tableau.com is not available in your region.
- Decrease Font Size
- Increase Font Size
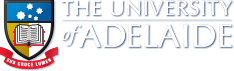
- Study At Adelaide
- Course Outlines
- Log-in
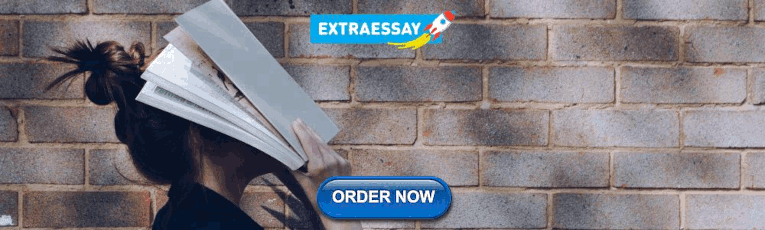
STATS 7058 - Time Series
North terrace campus - semester 2 - 2018, course details, course staff.
Course Coordinator: Professor Patricia Solomon
Course Timetable
The full timetable of all activities for this course can be accessed from Course Planner .
Course Learning Outcomes
University graduate attributes.
This course will provide students with an opportunity to develop the Graduate Attribute(s) specified below:
Required Resources
Recommended resources, online learning, learning & teaching modes.
The information below is provided as a guide to assist students in engaging appropriately with the course requirements.
Learning Activities Summary
Specific course requirements, assessment summary, assessment related requirements, assessment detail, course grading.
Grades for your performance in this course will be awarded in accordance with the following scheme:
Further details of the grades/results can be obtained from Examinations .
Grade Descriptors are available which provide a general guide to the standard of work that is expected at each grade level. More information at Assessment for Coursework Programs .
Final results for this course will be made available through Access Adelaide .
The University places a high priority on approaches to learning and teaching that enhance the student experience. Feedback is sought from students in a variety of ways including on-going engagement with staff, the use of online discussion boards and the use of Student Experience of Learning and Teaching (SELT) surveys as well as GOS surveys and Program reviews.
SELTs are an important source of information to inform individual teaching practice, decisions about teaching duties, and course and program curriculum design. They enable the University to assess how effectively its learning environments and teaching practices facilitate student engagement and learning outcomes. Under the current SELT Policy (http://www.adelaide.edu.au/policies/101/) course SELTs are mandated and must be conducted at the conclusion of each term/semester/trimester for every course offering. Feedback on issues raised through course SELT surveys is made available to enrolled students through various resources (e.g. MyUni). In addition aggregated course SELT data is available.
- Academic Integrity for Students
- Academic Support with Maths
- Academic Support with writing and study skills
- Careers Services
- International Student Support
- Library Services for Students
- LinkedIn Learning
- Student Life Counselling Support - Personal counselling for issues affecting study
- Students with a Disability - Alternative academic arrangements
- YouX Student Care - Advocacy, confidential counselling, welfare support and advice
This section contains links to relevant assessment-related policies and guidelines - all university policies .
- Academic Credit Arrangements Policy
- Academic Integrity Policy
- Academic Progress by Coursework Students Policy
- Assessment for Coursework Programs Policy
- Copyright Compliance Policy
- Coursework Academic Programs Policy
- Elder Conservatorium of Music Noise Management Plan
- Intellectual Property Policy
- IT Acceptable Use and Security Policy
- Modified Arrangements for Coursework Assessment Policy
- Reasonable Adjustments to Learning, Teaching & Assessment for Students with a Disability Policy
- Student Experience of Learning and Teaching Policy
- Student Grievance Resolution Process
Students are reminded that in order to maintain the academic integrity of all programs and courses, the university has a zero-tolerance approach to students offering money or significant value goods or services to any staff member who is involved in their teaching or assessment. Students offering lecturers or tutors or professional staff anything more than a small token of appreciation is totally unacceptable, in any circumstances. Staff members are obliged to report all such incidents to their supervisor/manager, who will refer them for action under the university's student’s disciplinary procedures.
The University of Adelaide is committed to regular reviews of the courses and programs it offers to students. The University of Adelaide therefore reserves the right to discontinue or vary programs and courses without notice. Please read the important information contained in the disclaimer .

- Copyright & Disclaimer
- Privacy Statement
- Freedom of Information
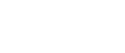
Information For
- Future Students
- International Students
- New Students
- Current Students
- Current Staff
- Future Staff
- Industry & Government
Information About
- The University
- Study at Adelaide
- Degrees & Courses
- Work at Adelaide
- Research at Adelaide
- Indigenous Education
- Learning & Teaching
- Giving to Adelaide
People & Places
- Faculties & Divisions
- Campuses & Maps
- Staff Directory
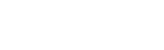
The University of Adelaide Adelaide , South Australia 5005 Australia Australian University Provider Number PRV12105 CRICOS Provider Number 00123M
Telephone: +61 8 8313 4455
Coordinates: -34.920843 , 138.604513
Browse Course Material
Course info.
- Prof. Anna Mikusheva
Departments
As taught in.
- Probability and Statistics
- Econometrics
- Macroeconomics
Learning Resource Types
Time series analysis, assignments and exams.
The assignments and exam from the course are not available, however some sample problems and a sample final exam have been included.
Problem Sets
The problem sets will emphasize different aspects of the course, including theory and estimation procedures we discuss in class. I strongly believe that the best way to learn the techniques is by doing. Every problem set will include an applied task that may include computer programming. I do not restrict you in your choice of computer language. I also do not require you to write all programs by yourself from scratch. You may use user-written parts of codes you find on the Internet, but I do require that you understand the program you use and properly document it with all needed citations of original sources. Collaboration with other students on problem sets is encouraged, however, the problem sets should be written independently.
Samples problems for 14.384 (PDF)
Sample final exam (PDF)

You are leaving MIT OpenCourseWare
Time Series Analysis Explained
Time series analysis is a powerful statistical method that examines data points collected at regular intervals to uncover underlying patterns and trends. This technique is highly relevant across various industries, as it enables informed decision making and accurate forecasting based on historical data. By understanding the past and predicting the future, time series analysis plays a crucial role in fields such as finance, health care, energy, supply chain management, weather forecasting, marketing, and beyond. In this guide, we will dive into the details of what time series analysis is, why it’s used, the value it creates, how it’s structured, and the important base concepts to learn in order to understand the practice of using time series in your data analytics practice.
Table of Contents
- What Is Time Series Analysis?
- Why Do Organizations Use Time Series Analysis?
- Components of Time Series Data
Types of Data
- Important Time Series Terms and Concepts
Time Series Analysis Techniques
- Advantages of Time Series Analysis
- Challenges of Time Series Analysis
- The Future of Time Series Analysis.
What Is Time Series Analysis?
Time series analysis is indispensable in data science, statistics, and analytics.
At its core, time series analysis focuses on studying and interpreting a sequence of data points recorded or collected at consistent time intervals. Unlike cross-sectional data, which captures a snapshot in time, time series data is fundamentally dynamic, evolving over chronological sequences both short and extremely long. This type of analysis is pivotal in uncovering underlying structures within the data, such as trends, cycles, and seasonal variations.
Technically, time series analysis seeks to model the inherent structures within the data, accounting for phenomena like autocorrelation, seasonal patterns, and trends. The order of data points is crucial; rearranging them could lose meaningful insights or distort interpretations. Furthermore, time series analysis often requires a substantial dataset to maintain the statistical significance of the findings. This enables analysts to filter out 'noise,' ensuring that observed patterns are not mere outliers but statistically significant trends or cycles.
To delve deeper into the subject, you must distinguish between time-series data, time-series forecasting, and time-series analysis. Time-series data refers to the raw sequence of observations indexed in time order. On the other hand, time-series forecasting uses historical data to make future projections, often employing statistical models like ARIMA (AutoRegressive Integrated Moving Average). But Time series analysis, the overarching practice, systematically studies this data to identify and model its internal structures, including seasonality, trends, and cycles. What sets time series apart is its time-dependent nature, the requirement for a sufficiently large sample size for accurate analysis, and its unique capacity to highlight cause-effect relationships that evolve.
Why Do Organizations Use Time Series Analysis?
Time series analysis has become a crucial tool for companies looking to make better decisions based on data. By studying patterns over time, organizations can understand past performance and predict future outcomes in a relevant and actionable way. Time series helps turn raw data into insights companies can use to improve performance and track historical outcomes.
For example, retailers might look at seasonal sales patterns to adapt their inventory and marketing. Energy companies could use consumption trends to optimize their production schedule. The applications even extend to detecting anomalies—like a sudden drop in website traffic—that reveal deeper issues or opportunities. Financial firms use it to respond to stock market shifts instantly. And health care systems need it to assess patient risk in the moment.
Rather than a series of stats, time series helps tell a story about evolving business conditions over time. It's a dynamic perspective that allows companies to plan proactively, detect issues early, and capitalize on emerging opportunities.
Components of Time Series Data
Time series data is generally comprised of different components that characterize the patterns and behavior of the data over time. By analyzing these components, we can better understand the dynamics of the time series and create more accurate models. Four main elements make up a time series dataset:
- Seasonality
Trends show the general direction of the data, and whether it is increasing, decreasing, or remaining stationary over an extended period of time. Trends indicate the long-term movement in the data and can reveal overall growth or decline. For example, e-commerce sales may show an upward trend over the last five years.
Seasonality refers to predictable patterns that recur regularly, like yearly retail spikes during the holiday season. Seasonal components exhibit fluctuations fixed in timing, direction, and magnitude. For instance, electricity usage may surge every summer as people turn on their air conditioners.
Cycles demonstrate fluctuations that do not have a fixed period, such as economic expansions and recessions. These longer-term patterns last longer than a year and do not have consistent amplitudes or durations. Business cycles that oscillate between growth and decline are an example.
Finally, noise encompasses the residual variability in the data that the other components cannot explain. Noise includes unpredictable, erratic deviations after accounting for trends, seasonality, and cycles.
In summary, the key components of time series data are:
- Trends: Long-term increases, decreases, or stationary movement
- Seasonality: Predictable patterns at fixed intervals
- Cycles: Fluctuations without a consistent period
- Noise: Residual unexplained variability
Understanding how these elements interact allows for deeper insight into the dynamics of time series data.
When embarking on time series analysis, the first step is often understanding the type of data you're working with. This categorization primarily falls into three distinct types: Time Series Data, Cross-Sectional Data, and Pooled Data. Each type has unique features that guide the subsequent analysis and modeling.
- Time Series Data: Comprises observations collected at different time intervals. It's geared towards analyzing trends, cycles, and other temporal patterns.
- Cross-Sectional Data: Involves data points collected at a single moment in time. Useful for understanding relationships or comparisons between different entities or categories at that specific point.
- Pooled Data: A combination of Time Series and Cross-Sectional data. This hybrid enriches the dataset, allowing for more nuanced and comprehensive analyses.
Understanding these data types is crucial for appropriately tailoring your analytical approach, as each comes with its own set of assumptions and potential limitations.
Important Time Series Terms & Concepts
Time series analysis is a specialized branch of statistics focused on studying data points collected or recorded sequentially over time. It incorporates various techniques and methodologies to identify patterns, forecast future data points, and make informed decisions based on temporal relationships among variables. This form of analysis employs an array of terms and concepts that help in the dissection and interpretation of time-dependent data.
- Dependence : The relationship between two observations of the same variable at different periods is crucial for understanding temporal associations.
- Stationarity : A property where the statistical characteristics like mean and variance are constant over time; often a prerequisite for various statistical models.
- Differencing : A transformation technique to turn stationary into non-stationary time series data by subtracting consecutive or lagged values.
- Specification : The process of choosing an appropriate analytical model for time series analysis could involve selection criteria, such as the type of curve or the degree of differencing.
- Exponential Smoothing : A forecasting method that uses a weighted average of past observations, prioritizing more recent data points for making short-term predictions.
- Curve Fitting : The use of mathematical functions to best fit a set of data points, often employed for non-linear relationships in the data.
- ARIMA (Auto Regressive Integrated Moving Average) : A widely-used statistical model for analyzing and forecasting time series data, encompassing aspects like auto-regression, integration (differencing), and moving average.
Time series analysis is critical for businesses to predict future outcomes, assess past performances, or identify underlying patterns and trends in various metrics. Time series analysis can offer valuable insights into stock prices, sales figures, customer behavior, and other time-dependent variables. By leveraging these techniques, businesses can make informed decisions, optimize operations, and enhance long-term strategies.
Time series analysis offers a multitude of benefits to businesses.The applications are also wide-ranging, whether it's in forecasting sales to manage inventory better, identifying the seasonality in consumer behavior to plan marketing campaigns, or even analyzing financial markets for investment strategies. Different techniques serve distinct purposes and offer varied granularity and accuracy, making it vital for businesses to understand the methods that best suit their specific needs.
- Moving Average : Useful for smoothing out long-term trends. It is ideal for removing noise and identifying the general direction in which values are moving.
- Exponential Smoothing : Suited for univariate data with a systematic trend or seasonal component. Assigns higher weight to recent observations, allowing for more dynamic adjustments.
- Autoregression : Leverages past observations as inputs for a regression equation to predict future values. It is good for short-term forecasting when past data is a good indicator.
- Decomposition : This breaks down a time series into its core components—trend, seasonality, and residuals—to enhance the understanding and forecast accuracy.
- Time Series Clustering : Unsupervised method to categorize data points based on similarity, aiding in identifying archetypes or trends in sequential data.
- Wavelet Analysis : Effective for analyzing non-stationary time series data. It helps in identifying patterns across various scales or resolutions.
- Intervention Analysis : Assesses the impact of external events on a time series, such as the effect of a policy change or a marketing campaign.
- Box-Jenkins ARIMA models : Focuses on using past behavior and errors to model time series data. Assumes data can be characterized by a linear function of its past values.
- Box-Jenkins Multivariate models : Similar to ARIMA, but accounts for multiple variables. Useful when other variables influence one time series.
- Holt-Winters Exponential Smoothing : Best for data with a distinct trend and seasonality. Incorporates weighted averages and builds upon the equations for exponential smoothing.
The Advantages of Time Series Analysis
Time series analysis is a powerful tool for data analysts that offers a variety of advantages for both businesses and researchers. Its strengths include:
- Data Cleansing : Time series analysis techniques such as smoothing and seasonality adjustments help remove noise and outliers, making the data more reliable and interpretable.
- Understanding Data : Models like ARIMA or exponential smoothing provide insight into the data's underlying structure. Autocorrelations and stationarity measures can help understand the data's true nature.
- Forecasting : One of the primary uses of time series analysis is to predict future values based on historical data. Forecasting is invaluable for business planning, stock market analysis, and other applications.
- Identifying Trends and Seasonality : Time series analysis can uncover underlying patterns, trends, and seasonality in data that might not be apparent through simple observation.
- Visualizations : Through time series decomposition and other techniques, it's possible to create meaningful visualizations that clearly show trends, cycles, and irregularities in the data.
- Efficiency : With time series analysis, less data can sometimes be more. Focusing on critical metrics and periods can often derive valuable insights without getting bogged down in overly complex models or datasets.
- Risk Assessment : Volatility and other risk factors can be modeled over time, aiding financial and operational decision-making processes.
Challenges of Time Series Analysis
While time series analysis has a lot to offer, it also comes with its own set of limitations and challenges, such as:
- Limited Scope : Time series analysis is restricted to time-dependent data. It's not suitable for cross-sectional or purely categorical data.
- Noise Introduction : Techniques like differencing can introduce additional noise into the data, which may obscure fundamental patterns or trends.
- Interpretation Challenges : Some transformed or differenced values may need more intuitive meaning, making it easier to understand the real-world implications of the results.
- Generalization Issues : Results may only sometimes be generalizable, primarily when the analysis is based on a single, isolated dataset or period.
- Model Complexity : The choice of model can greatly influence the results, and selecting an inappropriate model can lead to unreliable or misleading conclusions.
- Non-Independence of Data : Unlike other types of statistical analysis, time series data points are not always independent, which can introduce bias or error in the analysis.
- Data Availability : Time series analysis often requires many data points for reliable results, and such data may not always be easily accessible or available.
The Future of Time Series Analysis
The future of time series analysis will likely see significant advances thanks to innovations in machine learning and artificial intelligence. These technologies will enable more sophisticated and accurate forecasting models while also improving how we handle real-world complexities like missing data and sparse datasets.
Some key developments are likely to include:
- Hybrid models strategically combine multiple techniques —such as ARIMA, exponential smoothing, deep learning LSTM networks, and Fourier transforms—to capitalize on their respective strengths. Blending approaches in this way can produce more robust and precise forecasts.
- Advanced deep learning algorithms like LSTM recurrent neural networks can uncover subtle patterns and interdependencies in time series data. LSTMs excel at sequence modeling and time series forecasting tasks.
- Real-time analysis and monitoring using predictive analytics and anomaly detection over streaming data. Real-time analytics will become indispensable for time-critical monitoring and decision-making applications as computational speeds increase.
- Automated time series model selection using hyperparameter tuning, Bayesian methods, genetic algorithms, and other techniques to systematically determine the optimal model specifications and parameters for a given dataset and context. This relieves analysts of much tedious trial-and-error testing.
- State-of-the-art missing data imputation, cleaning, and preprocessing techniques to overcome data quality issues: For example, advanced interpolation, Kalman filtering, and robust statistical methods can minimize distortions caused by gaps, noise, outliers, and irregular intervals in time series data.
In summary, we can expect major leaps in time series forecasting accuracy, efficiency, and applicability as modern AI and data processing innovations integrate into standard applied analytics practice. The future is bright for leveraging these technologies to extract valuable insights from time series data.
Related Blogs
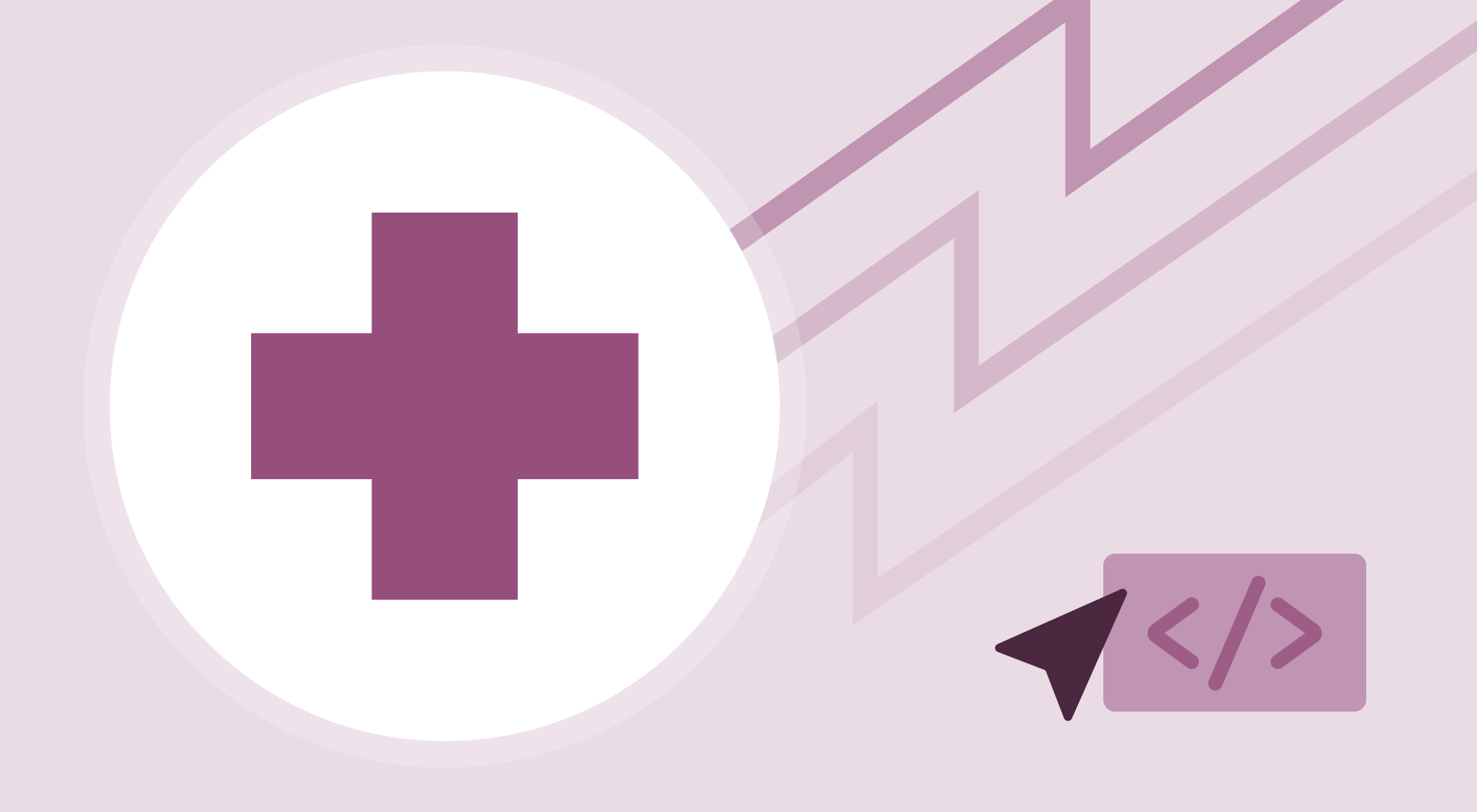
The Power of Embedded Analytics in Healthcare
Sigma provides the comfort of a spreadsheet interface and simple access to data without requiring knowledge of programming languages. The power of Sigma can be brought directly in front of healthcare professionals in the software that they already use daily.
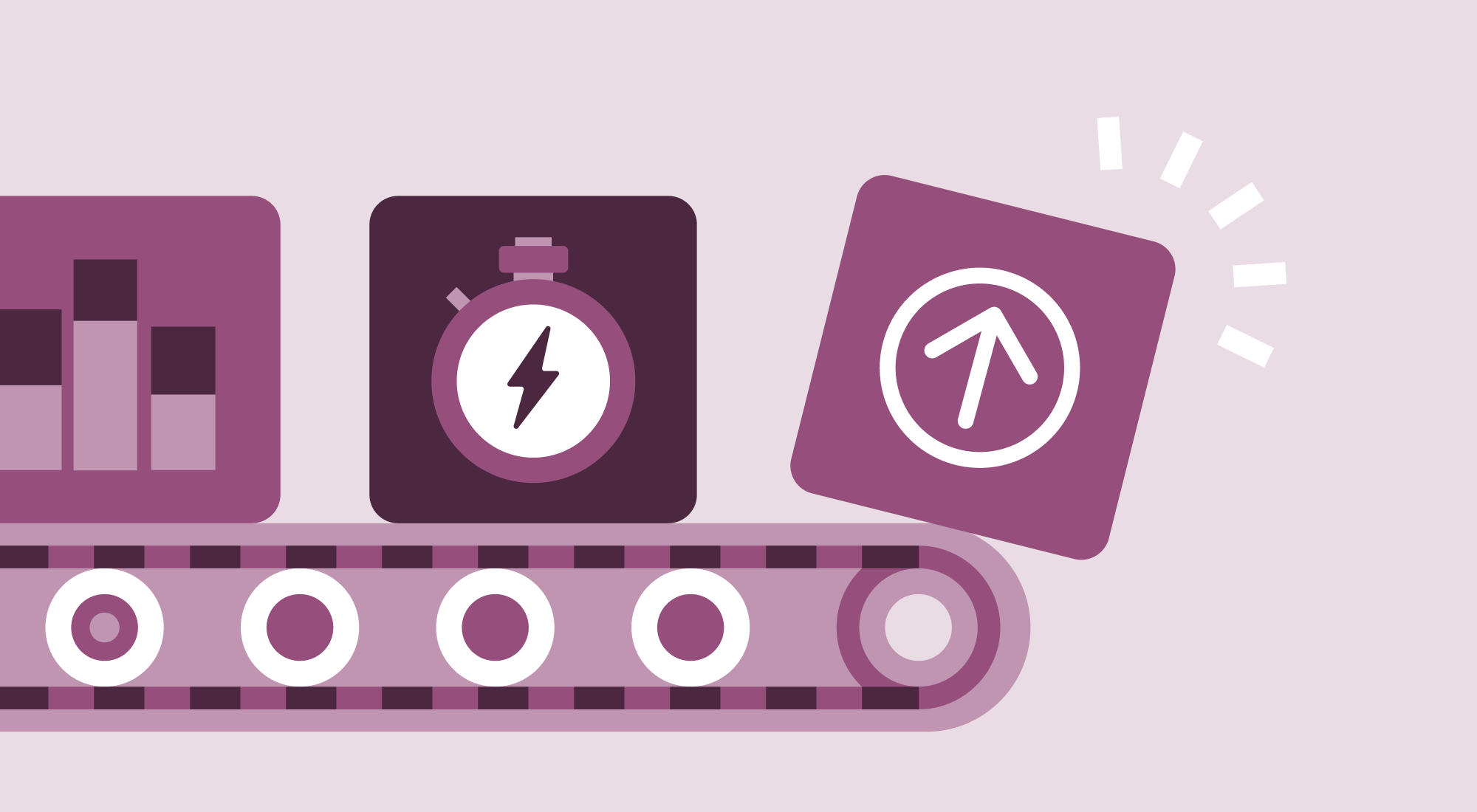
Sigma Embed Analytics to Simplify Supply Chain Management
Businesses that have instant access to data are able to make better, smarter decisions and stand out from the competition. The world’s most profitable manufacturers utilize analytics to make judgments based on factual data from past performance and current trends to maximize profits and boost productivity.
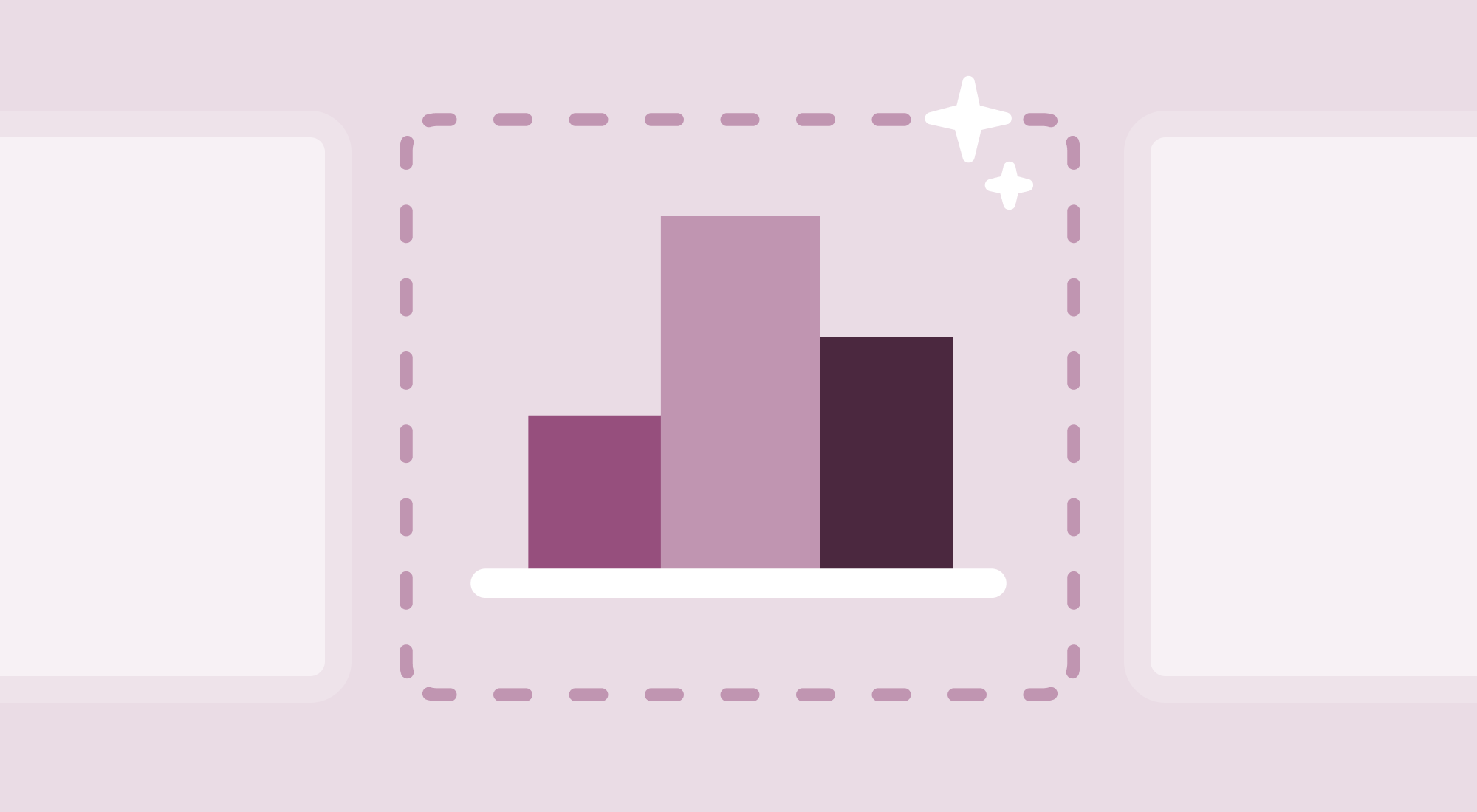
Enhancing Marketing and Social Media with Embedded Analytics
The main objective of digital marketing is to connect with your audience and potential customers online, where they spend the most time, but it's no secret the sheer amount of data collected via social media is daunting. Although Marketers often want to collect and measure consumer data spread across multiple digital platforms to continually refine and perfect their advertising spend, many data platforms struggle to keep up at scale.
- Time Series Analysis 2
- by Roy Wong
- Last updated almost 4 years ago
- Hide Comments (–) Share Hide Toolbars
Twitter Facebook Google+
Or copy & paste this link into an email or IM:
- Python for Machine Learning
- Machine Learning with R
- Machine Learning Algorithms
- Math for Machine Learning
- Machine Learning Interview Questions
- ML Projects
- Deep Learning
- Computer vision
- Data Science
- Artificial Intelligence
Time Series Analysis and Forecasting
- Univariate Time Series Analysis and Forecasting
- Time Series and Forecasting Using R
- Anomaly Detection in Time Series Data
- Autoregressive (AR) Model for Time Series Forecasting
- Time Series Analysis using Facebook Prophet
- Python | ARIMA Model for Time Series Forecasting
- Time Series Forecasting using Pytorch
- TIme Series Forecasting using TensorFlow
- Time Series Analysis & Visualization in Python
- 8 Types of Plots for Time Series Analysis using Python
- Time Series Analysis in R
- Signal Processing and Time Series (Data Analysis)
- Time Series Analysis using ARIMA model in R Programming
- Anomaly Detection in Time Series in R
- Python | Pandas Series.at_time()
- Time Series Analysis using Facebook Prophet in R Programming
- Financial Analysis: Need, Types, and Limitations
- Manipulating Time Series Data in Python
- Python | Math operations for Data analysis
Time series analysis and forecasting are crucial for predicting future trends, behaviors, and behaviours based on historical data. It helps businesses make informed decisions, optimize resources, and mitigate risks by anticipating market demand, sales fluctuations, stock prices, and more. Additionally, it aids in planning, budgeting, and strategizing across various domains such as finance, economics, healthcare, climate science, and resource management, driving efficiency and competitiveness.
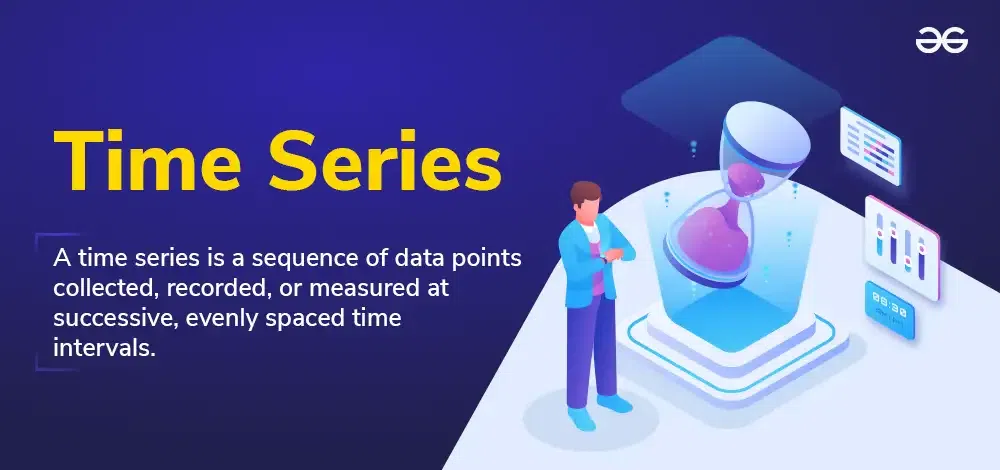
- What is a Time Series?
A time series is a sequence of data points collected, recorded, or measured at successive, evenly-spaced time intervals.
Each data point represents observations or measurements taken over time, such as stock prices, temperature readings, or sales figures. Time series data is commonly represented graphically with time on the horizontal axis and the variable of interest on the vertical axis, allowing analysts to identify trends, patterns, and changes over time.
Time series data is often represented graphically as a line plot, with time depicted on the horizontal x-axis and the variable’s values displayed on the vertical y-axis. This graphical representation facilitates the visualization of trends, patterns, and fluctuations in the variable over time, aiding in the analysis and interpretation of the data.
Table of Content
Components of Time Series Data
Time Series Visualization
Preprocessing time series data, time series analysis & decomposition, what is time series forecasting, evaluating time series forecasts, top python libraries for time series analysis & forecasting, frequently asked questions on time series analysis, importance of time series analysis.
- Predict Future Trends: Time series analysis enables the prediction of future trends, allowing businesses to anticipate market demand, stock prices, and other key variables, facilitating proactive decision-making.
- Detect Patterns and Anomalies: By examining sequential data points, time series analysis helps detect recurring patterns and anomalies, providing insights into underlying behaviors and potential outliers.
- Risk Mitigation: By spotting potential risks, businesses can develop strategies to mitigate them, enhancing overall risk management.
- Strategic Planning: Time series insights inform long-term strategic planning, guiding decision-making across finance, healthcare, and other sectors.
- Competitive Edge: Time series analysis enables businesses to optimize resource allocation effectively, whether it’s inventory, workforce, or financial assets. By staying ahead of market trends, responding to changes, and making data-driven decisions, businesses gain a competitive edge.
There are four main components of a time series :
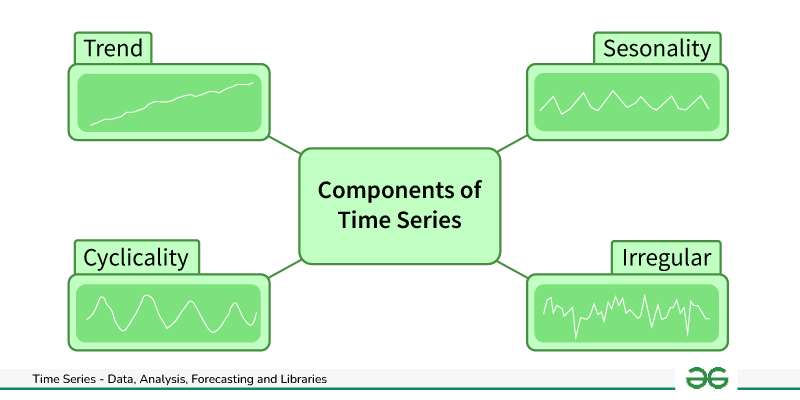
- Trend : Trend represents the long-term movement or directionality of the data over time. It captures the overall tendency of the series to increase, decrease, or remain stable. Trends can be linear, indicating a consistent increase or decrease, or nonlinear, showing more complex patterns.
- Seasonality : Seasonality refers to periodic fluctuations or patterns that occur at regular intervals within the time series. These cycles often repeat annually, quarterly, monthly, or weekly and are typically influenced by factors such as seasons, holidays, or business cycles.
- Cyclic variations: Cyclical variations are longer-term fluctuations in the time series that do not have a fixed period like seasonality. These fluctuations represent economic or business cycles, which can extend over multiple years and are often associated with expansions and contractions in economic activity.
- Irregularity (or Noise): Irregularity, also known as noise or randomness, refers to the unpredictable or random fluctuations in the data that cannot be attributed to the trend, seasonality, or cyclical variations. These fluctuations may result from random events, measurement errors, or other unforeseen factors. Irregularity makes it challenging to identify and model the underlying patterns in the time series data.
Time series visualization is the graphical representation of data collected over successive time intervals. It encompasses various techniques such as line plots, seasonal subseries plots, autocorrelation plots, histograms, and interactive visualizations. These methods help analysts identify trends, patterns, and anomalies in time-dependent data for better understanding and decision-making.
Different Time series visualization graphs
- Line Plots: Line plots display data points over time, allowing easy observation of trends, cycles, and fluctuations.
- Seasonal Plots: These plots break down time series data into seasonal components, helping to visualize patterns within specific time periods.
- Histograms and Density Plots: Shows the distribution of data values over time, providing insights into data characteristics such as skewness and kurtosis.
- Autocorrelation and Partial Autocorrelation Plots: These plots visualize correlation between a time series and its lagged values, helping to identify seasonality and lagged relationships.
- Spectral Analysis: Spectral analysis techniques, such as periodograms and spectrograms, visualize frequency components within time series data, useful for identifying periodicity and cyclical patterns.
- Decomposition Plots: Decomposition plots break down a time series into its trend, seasonal, and residual components, aiding in understanding the underlying patterns.
These visualization techniques allow analysts to explore, interpret, and communicate insights from time series data effectively, supporting informed decision-making and forecasting.
Time Series Visualization Techniques: Python and R Implementations
Time series preprocessing refers to the steps taken to clean, transform, and prepare time series data for analysis or forecasting. It involves techniques aimed at improving data quality, removing noise, handling missing values, and making the data suitable for modeling. Preprocessing tasks may include removing outliers, handling missing values through imputation, scaling or normalizing the data, detrending, deseasonalizing, and applying transformations to stabilize variance. The goal is to ensure that the time series data is in a suitable format for subsequent analysis or modeling.
- Handling Missing Values : Dealing with missing values in the time series data to ensure continuity and reliability in analysis.
- Dealing with Outliers: Identifying and addressing observations that significantly deviate from the rest of the data, which can distort analysis results.
- Stationarity and Transformation: Ensuring that the statistical properties of the time series, such as mean and variance, remain constant over time. Techniques like differencing, detrending, and deseasonalizing are used to achieve stationarity.
Time Series Preprocessing Techniques: Python and R Implementations
Time Series Analysis and Decomposition is a systematic approach to studying sequential data collected over successive time intervals. It involves analyzing the data to understand its underlying patterns, trends, and seasonal variations, as well as decomposing the time series into its fundamental components. This decomposition typically includes identifying and isolating elements such as trend, seasonality, and residual (error) components within the data.
Different Time Series Analysis & Decomposition Techniques
- Autocorrelation Analysis: A statistical method to measure the correlation between a time series and a lagged version of itself at different time lags. It helps identify patterns and dependencies within the time series data.
- Partial Autocorrelation Functions (PACF) : PACF measures the correlation between a time series and its lagged values, controlling for intermediate lags, aiding in identifying direct relationships between variables.
- Trend Analysis: The process of identifying and analyzing the long-term movement or directionality of a time series. Trends can be linear, exponential, or nonlinear and are crucial for understanding underlying patterns and making forecasts.
- Seasonality Analysis: Seasonality refers to periodic fluctuations or patterns that occur in a time series at fixed intervals, such as daily, weekly, or yearly. Seasonality analysis involves identifying and quantifying these recurring patterns to understand their impact on the data.
- Decomposition: Decomposition separates a time series into its constituent components, typically trend, seasonality, and residual (error). This technique helps isolate and analyze each component individually, making it easier to understand and model the underlying patterns.
- Spectrum Analysis: Spectrum analysis involves examining the frequency domain representation of a time series to identify dominant frequencies or periodicities. It helps detect cyclic patterns and understand the underlying periodic behavior of the data.
- Seasonal and Trend decomposition using Loess : STL decomposes a time series into three components: seasonal, trend, and residual. This decomposition enables modeling and forecasting each component separately, simplifying the forecasting process.
- Rolling Correlation: Rolling correlation calculates the correlation coefficient between two time series over a rolling window of observations, capturing changes in the relationship between variables over time.
- Cross-correlation Analysis: Cross-correlation analysis measures the similarity between two time series by computing their correlation at different time lags. It is used to identify relationships and dependencies between different variables or time series.
- Box-Jenkins Method: Box-Jenkins Method is a systematic approach for analyzing and modeling time series data. It involves identifying the appropriate autoregressive integrated moving average (ARIMA) model parameters, estimating the model, diagnosing its adequacy through residual analysis, and selecting the best-fitting model.
- Granger Causality Analysis: Granger causality analysis determines whether one time series can predict future values of another time series. It helps infer causal relationships between variables in time series data, providing insights into the direction of influence.
Time Series Analysis & Decomposition Techniques: Python and R Implementations
Time Series Forecasting is a statistical technique used to predict future values of a time series based on past observations. In simpler terms, it’s like looking into the future of data points plotted over time. By analyzing patterns and trends in historical data, Time Series Forecasting helps make informed predictions about what may happen next, assisting in decision-making and planning for the future.
Different Time Series Forecasting Algorithms
- Autoregressive (AR) Model : Autoregressive (AR) model is a type of time series model that predicts future values based on linear combinations of past values of the same time series. In an AR(p) model, the current value of the time series is modeled as a linear function of its previous p values, plus a random error term. The order of the autoregressive model (p) determines how many past values are used in the prediction.
- Autoregressive Integrated Moving Average (ARIMA): ARIMA is a widely used statistical method for time series forecasting. It models the next value in a time series based on linear combination of its own past values and past forecast errors. The model parameters include the order of autoregression (p), differencing (d), and moving average (q).
- ARIMAX : ARIMA model extended to include exogenous variables that can improve forecast accuracy.
- Seasonal Autoregressive Integrated Moving Average (SARIMA) : SARIMA extends ARIMA by incorporating seasonality into the model. It includes additional seasonal parameters (P, D, Q) to capture periodic fluctuations in the data.
- SARIMAX : Extension of SARIMA that incorporates exogenous variables for seasonal time series forecasting.
- Vector Autoregression (VAR) Models: VAR models extend autoregression to multivariate time series data by modeling each variable as a linear combination of its past values and the past values of other variables. They are suitable for analyzing and forecasting interdependencies among multiple time series.
- Theta Method : A simple and intuitive forecasting technique based on extrapolation and trend fitting.
- Exponential Smoothing Methods: Exponential smoothing methods, such as Simple Exponential Smoothing (SES) and Holt-Winters, forecast future values by exponentially decreasing weights for past observations. These methods are particularly useful for data with trend and seasonality.
- Gaussian Processes Regression: Gaussian Processes Regression is a Bayesian non-parametric approach that models the distribution of functions over time. It provides uncertainty estimates along with point forecasts, making it useful for capturing uncertainty in time series forecasting.
- Generalized Additive Models (GAM): A flexible modeling approach that combines additive components, allowing for nonlinear relationships and interactions.
- Random Forests: Random Forests is a machine learning ensemble method that constructs multiple decision trees during training and outputs the average prediction of the individual trees. It can handle complex relationships and interactions in the data, making it effective for time series forecasting.
- Gradient Boosting Machines (GBM): GBM is another ensemble learning technique that builds multiple decision trees sequentially, where each tree corrects the errors of the previous one. It excels in capturing nonlinear relationships and is robust against overfitting.
- State Space Models: State space models represent a time series as a combination of unobserved (hidden) states and observed measurements. These models capture both the deterministic and stochastic components of the time series, making them suitable for forecasting and anomaly detection.
- Dynamic Linear Models (DLMs): DLMs are Bayesian state-space models that represent time series data as a combination of latent state variables and observations. They are flexible models capable of incorporating various trends, seasonality, and other dynamic patterns in the data.
- Recurrent Neural Networks (RNNs) and Long Short-Term Memory (LSTM) Networks: RNNs and LSTMs are deep learning architectures designed to handle sequential data. They can capture complex temporal dependencies in time series data, making them powerful tools for forecasting tasks, especially when dealing with large-scale and high-dimensional data.
- Hidden Markov Model (HMM): A Hidden Markov Model (HMM) is a statistical model used to describe sequences of observable events generated by underlying hidden states. In time series, HMMs infer hidden states from observed data, capturing dependencies and transitions between states. They are valuable for tasks like speech recognition, gesture analysis, and anomaly detection, providing a framework to model complex sequential data and extract meaningful patterns from it.
Time Series Forecasting Algorithms: Python and R Implementations
Evaluating Time Series Forecasts involves assessing the accuracy and effectiveness of predictions made by time series forecasting models. This process aims to measure how well a model performs in predicting future values based on historical data. By evaluating forecasts, analysts can determine the reliability of the models, identify areas for improvement, and make informed decisions about their use in practical applications.
Performance Metrics:
Performance metrics are quantitative measures used to evaluate the accuracy and effectiveness of time series forecasts. These metrics provide insights into how well a forecasting model performs in predicting future values based on historical data. Common performance metrics which can be used for time series include:
- Mean Absolute Error (MAE) : Measures the average magnitude of errors between predicted and actual values.
- Mean Absolute Percentage Error (MAPE) : Calculates the average percentage difference between predicted and actual values.
- Mean Squared Error (MSE) : Computes the average squared differences between predicted and actual values.
- Root Mean Squared Error (RMSE) : The square root of MSE, providing a measure of the typical magnitude of errors.
- Forecast Bias: Determines whether forecasts systematically overestimate or underestimate actual values.
- Forecast Interval Coverage : Evaluates the percentage of actual values that fall within forecast intervals.
- Theil’s U Statistic: Compares the performance of the forecast model to a naïve benchmark model.
Cross-Validation Techniques
Cross-validation techniques are used to assess the generalization performance of time series forecasting models. These techniques involve splitting the available data into training and testing sets, fitting the model on the training data, and evaluating its performance on the unseen testing data. Common cross-validation techniques for time series data include:
- Train-Test Split for Time Series: Divides the dataset into a training set for model fitting and a separate testing set for evaluation.
- Rolling Window Validation: Uses a moving window approach to iteratively train and test the model on different subsets of the data.
- Time Series Cross-Validation : Splits the time series data into multiple folds, ensuring that each fold maintains the temporal order of observations.
- Walk-Forward Validation: Similar to rolling window validation but updates the training set with each new observation, allowing the model to adapt to changing data patterns.
Python Libraries for Time Series Analysis & Forecasting encompass a suite of powerful tools and frameworks designed to facilitate the analysis and forecasting of time series data. These libraries offer a diverse range of capabilities, including statistical modeling, machine learning algorithms, deep learning techniques, and probabilistic forecasting methods. With their user-friendly interfaces and extensive documentation, these libraries serve as invaluable resources for both beginners and experienced practitioners in the field of time series analysis and forecasting.
- Statsmodels: Statsmodels is a Python library for statistical modeling and hypothesis testing. It includes a wide range of statistical methods and models, including time series analysis tools like ARIMA, SARIMA, and VAR. Statsmodels is useful for performing classical statistical tests and building traditional time series models.
- Pmdarima: Pmdarima is a Python library that provides an interface to ARIMA models in a manner similar to that of scikit-learn. It automates the process of selecting optimal ARIMA parameters and fitting models to time series data.
- Prophet: Prophet is a forecasting tool developed by Facebook that is specifically designed for time series forecasting at scale. It provides a simple yet powerful interface for fitting and forecasting time series data, with built-in support for handling seasonality, holidays, and trend changes.
- tslearn: tslearn is a Python library for time series learning, which provides various algorithms and tools for time series classification, clustering, and regression. It offers implementations of state-of-the-art algorithms, such as dynamic time warping (DTW) and shapelets, for analyzing and mining time series data.
- ARCH: ARCH is a Python library for estimating and forecasting volatility models commonly used in financial econometrics. It provides tools for fitting autoregressive conditional heteroskedasticity (ARCH) and generalized autoregressive conditional heteroskedasticity (GARCH) models to time series data.
- GluonTS: GluonTS is a Python library for probabilistic time series forecasting developed by Amazon. It provides a collection of state-of-the-art deep learning models and tools for building and training probabilistic forecasting models for time series data.
- PyFlux: PyFlux is a Python library for time series analysis and forecasting, which provides implementations of various time series models, including ARIMA, GARCH, and stochastic volatility models. It offers an intuitive interface for fitting and forecasting time series data with Bayesian inference methods.
- Sktime: Sktime is a Python library for machine learning with time series data, which provides a unified interface for building and evaluating machine learning models for time series forecasting, classification, and regression tasks. It integrates seamlessly with scikit-learn and offers tools for handling time series data efficiently.
- PyCaret: PyCaret is an open-source, low-code machine learning library in Python that automates the machine learning workflow. It supports time series forecasting tasks and provides tools for data preprocessing, feature engineering, model selection, and evaluation in a simple and streamlined manner.
- Darts: Darts (Data Augmentation for Regression Tasks with SVD) is a Python library for time series forecasting. It provides a flexible and modular framework for developing and evaluating forecasting models, including classical and deep learning-based approaches. Darts emphasizes simplicity, scalability, and reproducibility in time series analysis and forecasting tasks.
- Kats: Kats, short for “Kits to Analyze Time Series,” is an open-source Python library developed by Facebook. It provides a comprehensive toolkit for time series analysis, offering a wide range of functionalities to handle various aspects of time series data. Kats includes tools for time series forecasting, anomaly detection, feature engineering, and model evaluation. It aims to simplify the process of working with time series data by providing an intuitive interface and a collection of state-of-the-art algorithms.
- AutoTS : AutoTS, or Automated Time Series, is a Python library developed to simplify time series forecasting by automating the model selection and parameter tuning process. It employs machine learning algorithms and statistical techniques to automatically identify the most suitable forecasting models and parameters for a given dataset. This automation saves time and effort by eliminating the need for manual model selection and tuning.
- Scikit-learn: Scikit-learn is a popular machine learning library in Python that provides a wide range of algorithms and tools for data mining and analysis. While not specifically tailored for time series analysis, Scikit-learn offers some useful algorithms for forecasting tasks, such as regression, classification, and clustering.
- TensorFlow: TensorFlow is an open-source machine learning framework developed by Google. It is widely used for building and training deep learning models, including recurrent neural networks (RNNs) and long short-term memory networks (LSTMs), which are commonly used for time series forecasting tasks.
- Keras: Keras is a high-level neural networks API written in Python, which runs on top of TensorFlow. It provides a user-friendly interface for building and training neural networks, including recurrent and convolutional neural networks, for various machine learning tasks, including time series forecasting.
- PyTorch: PyTorch is another popular deep learning framework that is widely used for building neural network models. It offers dynamic computation graphs and a flexible architecture, making it suitable for prototyping and experimenting with complex models for time series forecasting.
Comparative Analysis of Python Libraries for Time Series
Python offers a diverse range of libraries and frameworks tailored for time series tasks, each with its own set of strengths and weaknesses. In this comparative analysis, we evaluate top Python libraries, which is commonly used for time series analysis and forecasting.
This table provides an overview of each library’s focus area, strengths, and weaknesses in the context of time series analysis and forecasting.
Python offers a rich ecosystem of libraries and frameworks tailored for time series analysis and forecasting, catering to diverse needs across various domains. From traditional statistical modeling with libraries like Statsmodels to cutting-edge deep learning approaches enabled by TensorFlow and PyTorch, practitioners have a wide array of tools at their disposal. However, each library comes with its own trade-offs in terms of usability, flexibility, and computational requirements. Choosing the right tool depends on the specific requirements of the task at hand, balancing factors like model complexity, interpretability, and computational efficiency. Overall, Python’s versatility and the breadth of available libraries empower analysts and data scientists to extract meaningful insights and make accurate predictions from time series data across different domains.
Q. What is time series data?
Time series data is a sequence of data points collected, recorded, or measured at successive, evenly spaced time intervals. It represents observations or measurements taken over time, such as stock prices, temperature readings, or sales figures.
Q. What are the four main components of a time series?
The four main components of a time series are: Trend Seasonality Cyclical variations Irregularity (or Noise)
Q. What is stationarity in time series?
Stationarity in time series refers to the property where the statistical properties of the data, such as mean and variance, remain constant over time. It indicates that the time series data does not exhibit trends or seasonality and is crucial for building accurate forecasting models.
Q. What is the real-time application of time series analysis and forecasting?
Time series analysis and forecasting have various real-time applications across different domains, including: Financial markets for predicting stock prices and market trends. Weather forecasting for predicting temperature, precipitation, and other meteorological variables. Energy demand forecasting for optimizing energy production and distribution. Healthcare for predicting patient admissions, disease outbreaks, and medical resource allocation. Retail for forecasting sales, demand, and inventory management.
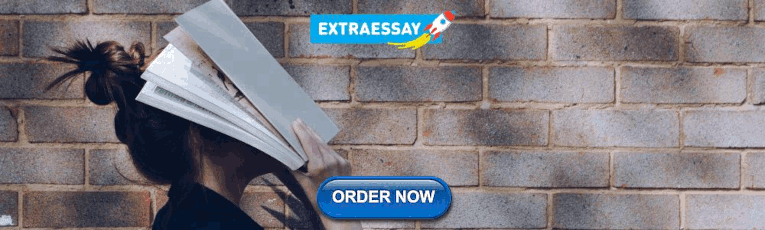
Q. What do you mean by Dynamic Time Warping?
Dynamic Time Warping (DTW) is a technique used to measure the similarity between two sequences of data that may vary in time or speed. It aligns the sequences by stretching or compressing them in time to find the optimal matching between corresponding points. DTW is commonly used in time series analysis, speech recognition, and pattern recognition tasks where the sequences being compared have different lengths or rates of change.
Please Login to comment...
Similar reads.
- Time Series
- Machine Learning
Improve your Coding Skills with Practice
What kind of Experience do you want to share?

April 2024 Global Climate Report
- Monthly Report
- Summary Info
- Monthly Briefings
Additional Resources
Temperature and precipitation maps, temperature anomalies time series.
- Year-to-Date
Supplemental Material
- 2024 Year-to-Date Temperatures Versus Previous Years
- Global Annual Temperature Rankings Outlook
- Mean Monthly Temperature Records Across the Globe
- Monthly Temperature Anomalies Versus El Niño
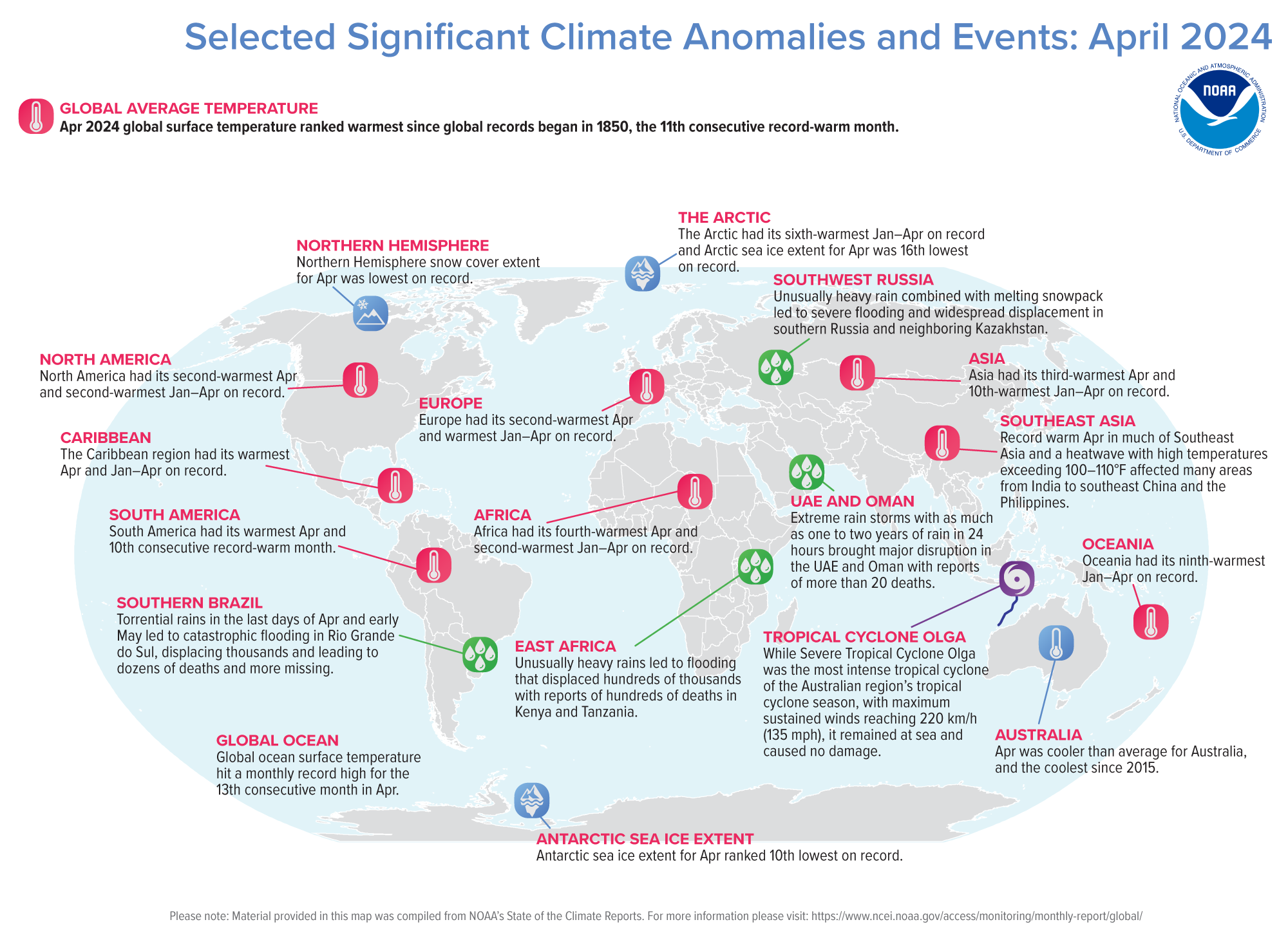
Temperature
In January 2024, the NOAA Global Surface Temperature (NOAAGlobalTemp) dataset version 6.0.0 replaced version 5.1.0. This new version incorporates an artificial neural network (ANN) method to improve the spatial interpolation of monthly land surface air temperatures. The period of record (1850-present) and complete global coverage remain the same as in the previous version of NOAAGlobalTemp. While anomalies and ranks might differ slightly from what was reported previously, the main conclusions regarding global climate change are very similar to the previous version. Please see our Commonly Asked Questions Document and web story for additional information.
NOAA's National Centers for Environmental Information calculates the global temperature anomaly every month based on preliminary data generated from authoritative datasets of temperature observations from around the globe. The major dataset, NOAAGlobalTemp version 6.0.0 , updated in 2024, uses comprehensive data collections of increased global area coverage over both land and ocean surfaces. NOAAGlobalTempv6.0.0 is a reconstructed dataset, meaning that the entire period of record is recalculated each month with new data. Based on those new calculations, the new historical data can bring about updates to previously reported values. These factors, together, mean that calculations from the past may be superseded by the most recent data and can affect the numbers reported in the monthly climate reports. The most current reconstruction analysis is always considered the most representative and precise of the climate system, and it is publicly available through Climate at a Glance .
April 2024 was the warmest April on record for the globe in NOAA's 175-year record. The April global surface temperature was 1.32°C (2.38°F) above the 20th-century average of 13.7°C (56.7°F). This is 0.18°C (0.32°F) warmer than the previous April record set most recently in 2020, and the eleventh consecutive month of record-high global temperatures. April 2024 marked the 48th consecutive April with global temperatures, at least nominally, above the 20th-century average.
Global land-only April temperature was warmest on record at 1.97°C (3.55°F) above average. The ocean-only temperature also ranked warmest on record for April at 1.03°C (1.85°F) above average, 0.17°C (0.31°F) warmer than the second warmest April of 2023, and the 13th-consecutive monthly ocean record high. These temperatures occurred as the current El Niño episode nears its end. El Niño conditions that emerged in June 2023 weakened further in April, and according to NOAA's Climate Prediction Center , a transition from El Niño to ENSO–neutral is likely in the next month, with odds of La Niña developing by June–August (49% chance) or July–September 2024 (69% chance).
Temperatures were much-above average to record warm throughout much of South America, Africa, central and southern Europe, southwestern Russia and Turkey, as well as much of Asia's Far East. Temperatures were also much warmer-than-average across large parts of the northeast U.S. and much of northern Canada. The largest temperature anomalies (greater than 3°C or 5.4°F above average) occurred in northern Canada, western and northern Greenland, eastern Europe, central Asia, southeast Asia, eastern China and parts of eastern Russia.
Record warm April temperatures in Southeast Asia were due in part to a heatwave in late April with daily high temperatures exceeding 38-43°C (100-110°F) in an area stretching from India to southeastern China and the Philippines. Examples of station's highest maximum temperatures to occur during the last half of April in this region from NCEI's Global Historical Climatology Network-Daily dataset include more than 75 stations with highest temperatures exceeding 40.5°C (105°F). Extremely warm overnight temperatures, exceeding 26.5°C (80°F) were also widespread .
As was the case in recent months, sea surface temperatures were above average across much of the northern, western, and equatorial Pacific Ocean, although the positive anomalies in the central and eastern equatorial Pacific were smaller than recent months as El Niño weakened. As they did in March, record-warm temperatures covered much of the tropical Atlantic Ocean as well as parts of the southern Atlantic and northwestern and southern Indian Ocean. Record warm temperatures covered 14.7% of the world's surface this month. This is more than twice the second-highest April value of 7.1% set in 2016 and the second highest record warm coverage for any month since records began in 1951, slightly below the 15.0% coverage in September 2023.
April temperatures were cooler than the 1991–2020 average in areas that included much of mainland Australia, southern parts of South America, much of Iceland, Scandinavia and northwest Russia, eastern Iran, Afghanistan, and Pakistan, as well as parts of East Africa including Sudan and South Sudan. Much of east Antarctica was cooler than the 1991-2020 average with anomalies lower than -2°C (-3.6°F) widespread. Sea surface temperatures were below average in parts of the southeastern Pacific and Southern Ocean. Record cold temperatures covered 0.1% of the world's surface in April.
In the Northern Hemisphere, April 2024 ranked warmest on record at 1.75°C (3.15°F) above average, 0.28°C (0.50°F) warmer than the previous April record of 2016. The Northern Hemisphere land temperature and ocean temperature each ranked warmest on record for the month. The Southern Hemisphere experienced its second warmest April on record at 0.88°C (1.58°F) above average, 0.05°C (0.09°F) cooler than 2023. The Southern Hemisphere land temperature for April tied 2010 as 20th warmest while April's ocean temperature was warmest on record.
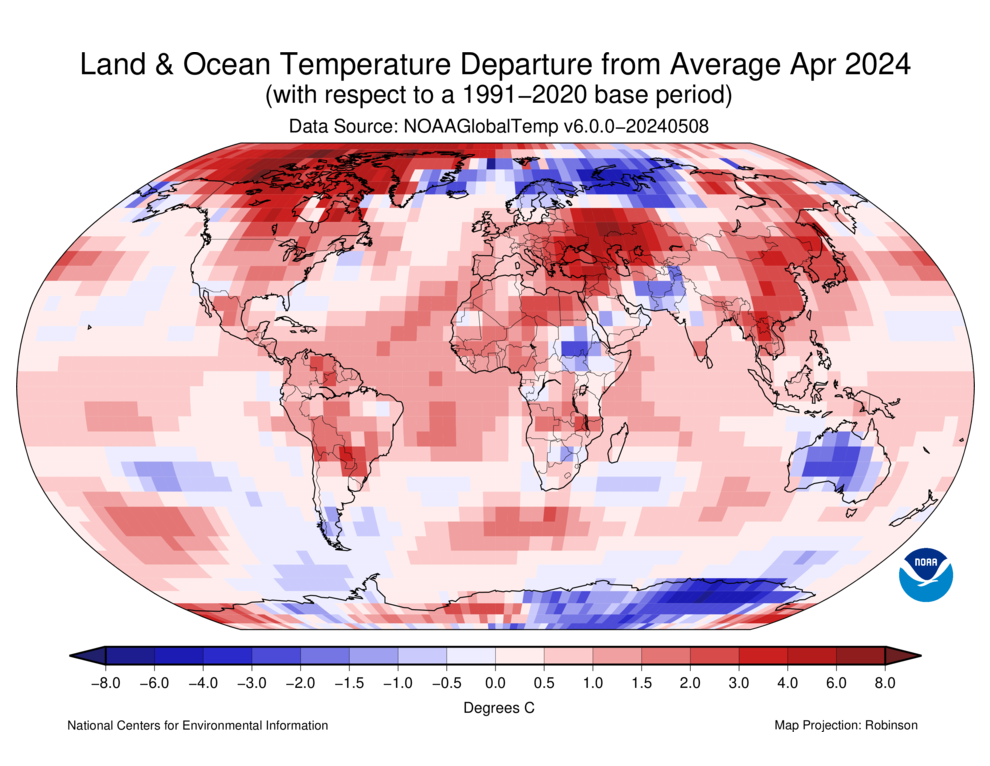
A smoothed map of blended land and sea surface temperature anomalies is also available.
South America had its warmest April while Europe had its second-warmest and Africa its fourth-warmest April on record.
- In Germany April 2024 was the 14th-warmest April since records began in 1881, 1.1°C warmer than the 1991–2020 average, 2.7°C warmer than the 1961–1990 average.
- April 2024 was the ninth-warmest April in Italy , based on preliminary data, 1.22°C warmer than the 1991–2020 average in a record that started in 1800. Northern Italy had its 18th-warmest April, central Italy its fourth-warmest, and southern Italy its fifth warmest.
- In Austria the first half of April was exceptionally warm, with 100 of 280 weather stations reaching new heat records for April. The highest April temperature since records began in the late 1800's occurred at the Innsbruck University station and Graz University station. Cold air intrusions during the latter half of the month were more typical of April and for the month as a whole the mean temperature for the lowlands of Austria was 1.2°C above then 1991–2020 average and 1.8°C above average in the mountains. These were, respectively, the 13th warmest in the 258-year lowlands record and 10th warmest in the 174-year mountain record.
- In the United Kingdom the first half of April was generally warmer than average and the second half of the month cooler than average. For April, the average mean temperature for the UK was 0.4°C above the 1991–2020 average, its 22nd-warmest April in a series that began in 1884, based on provisional data.
- In Iceland, April 2024 temperatures were cooler than average across the country. Anomalies as low as -1.8°C below the 1991–2020 average occurred in Akureyri and Egilsstadir, with even colder negative anomalies in many locations when compared to temperatures of the past ten years.
- In Argentina temperatures for April were generally below average in the southern half of the country and warmer than average in northern areas. Monthly anomalies exceeding +2°C above the 1991–2020 average were largely confined to parts of the northern provinces of Formosa, Chaco, and Misiones. Temperatures more than -1°C cooler than average occurred in the southern provinces of Chubut, Santa Cruz, and Río Negro.
Asia had its third-warmest April and Oceania its 54th warmest April on record.
- The April 2024 national mean monthly temperature for Pakistan was 0.87°C below the country average of 24.54°C. The hottest day of the month in Pakistan occurred at Shaheed Benazirabad (Sindh province), when the high temperature reached 43.5°C (110.3°F) on April 8.
- According to the Hong Kong Observatory , under the influence of warmer than normal sea surface temperatures and stronger than usual southerly flow over the northern part of the South China Sea, the mean temperature in April 2024 for Hong Kong was the warmest on record at 3.4°C above the 1991–2020 average. The mean maximum and mean minimum temperatures also were warmest on record for April.
- In Australia the national area-average mean temperature for April was 0.51°C below the 1961-1990 average, the lowest since 2015, and the mean minimum temperature in South Australia was 10th coolest on record. Temperatures were below-average across most of mainland Australia
North America had its second warmest April at 2.45°C (4.41°F) above average.
- The average temperature of the contiguous U.S. in April 2024 was 53.8°F, which is 2.7°F above the 1901-2000 average, the warmest April since 2017 and the 12th warmest such month on record.
- The Caribbean region had its warmest April on record, 1.40°C (2.52°F) above the 1910-2000 average. This is 0.22°C (0.40°F) above the second warmest April of 2020.
500 mb maps
In the atmosphere, 500-millibar height pressure anomalies correlate well with temperatures at the Earth's surface. The average position of the upper-level ridges of high pressure and troughs of low pressure—depicted by positive and negative 500-millibar height anomalies on the map—is generally reflected by areas of positive and negative temperature anomalies at the surface, respectively.
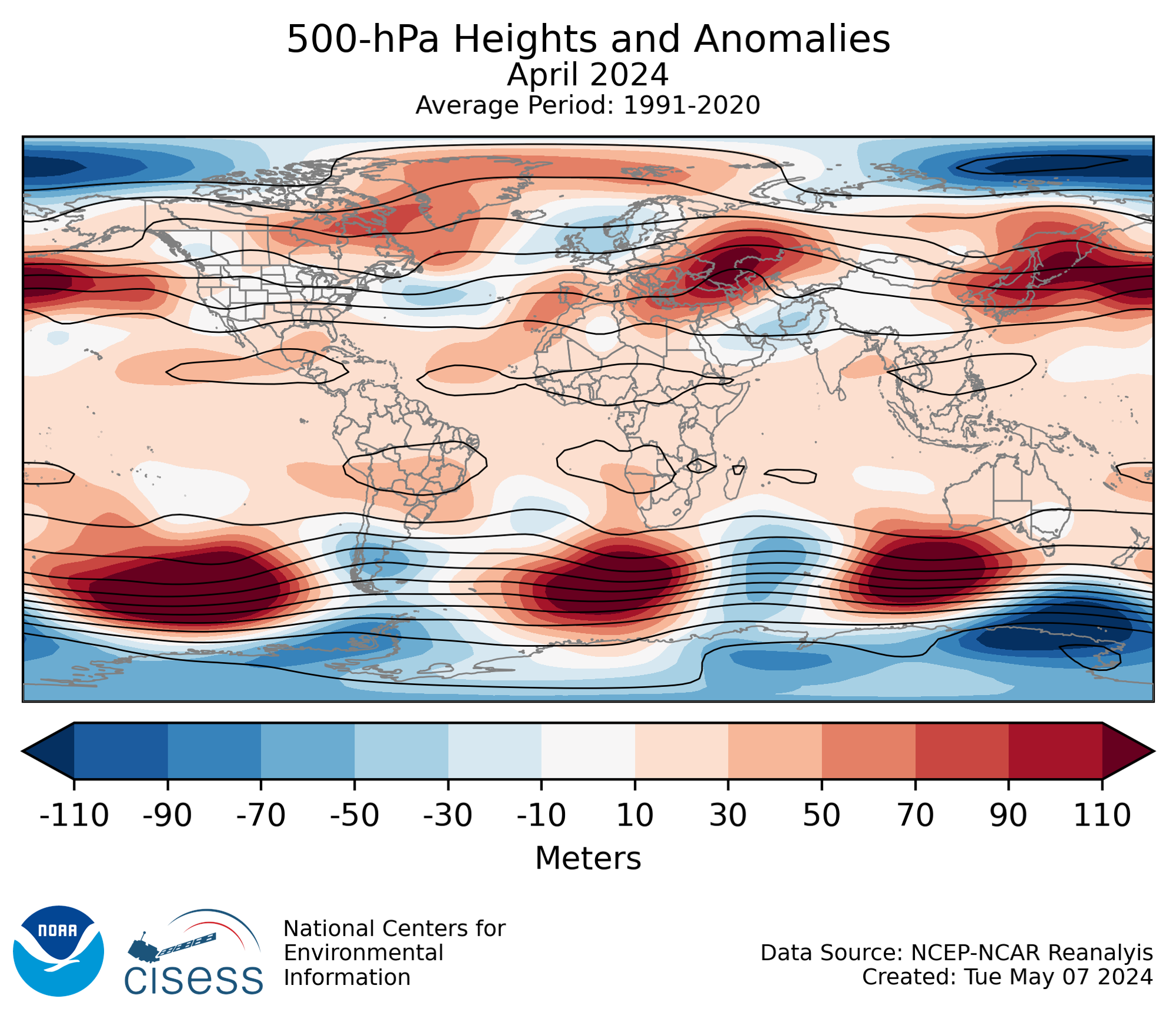
Year-to-date Temperature: January–April 2024
The January–April global surface temperature ranked warmest in the 175-year record at 1.34°C (2.41°F) above the 1901-2000 average of 12.6°C (54.7°F). According to NCEI's statistical analysis , there is a 61% chance that 2024 will rank as the warmest year on record and a 100% chance that it will rank in the top five.
For the January–April year-to-date period, South America and western and southern Europe are notable for the great expanse of record-warm temperatures. Much-warmer-than-average and record warm conditions also covered much of Africa, southern Asia, and a large part of eastern North America. Temperatures for the four-month year-to-date period also were warmer-than-average across central and northern Asia, much of southern and eastern Australia, and the western half of North America. Cooler-than-average temperatures were widespread in Antarctica. Temperatures below the 1991—2020 average also were notable in areas of eastern Greenland, Iceland, Scandinavia, much of northern India and northern Pakistan, northern Australia, and small parts of eastern Russia.
Sea surface temperatures were much warmer-than-average across much of the northern and equatorial Pacific as well as the southwest Pacific. Record-warm January–April sea surface temperatures stretched from the Caribbean Sea across the tropical Atlantic and to the northeastern Atlantic. Record warm temperatures also affected large parts of the Indian Ocean, the southern Atlantic and parts of the southwestern Pacific Ocean. The most widespread areas of sea surface temperatures below the 1991—2020 average occurred in the southeastern Pacific, southwestern Indian Ocean and parts of the Southern Ocean.
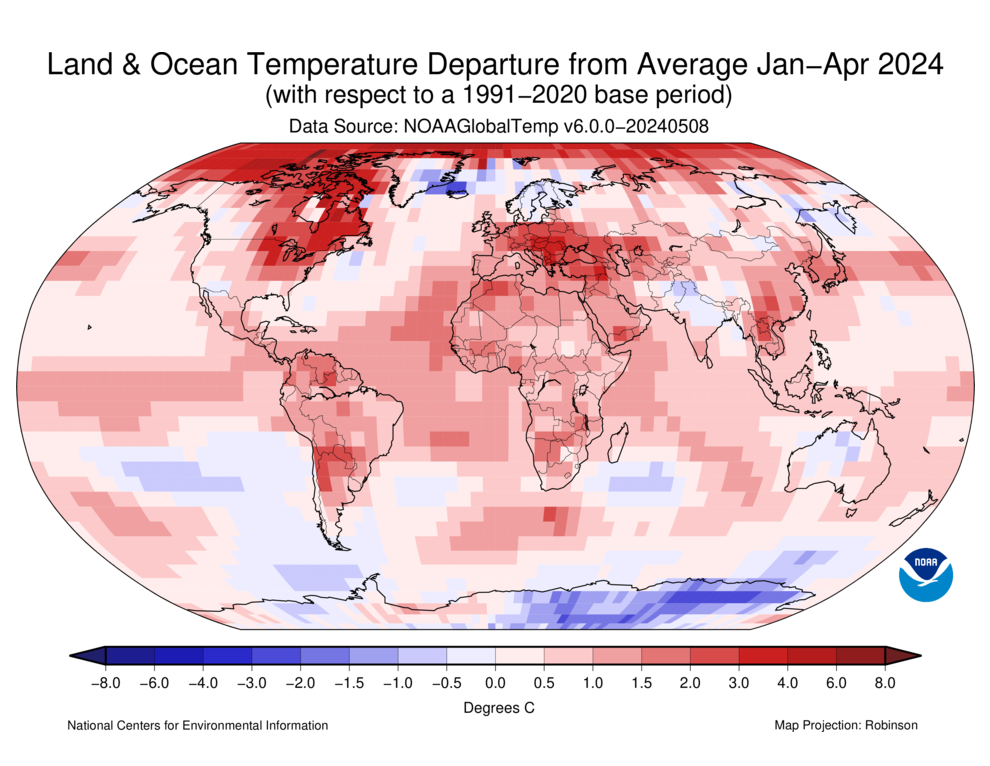
South America and Europe had their warmest January–April year-to-date period, and Africa and North America their second-warmest such period on record. Oceania had its ninth-warmest January–April period and Asia its 10th warmest. Overall, the Northern Hemisphere and Southern Hemisphere each had their warmest January–April on record.
- The average temperature of the contiguous U.S. for the January-April 2024 period was 43.0°F, which is 3.8°F above the 1901-2000 average, ranking as the fifth warmest on record.
- The Caribbean region had its warmest January-April on record, 1.28°C (2.30°F) above the 1910-2000 average.
Precipitation
The maps shown below represent precipitation percent of normal (left, using a base period of 1961–1990) and precipitation percentiles (right, using the period of record) based on the GHCN dataset of land surface stations.
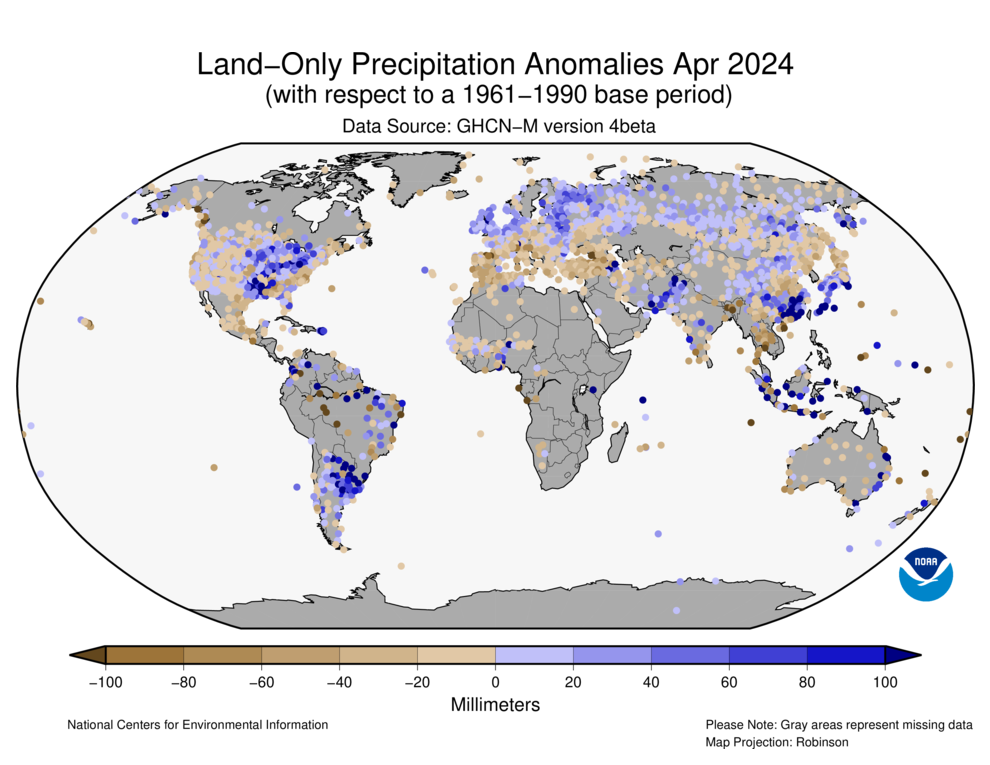
Below-average April precipitation occurred in areas that included a large region stretching across southern and central Europe from Portugal and Spain to Turkey and Ukraine. Drier-than-average conditions also occurred in much of western Iran, Nepal, Southeast Asia, and much of mainland Australia. Large parts of Mexico and the western United States and eastern Alaska were drier-than-average in April. Conversely, April was wetter than average in areas that included much of Argentina, southern and eastern Brazil, Uruguay and Paraguay, much of the central and northeast United States as well as western Alaska, northern Europe, much of Pakistan, parts of central and eastern China, and much of Russia.
- In the UAE and Oman exceptionally heavy rainfall, as much as one to two-years of rain in 24 hours, caused massive disruption in infrastructure and public life. Observations provided every six hours at Dubai International airport showed a combined total of 159 mm (6.26 in) in the 24-hour period ending at 10pm local on April 16. This was twice the normal amount of rain received in a year ; 79.2 mm (3.12 in). A group of international researchers determined contributing factors to this extreme event to be the influence of El Niño and an increase in heavy rainfall that has occurred as the global climate has warmed.
- In southern Russia and northern Kazahkstan heavy rains and rapid snowmelt under much-warmer-than-average conditions led to devastating floods as rivers rose and overflowed their banks, some to record levels . Widespread damage to infrastructure and displacement of thousands were reported.
- Devastating flooding also occurred in East Africa as unusually heavy rains caused rivers to overflow their banks with reports of hundreds of thousands displaced and hundreds of deaths . Countries most severely affected include Kenya , Tanzania , and Burundi.
- In Brazil torrential rains in the last days of April and early May led to catastrophic flooding in Rio Grande do Sul, reportedly displacing thousands and with dozens of deaths and many more missing . The flooding is reported to be the worst in 80 years .
- According to the Pakistan Meteorological Department Pakistan's nationally averaged rainfall for April 2024 was 164% above average, the record wettest April since 1961 (breaking the previous record set in 1983). Flash floods occurred in Balochistan and upper Khyber Pakhtunkhwa province associated with riverine flooding of the Kabul River.
- According to the Met Office , April precipitation for the UK as a whole was 155% of the 1991-2020 average based on provisional data, its sixth-wettest April in the 189-year record. All countries in the UK provisionally recorded over 100% of their average April rainfall. Scotland (160%) and northern England (176%) were particularly wet, and Edinburgh provisionally had its second wettest April on record dating back to 1836 (more than 200% of average).
- In Argentina , precipitation in April was most notably above average in northern provinces. Monthly totals exceeding 100 mm were widespread in the northern provinces of Salta, Formosa, Chaco, and Corrientes and anomalies were greater than 50 mm above average in many areas.
- According to the Australian Bureau of Meteorology the national area-averaged April rainfall total was 26.0% below the 1961-1990 average. It was the eighth-driest April on record for South Australia and rainfall in April was below average for western, southern and central parts of the country. Rainfall was generally above average in Australia's east and north.
- Adler, R., G. Gu, M. Sapiano, J. Wang, G. Huffman 2017. Global Precipitation: Means, Variations and Trends During the Satellite Era (1979-2014). Surveys in Geophysics 38: 679-699, doi:10.1007/s10712-017-9416-4
- Adler, R., M. Sapiano, G. Huffman, J. Wang, G. Gu, D. Bolvin, L. Chiu, U. Schneider, A. Becker, E. Nelkin, P. Xie, R. Ferraro, D. Shin, 2018. The Global Precipitation Climatology Project (GPCP) Monthly Analysis (New Version 2.3) and a Review of 2017 Global Precipitation. Atmosphere. 9(4), 138; doi:10.3390/atmos9040138
- Gu, G., and R. Adler, 2022. Observed Variability and Trends in Global Precipitation During 1979-2020. Climate Dynamics, doi:10.1007/s00382-022-06567-9
- Huang, B., Peter W. Thorne, et. al, 2017: Extended Reconstructed Sea Surface Temperature version 5 (ERSSTv5), Upgrades, validations, and intercomparisons. J. Climate, doi: 10.1175/JCLI-D-16-0836.1
- Huang, B., V.F. Banzon, E. Freeman, J. Lawrimore, W. Liu, T.C. Peterson, T.M. Smith, P.W. Thorne, S.D. Woodruff, and H-M. Zhang, 2016: Extended Reconstructed Sea Surface Temperature Version 4 (ERSST.v4). Part I: Upgrades and Intercomparisons. J. Climate , 28 , 911-930, doi:10.1175/JCLI-D-14-00006.1.
- Menne, M. J., C. N. Williams, B.E. Gleason, J. J Rennie, and J. H. Lawrimore, 2018: The Global Historical Climatology Network Monthly Temperature Dataset, Version 4. J. Climate, in press. https://doi.org/10.1175/JCLI-D-18-0094.1 .
- Peterson, T.C. and R.S. Vose, 1997: An Overview of the Global Historical Climatology Network Database. Bull. Amer. Meteorol. Soc. , 78 , 2837-2849.
- Vose, R., B. Huang, X. Yin, D. Arndt, D. R. Easterling, J. H. Lawrimore, M. J. Menne, A. Sanchez-Lugo, and H. M. Zhang, 2021. Implementing Full Spatial Coverage in NOAA's Global Temperature Analysis. Geophysical Research Letters 48(10), e2020GL090873; doi:10.1029/2020gl090873 .
Citing This Report
MATH1318 Time Series Analysis - Assignment - 1
Akash singh (s3871025), introduction.
The dataset represents the list of closing price which is also the last price if anyone paid for a share of that stock during business hours of the exchange where the stock trade is done. Whereas the opening price is the price where the first transaction is set to be the open transaction of any business day. Usually, ASX uses a Closing Single Price Auction where it generates a price that reflects the interaction of supply demands in the market.
To use a model-building technique as to examine the given dataset and select the best-fitting model, followed by a prediction for the next 5 observations. Determining the trends between Deterministic and Stochastic. Choosing appropriate model to satisfy the deterministic trend. Fitting the Regression Model to the Data
- R software is used for time series analysis on the data.
- Time Series Load
- Calculating Variance and Correlation techniques
- Regression modelling
- Forecasting
Data Exploration and Manipulation
Dataset description.
The dataset contains 144 observations out of a total of 252 trading days in a year, with all the data collected in the same year and on consecutive trading days.
Importing packages
For modelling and prediction, we’ll use functions from the TSA package. R functions and datasets includes in the TSA package. The TSA package includes utilities for performing unit root tests, which might be useful for diagnostics and model specification.
Reading the data and setting the path
The setwd() method is used to set the working directory, and R’s fetching the csv function is used as to read the data as Stock.csv The output tells us that the dataset is a dataframe with 144 observations with having 2 variables. We rename this variable to Stocks to make it clearer.
The expected datatype for time series analysis is “Time Series” as we don’t have a time series datatype by default, we’ll need to transform the datatype. To convert the data into a time series, we use the ts() method from the TSA package.
Syntax: ts(df$col, frequency=z)
The data is recorded for every business day where the shares of the stock during business hours is calculated. The analysis is done by working on day-to-day frequency report. Thus, the frequency is taken as total number of 144 days, which gives the analysis of data for 144days.
Analysis of deterministic trend models for model fitting
Using a model-building method, assess the given dataset and determine the best-fitting model among the linear, quadratic, cosine, and seasonal models. To use the best model to forecast annual changes for the following five years.
Plotting the data
The plot() function is used to plot the time series data, which is then examined for the following 5 valid points: 1. Seasonality 2. Trend 3. Behaviour 4. Intervention 5. Change in Variance
Analysis of the 5 Valid points
Seasonality : Overall, we can see that the time series plot has a definite repeating pattern. This is due to the mid-section of the plot, which ranges from 1.5 to 2.0, indicating that the model has seasonality. However, there are clues of pattern at the start of the plot, leading us to wonder if this model can display non-seasonality properties. At this stage, we can affirm that the time series is seasonal. As a result, it demonstrates the series’ seasonality features. Trend : The time series graphic demonstrates the significant declining trend. Change in Variance : We can say there is a slight change in variance. If you observe carefully there are fluctuations between 1.5 to 1.99 which indicates higher jumps exactly from 1.6 to 2.0. This indicates change in variance in the data. Intervention Point : Clearly, there is no point of intervention. A change point happens when the plot’s qualities undergo a significant/dramatic change. There is no comparable behaviour in the data over here, indicating that there was no intervention point.
Impact of previous year(s) on the current year
We do the correlation for the specified variables after loading the Time series; for variance, we use Zlag to generate the initial lag of stocks, and for y value, we use Stocks because the data is acquired from the closing prices of shares. After this index is generated, it assists us in eliminating the first NA values as well as the final five missing values. When it comes to correlation, if the cor value is close to 1, the stated analysis is acceptable to implement. The Pearson correlation coefficient can be used to determine the impact of prior days on the current day. It’s calculated by multiplying the covariance of the two variables by their standard deviations as a product. The result is normalized and always falls within the range of -1 and 1. The negative correlation between the two is explained by the negative value, while the positive correlation is explained by the positive value. Let’s start by calculating the pearson’s correlation between today’s Y[t] and yesterday’s Y[t-1], then move on to days Y[t-2], Y[t-3], and so on, until we get a value less than or equal to 1. This pearson’s correlation is followed by a correlation plot which helps us visually understand the relationship between the two.
PEARSON CORRELATION OF Y[t] and Y[t-1]
The correlation of 0.9678333 Y[t] and Y[t-1] appear to be positively connected. As a result, a substantial amount of data can be recovered from previous values (lag 1). By showing the first lag along the x axis and the actual value along the y axis, this correlation can be seen.
CORRELATION PLOT OF Y[t] and Y[t-1]
The positive correlation between the two is explained by the fact that as Y[t] increases, so does Y[t-1]. As a result, the past value can be used to retrieve a substantial amount of data (lag 1)
PEARSON CORRELATION OF Y[t] and Y[t-2]
The correlation of 0.9198548 suggest that Y[t] and Y[t-2] are positively correlated but the correlation and it is not stronger. Plotting second lag along the x axis and the actual value along the y axis reveals this correlation.
CORRELATION PLOT OF Y[t] and Y[t-2]
There is still a positive correlation between Y[t] and Y[t-2]. Higher values of Y[t] gives higher values of Y[t-2] which explains their correlation. However, it is not stronger compared to the first lag.
PEARSON CORRELATION OF Y[t] and Y[t-3]
The correlation of 0.9088598 suggest that Y[t] and Y[t-3] are positively correlated. This correlation can be understood by plotting second lag along the x axis and the real values along the y axis.
CORRELATION PLOT OF Y[t] and Y[t-3]
Not all higher values of Y[t] have higher values as of Y[t-3] which explains correlation is strong.
Model Estimation
Deterministic trend models.
The model’s qualities are still an unknown to us. One method of estimate is to fit a regression model. To model a non-constant mean trend, regression analysis can be applied.
Regression Trend Models: 1. Linear 2. Quadratic 3. Cosine
Linear trend Model
First, get the time stamps of the time series
The lm() function is used to calculate the regression model, while the abline() function is used to fit the model.
Summary of the model
- The coefficient of t is -37.983, this means there is a 37.983 decrement for each time period.
- Pr(>|t|) for t is < 0.05, therefore there is significant information in t and this component can contribute for modelling.
- R-squared value for the model is 0.4513. We would like to have R-squared value between 0.75 and 0.85. Over here 0.4513 says that the model is not good enough and it is rejected.
- The overall p-value is less than 0.05 which means this trend model is good for fitting.
Residual Analysis of the linear trend model
Time series plot of standardized residuals: A random white noise sequence of standardised residuals is ideal, as it indicates that the fitted trend model has caught the bulk of the observations.
Histogram Plot
The frequency of standardized residuals is represented via a histogram. To firmly support the trend fit, we need a symmetric distribution of standardized residuals, which ideally doesn’t fit.
On the QQ plot of normalized residuals, we want to see all the dots should stick to the reference line.
Shapiro-wilk Test
The Shapiro-Wilk test is used to determine whether standardized residuals are normal. Ho: Data are normally distributed HA: Data are not normally distributed If p > 0.05, the null hypothesis is rejected, and we conclude that the data is not normal. If p > 0.05, however, we cannot reject the null hypothesis and must conclude that the data is regularly distributed. As here p value is 0.01413.
ACF & PACF Plot
In an ACF and PACF graphic of standardized residuals, we anticipate observing all bars under the horizontal dashed confidence boundaries. This explains why all the data has been captured and there is no more data to capture in the residuals.
Summary of residual analysis
A random white noise sequence of standardized residuals is desirable since it suggests that the fitted trend model has correctly predicted most of the data. A histogram is used to depict the frequency of standardized residuals. A symmetric distribution of standardized residuals, which ideally does not fit, is required to firmly establish the trend fit. We want to see all the dots on the QQ plot of normalized residuals cling to the reference line. To evaluate if standardized residuals are normal, the Shapiro-Wilk test is utilized. The null hypothesis is rejected if p > 0.05, and we conclude that the data are not normal. If p > 0.05, however, the null hypothesis cannot be rejected, and we must infer that the data is normally distributed. Thus, histogram plot does not support that the trend model captures majority of the observation. We expect to see all bars beneath the horizontal dashed confidence boundaries in an ACF and PACF picture of standardized residuals. This explains why the residuals have no more data to record because all the data has been captured. p < 0.05 confirms that the data is not normally distributed.
Quadratic Trend Model
Over here, t2 is calculated by squaring t which is used as a parameter in a quadratic model.
1.The coefficient of t is 388.67, this means there is a 388.67 increment for each time period (t). 2.The coefficient of t2 is -142.55, this means there is a decrements of -142.55 for each time period (t2). 3.Pr(>|t|) for t and t2 is < 0.05, therefore there is significant information in t and t2. 4.R-squared value for the model is 0.8807. Ideally, we should be having R-squared value between 0.75 and 0.85. Over here 0.0.8807 says that the model is good for fitting, and it is best approach as compared to Linear trend model. 5.The overall p-value is less than 0.05 which means this trend model is good for fitting and capable of capturing the information. As, the value is smaller, so it signifies the stronger evidence to reject the Ho.
Residual Analysis of the quadratic trend model
Performing residual analysis on the quadratic trend model.
Summary of Residual Analysis of Quadratic trend
This model looks much better than the previous Linear model. Thus, the time series plot of the standardized residuals support that the trend model captures majority. The histogram plot of standardized residuals almost shows a symmetric distribution. Thus, it is a right skewed distribution. Almost all the dots stick to the reference line which suggest hat the model is fitted. Shapiro-wilk test gives a result of p = 0.2324 and p > 0.05 confirms that the data is normally distributed. Most of the bars are within the horizontal dashed confidence boundaries in an ACF picture of standardized residuals, which explains have data is recorded and captured simultaneously.
Cosine Trend Model
The harmonics are initially calculated using the harmonic(x,m) function, where “x” denotes the time series and “m” denotes the number of pairs of harmonic functions to be constructed. The time series data and harmonics are combined to generate a dataframe. The cosine behavior is then modelled using the regression function lm().
Trying to fit the cosine model for the time series data.
Summary of the Model
- The p-values for the coefficients for cosine and sine terms are more than 0.05, indicating that they are not significant and are ineffective at capturing information.
- The model’s R-squared value is -0.5545. The R-squared value should ideally be between 0.75 and 0.85. -0.5545 indicates that the model is unsuitable for fitting.
- The aggregate p-value is greater than 0.05, implying that this trend model is not significant and unfittable.
Residual Analysis of the cosine trend model
Let’s look at the residuals of the cosine trend model.
Summary of Residual Analysis
The time series plot of the standardized residuals shows no pattern. The standardized residuals time series plot indicates that the model did not capture the majority of the data. As a result, this trend model will provide a poor fit. The presence of a symmetric distribution is not indicated by a histogram plot. The majority of the dots are pointing away from the reference line, indicating that the trend model fit is poor. The Shapiro-Wilk test yields a p value of 1.002e-06, indicating that the data is not regularly distributed. There are many lags above the confidence interval in both ACF and PACF. This demonstrates that a significant amount of data is not gathered, indicating that this strategy is ineffective.
We may conclude that of the four deterministic models, the quadratic model has the most variety. It also adheres to the rules of normality. As a result, we may conclude that it is the most appropriate fit for our Stocks Data set. We will now use the Quadratic model to forecast the stock market’s trend for the next five years.
The data is first transformed to a time series and examined for 5 valid points, after which the data’s pearson correlation with its lags is computed. The ACF-PACF plot explains the series is seasonal nature while also it indicates that it is non-stationary. Assuming the series is deterministic, we try to fit linear, quadratic,and cosine regression models to it and examine the summary, followed by residual analysis for the four models. Following the research, it is obvious that the quadratic model provides the best fit and aids in the capture of the majority of data for prediction. The forecast for the next five years is calculated using a quadratic model. We may conclude from the deterministic trend modelling and residual analysis determines that the model has a weak deterministic trend and follows a stochastic trend.
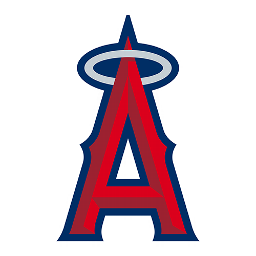
Los Angeles Angels
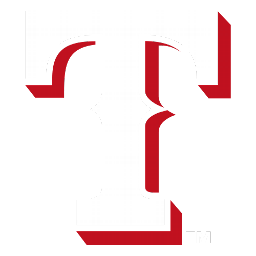
Texas Rangers
Soriano's career-long start and pillar's pinch hit get angels a series-clinching 4-1 win at texas.
ARLINGTON, Texas -- — Angels right-hander José Soriano pitched a career-high 7 2/3 innings, pinch-hitter Kevin Pillar had a tiebreaking two-run single for his 1,000th career hit and Los Angeles got a series-clinching 4-1 victory over the Texas Rangers on Sunday.
The Angels (18-29) took two of three over the reigning World Series champions in Ron Washington's first series in Texas as a visiting manager. He is the Rangers' winningest manager with his 664 wins and two American League pennants from 2007-14.
“A win against anyone is a confidence boost, especially for us,” said Washington, whose young Angels got only their third series victory this year. “We played good baseball, and all we want to do is keep playing good baseball. If we do that, we’ll be OK each day we play.”
Corey Seager homered for the Rangers (24-24), who have lost eight of their past 11 games.
Soriano (2-4), who struck out five and walked two, got the first two outs of the eighth, and then a visit from Washington after his first pitch to Seager was a ball.
“He told me I trust myself," Soriano said through a translator. “Trust my pitching ... Don’t try to do too much.”
Washington went back out to make a change after Seager walked and Nathaniel Lowe singled.
“We certainly needed that type of outing for us to come away with a win today, and he gave it to us. He stood out there all the way to the eighth inning,” Washington said of Soriano. “He really wanted to keep the ball, but it just didn’t work out for him to make it through. But I tell you what, it was an outstanding outing.”
Luis Garcia worked around a single to finish the eighth, then worked the ninth for his second save.
The Angels had the based loaded and had already tied the game in the seventh when Pillar lined a single to center field. He then scored on Luis Rengifo 's triple.
Rangers starter Michael Lorenzen (2-3) struck out six walked three and allowed three hits. The right-hander departed with two on and one out, and reliever Jonathan Hernández then walked a batter and gave up a tying RBI single to Mickey Moniak on a bloop that dropped barely fair behind third base before Pillar pinch-hit for No. 9 batter Kyren Paris .
“Both (starters) really pitched well. Their guy and he had great stuff,” Rangers manager Bruce Bochy said. “Michael just worked pretty hard there. ... They get a break on a bloop there that just hit by the line and things kind of unraveled there.”
TRAINER’S ROOM
Angels: C Matt Thaiss got shaken up twice while trying to bunt in the seventh inning. When he squared the first time, he fouled off the pitch, which pushed the knob of the bat into his stomach. A couple of pitches later, Thaiss tried to bunt again, and stung his left hand on another foul ball. Both times he momentarily walked away from the plate. He eventually struck out, but stayed in the game.
Rangers: Adolis García was scratched from the starting lineup as the DH and got an MRI on his right forearm, a day after a collision in the outfield with second baseman Marcus Semien while both All-Star players went after a popup. Bochy, without elaborating, said afterward that the MRI looked “pretty good.” ... OF Evan Carter started for the first time in 10 games after dealing with lower back stiffness. He missed five games in a row before pinch-hitting in the first two games against the Angels, when he didn't start against left-handers.
Angels: Stay in Texas to open a three-game series Monday night at Houston, with left-hander Reid Detmers (3-4, 5.19 ERA) starting for Los Angeles.
Rangers: An off day Monday before Jon Gray (2-1, 2.08) starts the opener of a three-game interleague series at Philadelphia. ------
AP MLB: https://apnews.com/hub/mlb
LAA win 2-1
Game information.
- Home Plate Umpire - Jeremy Riggs
- First Base Umpire - Ramon De Jesus
- Second Base Umpire - Adrian Johnson
- Third Base Umpire - Junior Valentine
2024 American League West Standings
Padres' xander bogaerts has fractured shoulder, lands on il, cole ragans allows 1 hit, strikes out 12 in royals' 8-3 win over tigers, rangers dane dunning returns from il, to start vs. phillies.
- Terms of Use
- Privacy Policy
- Your US State Privacy Rights
- Children's Online Privacy Policy
- Interest-Based Ads
- About Nielsen Measurement
- Do Not Sell or Share My Personal Information
- Disney Ad Sales Site
- Work for ESPN
- Corrections
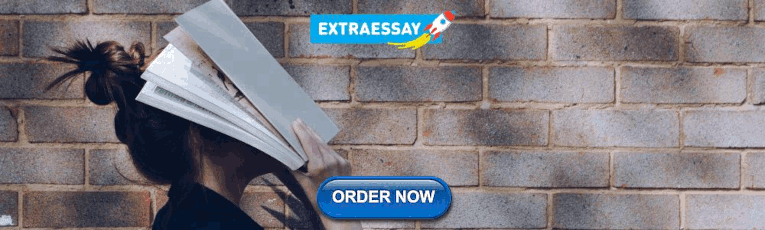
IMAGES
VIDEO
COMMENTS
This report summarises the analysis of the Antarctica land ice mass series, and the process followed to identify suitable ARIMA (p,d,q) models as part of Assignment 2 for Time Series Analysis. The report covers analysis of the dataset, addressing non-stationarity, and using model specification tools to suggest possible ARIMA models.
Password. Forgot your password? Sign InCancel. RPubs. by RStudio. Sign inRegister. Time Series Analysis - Assignment 2. by James Angus. Last updated11 months ago.
These involve a mix of pen-and-paper and computer exercises. You may use any programming language you choose (R, Splus, Matlab, python). Midterm Exams (30%): scheduled for October 7 and November 9, at the lecture. Project (10%): Analysis of a data set that you choose. Final Exam (35%): scheduled for Friday, December 17.
A course in Time Series Analysis Suhasini Subba Rao Email: [email protected] August 29, 2022
2. Objectives of time series analysis. Examples. 3. Overview of the course. 4. Time series models. 5. Time series modelling: Chasing stationarity. 1. ... assignment will involve analysis of a data set that you choose. Midterm Exam (25%): scheduled for October 16, at the lecture.
ECOM30004/90004 - TIME SERIES ANALYSIS AND FORECASTING Second Semester, 2023 Assignment 2 Due date and time: Wednesday 30 August, 11:00AM. Every mark should be some multiple of 0. Assignment Tasks and Questions. There are two exercises in this assignment: altogether six tasks and questions, for 1 or 2 marks (%) each.
Time Series Analysis STAT 4390/5390 OCEAN 4390/5390 Assignment 2: Due 23 January, 2007 1. Read Section 1.6 of Shumway and Stoffer (p29 to p34). ... 4.1, 4.2 and 4.3 (p174 to 187). 2. Question 1.20 (p 44 of Shumway and Stoffer). 3. The SOI (Southern Oscillation Index) time series of monthly values dis-cussed in Shumway and Stoffer has a ...
Time Series Analysis. Menu. More Info Syllabus Calendar Instructor Insights Readings ... FILES 1 Stationarity, lag operator, ARMA, and covariance structure Lecture 1 Notes (PDF) 2 Limit theorems, OLS, and HAC Lecture 2 Notes (PDF) 3 More HAC and intro to spectrum Lecture 3 Notes (PDF) ... assignment Problem Sets. co_present Instructor Insights ...
A time series is said to be stationary if its statistical properties such as mean, variance remain constant over time. As most time series models work on the assumption that the time series are stationary, it is important to validate that hypothesis. For general time series datasets, if it shows a particular behavior over time, there is a very ...
In time series analysis, analysts record data points at consistent intervals over a set period of time rather than just recording the data points intermittently or randomly. However, this type of analysis is not merely the act of collecting data over time. What sets time series data apart from other data is that the analysis can show how ...
Assignment-2 Time Series. by ankit munot. Last updated almost 4 years ago.
Concatenation, Five-number summary, Standard Deviation • 5 minutes. Histogram in R • 6 minutes. Scatterplot in R • 3 minutes. Review of Basic Statistics I - Simple Linear Regression • 6 minutes. Reviewing Basic Statistics II More Linear Regression • 8 minutes. Reviewing Basic Statistics III - Inference • 12 minutes.
2. Demonstrate familiarity with a range of examples for the different topics covered in the course. 3. Demonstrate an advanced understanding the underlying concepts in the time series and frequency domains. 4. Apply ideas to real time series data and interpret outcomes of analyses. 5. Demonstrate graduade-level skills in communicating ...
Problem Sets. The problem sets will emphasize different aspects of the course, including theory and estimation procedures we discuss in class. I strongly believe that the best way to learn the techniques is by doing. Every problem set will include an applied task that may include computer programming. I do not restrict you in your choice of ...
1 MATH5845: Time Series Analysis term 2, 2023 Assignment 2 Due: Thursday July 27 by 23:59 pm via Moodle submission Instructions: • This assignment contains 3 questions and worth a total of 50 points which will count towards 20% of the final mark for the subject. • You have two option to submit this assignment: 1. Convert the R Markdown file (with the answers to all of the questions) into a ...
Time series analysis is a specialized branch of statistics focused on studying data points collected or recorded sequentially over time. It incorporates various techniques and methodologies to identify patterns, forecast future data points, and make informed decisions based on temporal relationships among variables.
Or copy & paste this link into an email or IM:
Photo by Ales Krivec on Unsplash Introduction. Time series analysis certainly represents one of the most widespread topics in the field of data science and machine learning: whether predicting financial events, energy consumption, product sales or stock market trends, this field has always been of great interest to businesses.
Importance of Time Series Analysis. Predict Future Trends: Time series analysis enables the prediction of future trends, allowing businesses to anticipate market demand, stock prices, and other key variables, facilitating proactive decision-making. Detect Patterns and Anomalies: By examining sequential data points, time series analysis helps detect recurring patterns and anomalies, providing ...
2 FRM9649 MAY/JUNE 2019 TIME SERIES ANALYSIS AND FORECASTING (FRM9649) ASSIGNMENT 2 Due Date: 13 June 2019 by 23:59 Instruction(s): • Answer ALL the questions. • Each question is out of five marks but the amount of work per question is different. • Write your answers legibly (preferably typed, but I won't hold it against you if not typed, unless I can't read what you wrote).
The Royals continue their three-game series against the Tigers. On Tuesday, KC right-hander Alec Marsh will start against Detroit hurler Casey Mize. Marsh will look for his fourth win this season.
A smoothed map of blended land and sea surface temperature anomalies is also available.. South America had its warmest April while Europe had its second-warmest and Africa its fourth-warmest April on record. In Germany April 2024 was the 14th-warmest April since records began in 1881, 1.1°C warmer than the 1991-2020 average, 2.7°C warmer than the 1961-1990 average.
The setwd () method is used to set the working directory, and R's fetching the csv function is used as to read the data as Stock.csv The output tells us that the dataset is a dataframe with 144 observations with having 2 variables. We rename this variable to Stocks to make it clearer.
Expert recap and game analysis of the Los Angeles Angels vs. Texas Rangers MLB game from May 19, 2024 on ESPN. ... Soriano's career-long start and Pillar's pinch hit get Angels a series-clinching ...