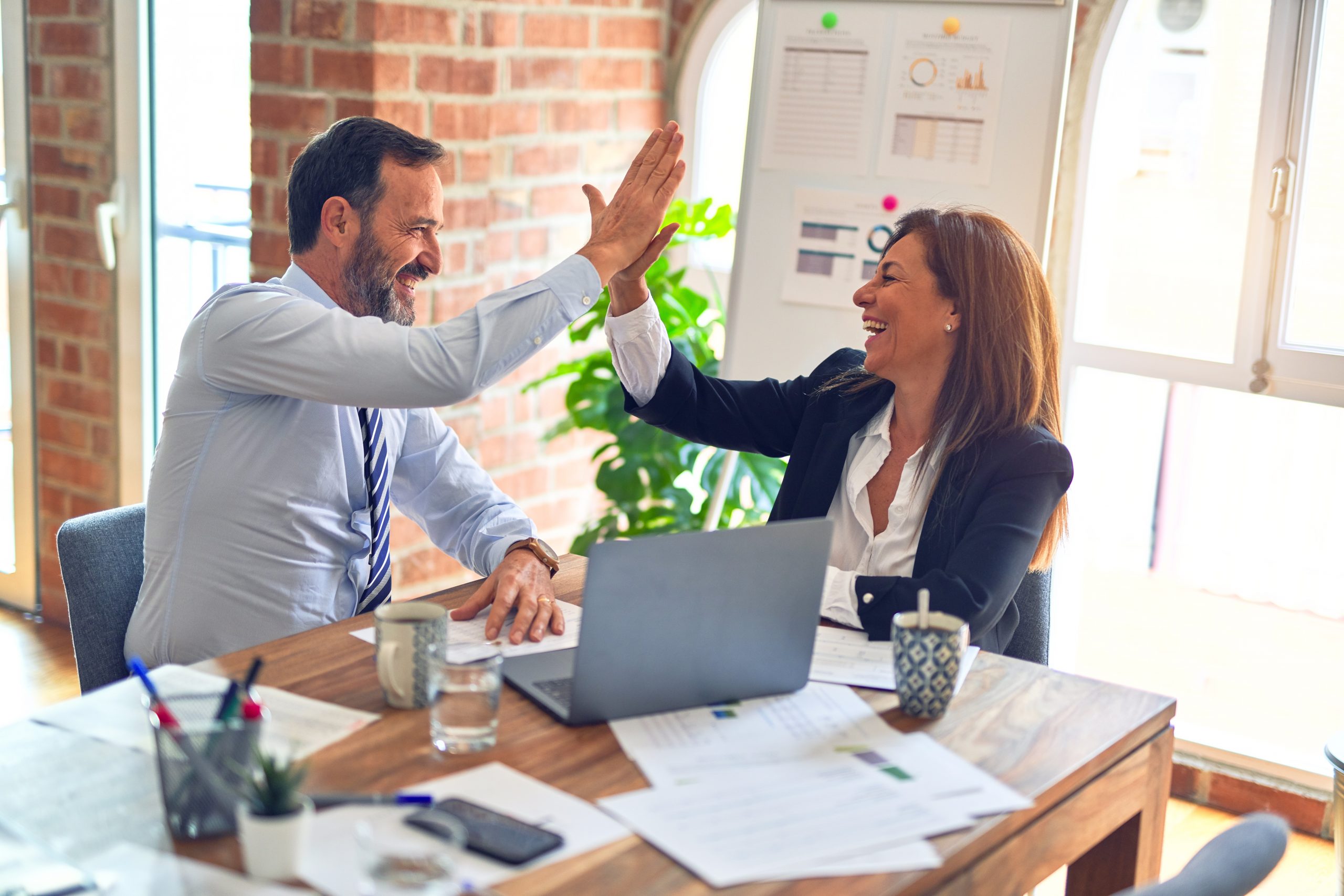
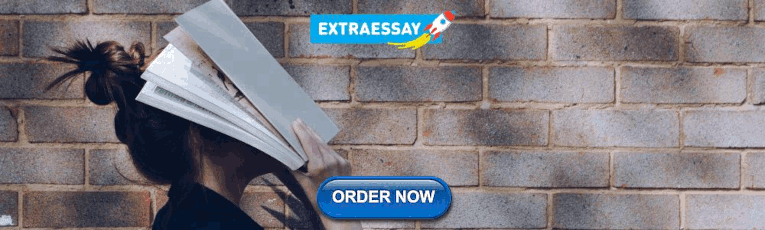
Assignable Cause
Published: November 7, 2018 by Ken Feldman
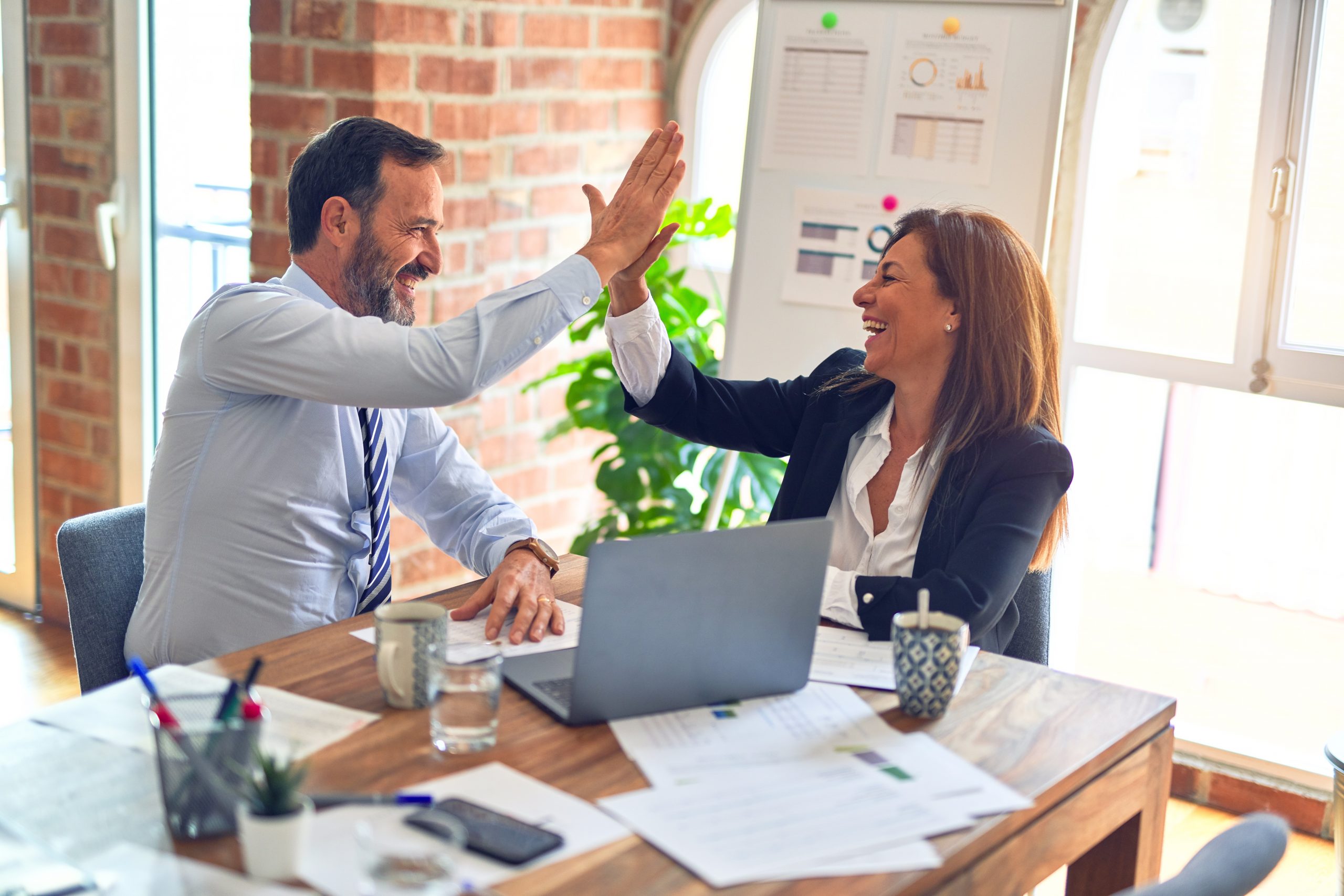
Assignable cause, also known as a special cause, is one of the two types of variation a control chart is designed to identify. Let’s define what an assignable cause variation is and contrast it with common cause variation. We will explore how to know if your control is signaling an assignable cause and how to react if it is.
Overview: What is an assignable cause?
A control chart identifies two different types of variation: common cause variation (random variation resulting from your process components or 6Ms ) and assignable or special cause variation.
Assignable cause variation is present when your control chart shows plotted points outside the control limits or a non-random pattern of variation. Since special cause variation is unexpected and due to some factor other than randomness, you should be able to assign a reason or cause to it.
When your control chart signals assignable cause variation, your process variable is said to be out of control, or unstable. Assignable cause variation signals can be identified by use of the Western Electric rules, which include:
- One point outside of the upper control limit or lower control limit
- A trend of 6 or 7 consecutive points increasing or decreasing
- A cycle or repeating pattern
- A run of 8 or more consecutive points on either side of the average or center line.
Assignable cause variation can be attributed to a defect, fault, mistake, delay, breakdown, accident, and/or shortage in the process. Or it can be a result of some unique combination of factors coming together to actually improve the process. When assignable causes are present, your process is unpredictable. The proper action and response is to search for and identify the specific assignable cause. If your process was improved as a result of your assignable cause, then incorporate it so that the cause is retained and improvement maintained. If your process was harmed by the assignable cause, then seek to eliminate it.
3 benefits of an assignable cause
Assignable causes can be good or bad. They are signals that something unexpected happened. Listen to the signal.
1. Signals something has happened
Special or assignable cause variation signals that something unexpected and non-random has occurred in your process.
2. Specific cause
By investigating and identifying the specific cause of your signal, you can narrow in on your next steps for bringing the process back into control.
3. Can become common cause variation
Good news! You found that your assignable cause for lowered production was due to a power outage. Unfortunately, you may not be able to stop power outages in your community. If nothing is done, your assignable cause becomes a common cause.
You might not be able to stop power outages, but could you install a back-up generator? Then, if the generator doesn’t kick on, you will have an assignable cause you can do something about.
Why is an assignable cause important to understand?
Interpreting what an assignable cause tells you is important to understand.
Provides direction for action
Since an assignable cause can be a signal of something good or bad, you need to understand the different actions. Don’t ignore special or assignable causes.
Not every unusual point has an assignable cause
While at your favorite casino, you may throw a pair of dice at the craps table. Is there an assignable cause for throwing an 11 or a 10, or is it random variation? No, you would expect the process of rolling a fair pair of dice to show 10s and 11s. What about a 13? That would be unexpected and probably the result of something unusual happening with the dice. The same is true for your process. Don’t assume an assignable special cause unless your control chart signals it.
Useful for determining whether your improvements worked
When you improve the process, your control chart should send signals of special cause variation — hopefully in the right direction. If you can link that signal to the specific assignable cause of your improvement, then you know it worked.
An industry example of an assignable cause
The accounts receivable department of a retail chain started to get complaints from its customers about overbilling. Fortunately, the manager of the department had participated in the company’s Lean Six Sigma training and had been using a control chart for errors.
Upon closer review, she noticed that errors seemed to occur more on Fridays than the rest of the week. In fact, the chart showed that almost every Friday, the data points were outside the upper control limit. She was concerned that nobody was identifying that as a signal of special cause.
She put together a small team of clerks to identify why this was happening and whether there was an assignable reason or cause for it. The assignable cause was determined to be the extra work load on Fridays.
The team recommended a change in procedure to better balance the workload during the week. Continued monitoring showed the problem was resolved. She also held an all-hands meeting to discuss the importance of not ignoring signals of special cause variation and the need to seek out an assignable cause and take the appropriate action.
3 best practices when thinking about an assignable cause
Signals of special cause variation require you to search for and identify the assignable cause.
1. Document your search
If you’ve identified the assignable cause, document everything. If this cause happens again in the future, people will have some background to act quickly and eliminate/incorporate any actions.
2. Quickly identify the cause
Time is of the essence. If the cause is resulting in a deteriorating process, act quickly to identify and eliminate the cause. The recommendation is the same if your cause made the process better, otherwise, whatever happened to improve the process will be lost as time goes by.
3. Don’t ignore signals of assignable cause
Even if you get a single signal of special cause, search for the assignable cause. You may choose not to take any action in the event it is a fleeting cause, but at least try to identify the assignable cause.
Frequently Asked Questions (FAQ) about an assignable cause
1. is an assignable cause always bad .
No. It is an indication that something unexpected happened in your process. It could be a good or bad thing. In either case, search for and identify the assignable cause and take the appropriate action.
2. What are some sources of an assignable cause?
Some sources may be your process components such as people, methods, environment, equipment, materials, or information. Your process variation can come from these items and can be the assignable cause of a signal of special cause variation.
3. How do I tell if I should look for an assignable cause?
Control charts were developed to distinguish between common and special cause variation. If they signal special cause variation in your process, seek out an assignable cause and take the appropriate action of either eliminating or incorporating your assignable cause.
Final thoughts on an assignable cause
All processes will exhibit two types of variation. Common cause variation is random, expected, and a result of variation in the process components. Special cause variation is non-random, unexpected, and a result of a specific assignable cause.
If you get a signal of special cause variation, you need to search for and identify the assignable cause. Once found, you will either seek to incorporate or eliminate the cause depending on whether the cause improved or hurt your process.
About the Author
Ken Feldman
Monday, August 17, 2015
Chance & assignable causes of variation.
Links to all courses Variation in quality of manufactured product in the respective process in industry is inherent & evitable. These variations are broadly classified as- i) Chance / Non assignable causes ii) Assignable causes i) Chance Causes: In any manufacturing process, it is not possible to produce goods of exactly the same quality. Variation is inevitable. Certain small variation is natural to the process, being due to chance causes and cannot be prevented. This variation is therefore called allowable . ii) Assignable Causes: This type of variation attributed to any production process is due to non-random or so called assignable causes and is termed as preventable variation . Assignable causes may creep in at any stage of the process, right from the arrival of the raw materials to the final delivery of goods. Some of the important factors of assignable causes of variation are - i) Substandard or defective raw materials ii) New techniques or operation iii) Negligence of the operators iv) Wrong or improper handling of machines v) Faulty equipment vi) Unskilled or inexperienced technical staff and so on. These causes can be identified and eliminated and are to discovered in a production process before the production becomes defective. SQC is a productivity enhancing & regulating technique ( PERT ) with three factors- i) Management ii) Methods iii) Mathematics Here, control is two-fold- controlling the process ( process control ) & controlling the finished products (products control).
About আব্দুল্যাহ আদিল মাহমুদ
2 comments:.
Totally awesome posting! Loads of valuable data and motivation, both of which we all need!Relay welcome your work. maggots in mouth treatment
Bishwo.com on Facebook
Popular Articles
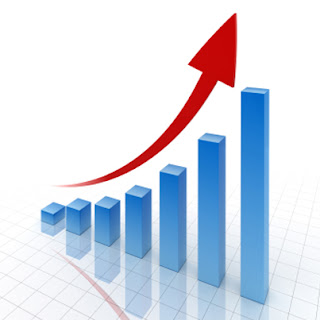
Like on Facebook
Join our newsletter, portal archive, basics of math, contact form.
- Privacy Policy
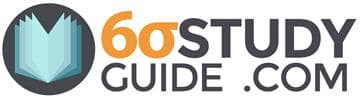
Six Sigma Study Guide
Study notes and guides for Six Sigma certification tests
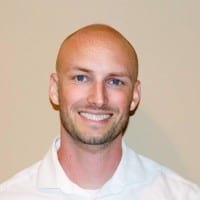
Statistical Process Control (SPC)
Posted by Ted Hessing
Statistical Process Control (SPC) is a statistical method to measure, monitor, and control a process. It is a scientific visual method to monitor, control, and improve the process by eliminating special cause variations in a process.
History of Statistical Process Control (SPC)
SPC has been used in Western industries since 1980, but it started in America’s twenties. Walter A. Shewhart developed the control chart and the concept that a process could be in statistical control in 1924 at Bell Telephone Laboratories, USA. Likewise, the SPC concepts were included in the management philosophy by Dr. W.E. Deming just before World War II. However, SPC became famous after Japanese industries implemented the concepts to compete with Western industries.
Meaning of SPC
- Statistics : Statistics is a science that deals with the collection, summarization, analysis, and drawing of information from the data.
- Process: It converts input resources into the desired output (goods or services) with a combination of people, materials, methods, and machines, as well as measurements.
- Control: System, policies, and procedures in place so the overall output meets the requirement.
Why use Statistical Process Control
Today companies face increasing competition and operational costs, including raw materials increasing. So, it is beneficial for organizations to have control over their operation.
Organizations must try to continuously improve quality, efficiency, and cost reduction. Many organizations still follow inspection after production for quality-related issues.
SPC helps companies move towards prevention-based quality control instead of detection-based quality controls. By monitoring SPC graphs, organizations can easily predict the behavior of the process.
Statistical Process Control Benefits
- Reduce scrap and rework
- Increase productivity
- Improve overall quality
- Match process capability to product requirement
- Continuously monitor processes to maintain control
- Provide data to support decision-making
- Streamline the process
- Increase in product reliability
- Opportunity for company-wide improvements
Statistical Process Control Objective
SPC focuses on optimizing continuous improvement by using statistical tools to analyze data, make inferences about process behavior, and then make appropriate decisions.
The basic assumption of SPC is that all processes are generally subject to variation. To that end, Variation measures how data are spread around the central tendency. Moreover, variation may be classified as one of two types, random or chance cause variation and assignable cause variation.
Common Cause: A cause of variation in the process is due to chance but not assignable to any factor. It is the variation that is inherent in the process. Likewise, a process under the influence of a common cause will always be stable and predictable.
Assignable Cause: It is also known as “special cause.” Therefore, the variation in a process that is not due to chance can be identified and eliminated. In this case, a process under the influence of a special cause will not be stable and predictable.
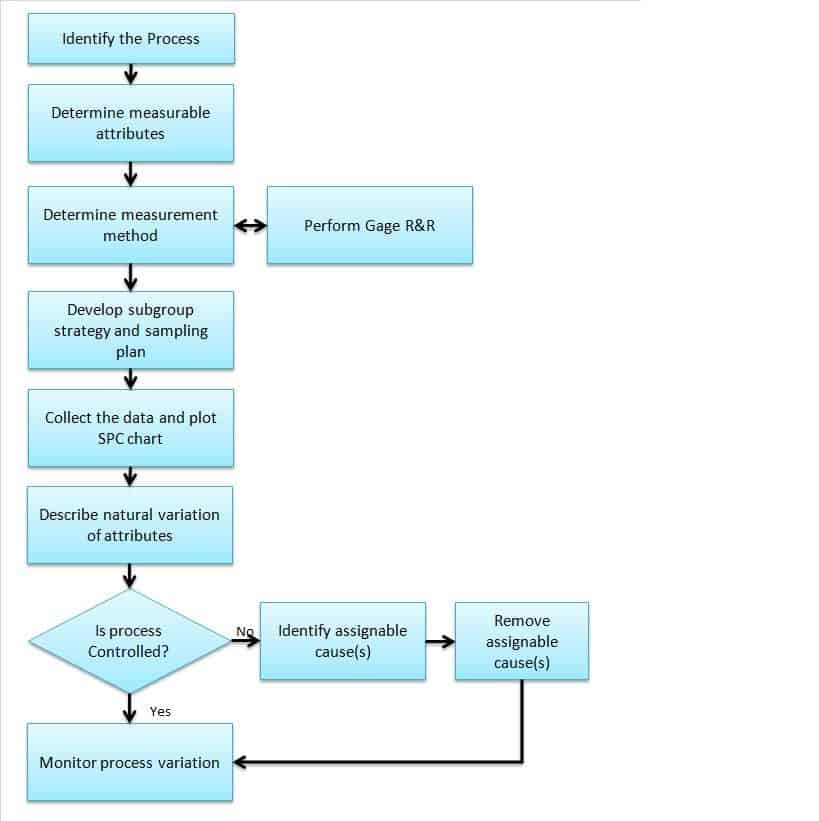
How to Perform SPC
1. identify the processes: .
Firstly, identify the key process that impacts the output of the product or the process that is very critical to the customer. For example, plate thickness impacts the product’s performance in a manufacturing company, then consider the plate manufacturing process.
2. Determine measurable attributes of the process:
Secondly, identify the attributes that need to be measured during production. For example, consider the plate thickness as a measurable attribute.
3. Determine the measurement method and also perform Gage R&R :
Thirdly, create a measurement method work instructions or procedure, including the measuring instrument. For example, consider a thickness gauge to measure the thickness and create an appropriate measuring procedure. Perform Gage Repeatability and Reproducibility (Gage R & R) to define the amount of variation in the measurement data due to the measurement system.
4. Develop a subgroup strategy and sampling plan:
Fourthly, determine the subgroup size based on the product’s criticality and determine the sampling size and frequency. For example, collect 20 sets of plate thicknesses in a time sequence with a subgroup size of 4.
5. Collect the data and plot the SPC chart:
Then, collect the data per sample size and select an appropriate SPC chart based on data type (Continuous or Discrete) and subgroup size. For Example, for plate thicknesses with a subgroup size of 4, select Xbar -R chart.
6. Describe the natural variation of attributes:
Next, calculate the control limits. From the above example, calculate the upper control limit (UCL) and lower control limit (LCL) for both Xbar Ranges .
7. Monitor process variation:
Finally, interpret the control chart and check whether any point is out of control and the pattern.
Example: Check the Xbar R chart if the process is not in control, then identify the assignable cause(s) and address the issue. This is an ongoing process, so monitor the process variation.
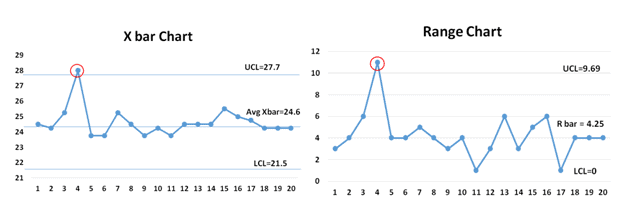
Additional Statistical Process Control Resources
Control limits are the voice of the process (different from specification limits , which are the customer’s voice.) They show what the process is doing and act as a guide for what it should be doing. Control limits also indicate that a process event or measurement is likely to fall within that limit.
Control charts : A Control chart is one of the primary statistical process control techniques (SPC). The control chart is a graphical display of quality characteristics that are measured or computed from a sample versus the sample number or time. Furthermore, the control chart contains a center line that represents the average value of the quality characteristics and two other horizontal lines known as the upper control limit (UCL) and lower control limit (LCL)
The selection of an appropriate control chart is very important in control chart mapping. Otherwise, it ends up with inaccurate control limits for the data. The control chart selection depends on the data type: Continuous or Discrete.
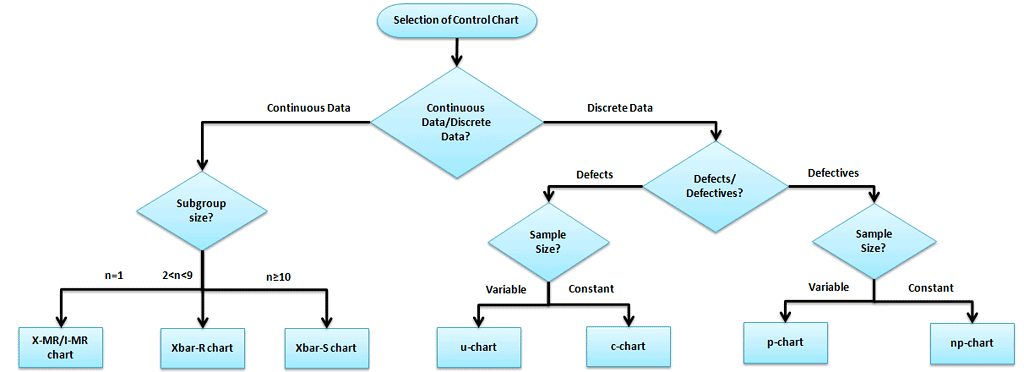
Variable (Continuous) Control Charts
Measure the output on a continuous scale. It is possible to measure the quality characteristics of a product.
X bar – R Charts (when data is readily available)
- X bar R chart is used to monitor the process performance of continuous data and the data to be collected in subgroups at set periods. In other words, two plots monitor the process mean and the process variation over time.
Run Charts (limited single-point data)
- A run chart displays observed data as they evolve over time. Just a basic graph that displays data values in a time order. It can be useful for identifying trends or shifts in the process but also allows you to test for randomness in the process.
X – MR Charts (I – MR, individual moving range)
- An I-MR chart is also known as an X-MR chart. An Individual moving range (I-MR ) chart is used when continuous data is not collected in subgroups. In other words, collect a single observation at a time. An I-MR chart provides process variation over time in a graphical method.
X bar – S Charts (when Sigma is readily available)
- X Bar S charts often used control charts to examine the process mean and standard deviation over time. These charts are used when the subgroups have large sample sizes, and the S chart provides a better understanding of the spread of subgroup data than the range.
- The EWMA – Exponentially Weighted Moving Average chart is used in statistical process control to monitor variables (or attributes that act like variables). Additionally, it makes use of the entire history of a given output. This differs from other control charts that treat each data point individually.
Attribute(Discrete) Control Charts :
The output is a decision or counting. It is not possible to measure the quality characteristics of a product. In other words, it is based on the visual inspection like good or bad, fail or pass, accept or reject.
- p Charts : (for defectives – sample size varies) – Use P chart when the data are the fraction defective of some set of the process output. It may also be shown as a percentage of defective. The points plotted on the p-chart are the fraction of non-confirming units or defective pieces found in n samples.
- np Charts (for defectives – sample size fixed) – Use an np-chart when the data is collected in subgroups that are the same size. Np-charts show how the process changes over time, measured by the number of nonconforming items (defectives) produced. In other words, the process describes pass or fail, yes or no.
- c Charts (for defects – sample size fixed) – Use c charts when the data are concerned with the number of defects in a product. The number of defects collected for the area of opportunity in each subgroup.
- u Charts (for defects – sample size varies) – A u chart is an attribute control chart that displays how the frequency of defects or nonconformities changes over time for a process or system. The number of defects collected for the area of opportunity in each subgroup.
Statistical Process Control Links
Great decision matrix here: https://www.moresteam.com/toolbox/statistical-process-control-spc.cfm
Statistical Process Control Videos
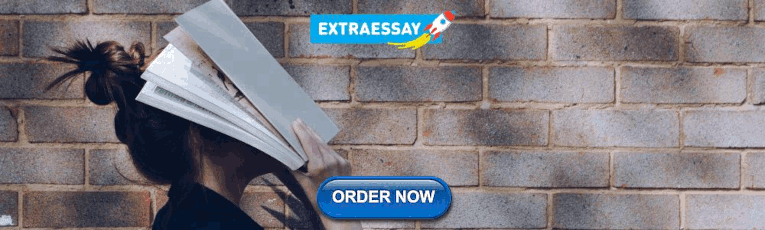
Six Sigma Green Belt Statistical Process Control Questions
Question: In the Control Phase of an LSS project, a Belt will identify key metrics that can be monitored and analyzed to give an indication that a process may be moving towards an out-of-spec condition. When he applies this approach, he is using __________________.
(A) Poisson Derivatives (B) Inferential Statistics (C) Kanban Analysis (D) Statistical Process Control
Unlock Additional Members-only Content!
Comments (11).
How to conduct SPC FOR BATCH ORDER TYPE PRODUCTION?
Try making a control chart depending on what your quality measures are.
Could you direct me to the AIAG information you mentioned in the control phase quiz? I followed the link provided but do not see the answer. I tried searching in the search bar but no results.
Larry, you don’t really need the Automotive Industry Action Group (AIAG) information. By definition out of control could be considered a certain number of points in a row on one side of the X Bar Bar or R in a control chart or a certain number of points in a row that are consistently increasing or decreasing.
I’ve seen some standards list 5 points and others list 7. The key to the question is knowing that a process shift or trend has begun if you see that behavior emerged.
I’ve updated the answer walkthrough with this.
You can find AIAG’s PDF on Academia.com here: https://www.academia.edu/7829906/AIAG_Statistical_Process_Control_SPC_2nd_Edition
Note from Admin: This question has been moved to the private member’s forum here.
How to perform SPC for Angular Dimension??
I’ve never considered it, Ranjan. Perhaps this helps?
can u explain what the difference between SPC and control charts as it is mentioned that to check causes of variation in process we use SPC that is either from natural or random causes but then why we use control charts
Think of SPC as a framework and Control charts as a tool you would use within the framework.
Control charts give you an excellent visual representation of what is going on with your process.
hello when is this article published originally
My first publication was on June 27, 2014. There have been several revisions since that time.
Leave a Reply Cancel reply
Your email address will not be published. Required fields are marked *
This site uses Akismet to reduce spam. Learn how your comment data is processed .
Insert/edit link
Enter the destination URL
Or link to existing content
Module 8. Statistical quality control
BASIC CONCEPTS OF STATSITICAL QUALITY CONTROL
26.1 Introduction
From the early days of industrial production, the emphasis had been on turning out products of uniform quality by ensuring use of similar raw materials, identical machines, and proper training of the operators. Inspite of these efforts, the causes of irregularity often crept in inadvertently. Besides, the men and machines are not infallible and give rise to the variation in the quality of the product. For keeping this variation within limits, in earlier days, the method used was 100 per cent inspection at various stages of manufacturing.
It was in 1924 that Dr. W.A. Shewhart of Bell Telephone Laboratories, USA developed a method based on statistical principles for controlling quality of products during the manufacturing and thus eliminating the need for 100 per cent inspection. This technique which is meant to be an integral part of any production process, does not provide an automatic corrective action but acts as sensor and signal for the variation in the quality. Therefore, the effectiveness of this method depends on the promptness with which a necessary corrective action is carried out on the process. This technique has since been developed by adding to its armory more and more charts, as a result of its extensive use in the industry during and after the Second World War. In this lesson various terms used in the context of Statistical Quality Control (SQC) have been illustrated.
26.2 Definitions of Various Terms Involved in Statistical Quality Control
The following terms are used to understand the concept of Statistical Quality Control
26.2.1 Quality
The most important word in the term ‘Statistical Quality Control’ is quality. By ‘Quality’ we mean an attribute of the product that determines its fitness for use. Quality can be further defined as “Composite product characteristics of engineering and manufacture that determine the degree to which the product in use will meet the expectations of the customer at reasonable cost.” Quality means conformity with certain prescribed standards in terms of size, weight, strength, colour , taste, package etc.
26.2.2 Quality characteristics
Quality of a product (or service) depends upon the various characteristics that a product possesses. For example, the Kulfi we buy should have the following characteristics.
(a) TS (b) Sugar (c) Flavour (d) Body & Texture.
All these individual characteristics constitute the quality of Kulfi . Of course, some of them are important (critical) without which the Kulfi is not acceptable. For example Minimum TS, Sugar, Body and Texture score is important. However, other characteristics such as Colour and Flavour may not be so important. The quality characteristics may be defined as the “distinguishing” factor of the product in the appearance, performance, length of life, dependability, reliability, durability, maintainability, taste, colour , usefulness etc. Control of these quality characteristics in turn means the control of the quality of product.
26.2.3 Types of characteristics
There are two types of characteristics viz., variable characteristics and attribute characteristics.
26.2.3.1 Variable characteristic
Whenever a record is made of an actual measured quality characteristic, such as dimension expressed in mm, cm etc. quality is said to be expressed by variables. This type of quality characteristics includes e.g., dimension (length, height, thickness etc.),hardness, temperature, tensile strength, weight, moisture percent, yield percent, fat percent etc.
26.2.3.2 Attribute characteristic
Whenever a record shows only the number of articles conforming and the number of articles failing to conform to any specified requirements, it is said to be a record of data by ‘attributes’. These include:
· Things judged by visual examination
· Conformance judged by gauges
· Number of defects in a given surface area etc.
26.2.4 Control
Control means organizing the following steps:
· Setting up standards of performance.
· Comparing the actual observations against the standards.
· Taking corrective action whenever necessary.
· Modifying the standards if necessary.
26.2.5 Quality control
Quality control is a powerful productivity technique for effective diagnosis of lack of quality (or conformity to set standards) in any of the materials, processes, machines or end products. It is essential that the end products possess the qualities that the consumer expects of them, for the progress of the industry depends on the successful marketing of products. Quality control ensures this by insisting on quality specifications all along the line from the arrival of materials through each of their processing to the final delivery of goods.Quality control, therefore, covers all the factors and processes of production which may be broadly classified as follows:
· Quality of materials : Material of good quality will result in smooth processing there by reducing the waste and increasing the output. It will also give better finish to end products.
· Quality of manpower : Trained and qualified personnel will give increased efficiency due to the better quality production through the application of skill and also reduce production cost and waste.
· Quality of machines : Better quality equipment will result in efficient working due to lack or scarcity of break downs thus reducing the cost of defectives.
· Quality of Management : A good management is imperative for increase in efficiency, harmony in relations, growth of business and markets.
26.2.6 Chance and assignable causes of variation
Variation in the quality of the manufactured product in the repetitive process in the industry is inherent and inevitable. These variations are broadly classified as being due to two causes viz., ( i ) chance causes, and (ii) assignable causes.
26.2.6.1 Chance causes
Some “Stable pattern of variation” or “a constant cause system” is inherent in any particular scheme of production and inspection. This pattern results from many minor causes that behave in a random manner. The variation due to these causes is beyond the control of human being and cannot be prevented or eliminated under any circumstance. Such type of variation has got to be allowed within the stable pattern, usually termed as Allowable Variation. The range of such variation is known as natural tolerance of the process.
26.2.6.2 Assignable causes
The second type of variation attributed to any production process is due to non-random or the so called assignable causes and is termed as Preventable Variation. The assignable causes may creep in at any stage of the process, right from the arrival of raw materials to the final delivery of the goods.
Some of the important factors of assignable causes of variation are substandard or defective raw material, new techniques or operations, negligence of the operators, wrong or improper handling of machines, faulty equipment, unskilled or inexperienced technical staff and so on. These causes can be identified and eliminated and are to be discovered in a production process before it goes wrong i.e., before the production becomes defective.
26.3 Statistical Quality Control
By Statistical Quality Control (SQC) we mean the various statistical methods used for the maintenance of quality in a continuous flow of manufactured goods. The main purpose of SQC is to devise statistical techniques which help us in separating the assignable causes from chance causes of variation thus enabling us to take remedial action wherever assignable causes are present. The elimination of assignable causes of erratic fluctuations is described as bringing a process under control. A production process is said to be in a state of statistical control if it is governed by chance causes alone, in the absence of assignable causes of variation.
In the above problem, the main aim is to control the manufacturing process so that the proportion of defective items is not excessively large. This is known as ‘ Process Control’ . In another type of problem we want to ensure that lots of manufactured goods do not contain an excessively large proportion of defective items. This is known as ‘ Product or Lot Control ’. The process control and product control are two distinct problems, because even when the process is in control, so that the proportion of defective products for the entire output over a long period will not be large, an individual lot of items may not be of satisfactory quality. Process Control is achieved mainly through the technique of ‘ Control Charts ’ whereas Product Control is achieved through ‘ Sampling Inspection’ .
26.4 Stages of Production Process
Before production starts, a decision is necessary as to what is to be made. Next comes the actual manufacturing of the product. Finally it must be determined whether the product manufactured is what was intended. It is therefore necessary that quality of manufactured product may be looked at in terms of three functions of specification, production and inspection.
26.4.1 Specification
This tells us what is to be produced and of what specification. That is, it gives us dimension and limits within which dimension can vary. These specifications are laid down by the manufacturer.
26.4.2 Production
Here we should look into what we have manufactured and what was intended to.
26.4.3 Inspection
Here we examine with the help of SQC techniques whether the manufactured goods are within the specified limits or whether there is any necessity to widen the specifications or not. So SQC tells us as to what are the capabilities of the production process.
Therefore statistical quality control is considered as a kit of tools, which may influence decisions, related to the functions of specification, production or inspection. The effective use of SQC generally requires cooperation among those responsible for these three different functions or decisions at a higher level than any one of them. For this reason, the techniques should be understood at a management level that encompasses all the three functions.
Quality America Inc.
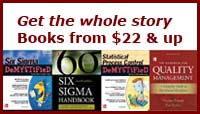
SPC defined
Control Chart Properties
Rational Subgroups
Distributions
Defining Control Limits
Short Run Techniques
Interpretation & Calculations
Attributes Charts
Pareto Charts
X-Bar / Range Charts
X-Bar / Sigma Charts
Individual-X Charts
Moving Average Charts
CuSum Charts
EWMA Charts
Multivariate Control Charts
Histograms, Process Capability
Autocorrelation Charts
Measurement Systems Analysis
Applications
Getting Started with SPC
Key Success Factors for the Implementation of SPC
How to Study Process Capability
Multiple Steam Processes
Analysis of Batch Processes
SPC to Improve Quality, Reduce Cost
Struggling with Independence
SPC Analysis of MLB Home Runs
Use Of SPC To Detect Process Manipulation
Using Data Mining and Knowledge Discovery With SPC
Common and Special Causes of Variation
The following is an excerpt on SPC implementation The Six Sigma Handbook: Fourth Edition by Paul Keller and Thomas Pyzdek (McGraw-Hill, 2014).
Shewhart (1931, 1980) defined control as follows:
A phenomenon will be said to be controlled when, through the use of past experience, we can predict, at least within limits, how the phenomenon may be expected to vary in the future. Here it is understood that prediction within limits means that we can state, at least approximately, the probability that the observed phenomenon will fall w i thin the given limits.
The critical point in this definition is that control is not defined as the complete absence of variation. Control is simply a state where all variation is predictable . A controlled process is not necessarily a sign of good management, nor is an out-of-control process necessarily producing non-conforming product.
In all forms of prediction there is an element of chance. For our purposes, we will call any unknown random cause of variation a chance cause or a common cause , the terms are synonymous and will be used as such. If the influence of any particular chance cause is very small, and if the number of chance causes of variation are very large and relatively constant, we have a situation where the variation is predictable within limits. You can see from the definition above, that a system such as this qualifies as a controlled system. Where Dr. Shewhart used the term chance cause, Dr. W. Edwards Deming coined the term common cause to describe the same phenomenon. Both terms are encountered in practice.
Needless to say, not all phenomena arise from constant systems of common causes. At times, the variation is caused by a source of variation that is not part of the constant system. These sources of variation were called assignable causes by Shewhart, special causes of variation by Dr. Deming. Experience indicates that special causes of variation can usually be found without undue difficulty, especially with easy to use SPC software , leading to a process that is less variable.
Statistical tools are needed to help us effectively identify the effects of special causes of variation. This leads to another definition: Statistical Process Control
Learn more about the SPC principles and tools for process improvement in Statistical Process Control Demystified (2011, McGraw-Hill) by Paul Keller , in his online SPC Concepts short course (only $39 ), or his online SPC certification course ( $350 ) or online Green Belt certification course ( $499 ).
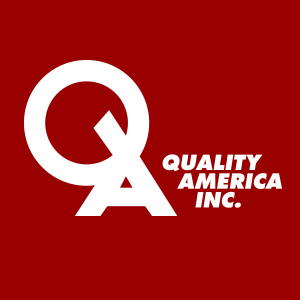
404 Not found
Operations Management: An Integrated Approach, 5th Edition by
Get full access to Operations Management: An Integrated Approach, 5th Edition and 60K+ other titles, with a free 10-day trial of O'Reilly.
There are also live events, courses curated by job role, and more.
SOURCES OF VARIATION: COMMON AND ASSIGNABLE CAUSES
If you look at bottles of a soft drink in a grocery store, you will notice that no two bottles are filled to exactly the same level. Some are filled slightly higher and some slightly lower. Similarly, if you look at blueberry muffins in a bakery, you will notice that some are slightly larger than others and some have more blueberries than others. These types of differences are completely normal. No two products are exactly alike because of slight differences in materials, workers, machines, tools, and other factors. These are called common , or random, causes of variation . Common causes of variation are based on random causes that we cannot identify. These types of variation are unavoidable and are due to slight differences in processing.

Random causes that cannot be identified.
An important task in quality control is to find out the range of natural random variation in a process. For example, if the average bottle of a soft drink called Cocoa Fizz contains 16 ounces of liquid, we may determine that the amount of natural variation is between 15.8 and 16.2 ounces. If this were the case, we would monitor the production process to make sure that the amount stays within this range. If production goes out of this range—bottles are found to contain on average 15.6 ounces—this would lead us to believe that there ...
Get Operations Management: An Integrated Approach, 5th Edition now with the O’Reilly learning platform.
O’Reilly members experience books, live events, courses curated by job role, and more from O’Reilly and nearly 200 top publishers.
Don’t leave empty-handed
Get Mark Richards’s Software Architecture Patterns ebook to better understand how to design components—and how they should interact.
It’s yours, free.
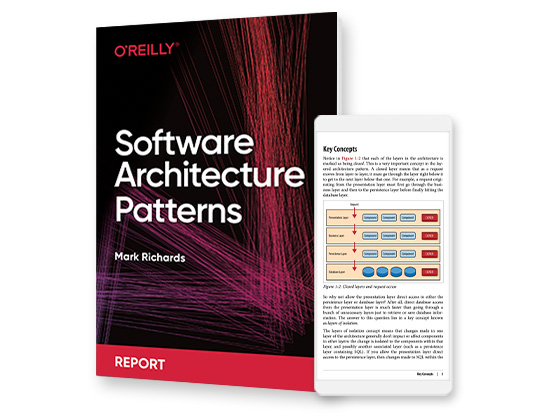
Check it out now on O’Reilly
Dive in for free with a 10-day trial of the O’Reilly learning platform—then explore all the other resources our members count on to build skills and solve problems every day.
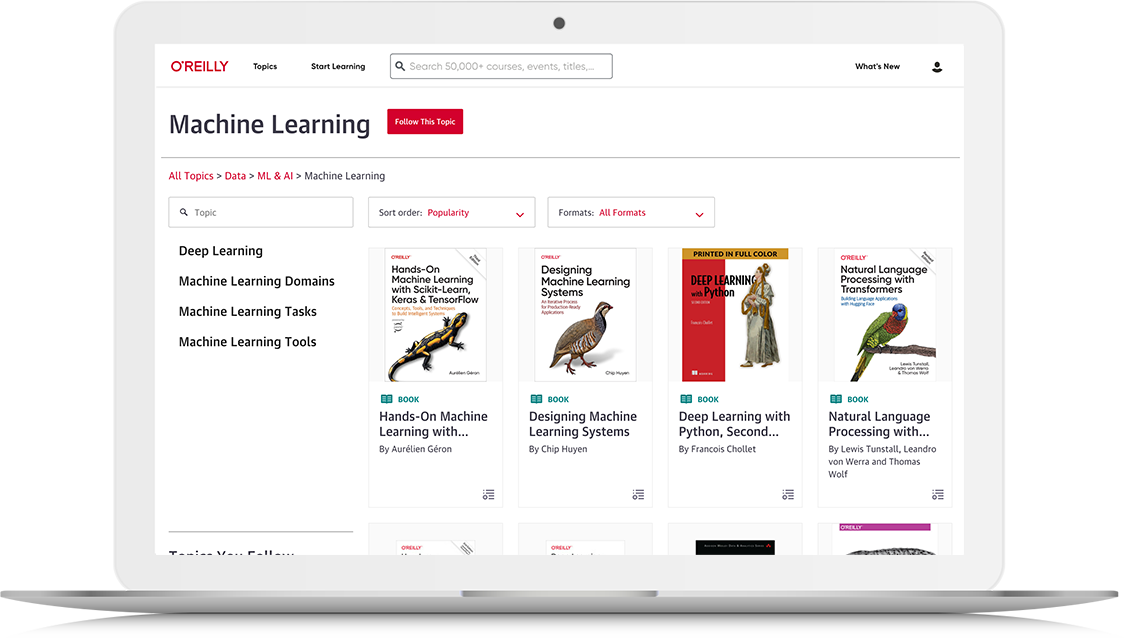

An official website of the United States government
The .gov means it’s official. Federal government websites often end in .gov or .mil. Before sharing sensitive information, make sure you’re on a federal government site.
The site is secure. The https:// ensures that you are connecting to the official website and that any information you provide is encrypted and transmitted securely.
- Publications
- Account settings
Preview improvements coming to the PMC website in October 2024. Learn More or Try it out now .
- Advanced Search
- Journal List
- BMJ Open Access

The meaning of variation to healthcare managers, clinical and health-services researchers, and individual patients
Duncan neuhauser.
1 Department of Epidemiology and Biostatistics, Case Western Reserve University, Cleveland, Ohio, USA
Lloyd Provost
2 Associates in Process Improvement, Austin, Texas, USA
3 Centre for Health Improvement, Chalmers University of Technology, Gothenburg, Sweden
Healthcare managers, clinical researchers and individual patients (and their physicians) manage variation differently to achieve different ends. First, managers are primarily concerned with the performance of care processes over time. Their time horizon is relatively short, and the improvements they are concerned with are pragmatic and ‘holistic.’ Their goal is to create processes that are stable and effective. The analytical techniques of statistical process control effectively reflect these concerns. Second, clinical and health-services researchers are interested in the effectiveness of care and the generalisability of findings. They seek to control variation by their study design methods. Their primary question is: ‘Does A cause B, everything else being equal?’ Consequently, randomised controlled trials and regression models are the research methods of choice. The focus of this reductionist approach is on the ‘average patient’ in the group being observed rather than the individual patient working with the individual care provider. Third, individual patients are primarily concerned with the nature and quality of their own care and clinical outcomes. They and their care providers are not primarily seeking to generalise beyond the unique individual. We propose that the gold standard for helping individual patients with chronic conditions should be longitudinal factorial design of trials with individual patients. Understanding how these three groups deal differently with variation can help appreciate these three approaches.
Introduction
Health managers, clinical researchers, and individual patients need to understand and manage variation in healthcare processes in different time frames and in different ways. In short, they ask different questions about why and how healthcare processes and outcomes change ( table 1 ). Confusing the needs of these three stakeholders results in misunderstanding.
Meaning of variation to managers, researchers and individual patients: questions, methods and time frames
Health managers
Our extensive experience in working with healthcare managers has taught us that their primary goal is to maintain and improve the quality of care processes and outcomes for groups of patients. Ongoing care and its improvement are temporal, so in their situation, learning from variation over time is essential. Data are organised over time to answer the fundamental management question: is care today as good as or better than it was in the past, and how likely is it to be better tomorrow? In answering that question, it becomes crucial to understand the difference between common-cause and special-cause variation (as will be discussed later). Common-cause variation appears as random variation in all measures from healthcare processes. 1 Special-cause variation appears as the effect of causes outside the core processes of the work. Management can reduce this variation by enabling the easy recognition of special-cause variation and by changing healthcare processes—by supporting the use of clinical practice guidelines, for example—but common-cause variation can never be eliminated.
The magnitude of common-cause variation creates the upper and lower control limits in Shewhart control charts. 2–5 Such charts summarise the work of health managers well. Figure 1 shows a Shewhart control chart (p-chart) developed by a quality-improvement team whose aim was to increase compliance with a new care protocol. The clinical records of eligible patients discharged (45–75 patients) were evaluated each week by the team, and records indicating that the complete protocol was followed were identified. The baseline control chart showed a stable process with a centre line (average performance) of 38% compliance. The team analysed the aspects of the protocol that were not followed and developed process changes to make it easier to complete these particular tasks. After successfully adapting the changes to the local environment (indicated by weekly points above the upper control limit in the ‘Implementing Changes’ period), the team formally implemented the changes in each unit. The team continued to monitor the process and eventually developed updated limits for the chart. The updated chart indicated a stable process averaging 83%.

Annotated Shewhart control chart—using protocol.
This control chart makes it clear that a stable but inferior process was operating for the first 11 weeks and, by inference, probably before that. The annotated changes (testing, adapting and implementing new processes of care) are linked to designed tests of change which are special (assignable) causes of variation, in this case, to improvement after week 15, after which a new better stable process has taken hold. Note that there is common-cause (random) variation in both the old and improved processes.
After updating the control limits, the chart reveals a new stable process with no special-cause variation, which is to say, no points above or below the control limits (the dotted lines). Note that the change after week 15 cannot easily be explained by chance (random, or common-cause, variation), since the probability of 13 points in a row occurring by chance above the baseline control limit is one divided by 2 to the 13th power. This is the same likelihood that in flipping a coin 13 times, it will come up heads every time. This level of statistical power to exclude randomness as an explanation is not to be found in randomised controlled trials (RCTs). Although there is no hard-and-fast rule about the number of observations over time needed to demonstrate process stability and establish change, we believe a persuasive control chart requires 20–30 or more observations.
The manager's task demonstrates several important characteristics. First is the need to define the key quality characteristics, and choose among them for focused improvement efforts. The choice should be made based on the needs of patients and families. The importance of these quality characteristics to those being served means that speed in learning and improvement is important. Indeed, for the healthcare manager, information for improvement must be as rapid as possible (in real time). Year-old research data are not very helpful here; just-in-time performance data in the hands of the decision-makers provide a potent opportunity for rapid improvement. 6
Second, managerial change is holistic; that is, every element of an intervention that might help to improve and can be done is put to use, sometimes incrementally, but simultaneously if need be. Healthcare managers are actively working to promote measurement of process and clinical outcomes, take problems in organisational performance seriously, consider the root causes of those problems, encourage the formation of problem solving clinical micro-system teams and promote the use of multiple, evolving Plan–Do–Study–Act (PDSA) tests of change.
This kind of improvement reasoning can be applied to a wide range of care processes, large and small. For example, good surgery is the appropriate combination of hundreds of individual tasks, many of which could be improved in small ways. Aggregating these many smaller changes may result in important, observable improvement over time. The protocol-driven, randomised trial research approach is a powerful tool for establishing efficacy but has limitations for evaluating and improving such complex processes as surgery, which are continually and purposefully changing over time. The realities of clinical improvement call for a move from after-the-fact quality inspection to building quality measures into medical information systems, thereby creating real-time quality data for providers to act upon. Caring for populations of similar patients in similar ways (economies of scale) can be of particular value, because the resulting large numbers and process stability can help rapidly demonstrate variation in care processes 7 ; very tight control limits (minimal common-cause variation) allow special-cause variation to be detected more quickly.
Clinical and health-services researchers
While quality-management thinking tends towards the use of data plotted over time in control-chart format, clinical researchers think in terms of true experimental methods, such as RCTs. Health-services researchers, in contrast, think in terms of regression analysis as their principal tool for discovering explainable variation in processes and outcomes of care. The data that both communities of researchers use are generally collected during fixed periods of time, or combined across time periods; neither is usually concerned with the analysis of data over time.
Take, for example, the question of whether age and sex are associated with the ability to undertake early ambulation after hip surgery. Clinical researchers try to control for such variables through the use of entry criteria into a trial, and random assignment of patients to experimental or control group. The usual health-services research approach would be to use a regression model to predict the outcome (early ambulation), over hundreds of patients using age and sex as independent variables. Such research could show that age and sex predict outcomes and are statistically significant, and that perhaps 10% of the variance is explained by these two independent variables. In contrast, quality-improvement thinking is likely to conclude that 90% of the variance is unexplained and could be common-cause variation. The health-services researcher is therefore likely to conclude that if we measured more variables, we could explain more of this variance, while improvement scientists are more likely to conclude that this unexplained variance is a reflection of common-cause variation in a good process that is under control.
The entry criteria into RCTs are carefully defined, which makes it a challenge to generalise the results beyond the kinds of patients included in such studies. Restricted patient entry criteria are imposed to reduce variation in outcomes unrelated to the experimental intervention. RCTs focus on the difference between point estimates of outcomes for entire groups (control and experimental), using statistical tests of significance to show that differences between the two arms of a trial are not likely to be due to chance.
Individual patients and their healthcare providers
The question an individual patient asks is different from those asked by manager and researcher, namely ‘How can I get better?’ The answer is unique to each patient; the question does not focus on generalising results beyond this person. At the same time, the question the patient's physician is asking is whether the group results from the best clinical trials will apply in this patient's case. This question calls for a different inferential approach. 8–10 The cost of projecting general findings to individual patients could be substantial, as described below.
Consider the implications of a drug trial in which 100 patients taking a new drug and 100 patients taking a placebo are reported as successful because 25 drug takers improved compared with 10 controls. This difference is shown as not likely to be due to chance. (The drug company undertakes a multimillion dollar advertising campaign to promote this breakthrough.) However, on closer examination, the meaning of these results for individual patients is not so clear. To begin with, 75 of the patients who took the drug did not benefit. And among those 25 who benefited, some, perhaps 15, responded extremely well, while the size of the benefit in the other 10 was much smaller. To have only the 15 ‘maximum responders’ take this drug instead of all 100 could save the healthcare system 85% of the drug's costs (as well as reduce the chance of unnecessary adverse drug effects); those ‘savings’ would, of course, also reduce the drug company's sales proportionally. These considerations make it clear that looking at more than group results could potentially make an enormous difference in the value of research studies, particularly from the point of view of individual patients and their providers.
In light of the above concerns, we propose that the longitudinal factorial study design should be the gold standard of evidence for efficacy, particularly for assessing whether interventions whose efficacy has been established through controlled trials are effective in individual patients for whom they might be appropriate ( box 1 ). Take the case of a patient with hypertension who measures her blood pressure at least twice every day and plots these numbers on a run chart. Through this informal observation, she has learnt about several factors that result in the variation in her blood pressure readings: time of day, the three different hypertension medicines she takes (not always regularly), her stress level, eating salty French fries, exercise, meditation (and, in her case, saying the rosary), and whether she slept well the night before. Some of these factors she can control; some are out of her control.
Longitudinal factorial design of experiments for individual patients
The six individual components of this approach are not new, but in combination they are new 8 9
- One patient with a chronic health condition; sometimes referred to as an ‘N-of-1 trial.’
- Care processes and health status are measured over time. These could include daily measures over 20 or more days, with the patient day as the unit of analysis.
- Whenever possible, data are numerical rather than simple clinical observation and classification.
- The patient is directly involved in making therapeutic changes and collecting data.
- Two or more inputs (factors) are experimentally and concurrently changed in a predetermined fashion.
- Therapeutic inputs are added or deleted in a predetermined, systematic way. For example: on day 1, drug A is taken; on day 2, drug B; on day 3, drug A and B; day 4, neither. For the next 4 days, this sequence could be randomly reordered.
Since she is accustomed to monitoring her blood pressure over time, she is in an excellent position to carry out an experiment that would help her optimise the effects of these various influences on her hypertension. Working with her primary care provider, she could, for example, set up a table of randomly chosen dates to make each of several of these changes each day, thereby creating a systematically predetermined mix of these controllable factors over time. This factorial design allows her to measure the effects of individual inputs on her blood pressure, and even interactions among them. After an appropriate number of days (perhaps 30 days, depending on the trade-off between urgency and statistical power), she might conclude that one of her three medications has no effect on her hypertension, and she can stop using it. She might also find that the combination of exercise and consistently low salt intake is as effective as either of the other two drugs. Her answers could well be unique to her. Planned experimental interventions involving single patients are known as ‘N-of-1’ trials, and hundreds have been reported. 10 Although longitudinal factorial design of experiments has long been used in quality engineering, as of 2005 there appears to have been only one published example of its use for an individual patient. 8 9 This method of investigation could potentially become widely used in the future to establish the efficacy of specific drugs for individual patients, 11 and perhaps even required, particularly for very expensive drug therapies for chronic conditions. Such individual trial results could be combined to obtain generalised knowledge.
This method can be used to show (1) the independent effect of each input on the outcome, (2) the interaction effect between the inputs (perhaps neither drug A or B is effective on its own, but in combination they work well), (3) the effect of different drug dosages and (4) the lag time between treatment and outcome. This approach will not be practical if the outcome of interest occurs years later. This method will be more practical with patient access to their medical record where they could monitor all five of Bergman's core health processes. 12
Understanding variation is one of the cornerstones of the science of improvement
This broad understanding of variation, which is based on the work of Walter Shewart in the 1920s, goes well beyond such simple issues as making an intended departure from a guideline or recognising a meaningful change in the outcome of care. It encompasses more than good or bad variation (meeting a target). It is concerned with more than the variation found by researchers in random samples from large populations.
Everything we observe or measure varies. Some variation in healthcare is desirable, even essential, since each patient is different and should be cared for uniquely. New and better treatments, and improvements in care processes result in beneficial variation. Special-cause variation should lead to learning. The ‘Plan–Do–Study’ portion of the Shewhart PDSA cycle can promote valuable change.
The ‘act’ step in the PDSA cycle represents the arrival of stability after a successful improvement has been made. Reducing unintended, and particularly harmful, variation is therefore a key improvement strategy. The more variation is controlled, the easier it is to detect changes that are not explained by chance. Stated differently, narrow limits on a Shewhart control chart make it easier and quicker to detect, and therefore respond to, special-cause variation.
The goal of statistical thinking in quality improvement is to make the available statistical tools as simple and useful as possible in meeting the primary goal, which is not mathematical correctness, but improvement in both the processes and outcomes of care. It is not fruitful to ask whether statistical process control, RCTs, regression equations or longitudinal factorial design of experiments is best in some absolute sense. Each is appropriate for answering different questions.
Forces driving this new way of thinking
The idea of reducing unwanted variation in healthcare represents a major shift in thinking, and it will take time to be accepted. Forces for this change include the computerisation of medical records leading to public reporting of care and outcome comparisons between providers and around the world. This in turn will promote pay for performance, and preferred provider contracting based on guideline use and good outcomes. This way of thinking about variation could spread across all five core systems of health, 12 including self-care and processes of healthy living.
Competing interests: None.
Provenance and peer review: Not commissioned; externally peer reviewed.
- Automation-Analytics-AI
- [email protected]
- +91 80 40109783
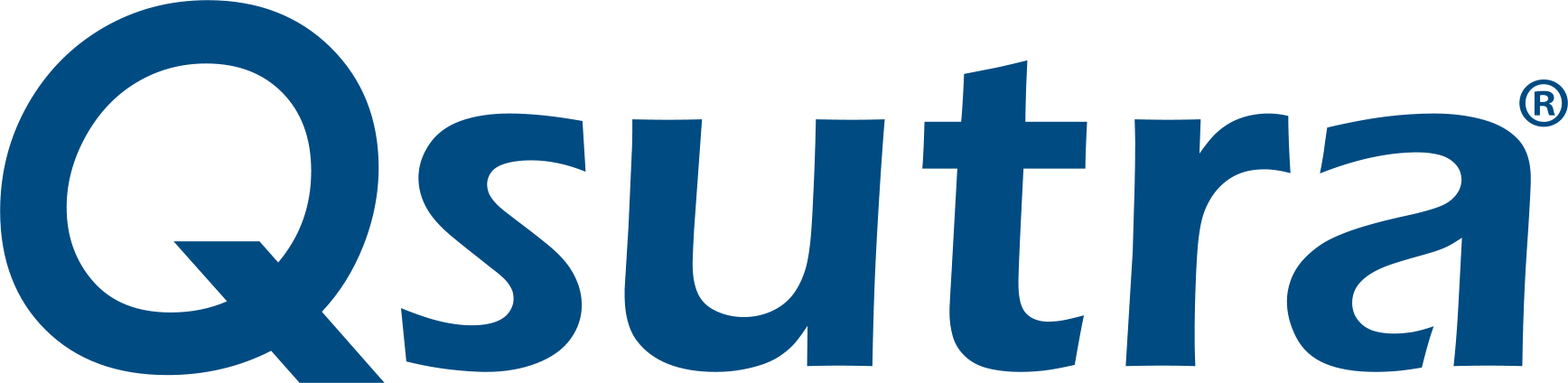
Statistical Process Control (SPC)
Introduction.
In our daily life, we utilize a variety of products and services from different outlets. We use products such as mobile, electrical bulbs, clothes, etc. and use different types of services such as health care, transportation, consulting, etc. All these services and products should attain certain specifications when we use it, whether it can be good or bad. We are on the tough competitive world and so the main aim of the manufacturer or provider is to achieve quality assurance where it can meet the customer expectations.
In such situations, we require a tool or technique through which we can control the process. This technique is known as statistical process control. For understanding SPC, first of all, we should understand the concept of process in quality control. A process is a series of operations or actions that transforms input to output.
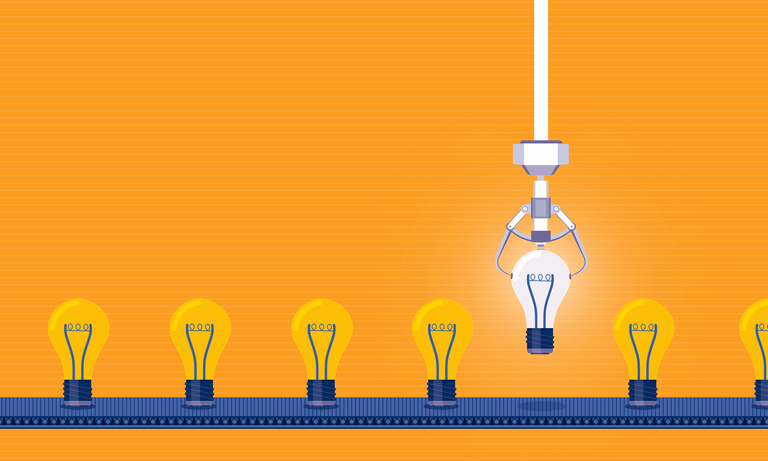
What is Statistical Process Control?
SPC is a method which is used for understanding and monitoring the process by collecting data on quality characteristics periodically from the process, analyzing them and taking suitable actions whenever there is a difference between actual quality and the specifications or standard. It is a decision-making tool and widely used in almost all manufacturing processes for achieving process stability to continuous improvements in product quality.
Brief History of the Origin of SPC
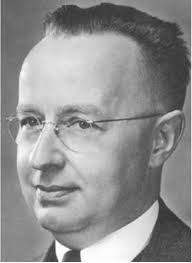
During the 1920s , Walter A. Shewhart discovered a way to distinguish between common and special causes of variation in a process. This lead to an invention of the widely known method as statistical process control (SPC) . He pioneered the use of statistical techniques for monitoring and controlling quality. Bell Labs wanted to economically monitor and control the variation in the quality of components and finished products. He recognized that inspecting and rejecting or reworking product was not the most economical way to produce a high-quality product. He demonstrated that monitoring and controlling variation throughout production was the more efficient and economical way.
Shewhart developed a visual tool for monitoring process variation, which came to be known as the control chart or the Shewhart control chart and the concept of a state of statistical control in 1924 at Bell Laboratories.
He has defined chance and assignable causes as the two sources of quality variation. A process that is operating with the only chance cause of variation is said to be in statistical control. A process that is operating with the assignable cause of variation is said to be out of control. The underlying concept of the Shewhart chart is to construct its limits based on variation allowable as it is in – control state and monitor the quality of the product produced.
Bell Labs was widely recognized as the ‘international standard for quality’ by the 1930s, due to the large applications of Shewhart’s techniques in the field of telecommunications. During this period, many initiatives were done by conducting extraordinary research in statistical methods to control and improve process variation. This leads to improve product quality in a great way.
His work was summarized in his books titled “Economic Control of Quality of Manufactured Product” (1931) and “Statistical Method from the Viewpoint of Quality Control” (1939) .
Image – Referred from leansixsigmadefinition.com
Breakthrough in the evolution of SPC
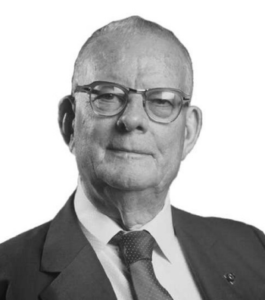
SPC was pioneered by Walter A. Shewhart in the 1920s. W. Edwards Deming applied SPC methods in the United States during World War II, to improve quality in the manufacture of weapons and other important products needed during the war period. After the devastating defeat of Japan in World War II, the United States led the Allies in the occupation and rehabilitation of the Japanese state. In 1947, Deming was involved in early planning for the 1951 Japanese Census. The Allied powers were occupying Japan, and he was asked by the United States Department of the Army to assist with the census. Deming was also instrumental in introducing SPC methods to Japanese industry after the war had ended.
Deming’s mastered the Shewhart’s ideas by implementing it to Japanese industry from 1950 onwards. He developed and added some of his techniques to Shewhart’s methodology. Later he named as the ‘Shewhart cycle’ . Deming’s approach to quality management results in continuous improvement of the production process to achieve conformance to specifications and reduce variability. He identifies two primary sources of process improvement: eliminating common causes of quality problems, such as poor product design and insufficient employee training, and eliminating special causes, such as specific equipment or an operator.
He was widely known for his work contribution for Japanese industry and for the new development era. He received an invitation from the Japanese Union of Scientists and Engineers (JUSE) and worked as an expert to teach statistical control. He trained hundreds of engineers, managers, and scholars in SPC and concepts of quality.
During the 1960s and 1970s, SPC grew rapidly in Japan and was a successful in quality improvement goals. Later other countries started implementing SPC in their process.
His work was summarized in his books titled “ Out of the Crisis” (1982 – 1986) and “ The New Economics for Industry, Government, Education” (1993) , which includes his System of Profound Knowledge and the 14 Points for Management.
Image – Referred from census.gov
Statistical process control also termed as SPC is a statistical method used to monitor, control and improve processes by eliminating variation from industrial, actuarial, service and many other processes. Here we can determine if an improvement is actually happening and also use them to predict whether it is statistically capable to meet the specific target or not. The main aim of using SPC is to understand where the focus of works needs to be done in order to make a difference. It has now been incorporated by organizations around the world as a primary tool to improve product quality by reducing process variation.
During the initial phase, the SPC was applied only on manufacturing industries for quality improvement and so on. As time evolves by, it was started applying on service industry such as airlines, hospitals, insurance companies, etc. Now on this advanced age of science and information technology, it has started applying on big data analytics to artificial intelligence and much more to advance.
Methodology
SPC involves following phases of activity –
- Collection of data from a process.
- Identification of causes and to eliminate it.
- Track process variation.
- Diagnosing the deviated process.
- Implementing corrective action.
(We use basic quality tools on these phases)
How many Types of variation are there in a process?
SPC is implemented in industry to detect a process variation and to eliminate it for better quality assurance. By monitoring the performance of a process, we can detect trends or changes in the process before they produce non-conforming product and scrap. [By reducing variation]
Variation can be divided as common cause variation and special cause variation.
- Chance causes are also known as random or natural or common causes . It is due to the natural variation of the process; i.e. Variation due to the way the process was designed and we cannot identify. For example, the fuel efficiency of machine varies slightly; the diameter of a bottle cap varies slightly and so on. (Statistically in control)
- Assignable causes are also known as special or non-random or unnatural causes. Causes can be identified and eliminated – poor employee training, equipment nonfunctional, etc. An example of special cause variation is the variation that might result if someone untrained is allowed to work in the process. (Out of control)
When to use SPC?
- To have an overall glimpse of a process.
- Monitoring a process to check whether it is under control or out of control.
- To track variation and to eliminate it from a process.
- Improvement in process capability aspects.
- To increase production by reducing scrap, rework and inspection cost.
What are the benefits of SPC?
- Early detection of variation in a process.
- Establish a consistent level of quality.
- Continuous improvement in a process by reducing variation.
- Helps in decision making by giving the insights of process.
- Reduce or eliminate the need for inspection during the supply chain.
- Lower investment because of process improvements.
- It provides real time analysis of a process and so we can focus on areas needed for improvement.
- Efficiency in data entry, analysis and reporting.
What is Process capability analysis?
It is one of the primary tools in SPC. Suppose in a manufacturing process or any process, we often required information about the process w.r.t its performance or capability. Basically, it refers to the capability of a process to meet customer requirements or industrial standards on a consistent basis.
Measures of Process Capability – Process capability can be measured by the following methods.
- Process capability Ratio (C p ) – It is often described as the capability of a process when the process data is centred and specification limits are known.
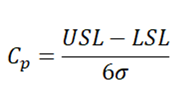
USL -> Upper specification limit
LSL -> Lower specification limit
σ -> Process standard deviation
- Process capability index (Cpk) – It is described as the capability of a process when the process data is not centered and only one of the specification limits are known.
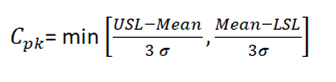
Some important considerations
- When Cp=Cpk –> process is centered at the midpoint of specifications.
- When Cp>Cpk –> process mean is nearer to one specification limit or the other.
- When Cp< 0 –> process mean lies outside the limit.
In a piston manufacturing industry, quality engineers want to assess the process capability. They collect 25 subgroups of five piston rings and measure the diameters. The specification limits for piston ring diameter are 74.0 mm ± 0.05 mm.
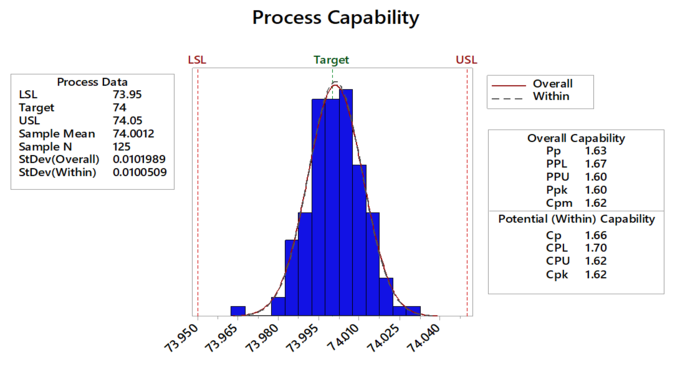
Interpretation
All the measurements are within the specification limits. The process is on target and the measurements are approximately centred between the specification limits.
What are Control limits and Specification limits?
Control limits – Control limits describe the behaviour of a process which operates in a normal condition. It is basically a horizontal lines drawn on a control chart to examines the outlook of a process. It consists of UCL (Upper control limit), CL (Control limit) and LCL (Lower control limit). If the points lie beyond the limits, then there is an occurrence of a special cause of variation and henceforth.
Specification limits – Specification limits are the values on which the process should give a response within the range. It is based on customer requirements. It can be a plot in a histogram and consists of USL (Upper specification limit) and LSL (Lower specification limit).
Control limits reflect the real capability of a process whereas specification limits reflect the requirement of a customer. A process under control may not deliver the products under the given specifications.
What are the Challenges we face while implementing SPC?
In this competitive world, every industry wants to be better than others and to achieve the highest level of success. Suppose it can be in the quality field, continuous improvement in a process, efficient productivity and so on. To achieve this level of success, SPC plays an enormous role in a company and there are some of the challenges one may face while implementing SPC.
Some of them are:-
- Lack of effective training – Training is an important factor for the successful implementation of SPC. Proper training should be given to all the employees who work on a ground level to topmost level in a process.
- Lack of basic statistics knowledge – One should have basic knowledge about statistics. So they can relate the background of SPC methods. Suppose if they were using the histogram in a process then they should have basic knowledge about it.
- Responsibilities should be properly defined – Starting from operators to engineers; everyone should have a clear picture of their responsibility in a process. Engineers should have the basic statistical concepts in SPC whereas operators should be good in measurement and plotting it.
- Management immense support – Elite members of a company should encourage all the employees in all the levels. Management should give time to implement SPC in a proper way. They should never carry away with the time and cost it took to implement it. And nevertheless, wait for its result. Hard work always paid off.
Where can we apply Statistical Process Control?
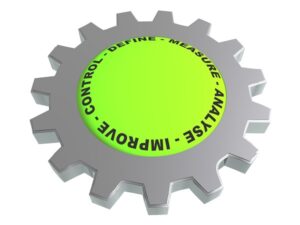
Some of them are discussed below.
- DMAIC – It is a well-known Six Sigma methodology and focused on improving the process. DMAIC stands for Define Measure Analyze Improve and Control. SPC is widely used in Measure, Analyze and Control phases. During the Measure phase, it is used to set the process baseline by doing control chart analysis and Capability analyses are done to check the capability of a process to meet specifications. During the Control phase, it is used to monitor and improve the process.
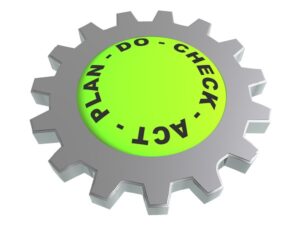
To enhance success in lean manufacturing , six sigma and lean six sigma projects, SPC has to be properly used. Apart from these scenarios, we can use SPC tool individually to check the process capability for continuous improvement. Also, prove useful while conducting DOE in a process.
SPC in Total Quality Management (TQM)
In this competitive world, every industry has to compete with each other in terms of quality, production, revenue and so on. The main terminology which satisfies customer needs is “ quality ” which defines the company standard and values.
Within an organization, when TQM has implemented it helps for continuous improvement of process and gives consistently high-quality products. Total Quality Management is defined by the Deming Prize Committee as
- set of systematic activities
- carried out by the entire organization to effectively and efficiently
- achieve the organization’s objectives
- so as to provide products and services
- with a level of quality
- that satisfies customers ,
- at the appropriate time and price.
Statistical process technique (SPC) is a method used in TQM framework for detecting and reducing variation in a process. It is a very powerful method to detect, control, analyze and improve the process by reducing the source of variation. Hence SPC contributes a lot in TQM goal of continuous improvements.
What kind of Organisations can benefit with SPC?
Statistical process control also termed as SPC is a statistical method used to monitor, control and improve processes by eliminating variation from industrial, actuarial, service and many other processes. When an organization first uses SPC, the main objective is to ensure that the process is stable and capable of producing product or services to the expectations. It is widely known as a decision-making tool.
During the initial phase of SPC, it was used in discrete manufacturing (Telecom, Defense, Automobiles, etc.) and later it was applied to process manufacturing (Glass, Pharmaceutical, Beverage, etc.) too. It is widely used in almost all manufacturing processes for achieving process stability to continuous improvements in product quality.
But in recent years, SPC has implemented in various service sectors like healthcare, financial institutions, call centres, hotels, etc. The service industry has been an integral part of our life. They offer services which are very essential to us – starting from health care, airlines, call centres, banks and so on. For e.g. we often travel to various destinations for official work on holidays by air and stay in a hotel. When we travel by particular airline and didn’t get the essential services – Will we travel again from that airline? Similarly, when we stay at a particular hotel and didn’t get the required services – Will we stay again at that hotel? Our answer will be no, never. So maintaining healthy growth and improving the service quality will have significant impacts on us. And also excellent service quality is noted as a major factor to make a profit in the service sector.
Some of the examples are:-
- Healthcare – While implementing SPC we can improve patient care by reducing waiting time, and monitoring clinical trials, operational performance and so on.
- Banking – While implementing SPC we can improve customer service by reducing waiting time, % errors in customer profile, etc.
- Customer service – While implementing SPC we can improve customer service by reducing the call waiting time, monitoring the response calls, identification of a process whether it is under time limit or not, etc.
In a can-filling process, the quality engineer wants to know whether the process is in control or not. Each hour, they collect a subgroup of 10 cans. To minimize the variation (within subgroup), they collect the cans for a given subgroup in a short period of time.
They create an X-bar chart to monitor the weight of the cans.
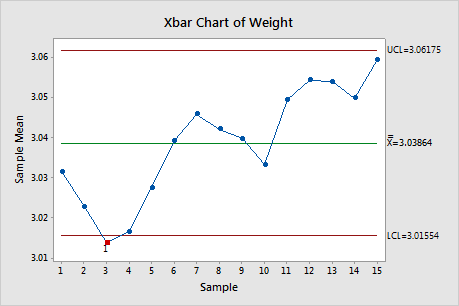
With reference from the X-bar chart, one point is out-of-control and they conclude that the process is not stable. Hence the process should be improved.
Attend our Training Program, to know more about Statistics and Statistical Software. We conduct various training programs – Statistical Training and Minitab Software Training. Some of the Statistical training certified courses are Predictive Analytics Masterclass, Essential Statistics For Business Analytics, SPC Masterclass, DOE Masterclass, etc. (Basic to Advanced Level). Some of the Minitab software training certified courses are Minitab Essentials, Statistical Tools for Pharmaceuticals, Statistical Quality Analysis & Factorial Designs, etc. (Basic to Advanced Level).
We also provide a wide range of Business Analytics Solutions and Business Consulting Services for Organisations to make data-driven decisions and thus enhance their decision support systems.
Create SPC Chart and Run Process Capability Analysis in Minitab
VARIABILITY DUE TO COMMON CAUSES
- Reference work entry
- Cite this reference work entry
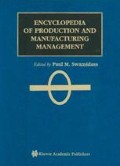
527 Accesses
This is a preview of subscription content, log in via an institution to check access.
Access this chapter
- Available as PDF
- Read on any device
- Instant download
- Own it forever
Tax calculation will be finalised at checkout
Purchases are for personal use only
Institutional subscriptions
Editor information
Rights and permissions.
Reprints and permissions
Copyright information
© 2000 Kluwer Academic Publishers
About this entry
Cite this entry.
(2000). VARIABILITY DUE TO COMMON CAUSES . In: Swamidass, P.M. (eds) Encyclopedia of Production and Manufacturing Management. Springer, Boston, MA . https://doi.org/10.1007/1-4020-0612-8_1030
Download citation
DOI : https://doi.org/10.1007/1-4020-0612-8_1030
Publisher Name : Springer, Boston, MA
Print ISBN : 978-0-7923-8630-8
Online ISBN : 978-1-4020-0612-8
eBook Packages : Springer Book Archive
Share this entry
Anyone you share the following link with will be able to read this content:
Sorry, a shareable link is not currently available for this article.
Provided by the Springer Nature SharedIt content-sharing initiative
- Publish with us
Policies and ethics
- Find a journal
- Track your research
How to deal with Assignable causes?
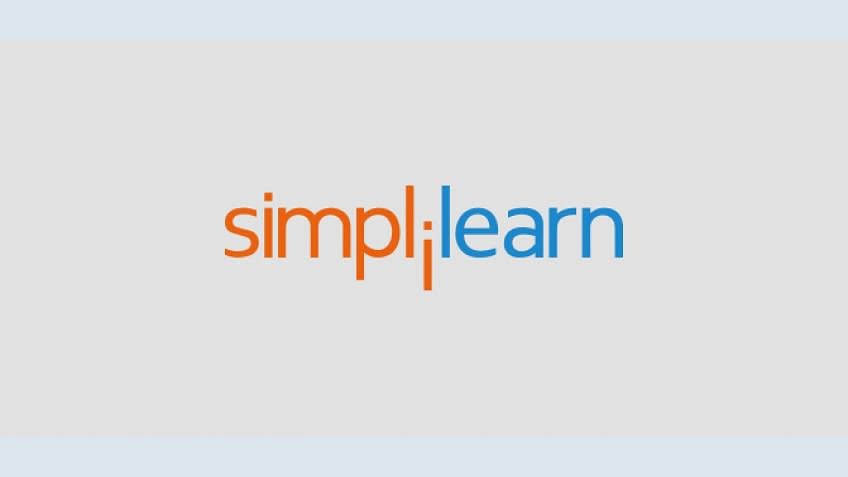
Across the many training sessions conducted one question that keeps raging on is “How do we deal with special causes of variation or assignable causes”. Although theoretically a lot of trainers have found a way of answering this situation, in the real world and especially in Six Sigma projects this is often an open deal. Through this article, I try to address this from a practical paradigm.
Any data you see on any of your charts will have a cause associated with it. Try telling me that the points which make your X MR, IMR or XBar R Charts have dropped the sky and I will tell you that you are not shooting down the right ducks. Then, the following causes seem possible for any data point to appear on the list.
- A new operator was running the process at the time.
- The raw material was near the edge of its specification.
- There was a long time since the last equipment maintenance.
- The equipment maintenance was just performed prior to the processing.
The moment any of our data points appear due to some of the causes mentioned below, a slew of steps are triggered. Yeah – Panic! Worse still, these actions below which may have been a result of a mindless brain haemorrhage backed by absolute lack of data, results in more panic!
- Operators get retraining.
- Incoming material specifications are tightened.
- Maintenance schedules change.
- New procedures are written.
My question is --- Do you really have to do all of this, if you have determined that the cause is a common or a special cause of variation ! Most Six Sigma trainers will tell you that a Control chart will help you identify special cause of variation. True – But did you know of a way you could validate your finding!
- Check the distribution first. If the data is not normal, transform the data to make it reasonably normal. See if it still has extreme points. Compare both the charts before and after transformation. If they are the same, you can be more or less sure it has common causes of variation.
- Plot all of the data, with the event on a control chart. If the point does not exceed the control limits, it is probably a common-cause event. Use the transformed data if used in step 1.
- Using a probability plot, estimate the probability of receiving the extreme value. Consider the probability plot confidence intervals to be like a confidence interval of the data by examining the vertical uncertainty in the plot at the extreme value. If the lower confidence boundary is within the 99% range, the point may be a common-cause event. If the lower CI bound is well outside of the 99% range, it may be a special cause. Of course the same concept works for lower extreme values.
- Finally, turn back the pages of the history. See how frequently these causes have occurred. If they have occurred rather frequently, you may want to think these are common causes of variation. Why – Did you forget special causes don’t really repeat themselves?
The four step approach you have taken may still not be enough for you to conclude if it is a common or a special cause of variation. Note – Any RCA approach may not be good enough to reduce or eliminate common causes. They only work with special causes in the truest sense.
So, what does that leave us with! A simple lesson that an RCA activity has to be conducted when you think even with a certain degree of probability that it could be a special cause of variation. To ascertain that if the cause genuinely was a Special cause all you got to do is look back into the history and see if these causes repeated. If they did, I don’t think you would even be tempted to think it to be a special cause of variation.
Remember one thing – While eliminating special causes is considered goal one for most Six Sigma projects, reducing common causes is another story you’d have to consider. The biggest benefit of dealing with common causes is that you can even deal with them in the long run, provided they are able to keep the process controlled and oh yes, the common causes don’t result in effects.
Merely by looking at a chart, I don’t think I have ever been able to say if the point has a Special cause attached to it or not. Yes – This even applies to a Control chart which is by far considered to be the best Special cause identification tool. The best way out is a diligently applied RCA and a simple act of going back and checking if the cause repeated or not.
Our Quality Management Courses Duration And Fees
Explore our top Quality Management Courses and take the first step towards career success
Recommended Reads
A Guide on How to Become a Site Reliability Engineer (SRE)
10 Major Causes of Project Failure
Your One-Stop Guide ‘On How Does the Internet Work?’
How to Improve Your Company’s Training Completion Rates
Root Cause Analysis: All You Need to Know
How to Become a Cybersecurity Engineer?
Get Affiliated Certifications with Live Class programs
Finance for non-financial professionals.
- 24x7 learner assistance and support
- PMP, PMI, PMBOK, CAPM, PgMP, PfMP, ACP, PBA, RMP, SP, and OPM3 are registered marks of the Project Management Institute, Inc.
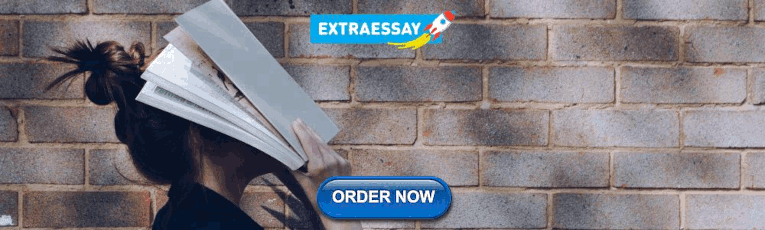
IMAGES
VIDEO
COMMENTS
A cycle or repeating pattern. A run of 8 or more consecutive points on either side of the average or center line. Assignable cause variation can be attributed to a defect, fault, mistake, delay, breakdown, accident, and/or shortage in the process. Or it can be a result of some unique combination of factors coming together to actually improve ...
the presence of assignable causes is said to be an out-of-control process.1 These chance and assignable causes of variation are illustrated in Fig. 5.1. Until time t 1 the process shown in this figure is in control; that is, only chance causes of variation are pre-sent.
ii) Assignable causes. i) Chance Causes: In any manufacturing process, it is not possible to produce goods of exactly the same quality. Variation is inevitable. Certain small variation is natural to the process, being due to chance causes and cannot be prevented. This variation is therefore called allowable. ii) Assignable Causes:
4.1.1 Special Cause Variation The variations which are relatively large in magnitude and viable to identify are the special causes of variation or assignable causes of variation. They may come and go sporadically. Quite often, any specific evidence of the lack of statistical control gives a signal that a special cause is likely to have occurred.
Common and special causes are the two distinct origins of variation in a process, as defined in the statistical thinking and methods of Walter A. Shewhart and W. Edwards Deming.Briefly, "common causes", also called natural patterns, are the usual, historical, quantifiable variation in a system, while "special causes" are unusual, not previously observed, non-quantifiable variation.
Moreover, variation may be classified as one of two types, random or chance cause variation and assignable cause variation. Common Cause: A cause of variation in the process is due to chance but not assignable to any factor. It is the variation that is inherent in the process. Likewise, a process under the influence of a common cause will ...
This video includes the following topics: Chance and Assignable Causes of Quality Variation, Statistical Basis of the Control Chart
The range of such variation is known as natural tolerance of the process. 26.2.6.2 Assignable causes. The second type of variation attributed to any production process is due to non-random or the so called assignable causes and is termed as Preventable Variation. The assignable causes may creep in at any stage of the process, right from the ...
Managing Variation over Time • Statistical Process Control often takes the form of a continuous Hypothesis testing. • The idea is to detect, as quickly as possible, a significant departure from the norm. • A significant change is often attributed to what is known as an assignable cause. •An assignable cause is something that can be
Assignable causes of variation are present in most production processes. These causes of variability are also called special causes of variation ( Deming, 1982 ). The sources of assignable variation can usually be identified (assigned to a specific cause) leading to their elimination. Tool wear, equipment that needs adjustment, defective ...
Both terms are encountered in practice. Needless to say, not all phenomena arise from constant systems of common causes. At times, the variation is caused by a source of variation that is not part of the constant system. These sources of variation were called assignable causes by Shewhart, special causes of variation by Dr. Deming.
Chance Causes: Assignable Causes (i) Composed for many individual causes. Consists of a or equitable a few individual motives. (ii) Any one chance causes results in only a minute amount of variation. (However, loads of the randomly causes act simultaneously so is the grand amount of chance variation a substantial).
Common causes of variation. Random causes that cannot be identified. An important task in quality control is to find out the range of natural random variation in a process. For example, if the average bottle of a soft drink called Cocoa Fizz contains 16 ounces of liquid, we may determine that the amount of natural variation is between 15.8 and ...
what are chance and assignable causes? | statistical quality control | Quality control | #statistics #statistics4all #statisticsforeconomics #qualityeducat...
(Such variability is also known as chance or random variation.) This natural variability is the cumulative effect of several minor factors. ... The graph helped identify common causes of trouble which he called assignable causes of variation. A process is normally stopped and the assignable cause of variation is fixed before restarting the process.
1.4 Causes of Variation Chance Causes Assignable Causes 1.5 Control Charts 1.6 3σ Limits 1.7 Control Chart Patterns Natural Patterns of Variation Unnatural Patterns of Variation 1.8 Advantages and Limitations of SQC 1.9 Summary 1.10 Solutions/Answers 1.1 INTRODUCTION In our day-to-day life, we use different products and services from the time ...
Managing variation is essential to quality improvement. Quality improvement is primarily concerned with two types of variation - common-cause variation and special-cause variation. Common-cause variation is random variation present in stable healthcare processes. Special-cause variation is an unpredictable deviation resulting from a cause ...
This paper presents a fresh approach to the analysis of Shewhart control chart's performance. We consider two different types of assignable causes of variation. One—called type I—affects only the parameters of a model of the underlying distribution. The other—called type X—impacts the type of the original distribution.
In answering that question, it becomes crucial to understand the difference between common-cause and special-cause variation (as will be discussed later). Common-cause variation appears as random variation in all measures from healthcare processes. 1 Special-cause variation appears as the effect of causes outside the core processes of the work ...
Assignable causes are also known as special or non-random or unnatural causes. Causes can be identified and eliminated - poor employee training, equipment nonfunctional, etc. An example of special cause variation is the variation that might result if someone untrained is allowed to work in the process. (Out of control)
Common causes of variability can be reduced but never completely eliminated. Variability due to common causes is due to several sources that are inherent to the process, and it impacts all items processed. Some examples of common causes of variation are as follows: poor product design, poor process design, unfit operation, unsuitable machine ...
This paper presents a fresh approach to the analysis of Shewhart control chart's performance. We consider two different types of assignable causes of variation. One—called type I—affects only the parameters of a model of the underlying distribution. The other—called type X—impacts the type of the original distribution.
Compare both the charts before and after transformation. If they are the same, you can be more or less sure it has common causes of variation. Plot all of the data, with the event on a control chart. If the point does not exceed the control limits, it is probably a common-cause event. Use the transformed data if used in step 1.