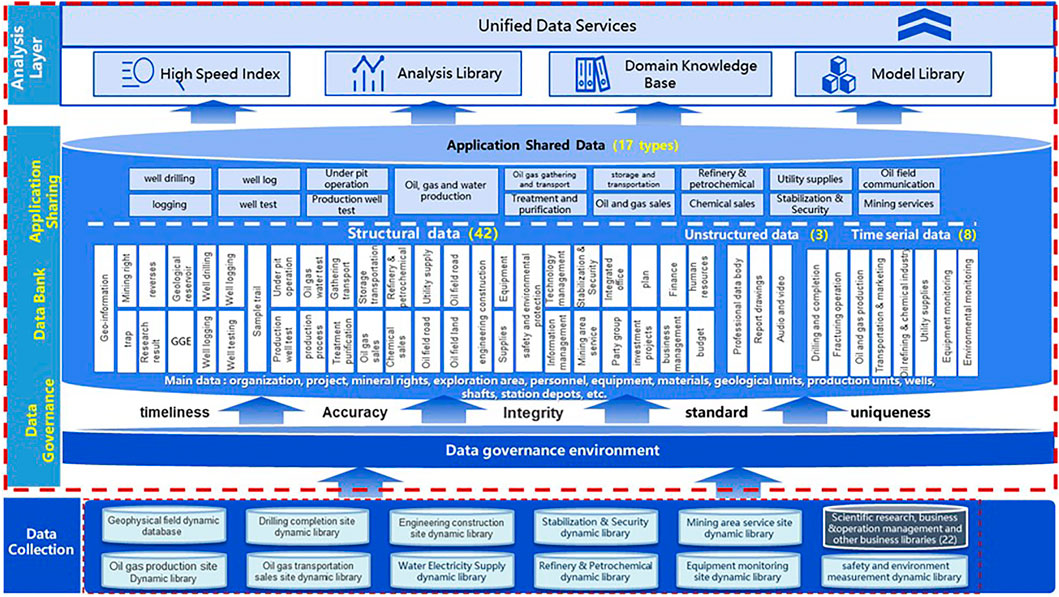
FIGURE 5 . *oil field Regional data lake.
Framework of Oil and Gas Data Governance System
Data management activities should be carried out with all relevant parties jointly in combination with “top-level design plus partial landing” with effective results to promote the company’s Data Governance system, as shown in Figure 6 , to improve data management capabilities. The Data Governance activities should fall into the front-line information system, data warehouse and be embedded in the full life cycle of the system to jointly manage outstanding data problems to improve the quality of data resources from the source. As a result, all parties involved can share high-quality Data Governance results, and create a new Data Governance pattern of “co-construction, co-governance, and sharing” for the company.
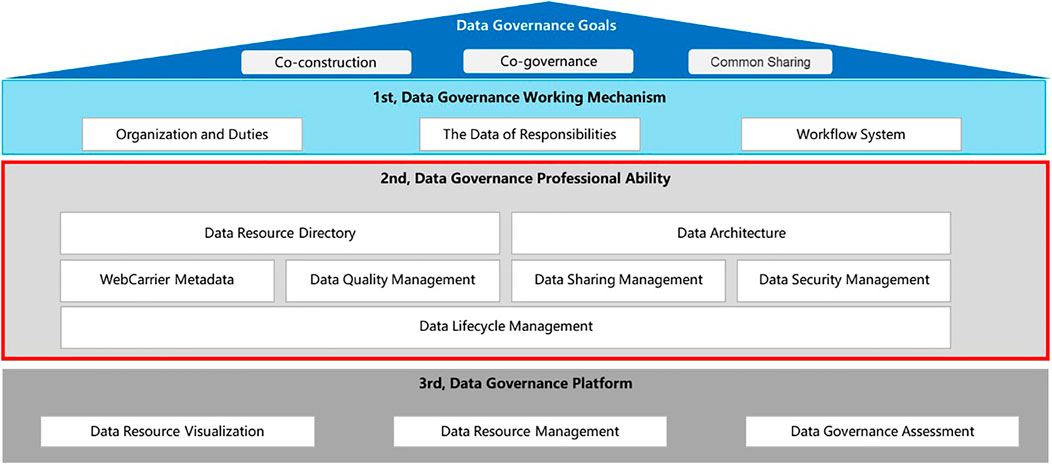
FIGURE 6 . Framework of oil and gas data governance system.
Data Governance Platform
Martijn et al. proposed a Data Governance platform that includes a three-tier system of technical architecture, process architecture, and business architecture ( Martijn et al., 2015 ); and Seine proposed a five-tier system that includes executive tier, strategic tier, tactical tier, operation tier and support tier ( Seiner, 2016 ). The system’s Data Governance platform also elaborated on the respective roles, processes, communication, indicators and tools at the five levels. With reference to the above design ideas, combined with the characteristics of the oil and gas industry’s entire industry chain, a Data Governance platform for the oil and gas industry has been built to follow the Data Governance system and implement relevant policies, procedures and methods as platform functions, realizing data consolidation, data maintenance and data application to improve the efficiency and effectiveness of all aspects of Data Governance. As shown in Figure 7 , the data management platform can be divided into the database, data management core, data service, and user access layers.
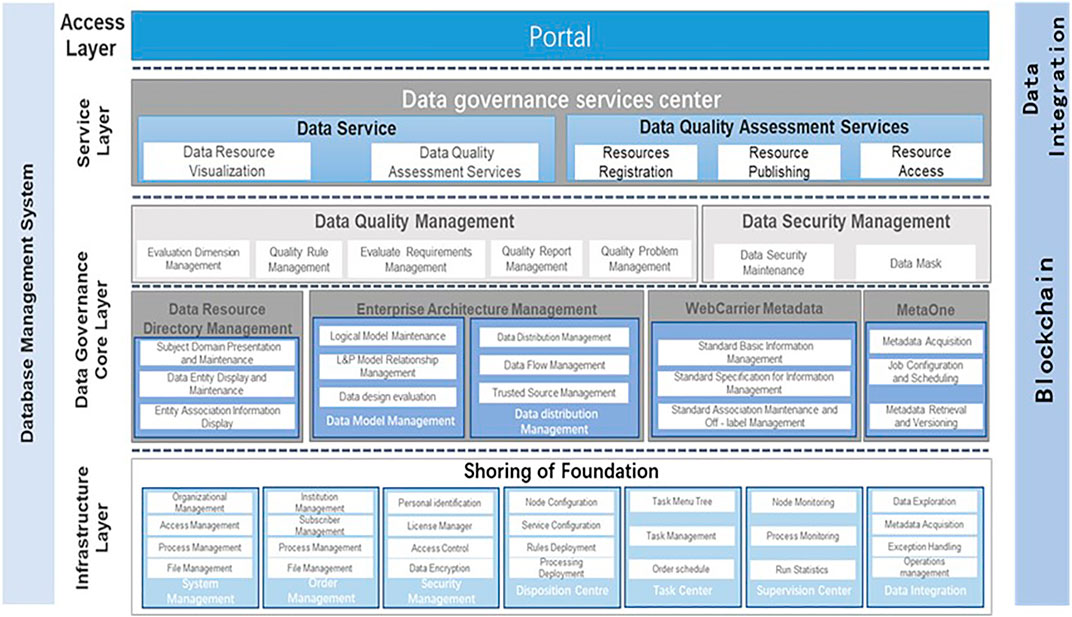
FIGURE 7 . Data governance platform.
Application Effectiveness of Data Governance Platform Pilot Construction
The pilot construction of *oilfield Data Governance platform covers the core activities and data management in shale gas exploration and production (E&P), reaching a plethora of achievements in multiple dimensions.
- Established an integrated data model covering *oilfield E&P core activities, to fundamentally realize the transparent sharing of cross-professional data;
- Established a unified data resource management system, to implement asset-based exploration and exploration data management;
- Established a unified data acquisition system based on Iot, to achieve unique source site, one-time acquisition and global shared;
- Established a unified E&P Data Blockchain storage system, to scientifically preserve valuable data assets which obtained at great expense;
- Established a unified E&P Data Governance platform, to realize the integrated application of E&P data;
- Automatic data quality check with high availability rate;
- Data application across departments and professions, integration and collaboration;
- Comprehensive research “one-click access” and significant improvement in work efficiency.
With oil and gas reservoir as the core, it realizes parameter interaction and optimization adjustment, professional mutual synergy and promotion, and builds connections for different professions and departments to communicate and break obstacles. Furthermore, it records instantly during the run process, gradually accumulates experience, continuously learns and iterates, forms efficient templates, and finally achieves maximum benefits.
*Oilfield Data Governance platform has stored the primary data of five business domains and 41 businesses of E&P, which fully supports all applications of E&P. Currently, the data content covers 608 exploratory wells and 575 development wells in 29 oil and gas fields, with a total of 1994 categories, more than 160 million entries, and about 1T data information (excluding seismic body data). Overall data normalization reaches more than 90%, providing use for seven professional databases and application systems, including dynamic exploration database, development production database, mud logging database, well logging database, geological reservoir library, target database and logging decision support system.
In the Digital Transformation development of the oil and gas industry, it is necessary to support the research and development of Blockchain technology vigorously, continuously upgrade and improve it through iterative construction, create a “sample room”, ensure the quality and efficiency of Data Governance and advocate the formation of a concept and culture of data co-construction, sharing and co-governance. The Digital Transformation of the industry can be carried out from the three aspects: technology development, scenario application, and standard formulation to promote the standardized and large-scale application of Blockchain technology in the oil and gas industry, and continue to implement all elements of the Data Governance system in place. Each element of the Data Governance system should be executed continuously to a full extent one by one with multi-party coordination, comprehensive policy implementation and long-term contributions to achieve collaborative innovation, efficient operation and value enhancement of the entire business chain of the oil and gas industry.
Data Availability Statement
The original contributions presented in the study are included in the article/Supplementary Material, further inquiries can be directed to the corresponding author.
Author Contributions
JS: performed the data analyses and wrote the manuscript; SY: contributed significantly to analysis and manuscript preparation; HL: helped perform the analysis with constructive discussions.
National Natural Science Foundation of China/Basic Science Center Project “The theory and application of resource and environment management in the digital economy era” (72088101).
Conflict of Interest
The authors declare that the research was conducted in the absence of any commercial or financial relationships that could be construed as a potential conflict of interest.
Publisher’s Note
All claims expressed in this article are solely those of the authors and do not necessarily represent those of their affiliated organizations, or those of the publisher, the editors and the reviewers. Any product that may be evaluated in this article, or claim that may be made by its manufacturer, is not guaranteed or endorsed by the publisher.
Autonomous, Author. (2012). All the Ingredients for success: Data Governance, Data Quality and Master Data Management. Available at: https://tdwi.org/whitepapers/2012/03/all–the–ingredients–for–success–data–governance–data–quality–and–master–data–management.aspx .
Google Scholar
Autonomous, Author. (2010). See Course Materials from EWSolutions, enterprise Data Governance and Stew Ardship[EB/OL]. Available at: http://www.EW-Solutions.com .
Bhansali, N. (2013). Data Governance: Creating Value from Information Assets . CRC Press , 28–122.
Dai, H. (2020). PetroChina: Drive the High-Quality Development of the Oil and Gas Industry with Digital Transformation . Website of State-owned Assets Supervision and Administration Commission of the State Council .
Fisher, T. (2008). The Four Stages of Data Maturity, Data Flux[EB/OL]. Available at: http://www.sas.com/news/sascom/2007q4/column_tech.html (Accesssed 10 05, 2008).
Fu, Rao. (2019). When the Oil and Gas Industry Meets the Block Chain . Ta Kung Pao , 11–13.
Gong, R., Yang, R., and Lan, M. (2019). Application Prospects of Block Chain Technology in the Petroleum Industry[J]. Inf. Syst. Eng. (11), 62–65.
He, P., Yu, G., Zhang, Y. F., et al. (2017). Survey on Blockchain Technology and its Application Prospect[J]. Comput.Sci 44 (4), 1–7.
IBM Data governance council (2008). IBM Data Governance council. Available at: http://www–01.ibm.com/software/tivoli/governance .
Khatri, V., and Brown, C. V. (2010). Designing Data Governance. Commun. ACM 53 (1), 148–152.
CrossRef Full Text | Google Scholar
Li, G., Wang, F., Xuejun, P., and Liu, H. (2019). Optimized Application of Geology-Engineering Integration Data of Unconventional Oil and Gas Reservoirs[4]. China Petroleum Exploration 24 (1), 147–152.
Liu, S. (2019). Research on Data Governance System in "Share China Petroleum. [J] No.6, 21–29.
Martijn, N., Hulstijn, J., Bruijne, M., and Tam, Y.-H. (2015). “Determining the Effects of Data Governance on the Performance and Compliance of Enterprises in the Logistics and Retail Sector,” in Conference on e-Business, e-Services and e-Society , 454–466.
Seiner, R. S. (2016). Noninvasive Framework for Data Governance Implementation: Details, Part 2[EB/OL]. Available at: http://tdan.com/non–invasive–framework–for–datagovernance–implementation–details–part–2/20130 (Accesssed 01 24, 2016).
Tallon, P. (2013). Corporate Governance of Big Data Perspectives on Value, Risk and Cost. Computer (46), 32–38.
Trope, R. L., and Power, M. (2005). Lessons in Data Governance: A Survey of Legal Developments in Data Management, Privacy and Security. Business Lawyer 61, 471–516.
Wende, K. (2007). “A Model for Data Governance–organizing Accountabilities for Data Quality Management,” in 18th Australasian Conference on Information Systems , 417–425.12
Zhao, G., Sun, J., Wang, M., et al. (2018). Block Chain Technology Boosts the Digital Transformation of Oil and Gas Exploration and Development[J]. Sinopec 397 (10), 72–74.
Keywords: data governance, digital transformation, oil and gas industry, exploration and development, data sharing, governance capabilities, block chain, shale gas
Citation: Su J, Yao S and Liu H (2022) Data Governance Facilitate Digital Transformation of Oil and Gas Industry. Front. Earth Sci. 10:861091. doi: 10.3389/feart.2022.861091
Received: 24 January 2022; Accepted: 31 March 2022; Published: 23 May 2022.
Reviewed by:
Copyright © 2022 Su, Yao and Liu. This is an open-access article distributed under the terms of the Creative Commons Attribution License (CC BY). The use, distribution or reproduction in other forums is permitted, provided the original author(s) and the copyright owner(s) are credited and that the original publication in this journal is cited, in accordance with accepted academic practice. No use, distribution or reproduction is permitted which does not comply with these terms.
*Correspondence: Shanglin Yao, [email protected]
This article is part of the Research Topic
New Advances in Geology and Engineering Technology of Unconventional Oil and Gas
Customer Logins
Obtain the data you need to make the most informed decisions by accessing our extensive portfolio of information, analytics, and expertise. Sign in to the product or service center of your choice.
- S&P Global
- S&P Dow Jones Indices
- S&P Global Market Intelligence
- S&P Global Mobility
- S&P Global Commodity Insights
- S&P Global Ratings
- S&P Global Sustainable1
- Investor Relations Overview
- Presentations
- Investor Fact Book
- News Releases
- Quarterly Earnings
- SEC Filings & Reports
- Executive Committee
- Merger Information
- Stock & Dividends
- Shareholder Services
- Español
- Português
- English (Australia)
- Get Support
- System Notifications
- Delivery Platforms
- Regulatory Engagement
- Commodity Insights Login
- Access IHS Markit Products
Data: Is the oil and gas industry’s most valuable resource being overlooked?
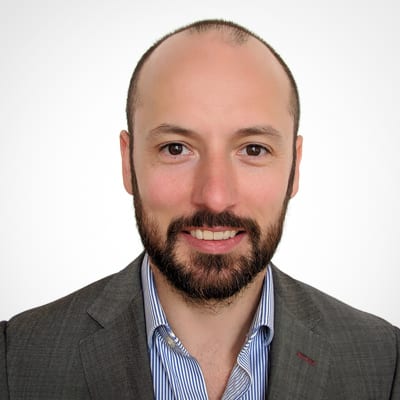
Executive Director, Global Practice Head, EDM for Energy, S&P Global
In the most recent oil and gas digital trends survey from Accenture and Microsoft [1] , two-thirds of upstream oil and gas professionals said analytics is one of the most important capabilities for transforming their company. The oil and gas industry is sitting on a wealth of data: from new geophones and logging tools to sensors, companies have access to massive and diverse data sets. The transformational impact of analytics provides a layer of insight on top of this data, helping to answer questions about what has happened, what could happen in the future and what steps should be taken next. As the industry moves towards an analytics-driven future, it should however heed the adage of ‘Garbage in, garbage out’. Analytics are only as good as the data that feeds them.
Good data management is a significant challenge for upstream oil and gas companies. Data volumes are now exceeding 10TB of data per day for a single well, for example. As this data pours in, it needs to be consumed by the business more quickly than ever before in order to make key decisions. In this drive for quicker access, quality is often sacrificed.
The typical technology architecture at oil and gas firms consists of four separate systems: ETL (extract, translate and load), MDM (Master Data Management), a data warehouse (or store) and some kind of analytics tool. As a result, there are multiple handoffs between disparate databases and systems with data often being manually moved between applications, and, in many cases, through an intermediate step, such as Excel.
Not only is this multi-application environment costly and cumbersome to maintain, but it also increases the potential for error in the data and introduces complexity in identifying, tracking and fixing data. Business users may be able to generate visually appealing reports in their business intelligence tools, but how accurate are they? Can users trace the source of data, the changes made to that data, by whom and when?
A more closely integrated architecture defined by workflows can help reduce the points of failure, enhance data quality and improve the data management process in general. With a solid data management foundation, combined with data collection and analysis more closely knitted together, oil and gas companies will be able to fully realize the potential of data, deliver accurate analytics and enable decisions to be made more quickly with the confidence that they are being made on good data.
Enterprise Data Management (EDM) from IHS Markit combines ETL, MDM and data warehousing into a single application, reducing support overhead, streamlining integrations and, importantly, empowering the user with full access to the data either within EDM or in any downstream analytics or BI tool. Data is validated, enriched and reconciled across multiple sources enabling full data lineage and a transparent audit trail.
By applying true data quality control measures, EDM eliminates the uncertainty and provides trusted data for analysis. Automating quality control also enables data managers to focus on the areas that need it most – the exceptions – and allows issues to be resolved before distribution downstream.
Removing the restrictions imposed by fixed data models, EDM leverages the models used by each company, eradicates the need for separate siloed data tools and enables all energy data, be it sub-surface, operations, midstream, economics or financial to be managed in a single environment.
To quote The Economist , “The world’s most valuable resource is no longer oil, but data”. If data trumps oil in terms of value, then surely this raw resource deserves some more attention.
[1] 2016 Upstream Oil and Gas Digital and Technology Trends Survey, sponsored by Accenture and Microsoft and conducted by PennEnergy Research <span/>
Nathan Amery, Director and Head of Strategy, EDM for Energy at IHS Markit [email protected]
Interested in learning more? Please visit EDM for Energy .
This article was published by S&P Global Commodity Insights and not by S&P Global Ratings, which is a separately managed division of S&P Global.
Mergers and Acquisitions Fueling Innovation and Growth in the CCUS Sector
Atlantic Margin’s favorable risk profiles position key basins for success
Higher and more stable oil prices are pushing oil sands growth higher … again
Use Kanaries Cloud for free as students and educators
Data Visualization in Oil & Gas Industry: A Complete Guide

Published on 7/24/2023
Introduction
Data visualization is a powerful tool that transforms raw data into meaningful and actionable insights. It allows us to understand complex data sets by representing them in a visual context, making it easier to identify patterns, trends, and outliers. In the oil and gas industry, data visualization plays a crucial role in making informed decisions, optimizing operations, and predicting future trends.
The oil and gas industry is a complex field with vast amounts of data generated from various sources such as seismic surveys, drilling operations, and production data. This data, when visualized effectively, can provide valuable insights that can lead to improved operational efficiencies, cost savings, and increased production.
Interested in the next-generation, AI-powered Data Visualization tool that is Open Source? You might want to take a look at RATH (opens in a new tab) ! RATH GitHub Link: https://github.com/Kanaries/Rath (opens in a new tab) Imagine you can easily clean and import your data (either in Excel, CSV files, or stored in online Databases), and generate data insights with visualization quickly and efficientl, and perform exploratory data analysis without complicated coding. That is exactly what RATH is designed for. Watch the following demo of RATH quickly identifying anomalies in data with the Data Painter feature:
Interested? RATH has more advanced features that rocks! Check out RATH website (opens in a new tab) for more details now!
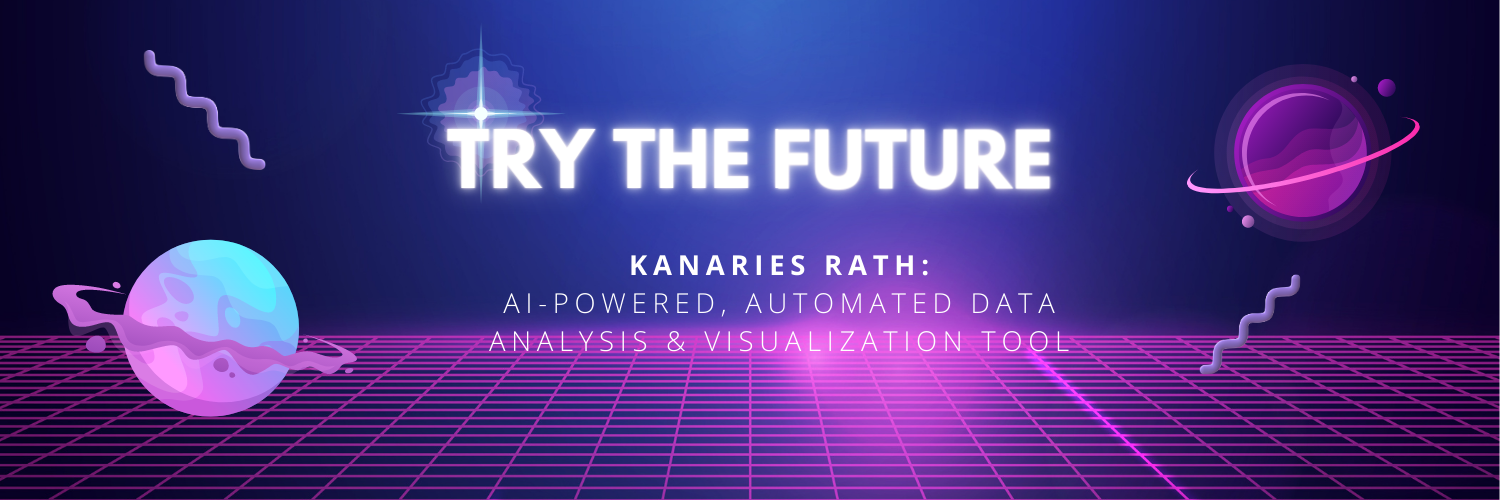
What is Data Visualization?
Data visualization is the process of representing data in a graphical or pictorial format. It enables decision-makers to see analytics presented visually, so they can grasp difficult concepts or identify new patterns. With interactive visualization, you can take the concept a step further by using technology to drill down into charts and graphs for more detail, interactively changing what data you see and how it’s processed.
In the context of the oil and gas industry, data visualization can be used to represent complex data sets such as seismic data, drilling logs, and production data in a way that is easy to understand and interpret. This allows industry professionals to make more informed decisions, optimize operations, and predict future trends.
Importance of Data Visualization in the Oil and Gas Industry
Data visualization is particularly important in the oil and gas industry due to the vast amounts of data generated from various sources. This data, if not properly visualized and interpreted, can lead to missed opportunities, inefficiencies, and increased costs.
For instance, seismic analysis, a key component of oil and gas exploration, involves the collection and interpretation of large amounts of data. Data visualization tools can help geologists and engineers to visualize this data in a meaningful way, enabling them to identify potential oil and gas reserves more accurately.
Moreover, data visualization can help in identifying operational inefficiencies in the upstream and downstream markets. By visualizing production data, companies can identify bottlenecks in their operations and take corrective measures to improve efficiency and reduce costs.
Best Data Visualization Tools for the Oil and Gas Industry
There are several data visualization tools available that are particularly suited for the oil and gas industry. These tools can handle the large and complex data sets typically found in this industry and provide powerful visualization capabilities.
Tableau : Tableau is a popular data visualization tool that allows users to create interactive and shareable dashboards. It can handle large data sets and provides a wide range of visualization options.
Power BI : Power BI is a business analytics tool developed by Microsoft. It provides interactive visualizations with self-service business intelligence capabilities.
TIBCO Spotfire : Spotfire is a powerful data visualization tool that is particularly suited for handling the complex data sets found in the oil and gas industry. It provides advanced analytics capabilities and can handle large volumes of data.
QlikView : QlikView is a business intelligence tool that allows users to create guided analytics applications and dashboards. It is particularly suited for handling the complex data sets found in the oil and gas industry.
These tools not only provide powerful visualization capabilities but also integrate with various data sources, allowing companies to pull in data from different systems and visualize it in a single platform.
Examples of Data Visualization in the Oil and Gas Industry
Data visualization in the oil and gas industry can take many forms, from simple charts and graphs to complex 3D models and simulations. Here are a few examples:
Seismic Analysis : Seismic surveys generate vast amounts of data that can be visualized to create a 3D model of the subsurface. This allows geologists and engineers to identify potential oil and gas reserves and plan drilling operations more effectively.
Drilling Data Visualization : Data from drilling operations, such as drilling speed, pressure, and temperature, can be visualized in real-time to monitor the drilling process and identify any potential issues.
Production Data Visualization : Production data, such as oil and gas flow rates, can be visualized to monitor the production process and identify any inefficiencies or bottlenecks.
Predictive Maintenance : Sensor data from equipment can be visualized and analyzed to predict when maintenance is required, reducing downtime and maintenance costs.
How Data Science Can Help the Oil and Gas Industry
Data science, which involves the use of statistical methods to extract insights from data, can provide significant benefits to the oil and gas industry. Here's how:
Predictive Analytics : Data science can be used to predict future trends, such as oil and gas prices, demand, and production levels. This can help companies to plan their operations more effectively and take advantage of market opportunities.
Optimization : Data science can be used to optimize operations, from drilling and production to distribution and sales. This can lead to significant cost savings and efficiency improvements.
Risk Assessment : Data science can be used to assess and manage risks, such as the risk of equipment failure or environmental impact. This can help to prevent accidents and reduce the potential for costly fines and reputational damage.
Exploration : Data science can be used to analyze and interpret seismic data, helping to identify potential oil and gas reserves and reduce the risk of dry wells.
Frequently Asked Questions
- What is the role of data visualization in the oil and gas industry?
Data visualization plays a crucial role in the oil and gas industry. It helps in transforming complex data sets into visual formats, making it easier to identify patterns, trends, and outliers. This aids in making informed decisions, optimizing operations, and predicting future trends.
- What are some examples of data visualization in the oil and gas industry?
Examples of data visualization in the oil and gas industry include seismic analysis, drilling data visualization, production data visualization, and predictive maintenance.
- How can data science benefit the oil and gas industry?
Data science can provide significant benefits to the oil and gas industry. It can be used for predictive analytics, optimization of operations, risk assessment, and exploration.
Oil and Gas Datasets
Key oil and gas datasets, accumulations →.
Oil and Gas Fields
Our Global Database of oil and gas fields provides key attributes on discovered hydrocarbons.
An accumulation on the map represents a distinct, discovered hydrocarbon pool of any size. Accumulations include Discoveries (economic or not), Fields that are Under Development, Producing Fields, and Fields that have Ceased Production. Attributes include Discovery Year, Hydrocarbon Type, Status and Description. Take a look at these datasets by signing up for free today .
For more advanced attributes, get in touch to discuss our Advanced Accumulation layer and oil and gas datasets.
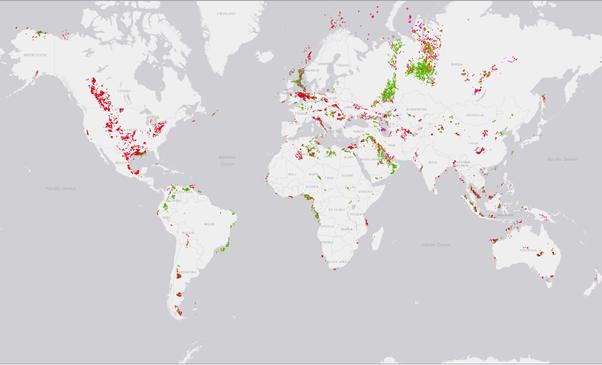
Blocks and Licences →
Licences, concessions and blocks.
We produce a series of layers to describe the location of oil and gas licences, key information on the terms and dates, as well as the companies and partners involved.
Blocks are used to define the grid system’s Administrative Areas for licensing. Grid systems can be regular or custom shapes can be used to show previous Licences/Concessions in the area.
Licence Header contains the basic information on a Licence, PSC, or Concession that is actively licenced for research, oil and gas exploration, production, geothermal or some renewable energy purposes. The layer also contains known applications and areas in Force Majeure.
Licence area or sub-area contains other parts of a licence such as unitised area of field areas. These can have their own operator and partnership distinct from the Licence Header.
Licence Area Partnership includes a licence area for each partner on the licence and their associated equity.
Take a look at our oil and gas datasets by signing up for free today .
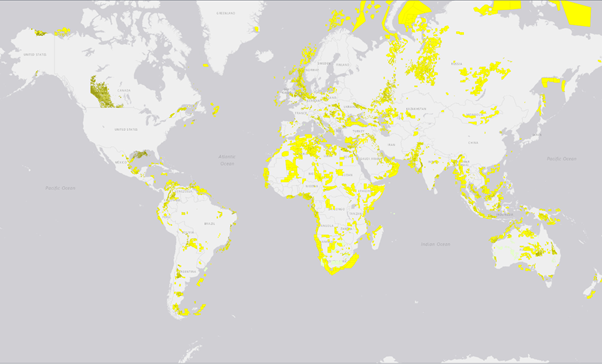
Licensing Rounds →
Licensing or bid rounds.
Licencing Rounds , Bid Rounds or Tender Rounds are times when areas are opened up for licensing. We cover oil and gas licensing rounds, along with bid and tender rounds for renewables.
This layer includes key dates and is tied to the Offered Areas layer which covers the individual blocks or areas on offer.
We have a distinct layer showing the Offered Areas as blocks/licences/concessions offered in a licensing round or tender.
Take a look at our licensing rounds datasets by signing up for free today .
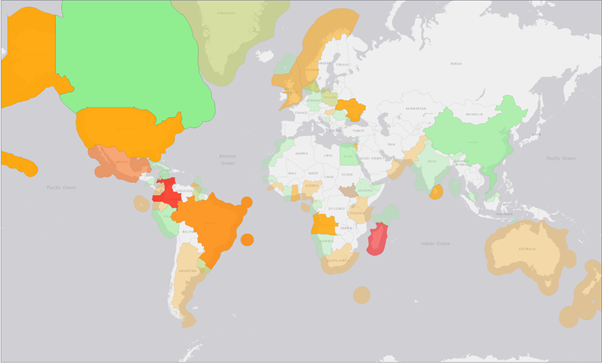
Boreholes →
The Borehole or Well layer includes oil wells, gas wells, geothermal wells and research on stratigraphic boreholes.
Well Headers contain the basic parameters that describe the location of the borehole, its purpose, result and current status.
Take a look at our boreholes, as well as other oil and gas datasets by signing up for free today .
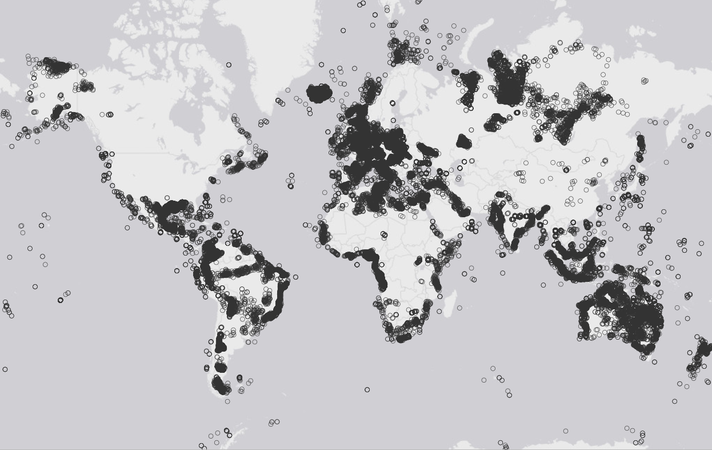
Seismic Surveys →
2d and 3d seismic surveys.
2D Seismic Surveys are made up of a series of 2D seismic lines often acquired or processed with similar parameters.
2D Seismic Lines show the location of 2D lines. These lines can form part of a 2D survey. Geophysical surveys are a key dataset used in understanding the subsurface structure and geophysical properties.
3D Seismic Surveys show the location of 3D seismic surveys. This dataset includes both original surveys and merged reprocessed areas. Datasets include both multi-client datasets and spec surveys.
Take a look at our seismic surveys datasets and other global data on oil and gas by signing up for free today .
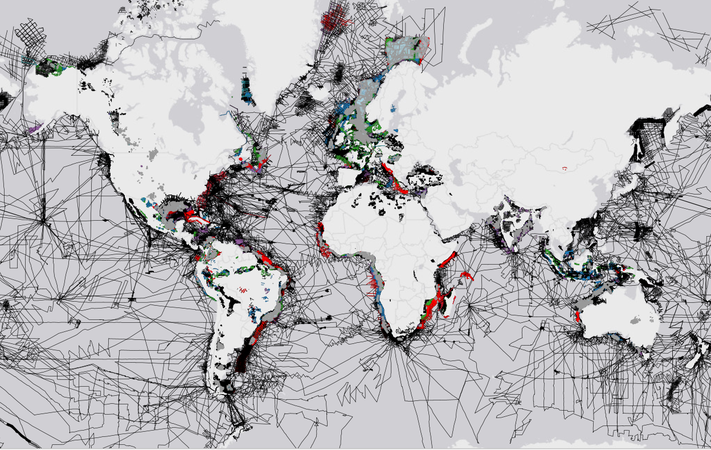
Pipelines →
Pipelines are used for transporting fluids. Our pipeline database includes global data Oil and Gas (Hydrocarbon, CO2, Hydrogen), Products (i.e Refined products), Chemicals and Water.
Take a look at our datasets for pipelines by signing up for free today .
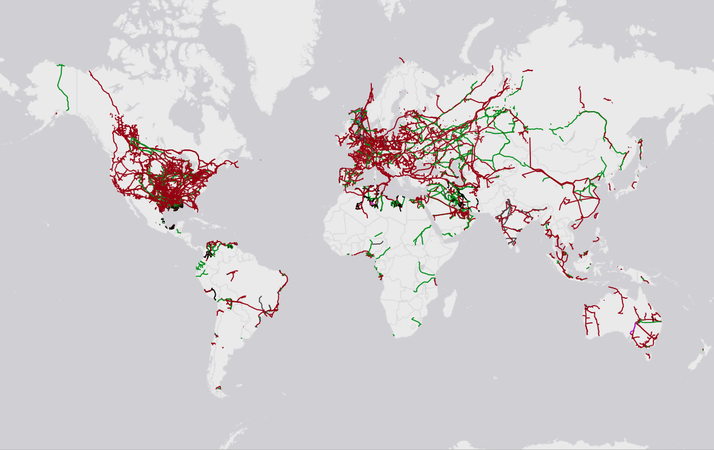
Terminals and Facilities →
Terminals and facilities.
Floating Facilities include FPSO (Floating Production, Storage and Offload), FLNG (Floating Liquid Natural Gas Facility), FSO (Floating Storage), and FSRU (Floating Storage Regasification Unit).
Platforms are offshore structures used in offshore wind or hydrocarbon development. These are predominantly in shallow water and fixed to the seafloor. Platforms can be small containing small production or processing units, or large with accommodation.
Seabed outlines all subsea infrastructure i.e. subsea production equipment, tanks or obstructions.
Terminals cover oil terminals, gas terminals, and LNG terminals. These generally have storage and have access to a port, pipeline road or rail for transporting the product.
Take a look at our datasets for terminals and facilities by signing up for free today .
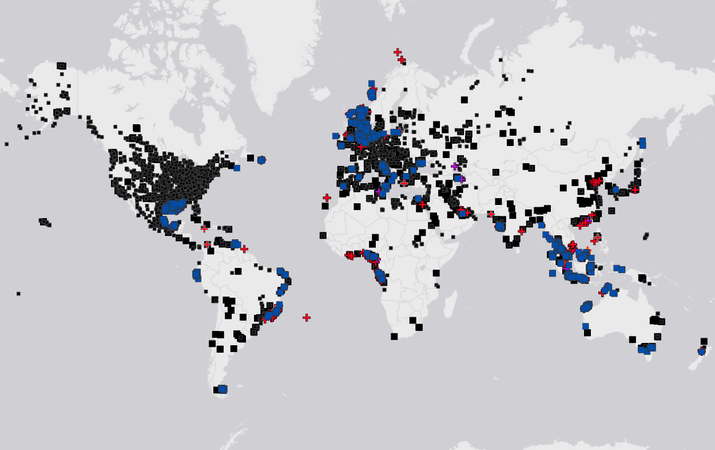
Additional Oil and Gas Datasets
Prospects and leads.
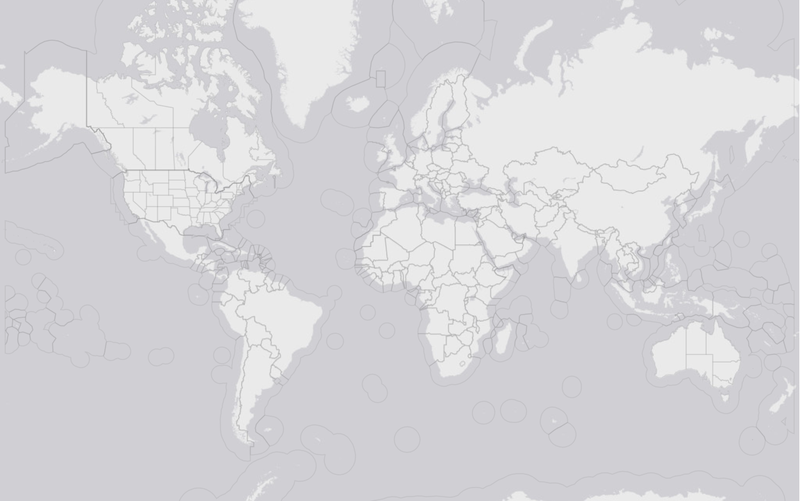
Prospects and Leads are structures or sites where there is a high possibility of finding hydrocarbons. A lead is generally higher risk and will require further technical work before it is drill-ready. Prospects should be well-defined with good seismic coverage and many of the key parameters are derisked.
Take a look at our datasets for prospects and leads by signing up for free today .
For more advanced attributes talk to us about our Advanced Undiscovered Accumulation layer.
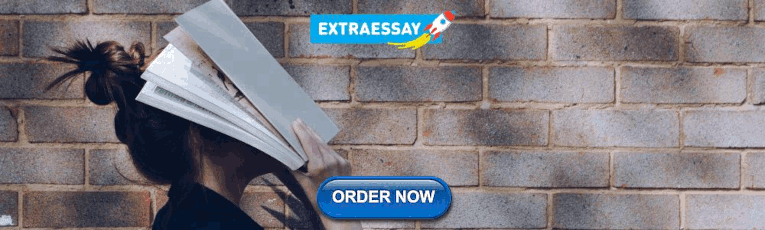
Admin Areas
Administrative Areas show countries or jurisdictions that manage Oil and Gas or Energy Licensing. This can be done at the Country, State, Territory or Regional level.
Within this layer, we include some areas which overlap due to disputes or unresolved territorial claims. In such cases, we try and show both sides of the claim without making a judgement on the validity of the claim.
Take a look at our datasets for admin areas by signing up for free today .
Rigs and Vessels
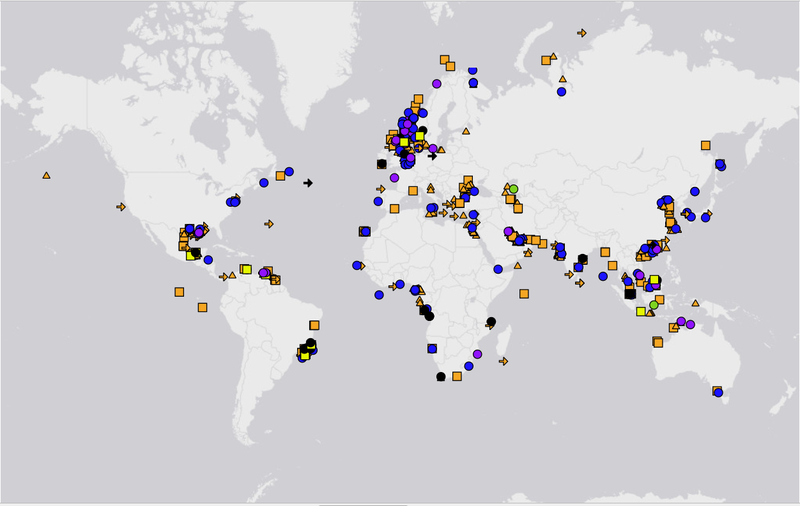
We track a fleet of key Oil Rigs and Vessels, locations are updated based on satellite AIS provided by Fleetmon. Rigs include Jack-ups, Semi-subs, Drill Ships and Barges. Vessels include Seismic, Offshore Support, Pipe-layer and Wind Support vessels.
Take a look at our datasets for rigs and vessels by signing up for free today .
Geological Basins
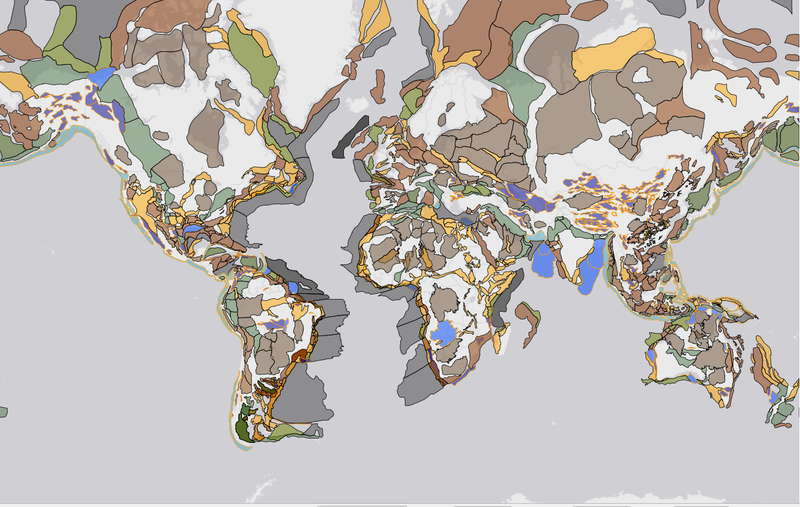
The Geological Basins layer describes the geological basins which are part of the global activity map.
Take a look at our datasets for geological basins by signing up for free today .
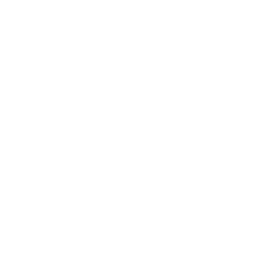
Seismic Lines
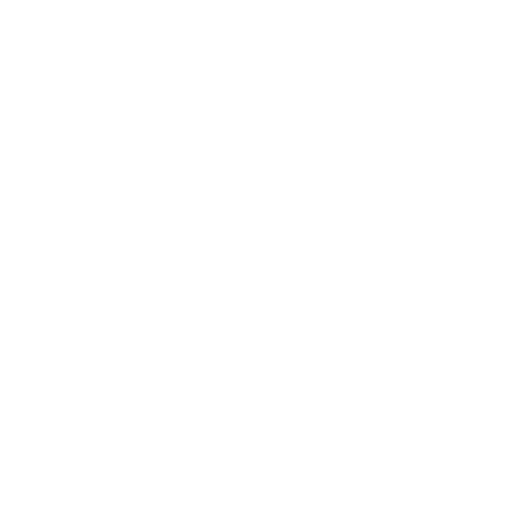
Data Sources
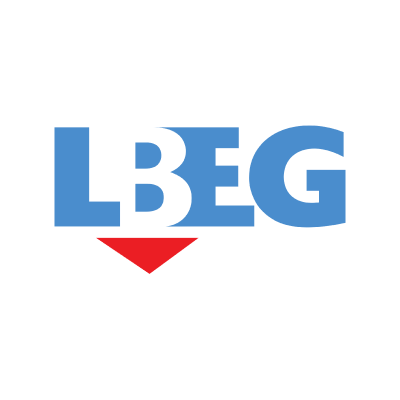
Ready to get started?
Join 307,012+ Monthly Readers
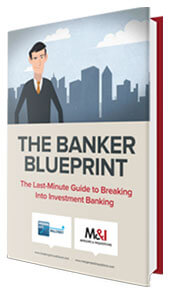
Get Free and Instant Access To The Banker Blueprint : 57 Pages Of Career Boosting Advice Already Downloaded By 115,341+ Industry Peers.

- Break Into Investment Banking
- Write A Resume or Cover Letter
- Win Investment Banking Interviews
- Ace Your Investment Banking Interviews
- Win Investment Banking Internships
- Master Financial Modeling
- Get Into Private Equity
- Get A Job At A Hedge Fund
- Recent Posts
- Articles By Category
Oil & Gas Financial Modeling 101
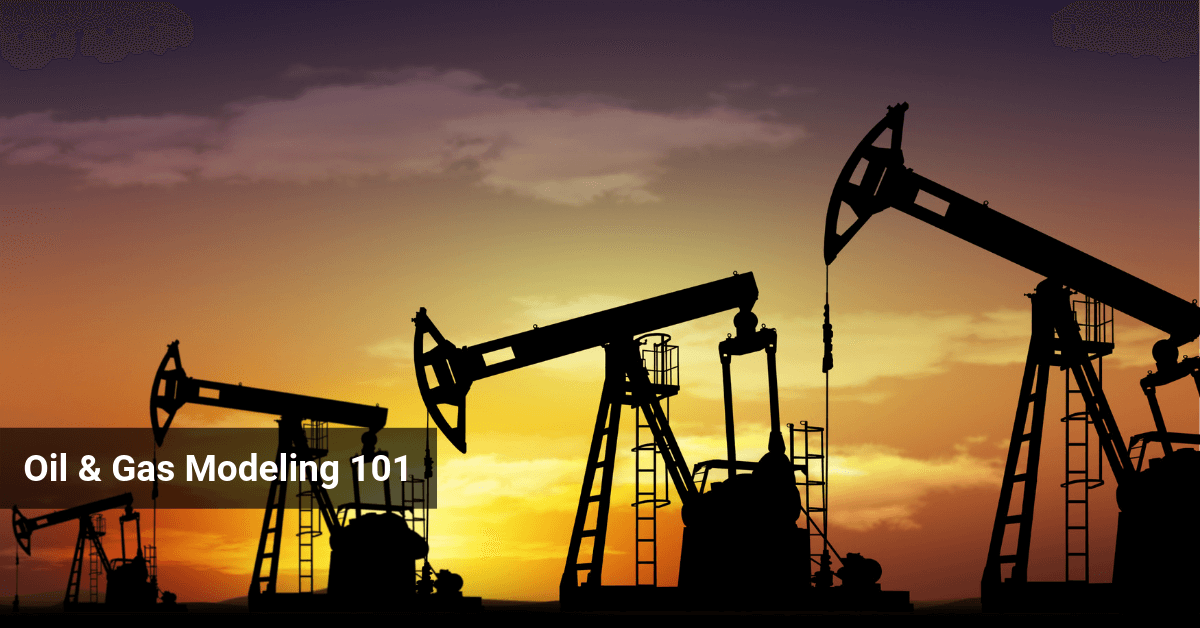
Out of all the industry-specific courses I’ve released, Oil & Gas Financial Modeling has drawn the most interest.
That surprised me at first because there’s no obvious reason why it would be more popular than the others.
But once you start learning something about the oil & gas industry, the reason becomes obvious: the strategies you use when modeling oil & gas companies apply to more than just oil & gas companies.
The obvious example is mining , where there’s a lot of overlap, but almost anything that depends on commodity prices is similar.
The good news is that while bank and insurance modeling is almost a different game entirely, oil & gas modeling is more like a variation on a game you’re already familiar with.
How Does the Oil and Gas Industry Work?
Oil & gas, mining, and other natural resource companies have a simple business model: find and extract valuable stuff from the ground, turn it into something useful, and then sell it to customers.
But if you read that statement carefully, you’ll immediately see how it gets more complex:
- So, what do you focus on? Finding and extracting valuable stuff? Turning it into something useful? Transporting and selling it to customers? All of those?
- If you make software or widgets or clothes, you can pretty much set prices yourself… within reason. Some products sell for $10, some for $1000, and there’s always a reason why. But if you sell gold, oil, or gas, you can’t determine the price – “the market” does.
Let’s address point #1 above first and see how the industry is divided:
- Upstream Companies: Also known as Exploration & Production, or E&P, companies, they focus on finding and extracting minerals and resources from the ground.
- Midstream Companies: They focus on transporting oil, gas, and other commodities to companies that will sell them. This is mostly done via “pipelines” that carry these commodities over great distances.
- Downstream Companies: They refine and market commodities (hence the “R&M” name for them), turn them into something useful – AKA jet fuel, automobile gasoline, diesel, and so on – and then sell them to customers.
- Oil Field Services: They don’t find, transport, or sell oil & gas, but instead provide services to companies that do. They might maintain their oil fields, repair broken spots, upgrade the technology, or even provide security for workers.
- Integrated Majors: Think BP and Exxon Mobil. These companies do everything above, just in different proportions. The most common combination is to focus on upstream and downstream, and then leave the rest to dedicated specialists.
How Are Oil & Gas Companies Different, Modeling-Wise?
For purposes of this tutorial, we’re going to focus on Upstream , or E&P (Exploration & Production) companies because those are the most “different” from normal companies – and they’re the most common topic in interviews.
We will also touch on diversified , or integrated major , companies, such as Exxon Mobil since you can learn a lot about other segments by analyzing them.
So how is oil & gas modeling different?
- You Can’t Control Prices or Revenue – This is the big difference that results in most of the other differences.
- They are Balance Sheet-Centric – Just like banks or insurance firms, an E&P company is far more dependent on its balance sheet than, say, a technology or consumer retail company. The balance sheet contains its most important asset: the reserves that will generate future revenue and profit.
- Accounting is Different – You not only see different line items, but you also see two different accounting methodologies: full cost and successful efforts .
- Depleting Assets Galore – For a normal company, assets tend to move… up and to the right. Items like PP&E, inventory, accounts receivable, and so on increase as the company grows. But as an oil & gas company produces more and more and earns revenue, its assets decline because they use up all those valuable resources they found in the ground.
- Cyclicality – Going along with the first point, oil & gas companies follow commodity price cycles. Investing in an energy or mining company is almost like investing in the underlying commodity, which means you have to be comfortable with giant price swings.
The good news is that unlike banks and insurance firms, oil & gas companies still sell tangible products to people – so your models are more similar.
Oil & Gas Financial Statements – Projecting Revenue and Expenses
Before you begin projecting an energy company’s financial statements, you need to know something about the units used.
Oil is typically measured in Barrels (1 Barrel = 42 Gallons, and yes, even countries that use the metric system still use Barrels); natural gas is measured in Cubic Feet (even with the metric system), and mining companies use whatever makes sense (iron ore/coal/aluminum/copper/lead/zinc/nickel/manganese/uranium = tonnes, diamonds = carats, and gold/silver = ounces).
You measure the company’s reserves (how much they have on their balance sheet, ready to extract, produce, and sell) and production (how much they produce and sell each day, month, quarter, year, etc.) in these units.
When you project a natural resource company’s statements, you begin by projecting its production by segment based on its reserves and its historical patterns.
So let’s say that a company has 12,000 billion cubic feet (12,000 Bcf) of natural gas in its reserves and produces 500 billion cubic feet (500 Bcf) annually.
You might assume a modest increase over that number, especially if the company is spending a lot on finding new resources.
So maybe you assume that they produce 550 Bcf next year and then 600 Bcf the year after – which you would then cross-check with equity research and their reserves (you don’t want to assume that they produce 100% of their reserves in 1 or 2 years).
And then you deduct this production from their reserves… and (hopefully) replace it with sufficient CapEx spending, linking the dollar amount of that spending to a specific amount of reserves.
Revenue is trickier because you can’t set prices yourself.
But the solution is surprisingly simple: you use scenarios in Excel.
So you might create a “low” scenario where oil prices are, say, $40 per barrel, a “middle” scenario where oil prices are $70 per barrel, and a “high” scenario where oil prices are $100 per barrel.
Doing that lets you see the range of possible outcomes for a company based on commodity prices.
There’s more to it than that because you also need to take into account hedging and the fact that the company will never get the full market price due to middlemen, commissions, and so on – but that’s the basic idea.
Expenses are more involved because you have both production-linked expenses – which you estimate on a dollar per barrel of oil or per cubic foot of gas basis – and then non-production-linked expenses , such as stock-based compensation and smaller, miscellaneous items.
Generally you look at a company’s filings and figure out what is production-linked and what isn’t, and then assume an increasing dollar value for the production-linked ones over time and make the non-production-linked expenses a percentage of revenue or other items.
To see a real-world example of these projections, click here to view a sample lesson from the Oil & Gas Modeling course on Price Hedging and Revenue by Segment .
Income Statement
Energy companies’ income statements do not have the usual Cost of Goods Sold / Gross Profit and Operating Expense distinction that you see for normal companies.
Instead, they are split into Revenue and (Operating) Expenses and then Other Income / Expenses. Here are the typical items:
- Revenue: Sometimes it’s categorized by Upstream vs. Midstream vs. Downstream; if the company just does Upstream, it is split into energy categories such as Gas & Natural Liquids, Oil, and Miscellaneous.
- Expenses: Production (anything associated with extraction from the ground); Taxes, Transportation & Other; Exploration (the cost of finding energy and minerals); Depreciation, Depletion , and Amortization (not only normal D&A but also the reserves being depleted over time); Selling, General & Administrative; Derivative Fair Value Gain / (Loss) (for companies that hedge); the Accretion of Discount in the Asset Retirement Obligation (more on this below).
- Other Income / (Expenses): You might see items like interest and investment income and expense and also gains and losses on asset sales, partnerships, and royalty trusts here.
The presentation is very similar to what you see for normal companies: Revenue Minus Expenses = Operating Income, Operating Income Plus Other Income / (Expenses) = Pre-Tax Income, and Pre-Tax Income * (1 – Tax Rate) = Net Income.
One final point: oil & gas companies tend to have very high deferred income taxes . Sometimes 75% or more of a company’s income statement taxes are deferred, meaning that they’re not paid in cash in the current period.
You see such high percentages because of the sky-high depreciation, depletion & amortization (DD&A) numbers for oil & gas companies and because many companies record them differently for book and tax purposes.
This doesn’t really affect the income statement, but you do need to add back deferred taxes on the cash flow statement.
Balance Sheet
Let’s start with the easy part: Shareholders’ Equity is pretty much the same for oil & gas companies.
Assets and Liabilities are still split into Current Assets, Long-Term Assets, Current Liabilities, and Long-Term Liabilities, but there are a few new items.
Sometimes, E&P companies also create a category for PP&E (Plants, Property & Equipment) on their balance sheet and list their reserves by category there (Proved, Unproved, and Other – basically, the probability of those reserves actually turning into something real).
- Current Assets: Cash & Cash-Equivalents, Accounts Receivable, Inventories, Derivative Fair Value (for companies that hedge), Income Tax Receivables / Deferred Tax Benefits, and Other.
- Long-Term Assets: Investments, PP&E (Proved, Unproved, Other, and Accumulated DD&A), Goodwill & Other Intangibles, and Other Assets.
And then on the Liabilities side:
- Current Liabilities: Accounts Payable , Current Portion of Debt, Derivative Fair Value (for hedging), Deferred Income Taxes, and Other.
- Long-Term Liabilities: Long-Term Debt, Pension Benefits, Deferred Income Taxes, the Asset Retirement Obligation , and Other.
If you’re familiar with normal companies, most of these items are self-explanatory and similar to what you see elsewhere. So I want to focus on the ones that are different or that don’t exist in other industries:
- Derivative Fair Value: This can actually show up everywhere – current assets, long-term assets, current liabilities, and long-term liabilities. Energy companies use hedging to protect themselves from falling commodity prices, and these “derivatives” consist of contracts that say, for example, “Even if oil prices fall to $50 per barrel, I can still buy oil from you at $60 per barrel.” Derivatives on the assets side represent long positions and on the liabilities side they represent short positions.
- PP&E: Normal companies have this as well, but oil & gas companies often break it into categories such as Proved Reserves , Probable Reserves , and Possible Reserves ; Proved Reserves have a 90%+ chance of being recovered, Probable is between 50% and 90%, and Possible is less than 50%. Accumulated Depreciation, Depletion & Amortization is also huge and is a contra-asset that’s counted against Gross PP&E to arrive at Net PP&E here.
- Asset Retirement Obligation: Just as it costs money to open up new oil wells and gas fields, it also costs money to shut them down . This liability represents that future cost, and you add to it over time by adding in the future cost of shutting down each new well or field you develop (reflected in the accretion of the asset retirement obligation discount on the income statement).
Cash Flow Statement
Similar to other industry-specific cash flow statements, oil & gas cash flow statements are very similar to what you see for normal companies: start with net income, add back non-cash charges, take into account working capital changes , and then go through the normal investing and financing sections.
The main differences lie in the magnitude and frequency of some of the items:
- Depreciation, Depletion & Amortization: Massive for O&G companies and sometimes even bigger than their net income.
- Other Non-Cash Expenses: Besides the usual ones, you’ve also got the accretion of discount in the asset retirement obligation, gains and losses on asset, partnership, and royalty sales, and possibly something called the dry hole expense (see the upcoming section on Successful Efforts vs. Full Cost).
- Investing Activities: CapEx is massive for natural resource companies, once again often exceeding their net income. Asset sales are also very common. These companies spend a huge amount exploring and buying assets, and also receive a good chunk of change for selling assets like individual oil fields and gas wells.
- Financing Activities: Since oil & gas companies are constantly buying assets and spending a small fortune to find more assets and since they deal with volatile commodity prices, they have huge financing needs. So you see massive amounts of debt being raised and paid off, along with equity financing in some cases.
How Everything Flows Together
We already went through part of this one with the revenue and expense projections above, but just to recap:
- You start by projecting annual production on a per-commodity basis, and then assume low, mid, and high price cases for each commodity to build multiple scenarios in Excel.
- Based on these numbers, you calculate revenue . You project expenses either on a production-linked basis (e.g. Production, Taxes, Transportation, DD&A) or a non-production-linked basis (Stock-Based Comp., Derivative Gains / (Losses), etc.).
- On the cash flow statement , you start with net income, add back non-cash charges (mostly flowing in from the income statement), and make sure working capital links in properly from the balance sheet once you’ve projected the BS in the next step.
- You also link CapEx to production and translate the dollar amounts back to natural resource units (Barrels, Cubic Feet, Tonnes, Ounces, etc.) to keep a running tally of how the company’s Reserves change over time – subtract Production, add new discoveries and acquisitions, and subtract sales of reserves.
- Debt and equity financing activities would be determined by a debt schedule you create; you could set these to $0 to simplify the model, but almost all energy companies need to raise capital consistently so you should at least make a simple estimate.
- Most of the balance sheet items flow in from the cash flow statement or can be linked to income statement items (e.g. accounts receivable to revenue); you will hold many of the items constant (Goodwill) or set them to $0 to simplify in some cases.
To get a sense of what the financial statements look like for a real company, click here to check out XTO Energy’s statements from just before they were acquired by Exxon Mobil .
A diversified oil & gas company has slightly different statements and you see more items related to its midstream and/or downstream capabilities; for a good example, click here to view Exxon Mobil’s financial statements .
Successful Efforts vs. Full Cost
Remember how I said above that oil & gas companies use “different” accounting?
Revenue recognition is straightforward, but the expenses get tricky. You always capitalize acquisitions and development (actually constructing the field or well), and you always expense production.
But exploration costs are more subtle: it seems obvious that you’d capitalize successful explorations, i.e. if you go out into the fields and actually find oil, but what about unsuccessful explorations?
Under the successful efforts methodology, you expense them, and under the full cost methodology you capitalize them and add that CapEx to the PP&E on your balance sheet.
That seems straightforward, but it gets confusing on the other financial statements because some companies apply these standards inconsistently and use a “mix” of both.
That “dry hole expense” I mentioned above is another name for unsuccessful exploration , and some companies actually add it back on their cash flow statements (long story, but essentially they are using a mix of both standards).
But there is some good news: if you’re outside the US and therefore use IFRS, all companies must use the “successful efforts” standard.
Large companies tend to use successful efforts because they can afford the hit to their net income, while smaller companies tend to use the full cost method to boost their earnings.
One downside of the full cost method is that you need to test the PP&E balance every so often and apply write-downs if the book value gets out of line with the market value – so write-down and impairment charges are common on full cost companies’ income statements.
Oil & Gas Valuation
The good news is that most of the same valuation methodologies you’re used to seeing – public comps, precedent transactions, and even the DCF model – still apply to (most) oil, gas & mining companies.
The bad news is that the metrics and multiples involved are different:
- No P/E or Revenue Multiples: P/E is not terribly useful because many energy companies have odd tax situations, huge depreciation numbers, and lots of impairment charges and write-downs; revenue multiples are useless because oil & gas companies have limited control over their revenue due to their dependence on commodity prices.
- EBITDAX, not EBITDA: The “X” stands for Exploration Expense, and we add back it to EBITDA when working with E&P companies because of the issue with successful efforts vs. full cost accounting above: we’re normalizing the metric. Sometimes you do see EBITDA as well, especially for more diversified companies, non-E&P ones, and even for the occasional E&P set if the companies all use one accounting standard.
- Production and Reserves Multiples: Since oil & gas companies are so dependent on natural resources, why not make the resources into multiples? And that’s exactly what you do with their Proved Reserves and Daily or Annual Production numbers. You use Enterprise Value in the numerator for both of these, and the values might be anywhere from $1.00 to $50.00 depending on the companies, region, economy, and so on.
- Different Screening Metrics: Rather than using revenue or EBITDA, you might pick companies and transactions based on Proved Reserves or Production volume; other important metrics include the R / P Ratio (Proved Reserves / Annual Production), the % Proved Reserves in their Total Reserves, and the % Oil Mix.
So there are some differences, but the mechanics of selecting and calculating the metrics and multiples for comps remain the same.
You can still use a DCF, but there are a few issues with the traditional Unlevered Free Cash Flow approach :
- Capital Expenditures tend to be massive, which depresses Free Cash Flow and makes almost all of the company’s value depend on the Terminal Value.
- Perpetual Growth is a big leap for oil & gas companies. There’s a limited amount of oil and gas on planet Earth, so can you really assume that the company will grow at 3%, 2%, or even 1% into perpetuity?
You do still see DCFs sometimes, but they are more common for midstream, downstream, and oilfield services companies.
For E&P companies, there’s an alternate intrinsic valuation methodology called the Net Asset Value (NAV) model that often gives more accurate results.
The Net Asset Value (NAV) Model
The NAV model flips the traditional DCF on its head because you no longer assume perpetual growth .
Instead, you assume that the company adds nothing to its reserves and that it produces 100% of its reserves until it runs out of natural resources completely.
Here’s a rough outline of how it works:
1. Set Up Columns to Track Each Commodity, Revenue, Expenses, and Cash Flows.
You want to track the beginning and ending reserves each year, the annual production volume, and the average price for each commodity; typically you use the same low/mid/high price cases that you used in the company’s operating model.
2. Assume Production Decline Rates and Calculate Revenue Until the Reserves Run Out.
Depending on the company’s previous history, you might assume a decline rate of 5-10% per year – potentially more or less depending on how mature it is.
In each year, you assume that you produce either the production volume of that year or the remaining reserves – whichever number is lower.
Then, you’d multiply the production volume times the average price each year for all commodities to get the revenue by year.
3. Project (Some) Expenses.
You focus on Production and Development expenses here, both of which may be linked to the company’s production in the first place.
You don’t assume anything for Exploration since you’re pretending that the company finds nothing and dwindles to $0 in the future, and you leave out items like corporate overhead and SG&A because we’re valuing the company on an asset-level .
4. Calculate and Discount After-Tax Cash Flows
Simply subtract the expenses from the revenue each year and then multiply by (1 – Tax Rate) to calculate the after-tax cash flows.
Then, you add up and discount everything based on the standard 10% discount rate used in the Oil & Gas industry (no WACC or Cost of Equity here).
5. Add in Other Assets and Business Segments
For example, if the company has undeveloped land or if it has midstream or downstream operations, you might estimate the value of those based on an EBITDA multiple (or $ per acre for land) and add them in.
You add all those up to arrive at Enterprise Value , then back into Equity Value the normal way, and calculate the company’s Implied Share Price by dividing by the diluted shares outstanding.
So that’s a Net Asset Value model in a nutshell. It is widely used in oil, gas, mining, and other commodity-based sectors, and it often produces more accurate results than the standard DCF analysis.
To get a real world example of this NAV model, click here to view a sample video on how to set up the revenue side in a NAV analysis for XTO Energy .
Sum of the Parts
I hinted at this in the last part of the NAV explanation above, but sum of the parts is a very common valuation methodology in the energy industry.
For cases where the company is highly diversified – think Exxon Mobil – you need to value its upstream, midstream, downstream, and other segments separately and add up the values at the end.
So you might, for example, use traditional multiples like EBITDA for the midstream and downstream segments, and then use Proved Reserves or Production multiples for the upstream segment and add them together to arrive at the final value.
Or you might use NAV for upstream and a DCF for other segments and add those up.
Merger Models and LBO Models
There’s surprisingly little to say about merger models and LBO models in the oil & gas industry.
A merger model is a merger model is a merger model no matter how the company earns revenue, so nothing changes the fact that you need to combine all 3 statements, allocate the purchase price, and factor in synergies, acquisition effects, and so on.
The key differences with merger models:
- Purchase Method: Stock tends to be very common because oil & gas companies are often highly leveraged (little capacity to raise additional debt) and also don’t have much cash available.
- Commodity Prices: Both the buyer and seller should be using the same price cases – you don’t want an acquisition to look good just because you’re assuming $50 per barrel for the buyer and $60 per barrel for the seller.
- Synergies: You generally assume no revenue synergies because they just don’t make sense – remember that commodities companies cannot control their “product” prices. You might assume expense synergies based on reducing production costs on a per Barrel / Cubic Foot / Tonne / Ounce basis.
- Accounting: If you have a full cost company buying a successful efforts one or vice versa, you need to normalize both companies’ statements and use the same standard.
- Sensitivities: In addition to the usual criteria (purchase method and synergies), you also need to include commodity prices in your sensitivity tables because they will impact whether or not the deal is viable.
You could also base a contribution analysis or accretion / dilution calculation on non-financial metrics, such as Production Per Share or Proved Reserves Per Share.
But those make more sense for 100% stock-based deals (you wouldn’t see the impact of foregone interest on cash or interest expense on new debt for these non-financial metrics).
LBO models are even more similar to what you see for normal companies, and just like with merger models you need to include a sensitivity analysis on commodity prices somewhere in your model.
The most important point about Oil & Gas LBO models, ironically, is that oil & gas leveraged buyouts rarely happen .
And you already understand why if you’ve made it this far:
- Extremely Unstable and Unpredictable Cash Flows: If oil, gas, or gold prices swing one or the other, your returns may be hosed.
- High CapEx: This makes it much harder to repay additional debt.
- Already Highly Leveraged: This makes it difficult for the private equity firm to use a lot of new debt to acquire the company.
And the list goes on.
Yes, some PE firms do focus on energy and mining, but typically they stick to utility and/or power generation companies rather than unpredictable E&P companies.
Ready For Oil & Gas Financial Modeling Mastery?
Going into this, I actually thought the Oil & Gas tutorial would be the easiest and shortest one to write (whoops!).
There are a lot of differences with oil, gas, and mining companies but the overarching ones are that they cannot control prices and that they have depleting assets that constantly need to be replaced.
Remember that, the accounting tips above, and the NAV model, and you’ll be more than ready to dominate your interviews.
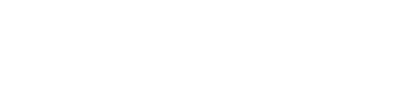
Along with Stanford news and stories, show me:
- Student information
- Faculty/Staff information
We want to provide announcements, events, leadership messages and resources that are relevant to you. Your selection is stored in a browser cookie which you can remove at any time using “Clear all personalization” below.
The researchers used two different but complementary approaches to measuring methane emissions from specific facilities via airplane-borne sensors. (Image credit: Carbon Mapper)
Oil and gas operations across the United States are emitting more than 6 million tons per year of methane, the main component of natural gas and the most abundant greenhouse gas after carbon dioxide, according to Stanford-led research published March 13 in Nature.
These emissions, which result from both intentional vents and unintentional leaks, amount to $1 billion in lost commercial value for energy producers. The annual cost rises to $10 billion when researchers account for harm to the economy and human well-being caused by adding this amount of heat-trapping methane to Earth’s atmosphere.
The new emission and cost estimates are roughly three times the level predicted by the U.S. government. The results are based on approximately 1 million aerial measurements of U.S. wells, pipelines, storage, and transmission facilities in six of the nation’s most productive regions, including the Permian and Forth Worth in Texas and New Mexico; California’s San Joaquin basin; Colorado’s Denver-Julesburg basin; Pennsylvania’s section of the Appalachian basin; and Utah’s Uinta basin. In all, the infrastructure surveyed in this study accounts for 52% of U.S. onshore oil production and 29% of gas production.
Troubling trends
Emissions in three of the six regions were well above expected values. The New Mexico portion of the Permian Basin was by far the highest emitter, with nearly 10% of total methane volume produced in 2019 going straight to the atmosphere. Surveys of some other regions, however, revealed emission rates well below U.S. EPA Greenhouse Gas Inventory estimates based on national averages, suggesting that good practices can reduce emissions.
“Costs aside, the main message here is that some regions show emissions at rates well above those the government itself uses to estimate methane losses,” said senior study author Adam Brandt , an associate professor of Energy Science & Engineering at the Stanford Doerr School of Sustainability . “We hope this will spur government methane inventories toward greater incorporation of remote sensing data at the heart of those estimates.”
Methane breaks down faster than carbon dioxide, but it is about 80 times more powerful than CO 2 when it comes to trapping heat during its first 20 years in our atmosphere. In that time frame, climate damage from the 6 million tons of annual methane emissions found in this study is roughly equivalent to a full year of carbon emissions from all fossil fuel use in Mexico.
Because methane can trap so much heat in the short term, accurate assessments of methane leaks are key to predicting the impacts of climate change that will be felt in our lifetime and verifying emissions reductions at a time when the U.S. and more than 100 other countries have pledged to cut emissions 30% below 2020 levels by 2030.
Eyes in the sky
By showing that leaks cost industry more than a billion dollars a year, the researchers hope to gain producers’ attention and motivate them to voluntarily stop emissions at their own facilities as a cost-saving measure. Additionally, the researchers say total costs from methane leaks and vents in the six-region study area are likely much higher, as the survey covered less than half of the facilities in the area.
The authors found fewer than two percent of emitters are responsible for 50 to 80% of emissions in all surveyed regions except for Colorado’s Denver-Julesburg basin and Utah’s Uinta basin. In terms of types of production facilities most likely to leak, the study noted that midstream infrastructure was responsible for about half of total emissions, which is higher than previous estimates. Midstream infrastructure includes gathering and transmission pipelines, compressor stations, and gas processing plants that shuttle gas from the wells to cities and towns.
“Solving the methane challenge is not quite as easy as simply finding and fixing a handful of leakers, as these stark numbers might suggest, but it does mean that efforts concentrated on relatively few operations could have considerable benefits,” said lead study author, Evan Sherwin , a research scientist at Lawrence Berkeley National Laboratory who worked on the research as a postdoctoral scholar in Brandt’s lab at Stanford.
“Climate change mitigation starts with better tracking of emissions, of course, but also accurate tracking of reduction efforts going forward.” —Adam Brandt Associate Professor of Energy Science & Engineering
Measurement matters
The research combined direct aerial measurements with an emissions simulation tool developed in Brandt’s group at Stanford by study co-author Jeffrey Rutherford , PhD ’22, to estimate the emissions that would be too small for the aircraft to reliably detect. The companies Kairos Aerospace and Carbon Mapper provided two different but complementary approaches to measuring methane emissions from specific facilities via airplane-borne sensors.
Total estimated leaked emissions range from just less than one percent to as much as 9.6% of total volume, with an average of 3% across the surveyed regions. The federal government estimates that methane emissions from oil and gas facilities nationwide average roughly 1% of gas production. Sherwin noted that in the surveyed regions of Pennsylvania and Colorado, the team’s estimates were on par with or lower than estimates from the U.S. Environmental Protection Agency .
“Climate change mitigation starts with better tracking of emissions, of course, but also accurate tracking of reduction efforts going forward,” Brandt said. “The method introduced here offers a path to combining measurements at several scales to greatly improve inventories that should lead us to much better tracking of those important reductions critical to national mitigation commitments.”
Brandt is also faculty director of the Stanford Natural Gas Initiative and a senior fellow at the Stanford Precourt Institute for Energy . Rutherford is now employed at Highwood Emissions Management. Additional Stanford co-authors include Energy Science & Engineering PhD students Zhan Zhang and Yuanlei (Yulia) Chen . Other coauthors are affiliated with Kairos Aerospace, Carbon Mapper, University of Arizona, and NASA Jet Propulsion Laboratory.
This study was funded by the Stanford Natural Gas Initiative, NASA’s Carbon Monitoring System and Advanced Information System Technology programs, Carbon Mapper, RMI, the Environmental Defense Fund, the California Air Resources Board, the University of Arizona, the U.S. Climate Alliance, Arizona State University’s Global Airborne Observatory , and the Mark Martinez and Joey Irwin Memorial Public Projects Fund with the support of the Colorado Oil and Gas Conservation Commission, the Colorado Department of Public Health and Environment. Portions of this research were carried out under a contract with NASA.
To read all stories about Stanford science, subscribe to the biweekly Stanford Science Digest .
Media Contacts
Evan Sherwin, Lawrence Berkeley National Laboratory: [email protected]
Adam Brandt, Stanford Doerr School of Sustainability: [email protected]
Josie Garthwaite, Stanford Doerr School of Sustainability: (650) 497-0947, [email protected]
Create an account
Create a free IEA account to download our reports or subcribe to a paid service.
News and commentaries
- Toggle filter Italy (1)
- Region Chevron down
- Technology Chevron down
- Toggle filter Electricity (4)
- Toggle filter Industry (3)
- Toggle filter Renewables (3)
- Toggle filter Transport (2)
- Toggle filter Buildings (1)
- Toggle filter Carbon Capture, Utilisation and Storage (1)
- Toggle filter Energy Efficiency and Demand (1)
- Toggle filter Fossil Fuels (1)
- Toggle filter Climate Change (1)
- Toggle filter COP28: Tracking the Energy Outcomes (1)
- Toggle filter Critical Minerals (1)
- Toggle filter Energy Efficiency (1)
- Toggle filter Energy Security (1)
- Toggle filter Renewable Integration (1)
- Programme Chevron down
- Toggle filter News (1)
- Toggle filter 2024 (1)
- Toggle filter 2023 (1)
- Toggle filter 2022 (2)
- Toggle filter 2020 (2)
- Toggle filter 2019 (2)
G7 ministers draw on wide range of IEA recommendations to strengthen energy security and accelerate clean energy transitions
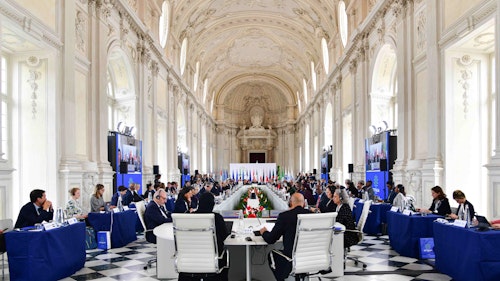
Subscription successful
Thank you for subscribing. You can unsubscribe at any time by clicking the link at the bottom of any IEA newsletter.
Capturing the next frontier of value: Operating models for oil and gas fields of the future
As predictions of an early peak in oil demand take hold, upstream operators must find ways to produce more energy, more efficiently. Many have made significant performance gains in recent years. Across the sector, production costs are down 30 percent; safety incident frequency has fallen by a third; and production losses have declined by 15 percent since 2014. Yet more is necessary.
Stay current on your favorite topics
A marked spread in performance remains between bottom and top quartile operators in every basin. On the UK Continental Shelf (UKCS), for instance, over 40 percentage points separate the lowest production efficiency asset from the top quartile. Similarly, the highest cost asset on the UKCS has twice the unit operating cost as the median and four times that of the top quartile in the basin. 1 1. Data from the Energy Insights Global Operations Benchmark.
Furthermore, new technologies and ways of working are resetting top quartile performance levels. Our research 2 2. Why oil and gas companies must act on analytics; Anders Brun, Monica Trench, and Thijs Vermaat describe the increase in production potential to be captured by deeper advanced analytic methods. shows digital technologies may improve total cash flows by USD 11 per barrel across the offshore oil and gas value chain, adding USD 300 billion a year by 2025.
What distinguishes the success cases from the also-rans? What sustains their improvement momentum? Through our extensive experience of leading asset turnarounds in Petroleum Asset eXcellence , we observe that upstream operators who sustain their improvement momentum do two things well.
First, they challenge five interlinked drivers of their operating model in an integrated way (Exhibit 1). These drivers are: their asset strategy; physical equipment-in-place; work required to operate and maintain that equipment; workflows and methods used to conduct that work; and the competencies required from the team deployed to do it. While each driver will yield some efficiency gains when used alone, in aggregate, they can more than double the value potential of existing operations.
Second, having had one go at improving their operating model, these operators are willing to build on what did not work in round one, and take a second, third, or even fourth look. In fact, they build a continually evolving operating model that achieves higher and more predictable production performance, operating costs for a ‘lower forever’ price environment, and smaller, flexible and more diverse teams that are better suited to the industry’s aging pool of skilled labor.
This article lays out a concrete logic that any operator might use to develop a continually evolving operating model and illustrates through real examples the success factors of making this change happen.
Developing a clean-slate vision of your operating model
In early 2015, an operator with upstream assets in various life stages found itself with negative cash flows, declining production and escalating costs. A vertiginous price drop and unconvincing track record of operational performance made any prospect of recovery seem unlikely. The operator went back to a clean slate: it took a hard look at its field and hub strategies—reprioritizing its efforts across near-field exploration, wells-reservoirs-facilities management and asset rejuvenation; made radical choices to optimize lifting costs and staffing levels; and pursued capital productivity relentlessly across its portfolio. Over the next year, as the operator’s competitiveness improved, its confidence rose as well.
It took another look at its operating model, replicating this end-to-end clean-slate approach, and emerged with an ambitious agenda to restore positive cash flows within two years. Since then, this operator has divested non-core assets, rezoned unwanted surplus capacity on declining assets, improved front-line agility, and embraced digital technologies. With a continually evolving operating model, it has reverted to positive cash flows a year earlier than planned, marking a first in its recent history.
1. How does your asset strategy fit with your asset’s life stage?
Exploration and production (E&P) companies rarely look at asset strategies in operational excellence programs. This is a missed opportunity. Clean-slate asset strategies help operators make deliberate choices on which fields to grow, operate as mature, swap with others, abandon, or divest. A Western European operator with mature operations realized that half the fields in its portfolio would generate 95 percent of its future cash flows. Consolidating the portfolio would free up scarce capital and talent for its most productive assets with material remaining reserves. Moreover, legacy ownership structures concealed bottlenecks in third-party infrastructure: this restricted current operating capacity and the ability to mature reserves through production. Redrawing portfolios in line with which operator-controlled critical processing capacity and evacuation routes — swapping assets and acreage with contiguous operators, for instance — could improve the basin’s future economics and simplify day-to-day operations for individual parties.
A regular discipline of considering clean-slate asset strategies — commonly in an annual cycle — helps revisit field development plans and improve recovery rates. An African client with a portfolio of 800 closed-in wells concluded that intervening in a mere 5 percent of the closed-in well stock could add 30 kboe/d in the first year, with payback also within the same period. It made wells and reservoir management a top priority in capital allocation and operational plans across its upstream portfolio. 3 3. An analytical approach to maximizing reservoir production: Francesco Verre, Otto van der Molen, and Anton Maximenko explore the recent impact they have had by applying deeper analytics to subsurface optimisation.
Would you like to learn more about Petroleum Asset eXcellence (PAX) ?
More than all else, clean-slate asset strategies enable customization of our remaining four drivers based on whether an asset is going through growth or decline. Operators commit to building and maintaining additional capacity, such as capital-intensive facilities improvement programs, only where there are remaining reserves and future value potential, or they eliminate expensive optionality wherever the asset’s maturity makes it irrelevant to future value creation.
2. What is the leanest physical footprint for your asset?
The physical footprint of an asset has always been a major driver of project economics. With increasingly small and stranded reserves and limited discretionary spending, it has become the single largest factor in project break-evens. Additionally, the physical footprint shapes operational processes, and determines the structural limits of operating cost optimization across asset lifecycles. Examples of these limits include deck space, number and type of crane, storage and layout, and redundancy in installed equipment. We recommend that operators consider the total value of owning their physical footprint — in design and in operations.
For new builds, considering the total value of owning their physical footprint may lead to smaller, modular, unmanned or energy self-sufficient designs. A North Sea independent used a standard platform design to shorten the engineering process and achieve first gas within 18 months versus industry averages of 30 to 36 months. The standard topsides — developed for two marginal fields were usable in other fields within a comparable range of gas throughput. The modular jacket was suitable for similar shallow water resources. Solar and wind power generation with battery storage reduced air emissions and offered energy self-sufficiency. Standardization and modularity rationalized maintenance costs just as much as FEED capital. As routines were replicable across the portfolio, a standard campaign-based maintenance approach yielded material synergies in engineering, work preparation, and spares management.
For mature assets, standard subsea design and equipment improves the economic attractiveness of brownfield expansions. Besides, obsolescence, fatigue or corrosion issues can all serve as triggers to make the asset easier and more economic to maintain. One operator in West Africa replaced traditional flowlines with thermoplastic ones. With better corrosion resistance, higher asset integrity and longer life, these new materials drastically extended schedules for inspections and maintenance routines. In a different example, a North Sea late-life asset systematically challenged the equipment in place to reduce surplus capacity in power generation, compression, and storage vessels. The lower physical footprint eliminated 25 percent of required maintenance hours and allowed redeployment of the maintenance team to more pressing pre-Cessation-of-Production imperatives. With a total value of ownership approach, this operator tackled the growing divergence of needs from means in its initial operating envelopes, and structurally reduced its operating cost base.
3. How can you compress your workload?
In asset turnarounds, we commonly encounter over-reliance on time-driven maintenance philosophies. Equipment strategies are set to standard specifications and adapted marginally as assets move through steady-state production into decline. The outcome is inflated workloads and costs, combined with an operations and maintenance plan that does not adapt adequately to emerging reliability or integrity challenges. Our proprietary maintenance benchmarks indicate that there can be a 5 to 10 percentage point differential in production efficiency and 20 to 30 percentage point differential in maintenance costs between top quartile operators and the also-rans.
Success cases exercise both traditional and digital levers to optimize the overall operations and maintenance workload. Traditional choices include stepping away from a 100 percent inspection approach to risk-based strategies in mid-life assets or run-to-failure for late life ones. However, next-generation operations and maintenance is centred on equipment sensors for performance data, advanced analytics and machine learning to predict and avoid failures, with maintenance or replacement on an as-needed basis. This end-to-end digitally enabled system makes activity workloads smaller and more predictable, feeds into more efficient and economic management of materials and people, and levels the operational risk-return profile of an oil and gas business towards the steadier profile of a manufacturing one.
A mature asset operator makes timely interventions through failure prediction to reduce asset downtime. Predictive maintenance incorporates sensor data and condition monitoring results in a machine-learning algorithm, which recognizes patterns associated with different failure modes on a specific machine. As no two machines are alike, the learning algorithm can customize trigger points for failures on each individual piece of equipment, thus allowing maintenance teams to plan better, reduce the incidence and severity of failures, and compress the time to recovery. The operator has reduced downtime on critical machines by as much as 30 to 50 percent.
Most significantly, predictive techniques are redefining the scope and composition of maintenance activities, enabling organizations to have smaller maintenance teams and lower operating costs. Exhibit 3 shows the expected future impact for this mature asset operator.
Predictive techniques are relevant regardless of the life stage of an asset. However, operators may choose to match upfront investment with the remaining life of their assets. While an overhaul of multiple systems into a single platform may have a positive business case at an early-life asset, a mature asset may better use an integrated platform that consolidates scattered data from legacy systems and rapidly digitizes key operational workflows.
4. How can you multiply the work hours you obtain?
Upstream operators consistently appear middle of the pack in time-in-motion studies, reporting an average of 20 to 30 percent of a shift as productive. However, world-class process-based industries and leading upstream operators can extract 7 hours of value-added work in a 12-hour shift; in some cases, particularly in campaign-based interventions, they can achieve 8 to 10 hours of useful work per shift.
Lean tools continue to be the mainstay of improving productivity. In addition, the vision for next-generation operations and maintenance is to put the employee at the core, flipping the model from ‘thinking like the manager’ to ‘thinking like the technician.’ This means that anything in the way of the technician’s doing value-added work must be minimized, or where possible, automated.
At an offshore asset, we shadowed technicians to uncover their pain points. Three pain points emerged at the top:
- A manual and substantial data reporting burden that went beyond industry compliance requirements: this trapped the offshore installation manager and supervisors at their desktops.
- A time-based schedule and planned loading approach in compliance with company maintenance execution standards : often, this imposed twice as many work orders and doubled the time per work order relative to actual execution data. While the asset was plan compliant, the maintenance teams had effective surplus capacity.
- Focus on a process rather than equipment or systems: this prompted compliance with complex process steps and reporting to relevant technical authorities over equipment care and ownership.
Addressing technician pain points along the maintenance execution process was the main lever for improving productivity. The operator reacted with three innovations:
- Digitization of key workflows had the secondary benefit of allowing most compliance data to be tracked autonomously and routed to a secure site for reporting to the parent company or regulator. This freed up offshore supervision capacity. Gradual deployment of IoT and mobile devices over the next two years was expected to provide further relief through real-time reporting.
- Time-based scheduling and plan loading was replaced with the use of actual execution data captured in digital work tracking systems. Surplus capacity in maintenance teams could be redeployed to liquidate maintenance backlogs or better utilized for standby work. The operator was beginning to implement next-generation control of work, with increased automation in integrated planning, permit-to-work processing, and work notifications.
- Process simplification liberated front-line time and capacity. Simple engineering was delegated to an offshore engineer who supervised ‘find and fix’ and accelerated simple jobs without routing them back to a central team or contractor.
- But front-line equipment care and ownership required organizational refinements. This brings us to the fifth driver of next-generation operating models.
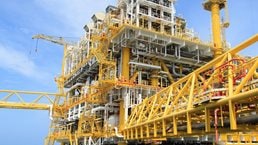
Rethinking the oil and gas organization
5. what is the minimum organization you need to achieve your business goals.
Upstream companies typically start and end reorganizations with the organization itself . Notwithstanding its limited impact on resourcing levels, this approach constrains companies’ abilities to visualize how they might adopt new technologies, such as digital tools, or introduce organizational agility, a premium functionality in our world of relentless change. 4 4. The oil and gas organization of the future; Christopher Handscomb, Scott Sharabura and Jannik Woxholth talk about five ideas that can help organisations adapt as technological and political trends reshape the industry.
Building a next-generation operations and maintenance team begins with drafting the minimum capabilities required for steady-state operations. At its most elemental, an operator takes a zero-based budgeting approach: desktop analyses and cross-functional scrums help set the size and shape of the smallest team with the skills to conduct the asset’s baseload activity set, and add incremental capacity only if there is a strong business case for it. So, while an early-life asset operator might aim for equipment familiarity through hands-on commissioning, a late-life asset operator would accommodate capacity to address integrity challenges. Even with this minimalist mindset, it is easy to rationalize why additional technicians should be on standby for unanticipated trips.
We have seen assets operating with teams less than half the prevailing norm, and specific activities, such as routine well interventions for reservoir data acquisition , run with team sizes of around 25 percent of what is typical. Three choices facilitate flexible access to the required capabilities:
- Fluid teaming. Multiskilling through a second service role, combined operations and maintenance roles or a secondary competence is more talked of than implemented. Many technicians often have broader competences than trades-based staffing models allow. In next-generation operations and maintenance teams, we go further towards an agile organizational structure, designed around equipment ownership. For instance, an equipment improvement team is cross-functional with representation from challenge areas, such as engineering, maintenance or supply chain. It is self-managing and has end-to-end accountability for the reliability of its equipment. Each team sets out with a performance target associated with its equipment and has compensation tied to the results achieved.
- Redefining skill requirements. As operators increasingly deploy digital technologies — improving work-scope predictability — unmanned operations become more feasible. An integrated remote operations centre staffed with data scientists and operations-skilled digital translators — who marshal advanced analytics models for production optimisation —i s no longer inconceivable.
- Use of innovative partnerships for non-core and peak load activities. Contracting is the traditional option for flexible access to skills. In a 21st-century organization, this might look more like a risk-sharing partnership. In a recent example, a large upstream oil and gas company established a long-term contract with two asset management contractors to increase production in a mature field. While reserves continued to be owned by the upstream company, the contractors operated under a cost recovery model with a bonus for how quickly they increased unit cash flows. Tailored alliances across the sector, with distinct contributions from participating upstream companies, can go beyond supply chain relationships. A recent merger of two operators combined the operational excellence of a leaner independent with a larger incumbent’s superior basin expertise. In the year following the transaction, the new entity nearly doubled production, providing greater financial robustness and a platform for long-term growth to both partners.
Ultimately, reorganizations must ensure access to the right talent within the asset’s business context. Organizational agility can achieve this without compromising process and personnel safety. Even with fluid teaming, the roles of the offshore installation manager or the site supervisor as safety custodian remain intact.
Achieving a continually evolving operating model will require new approaches to operational transformations, skill sets, and ways of working among the people who will make it happen. While the traditional transformation roadmap to arrive at well-defined goals is still relevant, an agile development and implementation process will be needed to accommodate greater collaboration and learning on the go. Multifunctional teams will work together on end-to-end processes to create new solutions, using shorter sprints to design minimum viable products, and being happy to fail fast as long as they learn in the process. This will put front-line teams and middle management at the heart of the transformation. And operators will have to invest in building both their belief in the value potential and their capability to deliver the required changes.
None of this will be easy, but it will be necessary if oil and gas operators are to attain the next wave of structural improvements amid the uncertainties of an ever-evolving industry.
Jayanti Kar is an associate partner in McKinsey’s London office , where Francesco Verre is an associate partner; Andy Thain is a partner in the Singapore office , and Otto van der Molen is a partner in the Amsterdam office .
Explore a career with us
Related articles.

Why oil and gas companies must act on analytics

What it takes to be successful: Three hallmarks of a healthy oil and gas organization
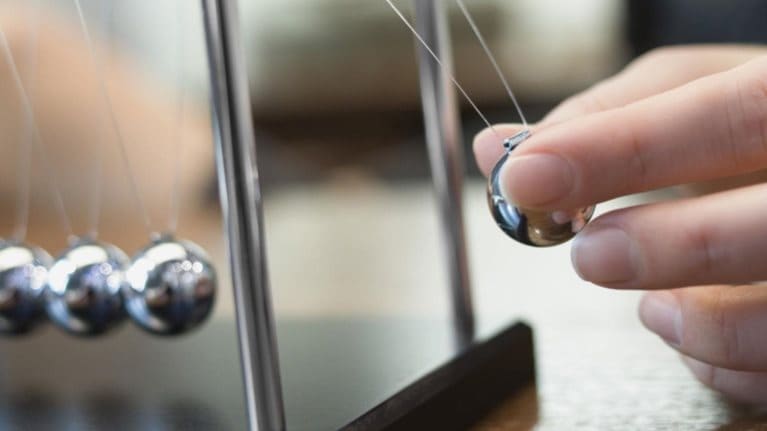
Winning the recovery: Sustainably holding down costs in an upturn
wn1sdwk000HOW
Lower 48 long-term oil and gas supply outlook: H1 2024
Get this report
You can pay by card or invoice
Submit your details to receive further information about this report.
- Submit Category
For details on how your data is used and stored, see our Privacy Notice .
- FAQs about online orders - Find out more about subscriptions
Report summary
Significant new drilling will be needed to offset base production declines and meet growing demand. By the early 2030s, the Lower 48 will add 10 million b/d of new oil production, but after accounting for declines, top-line growth will be just over 1 million b/d from current levels. While remaining oil inventory is decreasing, most top oil subplays still have runway. Soft prices are limiting near-term gas production growth, but resilient power demand and increasing LNG exports are driving long-term market expansion. Lower 48 gas production is expected to grow by 25 bcfd over the next decade. Most of this growth will come from Texas, New Mexico, and Louisiana. The recent wave of Lower 48 M&A has consolidated more production among fewer companies. As a result, corporate behaviors of the largest producers now have a greater impact on supply trajectory and response to commodity price changes.
Table of contents
- No table of contents specified
Tables and charts
No table or charts specified
What's included
This report contains:
Lower 48 Long Term Oil And Gas Supply Outlook H1 2024.pdf
PDF 823.74 KB
Lower 48 Long Term Oil And Gas Supply Outlook H1 2024.xlsx
XLSX 2.16 MB
PDF 883.20 KB
Other reports you may be interested in
Lower 48 oil and gas supply outlook h2 2023: a new paradigm.
Forecasting US Lower 48 oil and gas supply through 2033.
Romania upstream summary
A long-standing onshore producer, with future growth from Black Sea gas - Romania is a large source of oil and gas supply. The vast ...
Global oil supply short-term update - May 2024
Minor changes to the outlook as the market ponders early June OPEC+ meeting outcome
Browse reports by Industry Sector
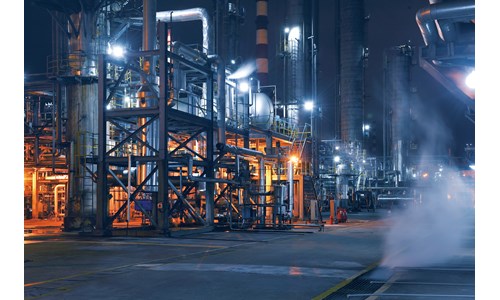
Power and renewables
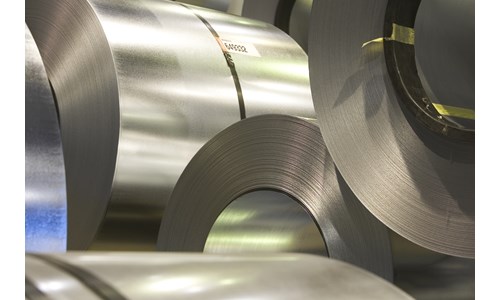
Metals markets
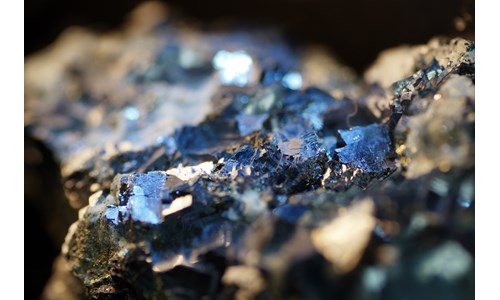
Metals costs
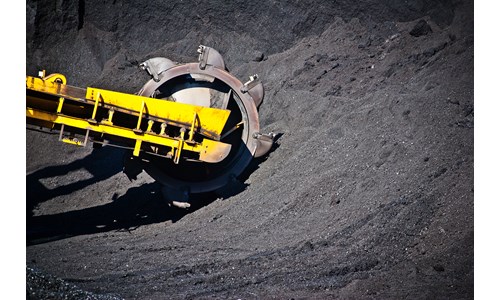
Macroeconomics, risk and global trends
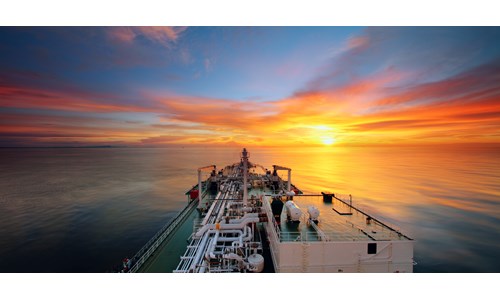
Upstream oil and gas
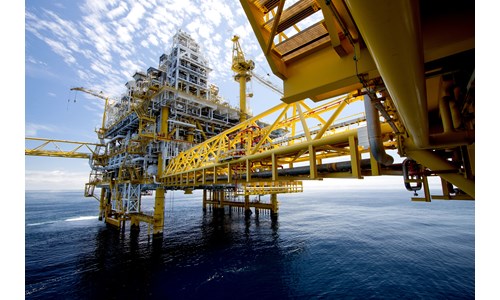
Oil and gas markets
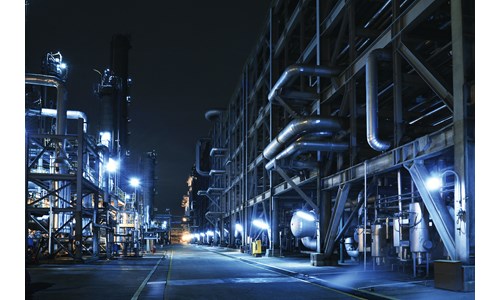
Downstream oil refining
Search our Database
Oil and gas contracts market sees 37% decline in value during q1 2024, reveals globaldata, media enquiries.
If you are a member of the press of media and require any further information, please get in touch, as we're very happy to help.
23 May, 2024 Oil and gas contracts market sees 37% decline in value during Q1 2024, reveals GlobalData
The oil and gas contracts market faced turbulence, with a notable quarter-on-quarter (QoQ) decline of 37% in disclosed contract value, dropping from $50.2 billion in Q4 2023 to $31.4 billion in Q1 2024. This decline was accompanied by a decrease in overall contract volume, reveals GlobalData , a leading data and analytics company.
GlobalData’s latest report, “ Oil and Gas Industry Contracts Review by Sector, Region, Terrain, Planned and Awarded Contracts and Top Contractors and Issuers, Q1 2024 ,” reveals that the overall contract volume decreased from 1,346 in Q4 2023 to 1,142 in Q1 2024.
Pritam Kad, Oil and Gas Analyst at GlobalData, comments : “Many traditional oil and gas industry projects are getting delayed or postponed due to concerns over demand outlook in oil and gas consuming countries amid the looming recession and high inflation, which is clearly evidenced by the decrease in both contract value and volume.”
Oil and gas contracts
Operation and Maintenance (O&M) represented 59% of the total contracts in Q1 2024, followed by procurement scope with 16%, and contracts with multiple scopes, such as construction, design and engineering, installation, O&M, and procurement accounted for 13%
Some of the notable contracts during the quarter include Samsung Heavy Industries’ $3.44 billion construction contract for 15 LNG carriers, each of 174,000 m3 capacity, and Tecnicas Reunidas and Sinopec Engineering Group’s two lumpsum contracts combined worth approximately $3.3 billion from Saudi Aramco for the Engineering, Procurement, and Construction (EPC) of the Riyas Natural Gas Liquids (NGL) fractionation facility in Saudi Arabia,
In the petrochemical sector, Tecnimont recorded approximately $1.1 billion contract from Sonatrach for the Engineering, Procurement, Construction, and Commissioning (EPCC) of a new Linear Alkyl Benzene (LAB) plant with a capacity of 100,000 tons per annum (tpa) and utilities infrastructure in east Algeria.
Kad concludes: “Contrarily, oil prices are anticipated to be favorable for producers due to potential supply disruptions arising from geopolitical risks. GlobalData expects that delayed or near completion projects are likely to be pushed forward in the mid-term.”
If you are a member of the press or media and require any further information, please get in touch, as we're very happy to help.
Related Reports
Related press releases, decoded your daily industry news round-up.

Total US Drilling Rig Tally Increases: Here's What it Means
I n its weekly release, Baker Hughes Company BKR stated that the U.S. rig count was higher than the prior week’s figure. The rotary rig count, issued by BKR, is usually published in major newspapers and trade publications.
Baker Hughes’ data, issued at the end of every week since 1944, helps energy service providers gauge the overall business environment of the oil and gas industry. The number of active rigs and its comparison with the week-ago figure indicates the demand trajectory for the company’s oilfield services from exploration and production companies.
Rig Count Data in Detail
Total U.S. Rig Count Rises: The number of rigs engaged in the exploration and production of oil and natural gas in the United States was 604 in the week ended May 17, higher than theweek-ago count of 603. The current national rig count was, however, lower than the year-ago level of 720, reflecting the fact that there has been a slowdown in drilling activities. Some analysts see this downside as a sign of increased efficiency among shale producers, who may need fewer rigs. However, there are doubts among a few about whether certain producers have sufficient promising land for drilling.
Onshore rigs in the week that ended on May 17 totaled 584, in line with the prior-week count. In offshore resources, 20 rigs were operating, which increased from the week-ago count of 19.
U.S. Oil Rig Count Rises: The oil rig count was 497 in the week ended May 17, increasing from the week-ago figure of 496. The current number of oil rigs — far from the peak of 1,609 attained in October 2014 — is, however, down from the year-ago figure of 575.
U.S. Natural Gas Rig Count Flat: The natural gas rig count of 103 was in line with the week-ago figure. The count of rigs exploring the commodity was, however, below the year-ago week’s tally of 141. Per the latest report, the number of natural gas-directed rigs is almost 93.6% lower than the all-time high of 1,606 recorded in 2008.
Rig Count by Type: The number of vertical drilling rigs totaled 18 units, higher than the week-ago count of 15 units. The horizontal/directional rig count (encompassing new drilling technology with the ability to drill and extract gas from dense rock formations, also known as shale formations) of 586 was lower than the prior-week level of 588.
Rig Count in the Most Prolific Basin
Permian — the most prolific basin in the United States — recorded a weekly oil and gas rig count of 312, lower than the week-ago figure of 314. The count is also below the prior-year level of 349.
The West Texas Intermediate crude price is trading close to the $80-per-barrel mark. Although the commodity pricing scenario is favorable for exploration and production operations, there has been a slowdown in drilling activities, which may continue as upstream players are prioritizing stockholder returns rather than boosting output.
Amid the backdrop, investors seeking medium to long-term gains may keep an eye on energy stocks like Diamondback Energy, Inc. FANG and Matador Resources Company MTDR.
Diamondback Energy, a leading pure-play Permian operator, reported ongoing enhancements in the average productivity per well in the Midland Basin. The exploration and production company expects growth in production volumes, benefiting from favorable commodity pricing conditions. FANG, carrying a Zacks Rank #3 (Hold), also has an investment-grade balance sheet. You can see the complete list of today’s Zacks #1 Rank (Strong Buy) stocks here .
Matador Resources has a strong presence in the oil-rich core acres of the Wolfcamp and Bone Spring plays in the Delaware Basin. Promising oil prices are likely to aid it in increasing production volumes. In fact, the #3 Ranked Matador expects oil production growth of 23% this year.
To read this article on Zacks.com click here.
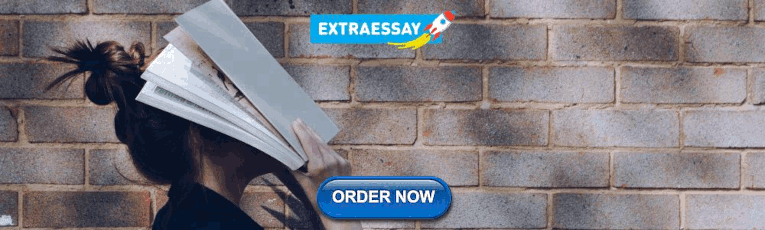
IMAGES
VIDEO
COMMENTS
Upstream Oil & Gas. The upstream segment of oil and gas industry encompasses exploration and production (E&P) activities related to exploring for, recovery and production of crude oil, natural gas, and natural gas liquids (NGLs). ADRM Software's Upstream Oil & Gas data model set consists of Enterprise, Business Area, and Data Warehouse logical ...
The PPDM Association defines and creates standards to help oil and gas companies manage exploration and production data. The members of the PPDM Association provide critical input and help to set the strategic direction for the organization. Under member direction, the PPDM Association has come to represent much more than the world's leading ...
Abstract. This paper reviews the utilization of Big Data analytics, as an emerging trend, in the upstream and downstream oil and gas industry. Big Data or Big Data analytics refers to a new technology which can be employed to handle large datasets which include six main characteristics of volume, variety, velocity, veracity, value, and complexity.
For example, HSBC has stated that "the opportunity for the digital oilfield to have a major impact across most parts of the value chain is significant.". Barclays estimates that digital could lower the cost of production by more than $3 per barrel, amounting to about $150 billion in value.
Capturing the next frontier of value: Operating models for oil and gas fields of the future 3 1 Data from the Energy Insights Global Operations Benchmark 2 Why oil and gas companies must act on analytics; Anders Brun, Monica Trench, and Thijs Vermaat describe the increase in production potential to be captured by deeper advanced analytic methods
Dr. Shahab D. Mohaghegh, a pioneer in the application of Artificial Intelligence and Machine Learning in the Exploration and Production industry, is Professor of Petroleum and Natural Gas Engineering at West Virginia University and the president and CEO of Intelligent Solutions, Inc. (ISI).He is the director of WVU-LEADS (Laboratory for Engineering Application of Data Science).
McKinsey's integrated energy demand cube. Our energy demand cube includes datasets across various dimensions: countries, industrial sectors, and energy products. 68 energy demand sectors including transport, buildings, industry, electricity generation, hydrogen production, refining, heat, and CCUS. 76 energy products including natural gas ...
Energistics provides the global upstream oil and gas industry with an open consortium to define, develop and maintain data standards. Energistics is dedicated to informing, educating and supporting all stakeholders to ensure a rapid and effective adoption of the standards in the pursuit of interoperability, efficiency and data integrity.
By Rich Hunzinger, B-Scada. The Oil and Gas Industry faces a number of new challenges in the coming years. From the obvious challenge of managing a finite resource to ever-changing environmental regulations and the consequent competitive pressures, it's easy to understand why industry leaders are continually striving to find ways to optimize and improve operational efficiency.
World oil markets are rebalancing after the Covid 19 crisis spurred an unprecedented collapse in demand in 2020, but they may never return to "normal". Oil 2021, the IEA's latest medium-term outlook, explains why. Rapid changes in behaviour from the pandemic and a stronger drive by governments towards a low-carbon future have caused a ...
Data gradually becomes a critical concern for the strategic resources in enterprises. Massive amounts of data with different types involved in the oil and gas industry can be obtained through IoT (Internet of Things) devices, supported by Blockchain technology with characteristics of immutability and credibility in distributed storage. Hence, credible data will be rationally used in Big Data ...
To quote The Economist, "The world's most valuable resource is no longer oil, but data". If data trumps oil in terms of value, then surely this raw resource deserves some more attention. [1] 2016 Upstream Oil and Gas Digital and Technology Trends Survey, sponsored by Accenture and Microsoft and conducted by PennEnergy Research <span/>.
9.1. Introduction. Oil and gas exploration and production data have outstanding big data characteristics. The industry is in the petabyte-exabyte stage in terms of the size of its big data (Boman, 2015).This is likely a result of the industry requiring large amounts of data to determine new drill sites and ensure sustainable production, among other tasks.
Examples of Data Visualization in the Oil and Gas Industry. Data visualization in the oil and gas industry can take many forms, from simple charts and graphs to complex 3D models and simulations. Here are a few examples: Seismic Analysis: Seismic surveys generate vast amounts of data that can be visualized to create a 3D model of the subsurface ...
The PPDM Association has been in the business of defining a standard data model for the Oil&Gas business since 1989. Worldwide adoption of the data model for data storage and accessibility has been attained. ... Through the above surveys and analysis of the data standards in the Oil&Gas industry, it can be seen that: at present, in order to ...
Oil and Gas Datasets. MapStand allows you to access upstream oil and gas geospatial data and gain full visibility into the oil and gas industry. View essential industry news, analysis and data focused on oil and gas exploration. Our datasets are updated several times a day from trustworthy sources. Join today Contact us.
Calculate and Discount After-Tax Cash Flows. Simply subtract the expenses from the revenue each year and then multiply by (1 - Tax Rate) to calculate the after-tax cash flows. Then, you add up and discount everything based on the standard 10% discount rate used in the Oil & Gas industry (no WACC or Cost of Equity here). 5.
The upstream integrated producing companies often organize and manage multifaceted data sources [20, 21] comprising of multiple dimensions (relational, hierarchical, and networking) with complex ...
In all, the infrastructure surveyed in this study accounts for 52% of U.S. onshore oil production and 29% of gas production. Troubling trends Emissions in three of the six regions were well above ...
G7 ministers draw on wide range of IEA recommendations to strengthen energy security and accelerate clean energy transitions. 30 April 2024. Get updates on the IEA's latest news, analysis, data and events delivered twice monthly. Subscribe. Explore our other newsletters. Read the latest IEA press releases, news and announcements highlighting ...
Here, we've highlighted the top five reads from the first half of 2024 to help you stay engaged with the industry this summer. While you relax on a beach somewhere, read or hear all about Parkland Corporation's Drive to Zero strategy, New Mexico's Ozone Precursor Rules, and more. #1 Energy Transition: An Inside Look at Parkland ...
As predictions of an early peak in oil demand take hold, upstream operators must find ways to produce more energy, more efficiently.Many have made significant performance gains in recent years. Across the sector, production costs are down 30 percent; safety incident frequency has fallen by a third; and production losses have declined by 15 percent since 2014.
By the early 2030s, the Lower 48 will add 10 million b/d of new oil production, but after accounting for declines, top-line growth will be just over 1 million b/d from current levels. While remaining oil inventory is decreasing, most top oil subplays still have runway. Soft prices are limiting near-term gas production growth, but resilient ...
The license agreement between Halliburton and Wintershall Dea for the use of the UEM solution marks a significant milestone in the oil and gas industry. By integrating static and dynamic data in ...
In this paper, a data-driven model based on multiple linear regression is developed to predict the failures of seawater pumps in the oil and gas industry. The model is developed, tested, and validated based on real data. Seawater pumps are rated as critical components, they have had a high number of failures in the last three years, which can ...
GOV.UK
The oil and gas contracts market faced turbulence, with a notable quarter-on-quarter (QoQ) decline of 37% in disclosed contract value, dropping from $50.2 billion in Q4 2023 to $31.4 billion in Q1 2024. This decline was accompanied by a decrease in overall contract volume, reveals GlobalData, a leading data and analytics company. GlobalData's ...
Rig Count Data in Detail. Total U.S. Rig Count Rises: The number of rigs engaged in the exploration and production of oil and natural gas in the United States was 604 in the week ended May 17 ...