ORIGINAL RESEARCH article
Effects of social media use on psychological well-being: a mediated model.
- 1 School of Finance and Economics, Jiangsu University, Zhenjiang, China
- 2 Research Unit of Governance, Competitiveness, and Public Policies (GOVCOPP), Center for Economics and Finance (cef.up), School of Economics and Management, University of Porto, Porto, Portugal
- 3 Department of Business Administration, Sukkur Institute of Business Administration (IBA) University, Sukkur, Pakistan
- 4 CETYS Universidad, Tijuana, Mexico
- 5 Department of Business Administration, Al-Quds University, Jerusalem, Israel
- 6 Business School, Shandong University, Weihai, China
The growth in social media use has given rise to concerns about the impacts it may have on users' psychological well-being. This paper's main objective is to shed light on the effect of social media use on psychological well-being. Building on contributions from various fields in the literature, it provides a more comprehensive study of the phenomenon by considering a set of mediators, including social capital types (i.e., bonding social capital and bridging social capital), social isolation, and smartphone addiction. The paper includes a quantitative study of 940 social media users from Mexico, using structural equation modeling (SEM) to test the proposed hypotheses. The findings point to an overall positive indirect impact of social media usage on psychological well-being, mainly due to the positive effect of bonding and bridging social capital. The empirical model's explanatory power is 45.1%. This paper provides empirical evidence and robust statistical analysis that demonstrates both positive and negative effects coexist, helping to reconcile the inconsistencies found so far in the literature.
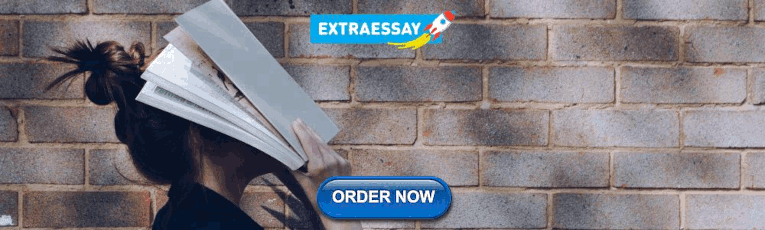
Introduction
The use of social media has grown substantially in recent years ( Leong et al., 2019 ; Kemp, 2020 ). Social media refers to “the websites and online tools that facilitate interactions between users by providing them opportunities to share information, opinions, and interest” ( Swar and Hameed, 2017 , p. 141). Individuals use social media for many reasons, including entertainment, communication, and searching for information. Notably, adolescents and young adults are spending an increasing amount of time on online networking sites, e-games, texting, and other social media ( Twenge and Campbell, 2019 ). In fact, some authors (e.g., Dhir et al., 2018 ; Tateno et al., 2019 ) have suggested that social media has altered the forms of group interaction and its users' individual and collective behavior around the world.
Consequently, there are increased concerns regarding the possible negative impacts associated with social media usage addiction ( Swar and Hameed, 2017 ; Kircaburun et al., 2020 ), particularly on psychological well-being ( Chotpitayasunondh and Douglas, 2016 ; Jiao et al., 2017 ; Choi and Noh, 2019 ; Chatterjee, 2020 ). Smartphones sometimes distract their users from relationships and social interaction ( Chotpitayasunondh and Douglas, 2016 ; Li et al., 2020a ), and several authors have stressed that the excessive use of social media may lead to smartphone addiction ( Swar and Hameed, 2017 ; Leong et al., 2019 ), primarily because of the fear of missing out ( Reer et al., 2019 ; Roberts and David, 2020 ). Social media usage has been associated with anxiety, loneliness, and depression ( Dhir et al., 2018 ; Reer et al., 2019 ), social isolation ( Van Den Eijnden et al., 2016 ; Whaite et al., 2018 ), and “phubbing,” which refers to the extent to which an individual uses, or is distracted by, their smartphone during face-to-face communication with others ( Chotpitayasunondh and Douglas, 2016 ; Jiao et al., 2017 ; Choi and Noh, 2019 ; Chatterjee, 2020 ).
However, social media use also contributes to building a sense of connectedness with relevant others ( Twenge and Campbell, 2019 ), which may reduce social isolation. Indeed, social media provides several ways to interact both with close ties, such as family, friends, and relatives, and weak ties, including coworkers, acquaintances, and strangers ( Chen and Li, 2017 ), and plays a key role among people of all ages as they exploit their sense of belonging in different communities ( Roberts and David, 2020 ). Consequently, despite the fears regarding the possible negative impacts of social media usage on well-being, there is also an increasing number of studies highlighting social media as a new communication channel ( Twenge and Campbell, 2019 ; Barbosa et al., 2020 ), stressing that it can play a crucial role in developing one's presence, identity, and reputation, thus facilitating social interaction, forming and maintaining relationships, and sharing ideas ( Carlson et al., 2016 ), which consequently may be significantly correlated to social support ( Chen and Li, 2017 ; Holliman et al., 2021 ). Interestingly, recent studies (e.g., David et al., 2018 ; Bano et al., 2019 ; Barbosa et al., 2020 ) have suggested that the impact of smartphone usage on psychological well-being depends on the time spent on each type of application and the activities that users engage in.
Hence, the literature provides contradictory cues regarding the impacts of social media on users' well-being, highlighting both the possible negative impacts and the social enhancement it can potentially provide. In line with views on the need to further investigate social media usage ( Karikari et al., 2017 ), particularly regarding its societal implications ( Jiao et al., 2017 ), this paper argues that there is an urgent need to further understand the impact of the time spent on social media on users' psychological well-being, namely by considering other variables that mediate and further explain this effect.
One of the relevant perspectives worth considering is that provided by social capital theory, which is adopted in this paper. Social capital theory has previously been used to study how social media usage affects psychological well-being (e.g., Bano et al., 2019 ). However, extant literature has so far presented only partial models of associations that, although statistically acceptable and contributing to the understanding of the scope of social networks, do not provide as comprehensive a vision of the phenomenon as that proposed within this paper. Furthermore, the contradictory views, suggesting both negative (e.g., Chotpitayasunondh and Douglas, 2016 ; Van Den Eijnden et al., 2016 ; Jiao et al., 2017 ; Whaite et al., 2018 ; Choi and Noh, 2019 ; Chatterjee, 2020 ) and positive impacts ( Carlson et al., 2016 ; Chen and Li, 2017 ; Twenge and Campbell, 2019 ) of social media on psychological well-being, have not been adequately explored.
Given this research gap, this paper's main objective is to shed light on the effect of social media use on psychological well-being. As explained in detail in the next section, this paper explores the mediating effect of bonding and bridging social capital. To provide a broad view of the phenomenon, it also considers several variables highlighted in the literature as affecting the relationship between social media usage and psychological well-being, namely smartphone addiction, social isolation, and phubbing. The paper utilizes a quantitative study conducted in Mexico, comprising 940 social media users, and uses structural equation modeling (SEM) to test a set of research hypotheses.
This article provides several contributions. First, it adds to existing literature regarding the effect of social media use on psychological well-being and explores the contradictory indications provided by different approaches. Second, it proposes a conceptual model that integrates complementary perspectives on the direct and indirect effects of social media use. Third, it offers empirical evidence and robust statistical analysis that demonstrates that both positive and negative effects coexist, helping resolve the inconsistencies found so far in the literature. Finally, this paper provides insights on how to help reduce the potential negative effects of social media use, as it demonstrates that, through bridging and bonding social capital, social media usage positively impacts psychological well-being. Overall, the article offers valuable insights for academics, practitioners, and society in general.
The remainder of this paper is organized as follows. Section Literature Review presents a literature review focusing on the factors that explain the impact of social media usage on psychological well-being. Based on the literature review, a set of hypotheses are defined, resulting in the proposed conceptual model, which includes both the direct and indirect effects of social media usage on psychological well-being. Section Research Methodology explains the methodological procedures of the research, followed by the presentation and discussion of the study's results in section Results. Section Discussion is dedicated to the conclusions and includes implications, limitations, and suggestions for future research.
Literature Review
Putnam (1995 , p. 664–665) defined social capital as “features of social life – networks, norms, and trust – that enable participants to act together more effectively to pursue shared objectives.” Li and Chen (2014 , p. 117) further explained that social capital encompasses “resources embedded in one's social network, which can be assessed and used for instrumental or expressive returns such as mutual support, reciprocity, and cooperation.”
Putnam (1995 , 2000) conceptualized social capital as comprising two dimensions, bridging and bonding, considering the different norms and networks in which they occur. Bridging social capital refers to the inclusive nature of social interaction and occurs when individuals from different origins establish connections through social networks. Hence, bridging social capital is typically provided by heterogeneous weak ties ( Li and Chen, 2014 ). This dimension widens individual social horizons and perspectives and provides extended access to resources and information. Bonding social capital refers to the social and emotional support each individual receives from his or her social networks, particularly from close ties (e.g., family and friends).
Overall, social capital is expected to be positively associated with psychological well-being ( Bano et al., 2019 ). Indeed, Williams (2006) stressed that interaction generates affective connections, resulting in positive impacts, such as emotional support. The following sub-sections use the lens of social capital theory to explore further the relationship between the use of social media and psychological well-being.
Social Media Use, Social Capital, and Psychological Well-Being
The effects of social media usage on social capital have gained increasing scholarly attention, and recent studies have highlighted a positive relationship between social media use and social capital ( Brown and Michinov, 2019 ; Tefertiller et al., 2020 ). Li and Chen (2014) hypothesized that the intensity of Facebook use by Chinese international students in the United States was positively related to social capital forms. A longitudinal survey based on the quota sampling approach illustrated the positive effects of social media use on the two social capital dimensions ( Chen and Li, 2017 ). Abbas and Mesch (2018) argued that, as Facebook usage increases, it will also increase users' social capital. Karikari et al. (2017) also found positive effects of social media use on social capital. Similarly, Pang (2018) studied Chinese students residing in Germany and found positive effects of social networking sites' use on social capital, which, in turn, was positively associated with psychological well-being. Bano et al. (2019) analyzed the 266 students' data and found positive effects of WhatsApp use on social capital forms and the positive effect of social capital on psychological well-being, emphasizing the role of social integration in mediating this positive effect.
Kim and Kim (2017) stressed the importance of having a heterogeneous network of contacts, which ultimately enhances the potential social capital. Overall, the manifest and social relations between people from close social circles (bonding social capital) and from distant social circles (bridging social capital) are strengthened when they promote communication, social support, and the sharing of interests, knowledge, and skills, which are shared with other members. This is linked to positive effects on interactions, such as acceptance, trust, and reciprocity, which are related to the individuals' health and psychological well-being ( Bekalu et al., 2019 ), including when social media helps to maintain social capital between social circles that exist outside of virtual communities ( Ellison et al., 2007 ).
Grounded on the above literature, this study proposes the following hypotheses:
H1a: Social media use is positively associated with bonding social capital.
H1b: Bonding social capital is positively associated with psychological well-being.
H2a: Social media use is positively associated with bridging social capital.
H2b: Bridging social capital is positively associated with psychological well-being.
Social Media Use, Social Isolation, and Psychological Well-Being
Social isolation is defined as “a deficit of personal relationships or being excluded from social networks” ( Choi and Noh, 2019 , p. 4). The state that occurs when an individual lacks true engagement with others, a sense of social belonging, and a satisfying relationship is related to increased mortality and morbidity ( Primack et al., 2017 ). Those who experience social isolation are deprived of social relationships and lack contact with others or involvement in social activities ( Schinka et al., 2012 ). Social media usage has been associated with anxiety, loneliness, and depression ( Dhir et al., 2018 ; Reer et al., 2019 ), and social isolation ( Van Den Eijnden et al., 2016 ; Whaite et al., 2018 ). However, some recent studies have argued that social media use decreases social isolation ( Primack et al., 2017 ; Meshi et al., 2020 ). Indeed, the increased use of social media platforms such as Facebook, WhatsApp, Instagram, and Twitter, among others, may provide opportunities for decreasing social isolation. For instance, the improved interpersonal connectivity achieved via videos and images on social media helps users evidence intimacy, attenuating social isolation ( Whaite et al., 2018 ).
Chappell and Badger (1989) stated that social isolation leads to decreased psychological well-being, while Choi and Noh (2019) concluded that greater social isolation is linked to increased suicide risk. Schinka et al. (2012) further argued that, when individuals experience social isolation from siblings, friends, family, or society, their psychological well-being tends to decrease. Thus, based on the literature cited above, this study proposes the following hypotheses:
H3a: Social media use is significantly associated with social isolation.
H3b: Social isolation is negatively associated with psychological well-being.
Social Media Use, Smartphone Addiction, Phubbing, and Psychological Well-Being
Smartphone addiction refers to “an individuals' excessive use of a smartphone and its negative effects on his/her life as a result of his/her inability to control his behavior” ( Gökçearslan et al., 2018 , p. 48). Regardless of its form, smartphone addiction results in social, medical, and psychological harm to people by limiting their ability to make their own choices ( Chotpitayasunondh and Douglas, 2016 ). The rapid advancement of information and communication technologies has led to the concept of social media, e-games, and also to smartphone addiction ( Chatterjee, 2020 ). The excessive use of smartphones for social media use, entertainment (watching videos, listening to music), and playing e-games is more common amongst people addicted to smartphones ( Jeong et al., 2016 ). In fact, previous studies have evidenced the relationship between social use and smartphone addiction ( Salehan and Negahban, 2013 ; Jeong et al., 2016 ; Swar and Hameed, 2017 ). In line with this, the following hypotheses are proposed:
H4a: Social media use is positively associated with smartphone addiction.
H4b: Smartphone addiction is negatively associated with psychological well-being.
While smartphones are bringing individuals closer, they are also, to some extent, pulling people apart ( Tonacci et al., 2019 ). For instance, they can lead to individuals ignoring others with whom they have close ties or physical interactions; this situation normally occurs due to extreme smartphone use (i.e., at the dinner table, in meetings, at get-togethers and parties, and in other daily activities). This act of ignoring others is called phubbing and is considered a common phenomenon in communication activities ( Guazzini et al., 2019 ; Chatterjee, 2020 ). Phubbing is also referred to as an act of snubbing others ( Chatterjee, 2020 ). This term was initially used in May 2012 by an Australian advertising agency to describe the “growing phenomenon of individuals ignoring their families and friends who were called phubbee (a person who is a recipients of phubbing behavior) victim of phubber (a person who start phubbing her or his companion)” ( Chotpitayasunondh and Douglas, 2018 ). Smartphone addiction has been found to be a determinant of phubbing ( Kim et al., 2018 ). Other recent studies have also evidenced the association between smartphones and phubbing ( Chotpitayasunondh and Douglas, 2016 ; Guazzini et al., 2019 ; Tonacci et al., 2019 ; Chatterjee, 2020 ). Vallespín et al. (2017 ) argued that phubbing behavior has a negative influence on psychological well-being and satisfaction. Furthermore, smartphone addiction is considered responsible for the development of new technologies. It may also negatively influence individual's psychological proximity ( Chatterjee, 2020 ). Therefore, based on the above discussion and calls for the association between phubbing and psychological well-being to be further explored, this study proposes the following hypotheses:
H5: Smartphone addiction is positively associated with phubbing.
H6: Phubbing is negatively associated with psychological well-being.
Indirect Relationship Between Social Media Use and Psychological Well-Being
Beyond the direct hypotheses proposed above, this study investigates the indirect effects of social media use on psychological well-being mediated by social capital forms, social isolation, and phubbing. As described above, most prior studies have focused on the direct influence of social media use on social capital forms, social isolation, smartphone addiction, and phubbing, as well as the direct impact of social capital forms, social isolation, smartphone addiction, and phubbing on psychological well-being. Very few studies, however, have focused on and evidenced the mediating role of social capital forms, social isolation, smartphone addiction, and phubbing derived from social media use in improving psychological well-being ( Chen and Li, 2017 ; Pang, 2018 ; Bano et al., 2019 ; Choi and Noh, 2019 ). Moreover, little is known about smartphone addiction's mediating role between social media use and psychological well-being. Therefore, this study aims to fill this gap in the existing literature by investigating the mediation of social capital forms, social isolation, and smartphone addiction. Further, examining the mediating influence will contribute to a more comprehensive understanding of social media use on psychological well-being via the mediating associations of smartphone addiction and psychological factors. Therefore, based on the above, we propose the following hypotheses (the conceptual model is presented in Figure 1 ):
H7: (a) Bonding social capital; (b) bridging social capital; (c) social isolation; and (d) smartphone addiction mediate the relationship between social media use and psychological well-being.
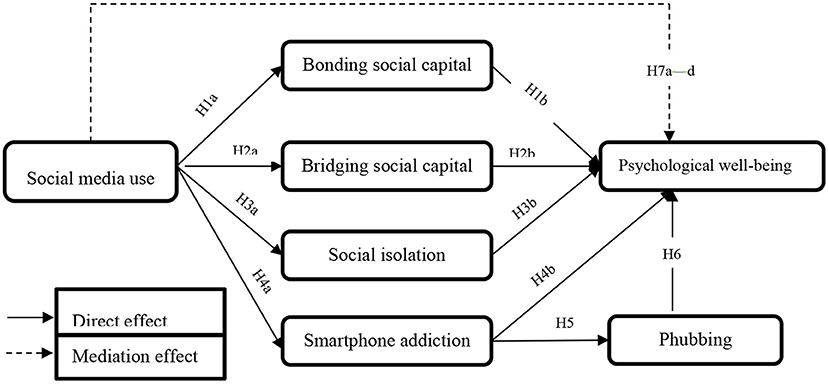
Figure 1 . Conceptual model.
Research Methodology
Sample procedure and online survey.
This study randomly selected students from universities in Mexico. We chose University students for the following reasons. First, students are considered the most appropriate sample for e-commerce studies, particularly in the social media context ( Oghazi et al., 2018 ; Shi et al., 2018 ). Second, University students are considered to be frequent users and addicted to smartphones ( Mou et al., 2017 ; Stouthuysen et al., 2018 ). Third, this study ensured that respondents were experienced, well-educated, and possessed sufficient knowledge of the drawbacks of social media and the extreme use of smartphones. A total sample size of 940 University students was ultimately achieved from the 1,500 students contacted, using a convenience random sampling approach, due both to the COVID-19 pandemic and budget and time constraints. Additionally, in order to test the model, a quantitative empirical study was conducted, using an online survey method to collect data. This study used a web-based survey distributed via social media platforms for two reasons: the COVID-19 pandemic; and to reach a large number of respondents ( Qalati et al., 2021 ). Furthermore, online surveys are considered a powerful and authenticated tool for new research ( Fan et al., 2021 ), while also representing a fast, simple, and less costly approach to collecting data ( Dutot and Bergeron, 2016 ).
Data Collection Procedures and Respondent's Information
Data were collected by disseminating a link to the survey by e-mail and social network sites. Before presenting the closed-ended questionnaire, respondents were assured that their participation would remain voluntary, confidential, and anonymous. Data collection occurred from July 2020 to December 2020 (during the pandemic). It should be noted that, because data were collected during the pandemic, this may have had an influence on the results of the study. The reason for choosing a six-month lag time was to mitigate common method bias (CMB) ( Li et al., 2020b ). In the present study, 1,500 students were contacted via University e-mail and social applications (Facebook, WhatsApp, and Instagram). We sent a reminder every month for 6 months (a total of six reminders), resulting in 940 valid responses. Thus, 940 (62.6% response rate) responses were used for hypotheses testing.
Table 1 reveals that, of the 940 participants, three-quarters were female (76.4%, n = 719) and nearly one-quarter (23.6%, n = 221) were male. Nearly half of the participants (48.8%, n = 459) were aged between 26 and 35 years, followed by 36 to 35 years (21.9%, n = 206), <26 (20.3%, n = 191), and over 45 (8.9%, n = 84). Approximately two-thirds (65%, n = 611) had a bachelor's degree or above, while one-third had up to 12 years of education. Regarding the daily frequency of using the Internet, nearly half (48.6%, n = 457) of the respondents reported between 5 and 8 h a day, and over one-quarter (27.2%) 9–12 h a day. Regarding the social media platforms used, over 38.5 and 39.6% reported Facebook and WhatsApp, respectively. Of the 940 respondents, only 22.1% reported Instagram (12.8%) and Twitter (9.2%). It should be noted, however, that the sample is predominantly female and well-educated.
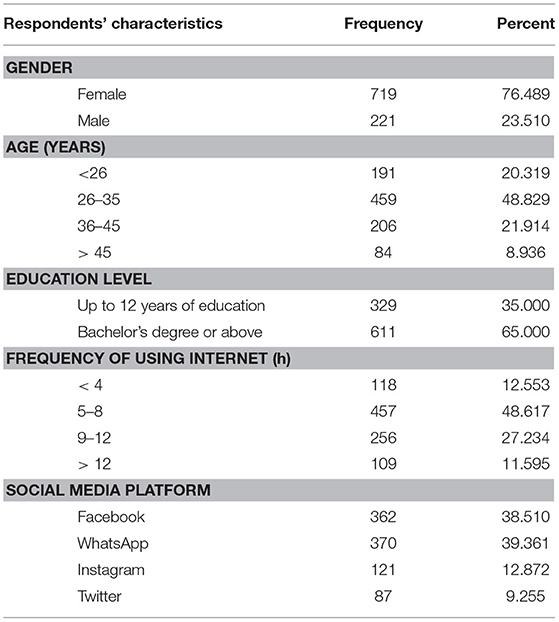
Table 1 . Respondents' characteristics.
Measurement Items
The study used five-point Likert scales (1 = “strongly disagree;” 5 = “strongly agree”) to record responses.
Social Media Use
Social media use was assessed using four items adapted from Karikari et al. (2017) . Sample items include “Social media is part of my everyday activity,” “Social media has become part of my daily life,” “I would be sorry if social media shut down,” and “I feel out of touch, when I have not logged onto social media for a while.” The adapted items had robust reliability and validity (CA = 783, CR = 0.857, AVE = 0.600).
Social Capital
Social capital was measured using a total of eight items, representing bonding social capital (four items) and bridging social capital (four items) adapted from Chan (2015) . Sample construct items include: bonging social capital (“I am willing to spend time to support general community activities,” “I interact with people who are quite different from me”) and bridging social capital (“My social media community is a good place to be,” “Interacting with people on social media makes me want to try new things”). The adapted items had robust reliability and validity [bonding social capital (CA = 0.785, CR = 0.861, AVE = 0.608) and bridging social capital (CA = 0.834, CR = 0.883, AVE = 0.601)].
Social Isolation
Social isolation was assessed using three items from Choi and Noh (2019) . Sample items include “I do not have anyone to play with,” “I feel alone from people,” and “I have no one I can trust.” This adapted scale had substantial reliability and validity (CA = 0.890, CR = 0.928, AVE = 0.811).
Smartphone Addiction
Smartphone addiction was assessed using five items taken from Salehan and Negahban (2013) . Sample items include “I am always preoccupied with my mobile,” “Using my mobile phone keeps me relaxed,” and “I am not able to control myself from frequent use of mobile phones.” Again, these adapted items showed substantial reliability and validity (CA = 903, CR = 0.928, AVE = 0.809).
Phubbing was assessed using four items from Chotpitayasunondh and Douglas (2018) . Sample items include: “I have conflicts with others because I am using my phone” and “I would rather pay attention to my phone than talk to others.” This construct also demonstrated significant reliability and validity (CA = 770, CR = 0.894, AVE = 0.809).
Psychological Well-Being
Psychological well-being was assessed using five items from Jiao et al. (2017) . Sample items include “I lead a purposeful and meaningful life with the help of others,” “My social relationships are supportive and rewarding in social media,” and “I am engaged and interested in my daily on social media.” This study evidenced that this adapted scale had substantial reliability and validity (CA = 0.886, CR = 0.917, AVE = 0.688).
Data Analysis
Based on the complexity of the association between the proposed construct and the widespread use and acceptance of SmartPLS 3.0 in several fields ( Hair et al., 2019 ), we utilized SEM, using SmartPLS 3.0, to examine the relationships between constructs. Structural equation modeling is a multivariate statistical analysis technique that is used to investigate relationships. Further, it is a combination of factor and multivariate regression analysis, and is employed to explore the relationship between observed and latent constructs.
SmartPLS 3.0 “is a more comprehensive software program with an intuitive graphical user interface to run partial least square SEM analysis, certainly has had a massive impact” ( Sarstedt and Cheah, 2019 ). According to Ringle et al. (2015) , this commercial software offers a wide range of algorithmic and modeling options, improved usability, and user-friendly and professional support. Furthermore, Sarstedt and Cheah (2019) suggested that structural equation models enable the specification of complex interrelationships between observed and latent constructs. Hair et al. (2019) argued that, in recent years, the number of articles published using partial least squares SEM has increased significantly in contrast to covariance-based SEM. In addition, partial least squares SEM using SmartPLS is more appealing for several scholars as it enables them to predict more complex models with several variables, indicator constructs, and structural paths, instead of imposing distributional assumptions on the data ( Hair et al., 2019 ). Therefore, this study utilized the partial least squares SEM approach using SmartPLS 3.0.
Common Method Bias (CMB) Test
This study used the Kaiser–Meyer–Olkin (KMO) test to measure the sampling adequacy and ensure data suitability. The KMO test result was 0.874, which is greater than an acceptable threshold of 0.50 ( Ali Qalati et al., 2021 ; Shrestha, 2021 ), and hence considered suitable for explanatory factor analysis. Moreover, Bartlett's test results demonstrated a significance level of 0.001, which is considered good as it is below the accepted threshold of 0.05.
The term CMB is associated with Campbell and Fiske (1959) , who highlighted the importance of CMB and identified that a portion of variance in the research may be due to the methods employed. It occurs when all scales of the study are measured at the same time using a single questionnaire survey ( Podsakoff and Organ, 1986 ); subsequently, estimates of the relationship among the variables might be distorted by the impacts of CMB. It is considered a serious issue that has a potential to “jeopardize” the validity of the study findings ( Tehseen et al., 2017 ). There are several reasons for CMB: (1) it mainly occurs due to response “tendencies that raters can apply uniformity across the measures;” and (2) it also occurs due to similarities in the wording and structure of the survey items that produce similar results ( Jordan and Troth, 2019 ). Harman's single factor test and a full collinearity approach were employed to ensure that the data was free from CMB ( Tehseen et al., 2017 ; Jordan and Troth, 2019 ; Ali Qalati et al., 2021 ). Harman's single factor test showed a single factor explained only 22.8% of the total variance, which is far below the 50.0% acceptable threshold ( Podsakoff et al., 2003 ).
Additionally, the variance inflation factor (VIF) was used, which is a measure of the amount of multicollinearity in a set of multiple regression constructs and also considered a way of detecting CMB ( Hair et al., 2019 ). Hair et al. (2019) suggested that the acceptable threshold for the VIF is 3.0; as the computed VIFs for the present study ranged from 1.189 to 1.626, CMB is not a key concern (see Table 2 ). Bagozzi et al. (1991) suggested a correlation-matrix procedure to detect CMB. Common method bias is evident if correlation among the principle constructs is >0.9 ( Tehseen et al., 2020 ); however, no values >0.9 were found in this study (see section Assessment of Measurement Model). This study used a two-step approach to evaluate the measurement model and the structural model.
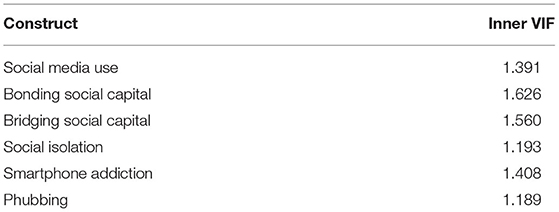
Table 2 . Common method bias (full collinearity VIF).
Assessment of Measurement Model
Before conducting the SEM analysis, the measurement model was assessed to examine individual item reliability, internal consistency, and convergent and discriminant validity. Table 3 exhibits the values of outer loading used to measure an individual item's reliability ( Hair et al., 2012 ). Hair et al. (2017) proposed that the value for each outer loading should be ≥0.7; following this principle, two items of phubbing (PHUB3—I get irritated if others ask me to get off my phone and talk to them; PHUB4—I use my phone even though I know it irritated others) were removed from the analysis Hair et al. (2019) . According to Nunnally (1978) , Cronbach's alpha values should exceed 0.7. The threshold values of constructs in this study ranged from 0.77 to 0.903. Regarding internal consistency, Bagozzi and Yi (1988) suggested that composite reliability (CR) should be ≥0.7. The coefficient value for CR in this study was between 0.857 and 0.928. Regarding convergent validity, Fornell and Larcker (1981) suggested that the average variance extracted (AVE) should be ≥0.5. Average variance extracted values in this study were between 0.60 and 0.811. Finally, regarding discriminant validity, according to Fornell and Larcker (1981) , the square root of the AVE for each construct should exceed the inter-correlations of the construct with other model constructs. That was the case in this study, as shown in Table 4 .
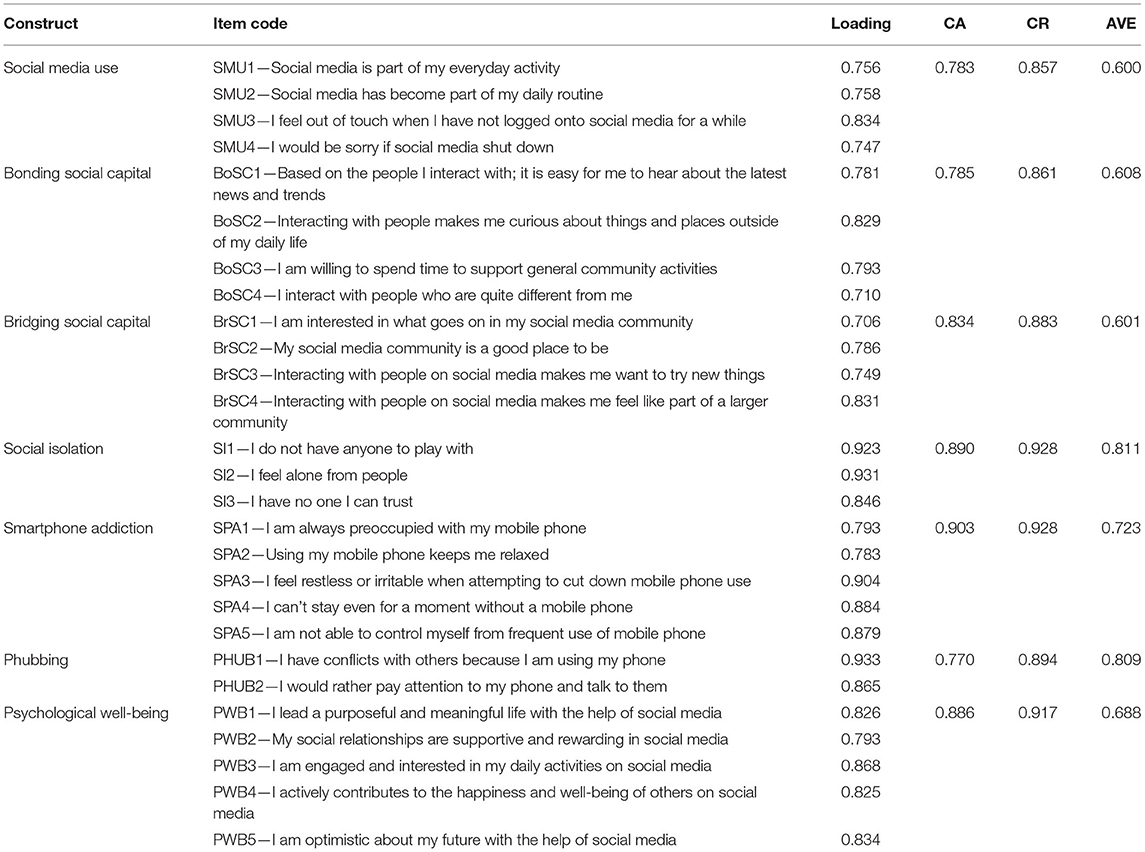
Table 3 . Study measures, factor loading, and the constructs' reliability and convergent validity.
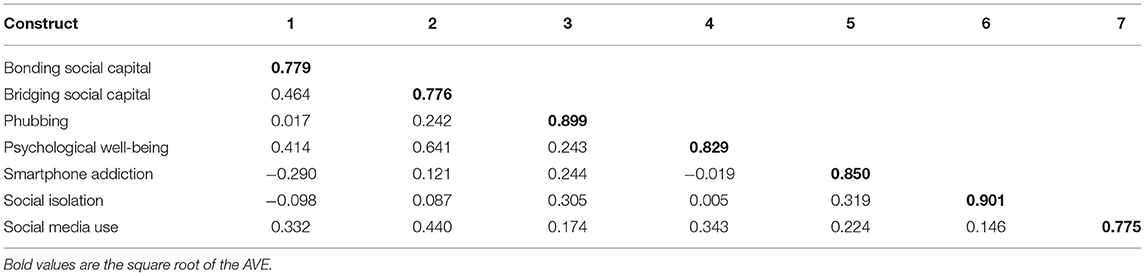
Table 4 . Discriminant validity and correlation.
Hence, by analyzing the results of the measurement model, it can be concluded that the data are adequate for structural equation estimation.
Assessment of the Structural Model
This study used the PLS algorithm and a bootstrapping technique with 5,000 bootstraps as proposed by Hair et al. (2019) to generate the path coefficient values and their level of significance. The coefficient of determination ( R 2 ) is an important measure to assess the structural model and its explanatory power ( Henseler et al., 2009 ; Hair et al., 2019 ). Table 5 and Figure 2 reveal that the R 2 value in the present study was 0.451 for psychological well-being, which means that 45.1% of changes in psychological well-being occurred due to social media use, social capital forms (i.e., bonding and bridging), social isolation, smartphone addiction, and phubbing. Cohen (1998) proposed that R 2 values of 0.60, 0.33, and 0.19 are considered substantial, moderate, and weak. Following Cohen's (1998) threshold values, this research demonstrates a moderate predicting power for psychological well-being among Mexican respondents ( Table 6 ).
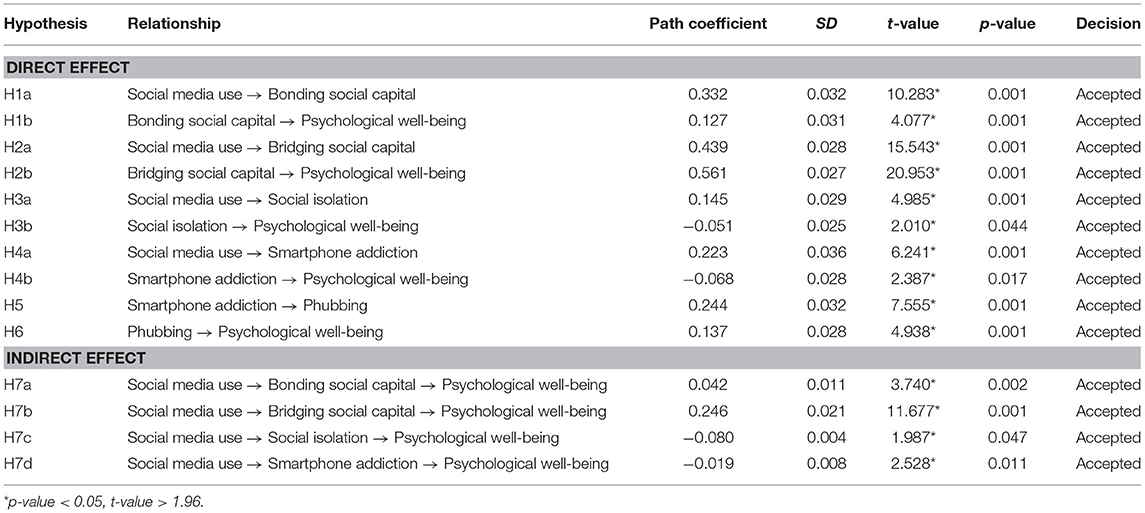
Table 5 . Summary of path coefficients and hypothesis testing.
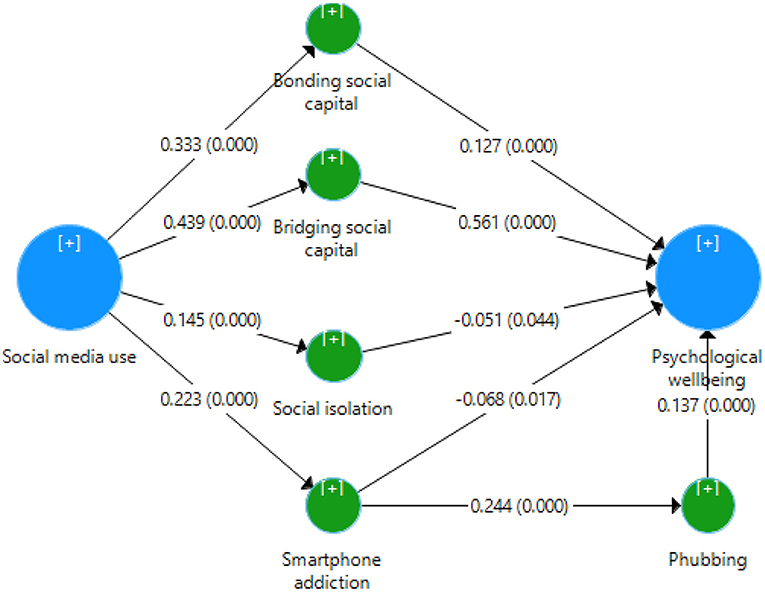
Figure 2 . Structural model.

Table 6 . Strength of the model (Predictive relevance, coefficient of determination, and model fit indices).
Apart from the R 2 measure, the present study also used cross-validated redundancy measures, or effect sizes ( q 2 ), to assess the proposed model and validate the results ( Ringle et al., 2012 ). Hair et al. (2019) suggested that a model exhibiting an effect size q 2 > 0 has predictive relevance ( Table 6 ). This study's results evidenced that it has a 0.15 <0.29 <0.35 (medium) predictive relevance, as 0.02, 0.15, and 0.35 are considered small, medium, and large, respectively ( Cohen, 1998 ). Regarding the goodness-of-fit indices, Hair et al. (2019) suggested the standardized root mean square residual (SRMR) to evaluate the goodness of fit. Standardized root mean square is an absolute measure of fit: a value of zero indicates perfect fit and a value <0.08 is considered good fit ( Hair et al., 2019 ). This study exhibits an adequate model fitness level with an SRMR value of 0.063 ( Table 6 ).
Table 5 reveals that all hypotheses of the study were accepted base on the criterion ( p -value < 0.05). H1a (β = 0.332, t = 10.283, p = 0.001) was confirmed, with the second most robust positive and significant relationship (between social media use and bonding social capital). In addition, this study evidenced a positive and significant relationship between bonding social capital and psychological well-being (β = 0.127, t = 4.077, p = 0.001); therefore, H1b was accepted. Regarding social media use and bridging social capital, the present study found the most robust positive and significant impact (β = 0.439, t = 15.543, p = 0.001); therefore, H2a was accepted. The study also evidenced a positive and significant association between bridging social capital and psychological well-being (β = 0.561, t = 20.953, p = 0.001); thus, H2b was accepted. The present study evidenced a significant effect of social media use on social isolation (β = 0.145, t = 4.985, p = 0.001); thus, H3a was accepted. In addition, this study accepted H3b (β = −0.051, t = 2.01, p = 0.044). Furthermore, this study evidenced a positive and significant effect of social media use on smartphone addiction (β = 0.223, t = 6.241, p = 0.001); therefore, H4a was accepted. Furthermore, the present study found that smartphone addiction has a negative significant influence on psychological well-being (β = −0.068, t = 2.387, p = 0.017); therefore, H4b was accepted. Regarding the relationship between smartphone addiction and phubbing, this study found a positive and significant effect of smartphone addiction on phubbing (β = 0.244, t = 7.555, p = 0.001); therefore, H5 was accepted. Furthermore, the present research evidenced a positive and significant influence of phubbing on psychological well-being (β = 0.137, t = 4.938, p = 0.001); therefore, H6 was accepted. Finally, the study provides interesting findings on the indirect effect of social media use on psychological well-being ( t -value > 1.96 and p -value < 0.05); therefore, H7a–d were accepted.
Furthermore, to test the mediating analysis, Preacher and Hayes's (2008) approach was used. The key characteristic of an indirect relationship is that it involves a third construct, which plays a mediating role in the relationship between the independent and dependent constructs. Logically, the effect of A (independent construct) on C (the dependent construct) is mediated by B (a third variable). Preacher and Hayes (2008) suggested the following: B is a construct acting as a mediator if A significantly influences B, A significantly accounts for variability in C, B significantly influences C when controlling for A, and the influence of A on C decreases significantly when B is added simultaneously with A as a predictor of C. According to Matthews et al. (2018) , if the indirect effect is significant while the direct insignificant, full mediation has occurred, while if both direct and indirect effects are substantial, partial mediation has occurred. This study evidenced that there is partial mediation in the proposed construct ( Table 5 ). Following Preacher and Hayes (2008) this study evidenced that there is partial mediation in the proposed construct, because the relationship between independent variable (social media use) and dependent variable (psychological well-being) is significant ( p -value < 0.05) and indirect effect among them after introducing mediator (bonding social capital, bridging social capital, social isolation, and smartphone addiction) is also significant ( p -value < 0.05), therefore it is evidenced that when there is a significant effect both direct and indirect it's called partial mediation.
The present study reveals that the social and psychological impacts of social media use among University students is becoming more complex as there is continuing advancement in technology, offering a range of affordable interaction opportunities. Based on the 940 valid responses collected, all the hypotheses were accepted ( p < 0.05).
H1a finding suggests that social media use is a significant influencing factor of bonding social capital. This implies that, during a pandemic, social media use enables students to continue their close relationships with family members, friends, and those with whom they have close ties. This finding is in line with prior work of Chan (2015) and Ellison et al. (2007) , who evidenced that social bonding capital is predicted by Facebook use and having a mobile phone. H1b findings suggest that, when individuals believe that social communication can help overcome obstacles to interaction and encourage more virtual self-disclosure, social media use can improve trust and promote the establishment of social associations, thereby enhancing well-being. These findings are in line with those of Gong et al. (2021) , who also witnessed the significant effect of bonding social capital on immigrants' psychological well-being, subsequently calling for the further evidence to confirm the proposed relationship.
The findings of the present study related to H2a suggest that students are more likely to use social media platforms to receive more emotional support, increase their ability to mobilize others, and to build social networks, which leads to social belongingness. Furthermore, the findings suggest that social media platforms enable students to accumulate and maintain bridging social capital; further, online classes can benefit students who feel shy when participating in offline classes. This study supports the previous findings of Chan (2015) and Karikari et al. (2017) . Notably, the present study is not limited to a single social networking platform, taking instead a holistic view of social media. The H2b findings are consistent with those of Bano et al. (2019) , who also confirmed the link between bonding social capital and psychological well-being among University students using WhatsApp as social media platform, as well as those of Chen and Li (2017) .
The H3a findings suggest that, during the COVID-19 pandemic when most people around the world have had limited offline or face-to-face interaction and have used social media to connect with families, friends, and social communities, they have often been unable to connect with them. This is due to many individuals avoiding using social media because of fake news, financial constraints, and a lack of trust in social media; thus, the lack both of offline and online interaction, coupled with negative experiences on social media use, enhances the level of social isolation ( Hajek and König, 2021 ). These findings are consistent with those of Adnan and Anwar (2020) . The H3b suggests that higher levels of social isolation have a negative impact on psychological well-being. These result indicating that, consistent with Choi and Noh (2019) , social isolation is negatively and significantly related to psychological well-being.
The H4a results suggests that substantial use of social media use leads to an increase in smartphone addiction. These findings are in line with those of Jeong et al. (2016) , who stated that the excessive use of smartphones for social media, entertainment (watching videos, listening to music), and playing e-games was more likely to lead to smartphone addiction. These findings also confirm the previous work of Jeong et al. (2016) , Salehan and Negahban (2013) , and Swar and Hameed (2017) . The H4b results revealed that a single unit increase in smartphone addiction results in a 6.8% decrease in psychological well-being. These findings are in line with those of Tangmunkongvorakul et al. (2019) , who showed that students with higher levels of smartphone addiction had lower psychological well-being scores. These findings also support those of Shoukat (2019) , who showed that smartphone addiction inversely influences individuals' mental health.
This suggests that the greater the smartphone addiction, the greater the phubbing. The H5 findings are in line with those of Chatterjee (2020) , Chotpitayasunondh and Douglas (2016) , Guazzini et al. (2019) , and Tonacci et al. (2019) , who also evidenced a significant impact of smartphone addiction and phubbing. Similarly, Chotpitayasunondh and Douglas (2018) corroborated that smartphone addiction is the main predictor of phubbing behavior. However, these findings are inconsistent with those of Vallespín et al. (2017 ), who found a negative influence of phubbing.
The H6 results suggests that phubbing is one of the significant predictors of psychological well-being. Furthermore, these findings suggest that, when phubbers use a cellphone during interaction with someone, especially during the current pandemic, and they are connected with many family members, friends, and relatives; therefore, this kind of action gives them more satisfaction, which simultaneously results in increased relaxation and decreased depression ( Chotpitayasunondh and Douglas, 2018 ). These findings support those of Davey et al. (2018) , who evidenced that phubbing has a significant influence on adolescents and social health students in India.
The findings showed a significant and positive effect of social media use on psychological well-being both through bridging and bonding social capital. However, a significant and negative effect of social media use on psychological well-being through smartphone addiction and through social isolation was also found. Hence, this study provides evidence that could shed light on the contradictory contributions in the literature suggesting both positive (e.g., Chen and Li, 2017 ; Twenge and Campbell, 2019 ; Roberts and David, 2020 ) and negative (e.g., Chotpitayasunondh and Douglas, 2016 ; Jiao et al., 2017 ; Choi and Noh, 2019 ; Chatterjee, 2020 ) effects of social media use on psychological well-being. This study concludes that the overall impact is positive, despite some degree of negative indirect impact.
Theoretical Contributions
This study's findings contribute to the current literature, both by providing empirical evidence for the relationships suggested by extant literature and by demonstrating the relevance of adopting a more complex approach that considers, in particular, the indirect effect of social media on psychological well-being. As such, this study constitutes a basis for future research ( Van Den Eijnden et al., 2016 ; Whaite et al., 2018 ) aiming to understand the impacts of social media use and to find ways to reduce its possible negative impacts.
In line with Kim and Kim (2017) , who stressed the importance of heterogeneous social networks in improving social capital, this paper suggests that, to positively impact psychological well-being, social media usage should be associated both with strong and weak ties, as both are important in building social capital, and hence associated with its bonding and bridging facets. Interestingly, though, bridging capital was shown as having the greatest impact on psychological well-being. Thus, the importance of wider social horizons, the inclusion in different groups, and establishing new connections ( Putnam, 1995 , 2000 ) with heterogeneous weak ties ( Li and Chen, 2014 ) are highlighted in this paper.
Practical Contributions
These findings are significant for practitioners, particularly those interested in dealing with the possible negative impacts of social media use on psychological well-being. Although social media use is associated with factors that negatively impact psychological well-being, particularly smartphone addiction and social isolation, these negative impacts can be lessened if the connections with both strong and weak ties are facilitated and featured by social media. Indeed, social media platforms offer several features, from facilitating communication with family, friends, and acquaintances, to identifying and offering access to other people with shared interests. However, it is important to access heterogeneous weak ties ( Li and Chen, 2014 ) so that social media offers access to wider sources of information and new resources, hence enhancing bridging social capital.
Limitations and Directions for Future Studies
This study is not without limitations. For example, this study used a convenience sampling approach to reach to a large number of respondents. Further, this study was conducted in Mexico only, limiting the generalizability of the results; future research should therefore use a cross-cultural approach to investigate the impacts of social media use on psychological well-being and the mediating role of proposed constructs (e.g., bonding and bridging social capital, social isolation, and smartphone addiction). The sample distribution may also be regarded as a limitation of the study because respondents were mainly well-educated and female. Moreover, although Internet channels represent a particularly suitable way to approach social media users, the fact that this study adopted an online survey does not guarantee a representative sample of the population. Hence, extrapolating the results requires caution, and study replication is recommended, particularly with social media users from other countries and cultures. The present study was conducted in the context of mainly University students, primarily well-educated females, via an online survey on in Mexico; therefore, the findings represent a snapshot at a particular time. Notably, however, the effect of social media use is increasing due to COVID-19 around the globe and is volatile over time.
Two of the proposed hypotheses of this study, namely the expected negative impacts of social media use on social isolation and of phubbing on psychological well-being, should be further explored. One possible approach is to consider the type of connections (i.e., weak and strong ties) to explain further the impact of social media usage on social isolation. Apparently, the prevalence of weak ties, although facilitating bridging social capital, may have an adverse impact in terms of social isolation. Regarding phubbing, the fact that the findings point to a possible positive impact on psychological well-being should be carefully addressed, specifically by psychology theorists and scholars, in order to identify factors that may help further understand this phenomenon. Other suggestions for future research include using mixed-method approaches, as qualitative studies could help further validate the results and provide complementary perspectives on the relationships between the considered variables.
Data Availability Statement
The raw data supporting the conclusions of this article will be made available by the authors, without undue reservation.
Ethics Statement
The studies involving human participants were reviewed and approved by Jiangsu University. The patients/participants provided their written informed consent to participate in this study.
Author Contributions
All authors listed have made a substantial, direct and intellectual contribution to the work, and approved it for publication.
This study is supported by the National Statistics Research Project of China (2016LY96).
Conflict of Interest
The authors declare that the research was conducted in the absence of any commercial or financial relationships that could be construed as a potential conflict of interest.
Abbas, R., and Mesch, G. (2018). Do rich teens get richer? Facebook use and the link between offline and online social capital among Palestinian youth in Israel. Inf. Commun. Soc. 21, 63–79. doi: 10.1080/1369118X.2016.1261168
CrossRef Full Text | Google Scholar
Adnan, M., and Anwar, K. (2020). Online learning amid the COVID-19 pandemic: students' perspectives. J. Pedagog. Sociol. Psychol. 2, 45–51. doi: 10.33902/JPSP.2020261309
PubMed Abstract | CrossRef Full Text | Google Scholar
Ali Qalati, S., Li, W., Ahmed, N., Ali Mirani, M., and Khan, A. (2021). Examining the factors affecting SME performance: the mediating role of social media adoption. Sustainability 13:75. doi: 10.3390/su13010075
Bagozzi, R. P., and Yi, Y. (1988). On the evaluation of structural equation models. J. Acad. Mark. Sci. 16, 74–94. doi: 10.1007/BF02723327
Bagozzi, R. P., Yi, Y., and Phillips, L. W. (1991). Assessing construct validity in organizational research. Admin. Sci. Q. 36, 421–458. doi: 10.2307/2393203
Bano, S., Cisheng, W., Khan, A. N., and Khan, N. A. (2019). WhatsApp use and student's psychological well-being: role of social capital and social integration. Child. Youth Serv. Rev. 103, 200–208. doi: 10.1016/j.childyouth.2019.06.002
Barbosa, B., Chkoniya, V., Simoes, D., Filipe, S., and Santos, C. A. (2020). Always connected: generation Y smartphone use and social capital. Rev. Ibérica Sist. Tecnol. Inf. E 35, 152–166.
Google Scholar
Bekalu, M. A., McCloud, R. F., and Viswanath, K. (2019). Association of social media use with social well-being, positive mental health, and self-rated health: disentangling routine use from emotional connection to use. Health Educ. Behav. 46(2 Suppl), 69S−80S. doi: 10.1177/1090198119863768
Brown, G., and Michinov, N. (2019). Measuring latent ties on Facebook: a novel approach to studying their prevalence and relationship with bridging social capital. Technol. Soc. 59:101176. doi: 10.1016/j.techsoc.2019.101176
Campbell, D. T., and Fiske, D. W. (1959). Convergent and discriminant validation by the multitrait-multimethod matrix. Psychol. Bull. 56, 81–105. doi: 10.1037/h0046016
Carlson, J. R., Zivnuska, S., Harris, R. B., Harris, K. J., and Carlson, D. S. (2016). Social media use in the workplace: a study of dual effects. J. Org. End User Comput. 28, 15–31. doi: 10.4018/JOEUC.2016010102
Chan, M. (2015). Mobile phones and the good life: examining the relationships among mobile use, social capital and subjective well-being. New Media Soc. 17, 96–113. doi: 10.1177/1461444813516836
Chappell, N. L., and Badger, M. (1989). Social isolation and well-being. J. Gerontol. 44, S169–S176. doi: 10.1093/geronj/44.5.s169
Chatterjee, S. (2020). Antecedents of phubbing: from technological and psychological perspectives. J. Syst. Inf. Technol. 22, 161–118. doi: 10.1108/JSIT-05-2019-0089
Chen, H.-T., and Li, X. (2017). The contribution of mobile social media to social capital and psychological well-being: examining the role of communicative use, friending and self-disclosure. Comput. Hum. Behav. 75, 958–965. doi: 10.1016/j.chb.2017.06.011
Choi, D.-H., and Noh, G.-Y. (2019). The influence of social media use on attitude toward suicide through psychological well-being, social isolation, and social support. Inf. Commun. Soc. 23, 1–17. doi: 10.1080/1369118X.2019.1574860
Chotpitayasunondh, V., and Douglas, K. M. (2016). How “phubbing” becomes the norm: the antecedents and consequences of snubbing via smartphone. Comput. Hum. Behav. 63, 9–18. doi: 10.1016/j.chb.2016.05.018
Chotpitayasunondh, V., and Douglas, K. M. (2018). The effects of “phubbing” on social interaction. J. Appl. Soc. Psychol. 48, 304–316. doi: 10.1111/jasp.12506
Cohen, J. (1998). Statistical Power Analysis for the Behavioural Sciences . Hillsdale, NJ: Lawrence Erlbaum Associates.
Davey, S., Davey, A., Raghav, S. K., Singh, J. V., Singh, N., Blachnio, A., et al. (2018). Predictors and consequences of “phubbing” among adolescents and youth in India: an impact evaluation study. J. Fam. Community Med. 25, 35–42. doi: 10.4103/jfcm.JFCM_71_17
David, M. E., Roberts, J. A., and Christenson, B. (2018). Too much of a good thing: investigating the association between actual smartphone use and individual well-being. Int. J. Hum. Comput. Interact. 34, 265–275. doi: 10.1080/10447318.2017.1349250
Dhir, A., Yossatorn, Y., Kaur, P., and Chen, S. (2018). Online social media fatigue and psychological wellbeing—a study of compulsive use, fear of missing out, fatigue, anxiety and depression. Int. J. Inf. Manag. 40, 141–152. doi: 10.1016/j.ijinfomgt.2018.01.012
Dutot, V., and Bergeron, F. (2016). From strategic orientation to social media orientation: improving SMEs' performance on social media. J. Small Bus. Enterp. Dev. 23, 1165–1190. doi: 10.1108/JSBED-11-2015-0160
Ellison, N. B., Steinfield, C., and Lampe, C. (2007). The benefits of Facebook “friends:” Social capital and college students' use of online social network sites. J. Comput. Mediat. Commun. 12, 1143–1168. doi: 10.1111/j.1083-6101.2007.00367.x
Fan, M., Huang, Y., Qalati, S. A., Shah, S. M. M., Ostic, D., and Pu, Z. (2021). Effects of information overload, communication overload, and inequality on digital distrust: a cyber-violence behavior mechanism. Front. Psychol. 12:643981. doi: 10.3389/fpsyg.2021.643981
Fornell, C., and Larcker, D. F. (1981). Evaluating structural equation models with unobservable variables and measurement error. J. Market. Res. 18, 39–50. doi: 10.1177/002224378101800104
Gökçearslan, S., Uluyol, Ç., and Sahin, S. (2018). Smartphone addiction, cyberloafing, stress and social support among University students: a path analysis. Child. Youth Serv. Rev. 91, 47–54. doi: 10.1016/j.childyouth.2018.05.036
Gong, S., Xu, P., and Wang, S. (2021). Social capital and psychological well-being of Chinese immigrants in Japan. Int. J. Environ. Res. Public Health 18:547. doi: 10.3390/ijerph18020547
Guazzini, A., Duradoni, M., Capelli, A., and Meringolo, P. (2019). An explorative model to assess individuals' phubbing risk. Fut. Internet 11:21. doi: 10.3390/fi11010021
Hair, J. F., Risher, J. J., Sarstedt, M., and Ringle, C. M. (2019). When to use and how to report the results of PLS-SEM. Eur. Bus. Rev. 31, 2–24. doi: 10.1108/EBR-11-2018-0203
Hair, J. F., Sarstedt, M., Pieper, T. M., and Ringle, C. M. (2012). The use of partial least squares structural equation modeling in strategic management research: a review of past practices and recommendations for future applications. Long Range Plann. 45, 320–340. doi: 10.1016/j.lrp.2012.09.008
Hair, J. F., Sarstedt, M., Ringle, C. M., and Gudergan, S. P. (2017). Advanced Issues in Partial Least Squares Structural Equation Modeling. Thousand Oaks, CA: Sage.
Hajek, A., and König, H.-H. (2021). Social isolation and loneliness of older adults in times of the CoViD-19 pandemic: can use of online social media sites and video chats assist in mitigating social isolation and loneliness? Gerontology 67, 121–123. doi: 10.1159/000512793
Henseler, J., Ringle, C. M., and Sinkovics, R. R. (2009). “The use of partial least squares path modeling in international marketing,” in New Challenges to International Marketing , Vol. 20, eds R.R. Sinkovics and P.N. Ghauri (Bigley: Emerald), 277–319.
Holliman, A. J., Waldeck, D., Jay, B., Murphy, S., Atkinson, E., Collie, R. J., et al. (2021). Adaptability and social support: examining links with psychological wellbeing among UK students and non-students. Fron. Psychol. 12:636520. doi: 10.3389/fpsyg.2021.636520
Jeong, S.-H., Kim, H., Yum, J.-Y., and Hwang, Y. (2016). What type of content are smartphone users addicted to? SNS vs. games. Comput. Hum. Behav. 54, 10–17. doi: 10.1016/j.chb.2015.07.035
Jiao, Y., Jo, M.-S., and Sarigöllü, E. (2017). Social value and content value in social media: two paths to psychological well-being. J. Org. Comput. Electr. Commer. 27, 3–24. doi: 10.1080/10919392.2016.1264762
Jordan, P. J., and Troth, A. C. (2019). Common method bias in applied settings: the dilemma of researching in organizations. Austr. J. Manag. 45, 3–14. doi: 10.1177/0312896219871976
Karikari, S., Osei-Frimpong, K., and Owusu-Frimpong, N. (2017). Evaluating individual level antecedents and consequences of social media use in Ghana. Technol. Forecast. Soc. Change 123, 68–79. doi: 10.1016/j.techfore.2017.06.023
Kemp, S. (January 30, 2020). Digital 2020: 3.8 billion people use social media. We Are Social . Available online at: https://wearesocial.com/blog/2020/01/digital-2020-3-8-billion-people-use-social-media .
Kim, B., and Kim, Y. (2017). College students' social media use and communication network heterogeneity: implications for social capital and subjective well-being. Comput. Hum. Behav. 73, 620–628. doi: 10.1016/j.chb.2017.03.033
Kim, K., Milne, G. R., and Bahl, S. (2018). Smart phone addiction and mindfulness: an intergenerational comparison. Int. J. Pharmaceut. Healthcare Market. 12, 25–43. doi: 10.1108/IJPHM-08-2016-0044
Kircaburun, K., Alhabash, S., Tosuntaş, S. B., and Griffiths, M. D. (2020). Uses and gratifications of problematic social media use among University students: a simultaneous examination of the big five of personality traits, social media platforms, and social media use motives. Int. J. Mental Health Addict. 18, 525–547. doi: 10.1007/s11469-018-9940-6
Leong, L.-Y., Hew, T.-S., Ooi, K.-B., Lee, V.-H., and Hew, J.-J. (2019). A hybrid SEM-neural network analysis of social media addiction. Expert Syst. Appl. 133, 296–316. doi: 10.1016/j.eswa.2019.05.024
Li, L., Griffiths, M. D., Mei, S., and Niu, Z. (2020a). Fear of missing out and smartphone addiction mediates the relationship between positive and negative affect and sleep quality among Chinese University students. Front. Psychiatr. 11:877. doi: 10.3389/fpsyt.2020.00877
Li, W., Qalati, S. A., Khan, M. A. S., Kwabena, G. Y., Erusalkina, D., and Anwar, F. (2020b). Value co-creation and growth of social enterprises in developing countries: moderating role of environmental dynamics. Entrep. Res. J. 2020:20190359. doi: 10.1515/erj-2019-0359
Li, X., and Chen, W. (2014). Facebook or Renren? A comparative study of social networking site use and social capital among Chinese international students in the United States. Comput. Hum. Behav . 35, 116–123. doi: 10.1016/j.chb.2014.02.012
Matthews, L., Hair, J. F., and Matthews, R. (2018). PLS-SEM: the holy grail for advanced analysis. Mark. Manag. J. 28, 1–13.
Meshi, D., Cotten, S. R., and Bender, A. R. (2020). Problematic social media use and perceived social isolation in older adults: a cross-sectional study. Gerontology 66, 160–168. doi: 10.1159/000502577
Mou, J., Shin, D.-H., and Cohen, J. (2017). Understanding trust and perceived usefulness in the consumer acceptance of an e-service: a longitudinal investigation. Behav. Inf. Technol. 36, 125–139. doi: 10.1080/0144929X.2016.1203024
Nunnally, J. (1978). Psychometric Methods . New York, NY: McGraw-Hill.
Oghazi, P., Karlsson, S., Hellström, D., and Hjort, K. (2018). Online purchase return policy leniency and purchase decision: mediating role of consumer trust. J. Retail. Consumer Serv. 41, 190–200.
Pang, H. (2018). Exploring the beneficial effects of social networking site use on Chinese students' perceptions of social capital and psychological well-being in Germany. Int. J. Intercult. Relat. 67, 1–11. doi: 10.1016/j.ijintrel.2018.08.002
Podsakoff, P. M., MacKenzie, S. B., Lee, J.-Y., and Podsakoff, N. P. (2003). Common method biases in behavioral research: a critical review of the literature and recommended remedies. J. Appl. Psychol. 88, 879–903. doi: 10.1037/0021-9010.88.5.879
Podsakoff, P. M., and Organ, D. W. (1986). Self-reports in organizational research: problems and prospects. J. Manag. 12, 531–544. doi: 10.1177/014920638601200408
Preacher, K. J., and Hayes, A. F. (2008). Asymptotic and resampling strategies for assessing and comparing indirect effects in multiple mediator models. Behav Res. Methods 40, 879–891. doi: 10.3758/brm.40.3.879
Primack, B. A., Shensa, A., Sidani, J. E., Whaite, E. O., yi Lin, L., Rosen, D., et al. (2017). Social media use and perceived social isolation among young adults in the US. Am. J. Prev. Med. 53, 1–8. doi: 10.1016/j.amepre.2017.01.010
Putnam, R. D. (1995). Tuning in, tuning out: the strange disappearance of social capital in America. Polit. Sci. Polit. 28, 664–684. doi: 10.2307/420517
Putnam, R. D. (2000). Bowling Alone: The Collapse and Revival of American Community . New York, NY: Simon and Schuster.
Qalati, S. A., Ostic, D., Fan, M., Dakhan, S. A., Vela, E. G., Zufar, Z., et al. (2021). The general public knowledge, attitude, and practices regarding COVID-19 during the lockdown in Asian developing countries. Int. Q. Commun. Health Educ. 2021:272684X211004945. doi: 10.1177/0272684X211004945
Reer, F., Tang, W. Y., and Quandt, T. (2019). Psychosocial well-being and social media engagement: the mediating roles of social comparison orientation and fear of missing out. New Media Soc. 21, 1486–1505. doi: 10.1177/1461444818823719
Ringle, C., Wende, S., and Becker, J. (2015). SmartPLS 3 [software] . Bönningstedt: SmartPLS.
Ringle, C. M., Sarstedt, M., and Straub, D. (2012). A critical look at the use of PLS-SEM in “MIS Quarterly.” MIS Q . 36, iii–xiv. doi: 10.2307/41410402
Roberts, J. A., and David, M. E. (2020). The social media party: fear of missing out (FoMO), social media intensity, connection, and well-being. Int. J. Hum. Comput. Interact. 36, 386–392. doi: 10.1080/10447318.2019.1646517
Salehan, M., and Negahban, A. (2013). Social networking on smartphones: when mobile phones become addictive. Comput. Hum. Behav. 29, 2632–2639. doi: 10.1016/j.chb.2013.07.003
Sarstedt, M., and Cheah, J.-H. (2019). Partial least squares structural equation modeling using SmartPLS: a software review. J. Mark. Anal. 7, 196–202. doi: 10.1057/s41270-019-00058-3
Schinka, K. C., VanDulmen, M. H., Bossarte, R., and Swahn, M. (2012). Association between loneliness and suicidality during middle childhood and adolescence: longitudinal effects and the role of demographic characteristics. J. Psychol. Interdiscipl. Appl. 146, 105–118. doi: 10.1080/00223980.2011.584084
Shi, S., Mu, R., Lin, L., Chen, Y., Kou, G., and Chen, X.-J. (2018). The impact of perceived online service quality on swift guanxi. Internet Res. 28, 432–455. doi: 10.1108/IntR-12-2016-0389
Shoukat, S. (2019). Cell phone addiction and psychological and physiological health in adolescents. EXCLI J. 18, 47–50. doi: 10.17179/excli2018-2006
Shrestha, N. (2021). Factor analysis as a tool for survey analysis. Am. J. Appl. Math. Stat. 9, 4–11. doi: 10.12691/ajams-9-1-2
Stouthuysen, K., Teunis, I., Reusen, E., and Slabbinck, H. (2018). Initial trust and intentions to buy: The effect of vendor-specific guarantees, customer reviews and the role of online shopping experience. Electr. Commer. Res. Appl. 27, 23–38. doi: 10.1016/j.elerap.2017.11.002
Swar, B., and Hameed, T. (2017). “Fear of missing out, social media engagement, smartphone addiction and distraction: moderating role of self-help mobile apps-based interventions in the youth ,” Paper presented at the 10th International Conference on Health Informatics (Porto).
Tangmunkongvorakul, A., Musumari, P. M., Thongpibul, K., Srithanaviboonchai, K., Techasrivichien, T., Suguimoto, S. P., et al. (2019). Association of excessive smartphone use with psychological well-being among University students in Chiang Mai, Thailand. PLoS ONE 14:e0210294. doi: 10.1371/journal.pone.0210294
Tateno, M., Teo, A. R., Ukai, W., Kanazawa, J., Katsuki, R., Kubo, H., et al. (2019). Internet addiction, smartphone addiction, and hikikomori trait in Japanese young adult: social isolation and social network. Front. Psychiatry 10:455. doi: 10.3389/fpsyt.2019.00455
Tefertiller, A. C., Maxwell, L. C., and Morris, D. L. (2020). Social media goes to the movies: fear of missing out, social capital, and social motivations of cinema attendance. Mass Commun. Soc. 23, 378–399. doi: 10.1080/15205436.2019.1653468
Tehseen, S., Qureshi, Z. H., Johara, F., and Ramayah, T. (2020). Assessing dimensions of entrepreneurial competencies: a type II (reflective-formative) measurement approach using PLS-SEM. J. Sustain. Sci. Manage. 15, 108–145.
Tehseen, S., Ramayah, T., and Sajilan, S. (2017). Testing and controlling for common method variance: a review of available methods. J. Manag. Sci. 4, 146–165. doi: 10.20547/jms.2014.1704202
Tonacci, A., Billeci, L., Sansone, F., Masci, A., Pala, A. P., Domenici, C., et al. (2019). An innovative, unobtrusive approach to investigate smartphone interaction in nonaddicted subjects based on wearable sensors: a pilot study. Medicina (Kaunas) 55:37. doi: 10.3390/medicina55020037
Twenge, J. M., and Campbell, W. K. (2019). Media use is linked to lower psychological well-being: evidence from three datasets. Psychiatr. Q. 90, 311–331. doi: 10.1007/s11126-019-09630-7
Vallespín, M., Molinillo, S., and Muñoz-Leiva, F. (2017). Segmentation and explanation of smartphone use for travel planning based on socio-demographic and behavioral variables. Ind. Manag. Data Syst. 117, 605–619. doi: 10.1108/IMDS-03-2016-0089
Van Den Eijnden, R. J., Lemmens, J. S., and Valkenburg, P. M. (2016). The social media disorder scale. Comput. Hum. Behav. 61, 478–487. doi: 10.1016/j.chb.2016.03.038
Whaite, E. O., Shensa, A., Sidani, J. E., Colditz, J. B., and Primack, B. A. (2018). Social media use, personality characteristics, and social isolation among young adults in the United States. Pers. Indiv. Differ. 124, 45–50. doi: 10.1016/j.paid.2017.10.030
Williams, D. (2006). On and off the'net: scales for social capital in an online era. J. Comput. Mediat. Commun. 11, 593–628. doi: 10.1016/j.1083-6101.2006.00029.x
Keywords: smartphone addiction, social isolation, bonding social capital, bridging social capital, phubbing, social media use
Citation: Ostic D, Qalati SA, Barbosa B, Shah SMM, Galvan Vela E, Herzallah AM and Liu F (2021) Effects of Social Media Use on Psychological Well-Being: A Mediated Model. Front. Psychol. 12:678766. doi: 10.3389/fpsyg.2021.678766
Received: 10 March 2021; Accepted: 25 May 2021; Published: 21 June 2021.
Reviewed by:
Copyright © 2021 Ostic, Qalati, Barbosa, Shah, Galvan Vela, Herzallah and Liu. This is an open-access article distributed under the terms of the Creative Commons Attribution License (CC BY) . The use, distribution or reproduction in other forums is permitted, provided the original author(s) and the copyright owner(s) are credited and that the original publication in this journal is cited, in accordance with accepted academic practice. No use, distribution or reproduction is permitted which does not comply with these terms.
*Correspondence: Sikandar Ali Qalati, sidqalati@gmail.com ; 5103180243@stmail.ujs.edu.cn ; Esthela Galvan Vela, esthela.galvan@cetys.mx
† ORCID: Dragana Ostic orcid.org/0000-0002-0469-1342 Sikandar Ali Qalati orcid.org/0000-0001-7235-6098 Belem Barbosa orcid.org/0000-0002-4057-360X Esthela Galvan Vela orcid.org/0000-0002-8778-3989 Feng Liu orcid.org/0000-0001-9367-049X
Disclaimer: All claims expressed in this article are solely those of the authors and do not necessarily represent those of their affiliated organizations, or those of the publisher, the editors and the reviewers. Any product that may be evaluated in this article or claim that may be made by its manufacturer is not guaranteed or endorsed by the publisher.
Thank you for visiting nature.com. You are using a browser version with limited support for CSS. To obtain the best experience, we recommend you use a more up to date browser (or turn off compatibility mode in Internet Explorer). In the meantime, to ensure continued support, we are displaying the site without styles and JavaScript.
- View all journals
- My Account Login
- Explore content
- About the journal
- Publish with us
- Sign up for alerts
- Open access
- Published: 01 July 2020
The effect of social media on well-being differs from adolescent to adolescent
- Ine Beyens ORCID: orcid.org/0000-0001-7023-867X 1 ,
- J. Loes Pouwels ORCID: orcid.org/0000-0002-9586-392X 1 ,
- Irene I. van Driel ORCID: orcid.org/0000-0002-7810-9677 1 ,
- Loes Keijsers ORCID: orcid.org/0000-0001-8580-6000 2 &
- Patti M. Valkenburg ORCID: orcid.org/0000-0003-0477-8429 1
Scientific Reports volume 10 , Article number: 10763 ( 2020 ) Cite this article
122k Accesses
206 Citations
119 Altmetric
Metrics details
- Human behaviour
The question whether social media use benefits or undermines adolescents’ well-being is an important societal concern. Previous empirical studies have mostly established across-the-board effects among (sub)populations of adolescents. As a result, it is still an open question whether the effects are unique for each individual adolescent. We sampled adolescents’ experiences six times per day for one week to quantify differences in their susceptibility to the effects of social media on their momentary affective well-being. Rigorous analyses of 2,155 real-time assessments showed that the association between social media use and affective well-being differs strongly across adolescents: While 44% did not feel better or worse after passive social media use, 46% felt better, and 10% felt worse. Our results imply that person-specific effects can no longer be ignored in research, as well as in prevention and intervention programs.
Similar content being viewed by others
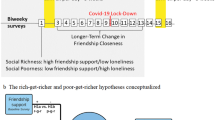
Some socially poor but also some socially rich adolescents feel closer to their friends after using social media
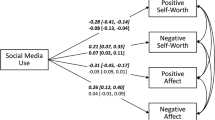
Associations between youth’s daily social media use and well-being are mediated by upward comparisons
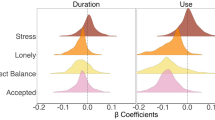
Variation in social media sensitivity across people and contexts
Introduction.
Ever since the introduction of social media, such as Facebook and Instagram, researchers have been studying whether the use of such media may affect adolescents’ well-being. These studies have typically reported mixed findings, yielding either small negative, small positive, or no effects of the time spent using social media on different indicators of well-being, such as life satisfaction and depressive symptoms (for recent reviews, see for example 1 , 2 , 3 , 4 , 5 ). Most of these studies have focused on between-person associations, examining whether adolescents who use social media more (or less) often than their peers experience lower (or higher) levels of well-being than these peers. While such between-person studies are valuable in their own right, several scholars 6 , 7 have recently called for studies that investigate within-person associations to understand whether an increase in an adolescent’s social media use is associated with an increase or decrease in that adolescent’s well-being. The current study aims to respond to this call by investigating associations between social media use and well-being within single adolescents across multiple points in time 8 , 9 , 10 .
Person-specific effects
To our knowledge, four recent studies have investigated within-person associations of social media use with different indicators of adolescent well-being (i.e., life satisfaction, depression), again with mixed results 6 , 11 , 12 , 13 . Orben and colleagues 6 found a small negative reciprocal within-person association between the time spent using social media and life satisfaction. Likewise, Boers and colleagues 12 found a small within-person association between social media use and increased depressive symptoms. Finally, Coyne and colleagues 11 and Jensen and colleagues 13 did not find any evidence for within-person associations between social media use and depression.
Earlier studies that investigated within-person associations of social media use with indicators of well-being have all only reported average effect sizes. However, it is possible, or even plausible, that these average within-person effects may have been small and nonsignificant because they result from sizeable heterogeneity in adolescents’ susceptibility to the effects of social media use on well-being (see 14 , 15 ). After all, an average within-person effect size can be considered an aggregate of numerous individual within-person effect sizes that range from highly positive to highly negative.
Some within-person studies have sought to understand adolescents’ differential susceptibility to the effects of social media by investigating differences between subgroups. For instance, they have investigated the moderating role of sex to compare the effects of social media on boys versus girls 6 , 11 . However, such a group-differential approach, in which potential differences in susceptibility are conceptualized by group-level moderators (e.g., gender, age) does not provide insights into more fine-grained differences at the level of the single individual 16 . After all, while girls and boys each represent a homogenous group in terms of sex, they may each differ on a wide array of other factors.
As such, although worthwhile, the average within-person effects of social media on well-being obtained in previous studies may have been small or non-significant because they are diluted across a highly heterogeneous population (or sub-population) of adolescents 14 , 15 . In line with the proposition of media effects theories that each adolescent may have a unique susceptibility to the effects of social media 17 , a viable explanation for the small and inconsistent findings in earlier studies may be that the effect of social media differs from adolescent to adolescent. The aim of the current study is to investigate this hypothesis and to obtain a better understanding of adolescents’ unique susceptibility to the effects of social media on their affective well-being.
Social media and affective well-being
Within-person studies have provided important insights into the associations of social media use with cognitive well-being (e.g., life satisfaction 6 ), which refers to adolescents’ cognitive judgment of how satisfied they are with their life 18 . However, the associations of social media use with adolescents’ affective well-being (i.e., adolescents’ affective evaluations of their moods and emotions 18 ) are still unknown. In addition, while earlier within-person studies have focused on associations with trait-like conceptualizations of well-being 11 , 12 , 13 , that is, adolescents’ average well-being across specific time periods 18 , there is a lack of studies that focus on well-being as a momentary affective state. Therefore, we extend previous research by examining the association between adolescents’ social media use and their momentary affective well-being. Like earlier experience sampling (ESM) studies among adults 19 , 20 , we measured adolescents’ momentary affective well-being with a single item. Adolescents’ momentary affective well-being was defined as their current feelings of happiness, a commonly used question to measure well-being 21 , 22 , which has high convergent validity, as evidenced by the strong correlations with the presence of positive affect and absence of negative affect.
To assess adolescents’ momentary affective well-being (henceforth referred to as well-being), we conducted a week-long ESM study among 63 middle adolescents ages 14 and 15. Six times a day, adolescents were asked to complete a survey using their own mobile phone, covering 42 assessments per adolescent, assessing their affective well-being and social media use. In total, adolescents completed 2,155 assessments (83.2% average compliance).
We focused on middle adolescence, since this is the period in life characterized by most significant fluctuations in well-being 23 , 24 . Also, in comparison to early and late adolescents, middle adolescents are more sensitive to reactions from peers and have a strong tendency to compare themselves with others on social media and beyond. Because middle adolescents typically use different social media platforms, in a complementary way 25 , 26 , 27 , each adolescent reported on his/her use of the three social media platforms that s/he used most frequently out of the five most popular social media platforms among adolescents: WhatsApp, followed by Instagram, Snapchat, YouTube, and, finally, the chat function of games 28 . In addition to investigating the association between overall social media use and well-being (i.e., the summed use of adolescents’ three most frequently used platforms), we examined the unique associations of the two most popular platforms, WhatsApp and Instagram 28 .
Like previous studies on social media use and well-being, we distinguished between active social media use (i.e., “activities that facilitate direct exchanges with others” 29 ) and passive social media use (i.e., “consuming information without direct exchanges” 29 ). Within-person studies among young adults have shown that passive but not active social media use predicts decreases in well-being 29 . Therefore, we examined the unique associations of adolescents’ overall active and passive social media use with their well-being, as well as active and passive use of Instagram and WhatsApp, specifically. We investigated categorical associations, that is, whether adolescents would feel better or worse if they had actively or passively used social media. And we investigated dose–response associations to understand whether adolescents’ well-being would change as a function of the time they had spent actively or passively using social media.
The hypotheses and the design, sampling and analysis plan were preregistered prior to data collection and are available on the Open Science Framework, along with the code used in the analyses ( https://osf.io/nhks2 ). For details about the design of the study and analysis approach, see Methods.
In more than half of all assessments (68.17%), adolescents had used social media (i.e., one or more of their three favorite social media platforms), either in an active or passive way. Instagram (50.90%) and WhatsApp (53.52%) were used in half of all assessments. Passive use of social media (66.21% of all assessments) was more common than active use (50.86%), both on Instagram (48.48% vs. 20.79%) and WhatsApp (51.25% vs. 40.07%).
Strong positive between-person correlations were found between the duration of active and passive social media use (overall: r = 0.69, p < 0.001; Instagram: r = 0.38, p < 0.01; WhatsApp: r = 0.85, p < 0.001): Adolescents who had spent more time actively using social media than their peers, had also spent more time passively using social media than their peers. Likewise, strong positive within-person correlations were found between the duration of active and passive social media use (overall: r = 0.63, p < 0.001; Instagram: r = 0.37, p < 0.001; WhatsApp: r = 0.57, p < 0.001): The more time an adolescent had spent actively using social media at a certain moment, the more time s/he had also spent passively using social media at that moment.
Table 1 displays the average number of minutes that adolescents had spent using social media in the past hour at each assessment, and the zero-order between- and within-person correlations between the duration of social media use and well-being. At the between-person level, the duration of active and passive social media use was not associated with well-being: Adolescents who had spent more time actively or passively using social media than their peers did not report significantly higher or lower levels of well-being than their peers. At the within-person level, significant but weak positive correlations were found between the duration of active and passive overall social media use and well-being. This indicates that adolescents felt somewhat better at moments when they had spent more time actively or passively using social media (overall), compared to moments when they had spent less time actively or passively using social media. When looking at specific platforms, a positive correlation was only found for passive WhatsApp use, but not for active WhatsApp use, and not for active and passive Instagram use.
Average and person-specific effects
The within-person associations of social media use with well-being and differences in these associations were tested in a series of multilevel models. We ran separate models for overall social media use (i.e., active use and passive use of adolescents’ three favorite social media platforms, see Table 2 ), Instagram use (see Table 3 ), and WhatsApp use (see Table 4 ). In a first step we examined the average categorical associations for each of these three social media uses using fixed effects models (Models 1A, 3A, and 5A) to investigate whether, on average, adolescents would feel better or worse at moments when they had used social media compared to moments when they had not (i.e., categorical predictors: active use versus no active use, and passive use versus no passive use). In a second step, we examined heterogeneity in the within-person categorical associations by adding random slopes to the fixed effects models (Models 1B, 3B, and 5B). Next, we examined the average dose–response associations using fixed effects models (Models 2A, 4A, and 6A), to investigate whether, on average, adolescents would feel better or worse when they had spent more time using social media (i.e., continuous predictors: duration of active use and duration of passive use). Finally, we examined heterogeneity in the within-person dose–response associations by adding random slopes to the fixed effects models (Models 2B, 4B, and 6B).
Overall social media use.
The model with the categorical predictors (see Table 2 ; Model 1A) showed that, on average, there was no association between overall use and well-being: Adolescents’ well-being did not increase or decrease at moments when they had used social media, either in a passive or active way. However, evidence was found that the association of passive (but not active) social media use with well-being differed from adolescent to adolescent (Model 1B), with effect sizes ranging from − 0.24 to 0.68. For 44.26% of the adolescents the association was non-existent to small (− 0.10 < r < 0.10). However, for 45.90% of the adolescents there was a weak (0.10 < r < 0.20; 8.20%), moderate (0.20 < r < 0.30; 22.95%) or even strong positive ( r ≥ 0.30; 14.75%) association between overall passive social media use and well-being, and for almost one in ten (9.84%) adolescents there was a weak (− 0.20 < r < − 0.10; 6.56%) or moderate negative (− 0.30 < r < − 0.20; 3.28%) association.
The model with continuous predictors (Model 2A) showed that, on average, there was a significant dose–response association for active use. At moments when adolescents had used social media, the time they spent actively (but not passively) using social media was positively associated with well-being: Adolescents felt better at moments when they had spent more time sending messages, posting, or sharing something on social media. The associations of the time spent actively and passively using social media with well-being did not differ across adolescents (Model 2B).
Instagram use
As shown in Model 3A in Table 3 , on average, there was a significant categorical association between passive (but not active) Instagram use and well-being: Adolescents experienced an increase in well-being at moments when they had passively used Instagram (i.e., viewing posts/stories of others). Adolescents did not experience an increase or decrease in well-being when they had actively used Instagram. The associations of passive and active Instagram use with well-being did not differ across adolescents (Model 3B).
On average, no significant dose–response association was found for Instagram use (Model 4A): At moments when adolescents had used Instagram, the time adolescents spent using Instagram (either actively or passively) was not associated with their well-being. However, evidence was found that the association of the time spent passively using Instagram differed from adolescent to adolescent (Model 4B), with effect sizes ranging from − 0.48 to 0.27. For most adolescents (73.91%) the association was non-existent to small (− 0.10 < r < 0.10), but for almost one in five adolescents (17.39%) there was a weak (0.10 < r < 0.20; 10.87%) or moderate (0.20 < r < 0.30; 6.52%) positive association, and for almost one in ten adolescents (8.70%) there was a weak (− 0.20 < r < − 0.10; 2.17%), moderate (− 0.30 < r < − 0.20; 4.35%), or strong ( r ≤ − 0.30; 2.17%) negative association. Figure 1 illustrates these differences in the dose–response associations.
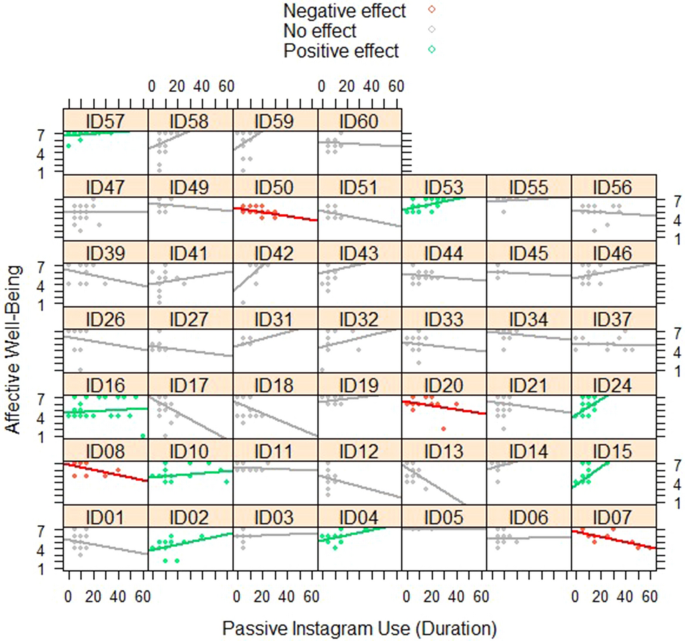
The dose–response association between passive Instagram use (in minutes per hour) and affective well-being for each individual adolescent (n = 46). Red lines represent significant negative within-person associations, green lines represent significant positive within-person associations, and gray lines represent non-significant within-person associations. A graph was created for each participant who had completed at least 10 assessments. A total of 13 participants were excluded because they had completed less than 10 assessments of passive Instagram use. In addition, one participant was excluded because no graph could be computed, since this participant's passive Instagram use was constant across assessments.
WhatsApp use
As shown in Model 5A in Table 4 , just as for Instagram, we found that, on average, there was a significant categorical association between passive (but not active) WhatsApp use and well-being: Adolescents reported that they felt better at moments when they had passively used WhatsApp (i.e., read WhatsApp messages). For active WhatsApp use, no significant association was found. Also, in line with the results for Instagram use, no differences were found regarding the associations of active and passive WhatsApp use (Model 5B).
In addition, a significant dose–response association was found for passive (but not active) use (Model 6A). At moments when adolescents had used WhatsApp, we found that, on average, the time adolescents spent passively using WhatsApp was positively associated with well-being: Adolescents felt better at moments when they had spent more time reading WhatsApp messages. The time spent actively using WhatsApp was not associated with well-being. No differences were found in the dose–response associations of active and passive WhatsApp use (Model 6B).
This preregistered study investigated adolescents’ unique susceptibility to the effects of social media. We found that the associations of passive (but not active) social media use with well-being differed substantially from adolescent to adolescent, with effect sizes ranging from moderately negative (− 0.24) to strongly positive (0.68). While 44.26% of adolescents did not feel better or worse if they had passively used social media, 45.90% felt better, and a small group felt worse (9.84%). In addition, for Instagram the majority of adolescents (73.91%) did not feel better or worse when they had spent more time viewing post or stories of others, whereas some felt better (17.39%), and others (8.70%) felt worse.
These findings have important implications for social media effects research, and media effects research more generally. For decades, researchers have argued that people differ in their susceptibility to the effects of media 17 , leading to numerous investigations of such differential susceptibility. These investigations have typically focused on moderators, based on variables such as sex, age, or personality. Yet, over the years, studies have shown that such moderators appear to have little power to explain how individuals differ in their susceptibility to media effects, probably because a group-differential approach does not account for the possibility that media users may differ across a range of factors, that are not captured by only one (or a few) investigated moderator variables.
By providing insights into each individual’s unique susceptibility, the findings of this study provide an explanation as to why, up until now, most media effects research has only found small effects. We found that the majority of adolescents do not experience any short-term changes in well-being related to their social media use. And if they do experience any changes, these are more often positive than negative. Because only small subsets of adolescents experience small to moderate changes in well-being, the true effects of social media reported in previous studies have probably been diluted across heterogeneous samples of individuals that differ in their susceptibility to media effects (also see 30 ). Several scholars have noted that overall effect sizes may mask more subtle individual differences 14 , 15 , which may explain why previous studies have typically reported small or no effects of social media on well-being or indicators of well-being 6 , 11 , 12 , 13 . The current study seems to confirm this assumption, by showing that while the overall effect sizes are small at best, the person-specific effect sizes vary considerably, from tiny and small to moderate and strong.
As called upon by other scholars 5 , 31 , we disentangled the associations of active and passive use of social media. Research among young adults found that passive (but not active) social media use is associated with lower levels of affective well-being 29 . In line with these findings, the current study shows that active and passive use yielded different associations with adolescents’ affective well-being. Interestingly though, in contrast to previous findings among adults, our study showed that, on average, passive use of Instagram and WhatsApp seemed to enhance rather than decrease adolescents’ well-being. This discrepancy in findings may be attributed to the fact that different mechanisms might be involved. Verduyn and colleagues 29 found that passive use of Facebook undermines adults’ well-being by enhancing envy, which may also explain the decreases in well-being found in our study among a small group of adolescents. Yet, adolescents who felt better by passively using Instagram and WhatsApp, might have felt so because they experienced enjoyment. After all, adolescents often seek positive content on social media, such as humorous posts or memes 32 . Also, research has shown that adolescents mainly receive positive feedback on social media 33 . Hence, their passive Instagram and WhatsApp use may involve the reading of positive feedback, which may explain the increases in well-being.
Overall, the time spent passively using WhatsApp improved adolescents’ well-being. This did not differ from adolescent to adolescent. However, the associations of the time spent passively using Instagram with well-being did differ from adolescent to adolescent. This discrepancy suggests that not all social media uses yield person-specific effects on well-being. A possible explanation may be that adolescents’ responses to WhatsApp are more homogenous than those to Instagram. WhatsApp is a more private platform, which is mostly used for one-to-one communication with friends and acquaintances 26 . Instagram, in contrast, is a more public platform, which allows its users to follow a diverse set of people, ranging from best friends to singers, actors, and influencers 28 , and to engage in intimate communication as well as self-presentation and social comparison. Such diverse uses could lead to more varied, or even opposing responses, such as envy versus inspiration.
Limitations and directions for future research
The current study extends our understanding of differential susceptibility to media effects, by revealing that the effect of social media use on well-being differs from adolescent to adolescent. The findings confirm our assumption that among the great majority of adolescents, social media use is unrelated to well-being, but that among a small subset, social media use is either related to decreases or increases in well-being. It must be noted, however, that participants in this study felt relatively happy, overall. Studies with more vulnerable samples, consisting of clinical samples or youth with lower social-emotional well-being may elicit different patterns of effects 27 . Also, the current study focused on affective well-being, operationalized as happiness. It is plausible that social media use relates differently with other types of well-being, such as cognitive well-being. An important next step is to identify which adolescents are particularly susceptible to experience declines in well-being. It is conceivable, for instance, that the few adolescents who feel worse when they use social media are the ones who receive negative feedback on social media 33 .
In addition, future ESM studies into the effects of social media should attempt to include one or more follow-up measures to improve our knowledge of the longer-term influence of social media use on affective well-being. While a week-long ESM is very common and applied in most earlier ESM studies 34 , a week is only a snapshot of adolescent development. Research is needed that investigates whether the associations of social media use with adolescents’ momentary affective well-being may cumulate into long-lasting consequences. Such investigations could help clarify whether adolescents who feel bad in the short term would experience more negative consequences in the long term, and whether adolescents who feel better would be more resistant to developing long-term negative consequences. And while most adolescents do not seem to experience any short-term increases or decreases in well-being, more research is needed to investigate whether these adolescents may experience a longer-term impact of social media.
While the use of different platforms may be differently associated with well-being, different types of use may also yield different effects. Although the current study distinguished between active and passive use of social media, future research should further differentiate between different activities. For instance, because passive use entails many different activities, from reading private messages (e.g., WhatsApp messages, direct messages on Instagram) to browsing a public feed (e.g., scrolling through posts on Instagram), research is needed that explores the unique effects of passive public use and passive private use. Research that seeks to explore the nuances in adolescents’ susceptibility as well as the nuances in their social media use may truly improve our understanding of the effects of social media use.
Participants
Participants were recruited via a secondary school in the south of the Netherlands. Our preregistered sampling plan set a target sample size of 100 adolescents. We invited adolescents from six classrooms to participate in the study. The final sample consisted of 63 adolescents (i.e., 42% consent rate, which is comparable to other ESM studies among adolescents; see, for instance 35 , 36 ). Informed consent was obtained from all participants and their parents. On average, participants were 15 years old ( M = 15.12 years, SD = 0.51) and 54% were girls. All participants self-identified as Dutch, and 41.3% were enrolled in the prevocational secondary education track, 25.4% in the intermediate general secondary education track, and 33.3% in the academic preparatory education track.
The study was approved by the Ethics Review Board of the Faculty of Social and Behavioral Sciences at the University of Amsterdam and was performed in accordance with the guidelines formulated by the Ethics Review Board. The study consisted of two phases: A baseline survey and a personalized week-long experience sampling (ESM) study. In phase 1, researchers visited the school during school hours. Researchers informed the participants of the objective and procedure of the study and assured them that their responses would be treated confidentially. Participants were asked to sign the consent form. Next, participants completed a 15-min baseline survey. The baseline survey included questions about demographics and assessed which social media each adolescent used most frequently, allowing to personalize the social media questions presented during the ESM study in phase 2. After completing the baseline survey, participants were provided detailed instructions about phase 2.
In phase 2, which took place two and a half weeks after the baseline survey, a 7-day ESM study was conducted, following the guidelines for ESM studies provided by van Roekel and colleagues 34 . Aiming for at least 30 assessments per participant and based on an average compliance rate of 70 to 80% reported in earlier ESM studies among adolescents 34 , we asked each participant to complete a total of 42 ESM surveys (i.e., six 2-min surveys per day). Participants completed the surveys using their own mobile phone, on which the ESM software application Ethica Data was installed during the instruction session with the researchers (phase 1). Each 2-min survey consisted of 22 questions, which assessed adolescents’ well-being and social media use. Two open-ended questions were added to the final survey of the day, which asked about adolescents’ most pleasant and most unpleasant events of the day.
The ESM sampling scheme was semi-random, to allow for randomization and avoid structural patterns in well-being, while taking into account that adolescents were not allowed to use their phone during school time. The Ethica Data app was programmed to generate six beep notifications per day at random time points within a fixed time interval that was tailored to the school’s schedule: before school time (1 beep), during school breaks (2 beeps), and after school time (3 beeps). During the weekend, the beeps were generated during the morning (1 beep), afternoon (3 beeps), and evening (2 beeps). To maximize compliance, a 30-min time window was provided to complete each survey. This time window was extended to one hour for the first survey (morning) and two hours for the final survey (evening) to account for travel time to school and time spent on evening activities. The average compliance rate was 83.2%. A total of 2,155 ESM assessments were collected: Participants completed an average of 34.83 surveys ( SD = 4.91) on a total of 42 surveys, which is high compared to previous ESM studies among adolescents 34 .
The questions of the ESM study were personalized based on the responses to the baseline survey. During the ESM study, each participant reported on his/her use of three different social media platforms: WhatsApp and either Instagram, Snapchat, YouTube, and/or the chat function of games (i.e., the most popular social media platforms among adolescents 28 ). Questions about Instagram and WhatsApp use were only included if the participant had indicated in the baseline survey that s/he used these platforms at least once a week. If a participant had indicated that s/he used Instagram or WhatsApp (or both) less than once a week, s/he was asked to report on the use of Snapchat, YouTube, or the chat function of games, depending on what platform s/he used at least once a week. In addition to Instagram and WhatsApp, questions were asked about a third platform, that was selected based on how frequently the participant used Snapchat, YouTube, or the chat function of games (i.e., at least once a week). This resulted in five different combinations of three platforms: Instagram, WhatsApp, and Snapchat (47 participants); Instagram, WhatsApp, and YouTube (11 participants); Instagram, WhatsApp, and chatting via games (2 participants); WhatsApp, Snapchat, and YouTube (1 participant); and WhatsApp, YouTube, and chatting via games (2 participants).
Frequency of social media use
In the baseline survey, participants were asked to indicate how often they used and checked Instagram, WhatsApp, Snapchat, YouTube, and the chat function of games, using response options ranging from 1 ( never ) to 7 ( more than 12 times per day ). These platforms are the five most popular platforms among Dutch 14- and 15-year-olds 28 . Participants’ responses were used to select the three social media platforms that were assessed in the personalized ESM study.
Duration of social media use
In the ESM study, duration of active and passive social media use was measured by asking participants how much time in the past hour they had spent actively and passively using each of the three platforms that were included in the personalized ESM surveys. Response options ranged from 0 to 60 min , with 5-min intervals. To measure active Instagram use, participants indicated how much time in the past hour they had spent (a) “posting on your feed or sharing something in your story on Instagram” and (b) “sending direct messages/chatting on Instagram.” These two items were summed to create the variable duration of active Instagram use. Sum scores exceeding 60 min (only 0.52% of all assessments) were recoded to 60 min. To measure duration of passive Instagram use, participants indicated how much time in the past hour they had spent “viewing posts/stories of others on Instagram.” To measure the use of WhatsApp, Snapchat, YouTube and game-based chatting, we asked participants how much time they had spent “sending WhatsApp messages” (active use) and “reading WhatsApp messages” (passive use); “sending snaps/messages or sharing something in your story on Snapchat” (active use) and “viewing snaps/stories/messages from others on Snapchat” (passive use); “posting YouTube clips” (active use) and “watching YouTube clips” (passive use); “sending messages via the chat function of a game/games” (active use) and “reading messages via the chat function of a game/games” (passive use). Duration of active and passive overall social media use were created by summing the responses across the three social media platforms for active and passive use, respectively. Sum scores exceeding 60 min (2.13% of all assessments for active overall use; 2.90% for passive overall use) were recoded to 60 min. The duration variables were used to investigate whether the time spent actively or passively using social media was associated with well-being (dose–response associations).
Use/no use of social media
Based on the duration variables, we created six dummy variables, one for active and one for passive overall social media use, one for active and one for passive Instagram use, and one for active and one for passive WhatsApp use (0 = no active use and 1 = active use , and 0 = no passive use and 1 = passive use , respectively). These dummy variables were used to investigate whether the use of social media, irrespective of the duration of use, was associated with well-being (categorical associations).
Consistent with previous ESM studies 19 , 20 , we measured affective well-being using one item, asking “How happy do you feel right now?” at each assessment. Adolescents indicated their response to the question using a 7-point scale ranging from 1 ( not at all ) to 7 ( completely ), with 4 ( a little ) as the midpoint. Convergent validity of this item was established in a separate pilot ESM study among 30 adolescents conducted by the research team of the fourth author: The affective well-being item was strongly correlated with the presence of positive affect and absence of negative affect (assessed by a 10-item positive and negative affect schedule for children; PANAS-C) at both the between-person (positive affect: r = 0.88, p < 0.001; negative affect: r = − 0.62, p < 0.001) and within-person level (positive affect: r = 0.74, p < 0.001; negative affect: r = − 0.58, p < 0.001).
Statistical analyses
Before conducting the analyses, several validation checks were performed (see 34 ). First, we aimed to only include participants in the analyses who had completed more than 33% of all ESM assessments (i.e., at least 14 assessments). Next, we screened participants’ responses to the open questions for unserious responses (e.g., gross comments, jokes). And finally, we inspected time series plots for patterns in answering tendencies. Since all participants completed more than 33% of all ESM assessments, and no inappropriate responses or low-quality data patterns were detected, all participants were included in the analyses.
Following our preregistered analysis plan, we tested the proposed associations in a series of multilevel models. Before doing so, we tested the homoscedasticity and linearity assumptions for multilevel analyses 37 . Inspection of standardized residual plots indicated that the data met these assumptions (plots are available on OSF at https://osf.io/nhks2 ). We specified separate models for overall social media use, use of Instagram, and use of WhatsApp. To investigate to what extent adolescents’ well-being would vary depending on whether they had actively or passively used social media/Instagram/WhatsApp or not during the past hour (categorical associations), we tested models including the dummy variables as predictors (active use versus no active use, and passive use versus no passive use; models 1, 3, and 5). To investigate whether, at moments when adolescents had used social media/Instagram/WhatsApp during the past hour, their well-being would vary depending on the duration of social media/Instagram/WhatsApp use (dose–response associations), we tested models including the duration variables as predictors (duration of active use and duration of passive use; models 2, 4, and 6). In order to avoid negative skew in the duration variables, we only included assessments during which adolescents had used social media in the past hour (overall, Instagram, or WhatsApp, respectively), either actively or passively. All models included well-being as outcome variable. Since multilevel analyses allow to include all available data for each individual, no missing data were imputed and no data points were excluded.
We used a model building approach that involved three steps. In the first step, we estimated an intercept-only model to assess the relative amount of between- and within-person variance in affective well-being. We estimated a three-level model in which repeated momentary assessments (level 1) were nested within adolescents (level 2), who, in turn, were nested within classrooms (level 3). However, because the between-classroom variance in affective well-being was small (i.e., 0.4% of the variance was explained by differences between classes), we proceeded with estimating two-level (instead of three-level) models, with repeated momentary assessments (level 1) nested within adolescents (level 2).
In the second step, we assessed the within-person associations of well-being with (a) overall active and passive social media use (i.e., the total of the three platforms), (b) active and passive use of Instagram, and (c) active and passive use of WhatsApp, by adding fixed effects to the model (Models 1A-6A). To facilitate the interpretation of the associations and control for the effects of time, a covariate was added that controlled for the n th assessment of the study week (instead of the n th assessment of the day, as preregistered). This so-called detrending is helpful to interpret within-person associations as correlated fluctuations beyond other changes in social media use and well-being 38 . In order to obtain within-person estimates, we person-mean centered all predictors 38 . Significance of the fixed effects was determined using the Wald test.
In the third and final step, we assessed heterogeneity in the within-person associations by adding random slopes to the models (Models 1B-6B). Significance of the random slopes was determined by comparing the fit of the fixed effects model with the fit of the random effects model, by performing the Satorra-Bentler scaled chi-square test 39 and by comparing the Bayesian information criterion (BIC 40 ) and Akaike information criterion (AIC 41 ) of the models. When the random effects model had a significantly better fit than the fixed effects model (i.e., pointing at significant heterogeneity), variance components were inspected to investigate whether heterogeneity existed in the association of either active or passive use. Next, when evidence was found for significant heterogeneity, we computed person-specific effect sizes, based on the random effect models, to investigate what percentages of adolescents experienced better well-being, worse well-being, and no changes in well-being. In line with Keijsers and colleagues 42 we only included participants who had completed at least 10 assessments. In addition, for the dose–response associations, we constructed graphical representations of the person-specific slopes, based on the person-specific effect sizes, using the xyplot function from the lattice package in R 43 .
Three improvements were made to our original preregistered plan. First, rather than estimating the models with multilevel modelling in R 43 , we ran the preregistered models in Mplus 44 . Mplus provides standardized estimates for the fixed effects models, which offers insight into the effect sizes. This allowed us to compare the relative strength of the associations of passive versus active use with well-being. Second, instead of using the maximum likelihood estimator, we used the maximum likelihood estimator with robust standard errors (MLR), which are robust to non-normality. Sensitivity tests, uploaded on OSF ( https://osf.io/nhks2 ), indicated that the results were almost identical across the two software packages and estimation approaches. Third, to improve the interpretation of the results and make the scales of the duration measures of social media use and well-being more comparable, we transformed the social media duration scores (0 to 60 min) into scales running from 0 to 6, so that an increase of 1 unit reflects 10 min of social media use. The model estimates were unaffected by this transformation.
Reporting summary
Further information on the research design is available in the Nature Research Reporting Summary linked to this article.
Data availability
The dataset generated and analysed during the current study is available in Figshare 45 . The preregistration of the design, sampling and analysis plan, and the analysis scripts used to analyse the data for this paper are available online on the Open Science Framework website ( https://osf.io/nhks2 ).
Best, P., Manktelow, R. & Taylor, B. Online communication, social media and adolescent wellbeing: A systematic narrative review. Child Youth Serv. Rev. 41 , 27–36. https://doi.org/10.1016/j.childyouth.2014.03.001 (2014).
Article Google Scholar
James, C. et al. Digital life and youth well-being, social connectedness, empathy, and narcissism. Pediatrics 140 , S71–S75. https://doi.org/10.1542/peds.2016-1758F (2017).
Article PubMed Google Scholar
McCrae, N., Gettings, S. & Purssell, E. Social media and depressive symptoms in childhood and adolescence: A systematic review. Adolesc. Res. Rev. 2 , 315–330. https://doi.org/10.1007/s40894-017-0053-4 (2017).
Sarmiento, I. G. et al. How does social media use relate to adolescents’ internalizing symptoms? Conclusions from a systematic narrative review. Adolesc Res Rev , 1–24, doi:10.1007/s40894-018-0095-2 (2018).
Orben, A. Teenagers, screens and social media: A narrative review of reviews and key studies. Soc. Psychiatry Psychiatr. Epidemiol. https://doi.org/10.1007/s00127-019-01825-4 (2020).
Orben, A., Dienlin, T. & Przybylski, A. K. Social media’s enduring effect on adolescent life satisfaction. Proc. Natl. Acad. Sci. USA 116 , 10226–10228. https://doi.org/10.1073/pnas.1902058116 (2019).
Article CAS PubMed Google Scholar
Whitlock, J. & Masur, P. K. Disentangling the association of screen time with developmental outcomes and well-being: Problems, challenges, and opportunities. JAMA https://doi.org/10.1001/jamapediatrics.2019.3191 (2019).
Hamaker, E. L. In Handbook of Research Methods for Studying Daily Life (eds Mehl, M. R. & Conner, T. S.) 43–61 (Guilford Press, New York, 2012).
Schmiedek, F. & Dirk, J. In The Encyclopedia of Adulthood and Aging (ed. Krauss Whitbourne, S.) 1–6 (Wiley, 2015).
Keijsers, L. & van Roekel, E. In Reframing Adolescent Research (eds Hendry, L. B. & Kloep, M.) (Routledge, 2018).
Coyne, S. M., Rogers, A. A., Zurcher, J. D., Stockdale, L. & Booth, M. Does time spent using social media impact mental health? An eight year longitudinal study. Comput. Hum. Behav. 104 , 106160. https://doi.org/10.1016/j.chb.2019.106160 (2020).
Boers, E., Afzali, M. H., Newton, N. & Conrod, P. Association of screen time and depression in adolescence. JAMA 173 , 853–859. https://doi.org/10.1001/jamapediatrics.2019.1759 (2019).
Jensen, M., George, M. J., Russell, M. R. & Odgers, C. L. Young adolescents’ digital technology use and mental health symptoms: Little evidence of longitudinal or daily linkages. Clin. Psychol. Sci. https://doi.org/10.1177/2167702619859336 (2019).
Valkenburg, P. M. The limited informativeness of meta-analyses of media effects. Perspect. Psychol. Sci. 10 , 680–682. https://doi.org/10.1177/1745691615592237 (2015).
Pearce, L. J. & Field, A. P. The impact of “scary” TV and film on children’s internalizing emotions: A meta-analysis. Hum. Commun.. Res. 42 , 98–121. https://doi.org/10.1111/hcre.12069 (2016).
Howard, M. C. & Hoffman, M. E. Variable-centered, person-centered, and person-specific approaches. Organ. Res. Methods 21 , 846–876. https://doi.org/10.1177/1094428117744021 (2017).
Valkenburg, P. M. & Peter, J. The differential susceptibility to media effects model. J. Commun. 63 , 221–243. https://doi.org/10.1111/jcom.12024 (2013).
Eid, M. & Diener, E. Global judgments of subjective well-being: Situational variability and long-term stability. Soc. Indic. Res. 65 , 245–277. https://doi.org/10.1023/B:SOCI.0000003801.89195.bc (2004).
Kross, E. et al. Facebook use predicts declines in subjective well-being in young adults. PLoS ONE 8 , e69841. https://doi.org/10.1371/journal.pone.0069841 (2013).
Article ADS CAS PubMed PubMed Central Google Scholar
Reissmann, A., Hauser, J., Stollberg, E., Kaunzinger, I. & Lange, K. W. The role of loneliness in emerging adults’ everyday use of facebook—An experience sampling approach. Comput. Hum. Behav. 88 , 47–60. https://doi.org/10.1016/j.chb.2018.06.011 (2018).
Rutledge, R. B., Skandali, N., Dayan, P. & Dolan, R. J. A computational and neural model of momentary subjective well-being. Proc. Natl. Acad. Sci. USA 111 , 12252–12257. https://doi.org/10.1073/pnas.1407535111 (2014).
Article ADS CAS PubMed Google Scholar
Tov, W. In Handbook of Well-being (eds Diener, E.D. et al. ) (DEF Publishers, 2018).
Harter, S. The Construction of the Self: Developmental and Sociocultural Foundations (Guilford Press, New York, 2012).
Steinberg, L. Adolescence . Vol. 9 (McGraw-Hill, 2011).
Rideout, V. & Fox, S. Digital Health Practices, Social Media Use, and Mental Well-being Among Teens and Young Adults in the US (HopeLab, San Francisco, 2018).
Google Scholar
Waterloo, S. F., Baumgartner, S. E., Peter, J. & Valkenburg, P. M. Norms of online expressions of emotion: Comparing Facebook, Twitter, Instagram, and WhatsApp. New Media Soc. 20 , 1813–1831. https://doi.org/10.1177/1461444817707349 (2017).
Article PubMed PubMed Central Google Scholar
Rideout, V. & Robb, M. B. Social Media, Social Life: Teens Reveal their Experiences (Common Sense Media, San Fransico, 2018).
van Driel, I. I., Pouwels, J. L., Beyens, I., Keijsers, L. & Valkenburg, P. M. 'Posting, Scrolling, Chatting & Snapping': Youth (14–15) and Social Media in 2019 (Center for Research on Children, Adolescents, and the Media (CcaM), Universiteit van Amsterdam, 2019).
Verduyn, P. et al. Passive Facebook usage undermines affective well-being: Experimental and longitudinal evidence. J. Exp. Psychol. 144 , 480–488. https://doi.org/10.1037/xge0000057 (2015).
Valkenburg, P. M. & Peter, J. Five challenges for the future of media-effects research. Int. J. Commun. 7 , 197–215 (2013).
Verduyn, P., Ybarra, O., Résibois, M., Jonides, J. & Kross, E. Do social network sites enhance or undermine subjective well-being? A critical review. Soc. Issues Policy Rev. 11 , 274–302. https://doi.org/10.1111/sipr.12033 (2017).
Radovic, A., Gmelin, T., Stein, B. D. & Miller, E. Depressed adolescents’ positive and negative use of social media. J. Adolesc. 55 , 5–15. https://doi.org/10.1016/j.adolescence.2016.12.002 (2017).
Valkenburg, P. M., Peter, J. & Schouten, A. P. Friend networking sites and their relationship to adolescents’ well-being and social self-esteem. Cyberpsychol. Behav. 9 , 584–590. https://doi.org/10.1089/cpb.2006.9.584 (2006).
van Roekel, E., Keijsers, L. & Chung, J. M. A review of current ambulatory assessment studies in adolescent samples and practical recommendations. J. Res. Adolesc. 29 , 560–577. https://doi.org/10.1111/jora.12471 (2019).
van Roekel, E., Scholte, R. H. J., Engels, R. C. M. E., Goossens, L. & Verhagen, M. Loneliness in the daily lives of adolescents: An experience sampling study examining the effects of social contexts. J. Early Adolesc. 35 , 905–930. https://doi.org/10.1177/0272431614547049 (2015).
Neumann, A., van Lier, P. A. C., Frijns, T., Meeus, W. & Koot, H. M. Emotional dynamics in the development of early adolescent psychopathology: A one-year longitudinal Study. J. Abnorm. Child Psychol. 39 , 657–669. https://doi.org/10.1007/s10802-011-9509-3 (2011).
Hox, J., Moerbeek, M. & van de Schoot, R. Multilevel Analysis: Techniques and Applications 3rd edn. (Routledge, London, 2018).
Wang, L. P. & Maxwell, S. E. On disaggregating between-person and within-person effects with longitudinal data using multilevel models. Psychol. Methods 20 , 63–83. https://doi.org/10.1037/met0000030 (2015).
Satorra, A. & Bentler, P. M. Ensuring positiveness of the scaled difference chi-square test statistic. Psychometrika 75 , 243–248. https://doi.org/10.1007/s11336-009-9135-y (2010).
Article MathSciNet PubMed PubMed Central MATH Google Scholar
Schwarz, G. Estimating the dimension of a model. Ann. Stat. 6 , 461–464. https://doi.org/10.1214/aos/1176344136 (1978).
Article MathSciNet MATH Google Scholar
Akaike, H. A new look at the statistical model identification. IEEE Trans. Autom. Control 19 , 716–723. https://doi.org/10.1109/TAC.1974.1100705 (1974).
Article ADS MathSciNet MATH Google Scholar
Keijsers, L. et al. What drives developmental change in adolescent disclosure and maternal knowledge? Heterogeneity in within-family processes. Dev. Psychol. 52 , 2057–2070. https://doi.org/10.1037/dev0000220 (2016).
R Core Team R: A Language and Environment for Statistical Computing. (R Foundation for Statistical Computing, Vienna, 2017).
Muthén, L. K. & Muthén, B. O. Mplus User’s Guide 8th edn. (Muthén & Muthén, Los Angeles, 2017).
Beyens, I., Pouwels, J. L., van Driel, I. I., Keijsers, L. & Valkenburg, P. M. Dataset belonging to Beyens et al. (2020). The effect of social media on well-being differs from adolescent to adolescent. https://doi.org/10.21942/uva.12497990 (2020).
Download references
Acknowledgements
This study was funded by the NWO Spinoza Prize and the Gravitation grant (NWO Grant 024.001.003; Consortium on Individual Development) awarded to P.M.V. by the Dutch Research Council (NWO). Additional funding was received from the VIDI grant (NWO VIDI Grant 452.17.011) awarded to L.K. by the Dutch Research Council (NWO). The authors would like to thank Savannah Boele (Tilburg University) for providing her pilot ESM results.
Author information
Authors and affiliations.
Amsterdam School of Communication Research, University of Amsterdam, 1001 NG, Amsterdam, The Netherlands
Ine Beyens, J. Loes Pouwels, Irene I. van Driel & Patti M. Valkenburg
Department of Developmental Psychology, Tilburg University, 5000 LE, Tilburg, The Netherlands
Loes Keijsers
You can also search for this author in PubMed Google Scholar
Contributions
I.B., J.L.P., I.I.v.D., L.K., and P.M.V. designed the study; I.B., J.L.P., and I.I.v.D. collected the data; I.B., J.L.P., and L.K. analyzed the data; and I.B., J.L.P., I.I.v.D., L.K., and P.M.V. contributed to writing and reviewing the manuscript.
Corresponding author
Correspondence to Ine Beyens .
Ethics declarations
Competing interests.
The authors declare no competing interests.
Additional information
Publisher's note.
Springer Nature remains neutral with regard to jurisdictional claims in published maps and institutional affiliations.
Rights and permissions
Open Access This article is licensed under a Creative Commons Attribution 4.0 International License, which permits use, sharing, adaptation, distribution and reproduction in any medium or format, as long as you give appropriate credit to the original author(s) and the source, provide a link to the Creative Commons license, and indicate if changes were made. The images or other third party material in this article are included in the article’s Creative Commons license, unless indicated otherwise in a credit line to the material. If material is not included in the article’s Creative Commons license and your intended use is not permitted by statutory regulation or exceeds the permitted use, you will need to obtain permission directly from the copyright holder. To view a copy of this license, visit http://creativecommons.org/licenses/by/4.0/ .
Reprints and permissions
About this article
Cite this article.
Beyens, I., Pouwels, J.L., van Driel, I.I. et al. The effect of social media on well-being differs from adolescent to adolescent. Sci Rep 10 , 10763 (2020). https://doi.org/10.1038/s41598-020-67727-7
Download citation
Received : 24 January 2020
Accepted : 11 June 2020
Published : 01 July 2020
DOI : https://doi.org/10.1038/s41598-020-67727-7
Share this article
Anyone you share the following link with will be able to read this content:
Sorry, a shareable link is not currently available for this article.
Provided by the Springer Nature SharedIt content-sharing initiative
This article is cited by
Mechanisms linking social media use to adolescent mental health vulnerability.
- Adrian Meier
- Sarah-Jayne Blakemore
Nature Reviews Psychology (2024)
- Sumer S. Vaid
- Lara Kroencke
- Gabriella M. Harari
Scientific Reports (2024)
Social Media and Youth Mental Health: Assessing the Impact Through Current and Novel Digital Phenotyping Methods
- Elana Perlmutter
- Bridget Dwyer
- John Torous
Current Treatment Options in Psychiatry (2024)
Childhood internalizing, externalizing and attention symptoms predict changes in social and nonsocial screen time
- Katherine Keyes
- Ava Hamilton
- Noah Kreski
Social Psychiatry and Psychiatric Epidemiology (2024)
Problematic social media use and psychological symptoms in adolescents
- Ramin Mojtabai
By submitting a comment you agree to abide by our Terms and Community Guidelines . If you find something abusive or that does not comply with our terms or guidelines please flag it as inappropriate.
Quick links
- Explore articles by subject
- Guide to authors
- Editorial policies
Sign up for the Nature Briefing newsletter — what matters in science, free to your inbox daily.

Social Media Adoption, Usage And Impact In Business-To-Business (B2B) Context: A State-Of-The-Art Literature Review
- Open access
- Published: 02 February 2021
- Volume 25 , pages 971–993, ( 2023 )
Cite this article
You have full access to this open access article
- Yogesh K. Dwivedi 1 ,
- Elvira Ismagilova 2 ,
- Nripendra P. Rana 2 &
- Ramakrishnan Raman 3
98k Accesses
66 Citations
4 Altmetric
Explore all metrics
Social media plays an important part in the digital transformation of businesses. This research provides a comprehensive analysis of the use of social media by business-to-business (B2B) companies. The current study focuses on the number of aspects of social media such as the effect of social media, social media tools, social media use, adoption of social media use and its barriers, social media strategies, and measuring the effectiveness of use of social media. This research provides a valuable synthesis of the relevant literature on social media in B2B context by analysing, performing weight analysis and discussing the key findings from existing research on social media. The findings of this study can be used as an informative framework on social media for both, academic and practitioners.
Similar content being viewed by others
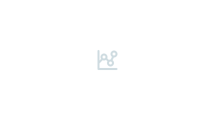
The future of social media in marketing
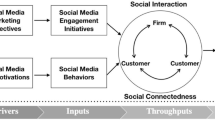
Social media marketing strategy: definition, conceptualization, taxonomy, validation, and future agenda
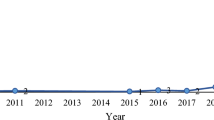
Social media influencer marketing: foundations, trends, and ways forward
Avoid common mistakes on your manuscript.
1 Introduction
The Internet has changed social communications and social behaviour, which lead to the development of new forms of communication channels and platforms (Ismagilova et al. 2017 ). Social media plays an important part in the digital transformation of businesses (Kunsman 2018 ). Digital transformation refers to the globally accelerated process of technical adaptation by companies and communities as a result of digitalisation (Sivarajah et al. 2019 ; Westerman et al. 2014 ). Web is developed from a tool used to provide passive information into the collaborative web, which allows and encourages active user engagement and contribution. If before social networks were used to provide the information about a company or brand, nowadays businesses use social media in their marketing aims and strategies to improve consumers’ involvement, relationship with customers and get useful consumers’ insights (Alalwan et al. 2017 ). Business-to-consumer (B2C) companies widely use social media as part of their digital transformation and enjoy its benefits such as an increase in sales, brand awareness, and customer engagement to name a few (Barreda et al. 2015 ; Chatterjee and Kar 2020 ; Harrigan et al. 2020 ; Kamboj et al. 2018 ; Kapoor et al. 2018 ).
From a marketing and sales research perspective, social media is defined as “the technological component of the communication, transaction and relationship building functions of a business which leverages the network of customers and prospects to promote value co-creation” (Andzulis et al. 2012 p.308). Industrial buyers use social media for their purchase as they compare products, research the market and build relationships with salesperson (Itani et al. 2017 ). Social media changed the way how buyers and sellers interact (Agnihotri et al. 2016 ) by enabling open and broad communications and cooperation between them (Rossmann and Stei 2015 ). Social media is an important facilitator of relationships between a company and customers (Agnihotri et al. 2012 ; Tedeschi 2006 ). Customers are more connected to companies, which make them more knowledgable about product selection and more powerful in buyer-seller relationships (Agnihotri et al. 2016 ). Social media also helps companies to increase business exposure, traffic and providing marketplace insight (Agnihotri et al. 2016 ; Stelzner 2011 ). As a result, the use of social media supports business decision processes and helps to improve companies’ performance (Rossmann and Stei 2015 ).
Due to digitalisation customers are becoming more informed and rely less on traditional selling initiatives (Ancillai et al. 2019 ). Buyers are relying more on digital resources and their buying process more often involves the use of social media. For example, in the research B2B buyer survey, 82% of buyers stated that social media content has a significant impact on the purchase decision (Ancillai et al. 2019 ; Minsky and Quesenberry 2016 ). As a result, these changes in consumer behaviour place high pressure on B2B salespeople and traditional sales companies (Ancillai et al. 2019 ). By using evidence from major B2B companies and consultancy report some studies claim that social media can be applied in sales to establish effective dialogues with buyers (Ancillai et al. 2019 ; Kovac 2016 ; McKinsey and Company 2015 ).
Now, business-to-business (B2B) companies started using social media as part of their digital transformation. 83% of B2B companies use social media, which makes it the most common marketing tactic (Pulizzi and Handley 2017 ; Sobal 2017 ). More than 70% of B2B companies use at least one of the “big 4” social media sites such as LinkedIn, Twitter, Facebook and YouTube. Additionally, 50% of the companies stated that social media has improved their marketing optimization and customer experience, while 25% stated that their revenue went up (Gregorio 2017 ; Sobal 2017 ). Even though B2B companies are benefitting from social media used by marketers, it is argued that research on that area is still in the embryonic stage and future research is needed (Salo 2017 ; Siamagka et al. 2015 ; Juntunen et al. 2020 ; Iannacci et al. 2020 ). There is a limited understanding of how B2B companies need to change to embrace recent technological innovations and how it can lead to business and societal transformation (Chen et al. 2012 ; Loebbecke and Picot 2015 ; Pappas et al. 2018 ).
The topic of social media in the context of B2B companies has started attracting attention from both academics and practitioners. This is evidenced by the growing number of research output within academic journals and conference proceedings. Some studies provided a comprehensive literature review on social media use by B2B companies (Pascucci et al. 2018 ; Salo 2017 ), but focused only on adoption of social media by B2B or social media influence, without providing the whole picture of the use of social media by B2B companies. Thus, this study aims to close this gap in the literature by conducting a comprehensive analysis of the use of social media by B2B companies and discuss its role in the digital transformation of B2B companies. The findings of this study can provide an informative framework for research on social media in the context of B2B companies for academics and practitioners.
The remaining sections of the study are organised as follows. Section 2 offers a brief overview of the methods used to identify relevant studies to be included in this review. Section 3 synthesises the studies identified in the previous section and provides a detailed overview. Section 4 presents weight analysis and its findings. Next section discusses the key aspects of the research, highlights any limitations within existing studies and explores the potential directions for future research. Finally, the paper is concluded in Section 6 .
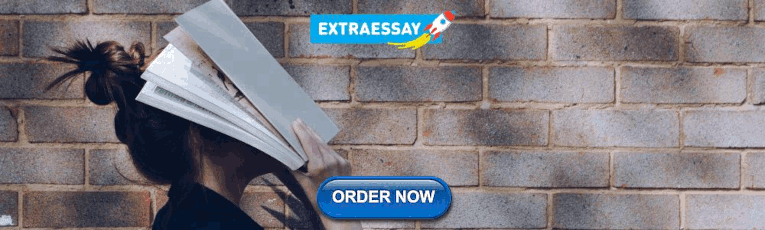
2 Literature Search Method
The approach utilised in this study aligns with the recommendations in Webster and Watson ( 2002 ). This study used a keyword search-based approach for identifying relevant articles (Dwivedi et al. 2019b ; Ismagilova et al. 2020a ; Ismagilova et al. 2019 ; Jeyaraj and Dwivedi 2020 ; Williams et al. 2015 ). Keywords such as “Advertising” OR “Marketing” OR “Sales” AND TITLE (“Social Media” OR “Web 2.0” OR “Facebook” OR “LinkedIn” OR “Instagram” OR “Twitter” OR “Snapchat” OR “Pinterest” OR “WhatsApp” OR “Social Networking Sites”) AND TITLE-ABS-KEY (“B2B” OR “B to B” OR “Business to Business” OR “Business 2 Business”) were searched via the Scopus database. Scopus database was chosen to ensure the inclusion of only high quality studies. Use of online databases for conducting a systematic literature review became an emerging culture used by a number of information systems research studies (Dwivedi et al. 2019a ; Gupta et al. 2019 ; Ismagilova et al. 2020b ; Muhammad et al. 2018 ; Rana et al. 2019 ). The search resulted in 80 articles. All studies were processed by the authors in order to ensure relevance and that the research offered a contribution to the social media in the context B2B discussion. The search and review resulted in 70 articles and conference papers that formed the literature review for this study. The selected studies appeared in 33 separate journals and conference proceedings, including journals such as Industrial Marketing Management, Journal of Business and Industrial Marketing and Journal of Business Research.
3 Literature Synthesis
The studies on social media research in the context of B2B companies were divided into the following themes: effect of social media, adoption of social media, social media strategies, social media use, measuring the effectiveness of use of social media, and social media tools (see Table 1 ). The following subsections provide an overview of each theme.
3.1 Effect of Social Media
Some studies focus on the effect of social media for B2B companies, which include customer satisfaction, value creation, intention to buy and sales, building relationships with customers, brand awareness, knowledge creation, perceived corporate credibility, acquiring of new customers, salesperson performance, employee brand engagement, and sustainability (Table 2 ).
3.1.1 Customer Satisfaction
Some studies investigated how the use of social media affected customer satisfaction (Agnihotri et al. 2016 ; Ancillai et al. 2019 ; Rossmann and Stei 2015 ). For example, Agnihotri et al. ( 2016 ) investigated how the implementation of social media by B2B salesperson affects consumer satisfaction. Salesperson’s social media use is defined as a “salesperson’s utilization and integration of social media technology to perform his or her job” (Agnihotri et al. 2016 , p.2). The study used data from 111 sales professionals involved in B2B industrial selling to test the proposed hypotheses. It was found that a salesperson’s use of social media will have a positive effect on information communication, which will, in turn, lead to improved customer satisfaction with the salesperson. Also, it was investigated that information communication will be positively related to responsiveness, which impacts customer satisfaction.
Another study by Rossmann and Stei ( 2015 ) looked at the antecedents of social media use, social media use by B2B companies and their effect on customers. By using data from 362 chief information officers of B2B companies the study found the following. Social media usage of sales representative has a positive impact on customer satisfaction. Age has a negative effect on content generation. It seems that older salespeople use social media in passive ways or interacting with the customer rather than creating their own content. It was found that the quality of corporate social media strategy has a positive impact on social media usage in terms of the consumption of information, content generation, and active interaction with customers. Also, the expertise of a salesperson in the area of social media has a positive impact on social media usage.
3.1.2 Value Creation
Research in B2B found that social media can create value for customers and salesperson (Agnihotri et al. 2012 ; Agnihotri et al. 2017 ). Agnihotri et al. ( 2012 ) proposed a theoretical framework to explain the mechanisms through which salespeople’s use of social media operates to create value and propose a strategic approach to social media use to achieve competitive goals. The study draws on the existing literature on relationship marketing, task–technology fit theory, and sales service behavior to sketch a social media strategy for business-to-business sales organizations with relational selling objectives. The proposed framework describes how social media tools can help salespeople perform service behaviors (information sharing, customer service, and trust-building) leading to value creation.
Some researchers investigated the role of the salesperson in the value creation process after closing the sale. By employing salesperson-customer data within a business-to-business context, Agnihotri et al. ( 2017 ) analysed the direct effects of sales-based CRM technology on the post-sale service behaviors: diligence, information communication, inducements, empathy, and sportsmanship. Additionally, the study examines the interactive effects of sales-based CRM technology and social media on these behaviors. The results indicate that sales-based CRM technology has a positive influence on salesperson service behaviors and that salespeople using CRM technology in conjunction with social media are more likely to exhibit higher levels of SSBs than their counterparts with low social media technology use. Data were collected from 162 salespeople from India. SmartPLS was used to analyse the data.
3.1.3 Intention to Buy and Sales
Another group of studies investigated the effect of social media on the level of sales and consumer purchase intention (Ancillai et al. 2019 ; Itani et al. 2017 ; Salo 2017 ; Hsiao et al. 2020 ; Mahrous 2013 ). For example, Itani et al. ( 2017 ) used the theory of reasoned actions to develop a model that tests the factors affecting the use of social media by salesperson and its impact. By collecting data from 120 salespersons from different industries and using SmartPLS to analyse the data, it was found that attitude towards social media usefulness did not affect the use of social media. It was found that social media use positively affects competitive intelligence collection, adaptive selling behaviour, which in turn influenced sales performance. Another study by Ancillai et al. ( 2019 ) used in-depth interviews with social selling professionals. The findings suggest that the use of social media improves not only the level of sales but also affects relationship and customer performance (trust, customer satisfaction, customer referrals); and organisational performance (organisational selling performance and brand performance).
It was investigated that social media has a positive effect on the intention to purchase (Hsiao et al. 2020 ; Mahrous 2013 ). For instance, Mahrous ( 2013 ) by reviewing the literature on B2B and B2C companies concluded that social media has a significant influence on consumer buying behaviour.
3.1.4 Customer Relationships
Another group of studies focused on the effect of social media on customer relationships (Bhattacharjya and Ellison 2015 ; Gáti et al. 2018 ; Gruner and Power 2018 ; Hollebeek 2019 ; Iankova et al. 2018 ; Jussila et al. 2011 ; Kho 2008 ; Niedermeier et al. 2016 ; Ogilvie et al. 2018 ). For example, Bhattacharjya and Ellison ( 2015 ) investigated the way companies build relationships with customers by using responsive customer relationship management. The study analysed customer relationship management activities from Twitter account of a Canadian company Shopify (B2B service provider). The company uses Twitter to engage with small business customers, develops and consumers. Jussila et al. ( 2011 ), by reviewing the literature, found that social media leads to increased customer focus and understanding, increased level of customer service and decreased time-to-market.
Gáti et al. ( 2018 ) focused their research efforts on social media use in customer relationship performance, particularly in customer relations. The study investigated the adoption and impact of social media by salespeople of B2B companies. By using data of 112 salespeople from several industries the study found that the intensity of technology use positively affects attitude towards social media, which positively affects social media use. Intensive technology use in turn positively affects customer relationship performance (customer retention). PLS-SEM was applied for analysis.
Another study by Gruner and Power ( 2018 ) investigated the effectiveness of the use of multiple social media platforms in communications with customers. By using data from 208 large Australian organisations, the paper explores how companies’ investment in one form of social media impacts activity on another form of social media. A regression analysis was performed to analyse the data. It was found that widespread activities on LinkedIn, Twitter and YouTube have a negative effect on a company’s marketing activity on Facebook. Thus, having it is more effective for the company to focus on a specific social media platform in forming successful inter-organisational relationships with customers.
Hollebeek ( 2019 ) proposed an integrative S-D logic/resource-based view (RBV) model of customer engagement. The proposed model considers business customer actors and resources in driving business customer resource integration, business customer resource integration effectiveness and business customer resource integration efficiency, which are antecedents of business customer engagement. Business customer engagement, in turn, results in business customer co-creation and relationship productivity.
Niedermeier et al. ( 2016 ) investigated the use of social media among salespeople in the pharmaceutical industry in China. Also, the study investigated the impact of social media on building culturally specific Guanxi relationships-it involves the exchange of factors to build trust and connection for business purpose. By using in-depth interviews with 3 sales managers and a survey of 42 pharmaceutical sales representatives that study found that WeChat is the most common social media platform used by businesses. Also, it was found to be an important tool in building Guanxi. Future studies should focus on other industries and other types of cultural features in doing business.
Ogilvie et al. ( 2018 ) investigated the effect of social media technologies on customer relationship performance and objective sales performance by using two empirical studies conducted in the United States. The first study used 375 salespeople from 1200 B2B companies. The second study used 181 respondents from the energy solution company. It was found that social media significantly affects salesperson product information communication, diligence, product knowledge and adaptability, which in turn affect customer relationship performance. It was also found that the use of social media technologies without training on technology will not lead to good results. Thus, the results propose that companies should allocate the resources required for the proper implementation of social media strategies. Future research should examine how the personality traits of a salesperson can moderate the implementation of social media technologies.
While most of the studies focused on a single country, Iankova et al. ( 2018 ) investigated the perceived effectiveness of social media by different types of businesses in two countries. By using 449 respondents from the US and the UK businesses, it was found that social media is potentially less important, at the present time, for managing ongoing relationships in B2B organizations than for B2C, Mixed or B2B2C organizations. All types of businesses ascribe similar importance to social media for acquisition-related activities. Also it was found that B2B organizations see social media as a less effective communication channel, and to have less potential as a channel for the business.
3.1.5 Brand Awareness
Some researchers argued that social media can influence brand awareness (Ancillai et al. 2019 ; Hsiao et al. 2020 ). For instance, Hsiao et al. ( 2020 ) investigated the effect of social media in the fashion industry. By collecting 1395 posts from lookbook.nu and employing regression analysis it was found that the inclusion of national brand and private fashion brands in the post increased the level of popularity which leads to purchasing interest and brand awareness.
3.1.6 Knowledge Creation
Multiple types of collaborative web tools can help and significantly increase the collaboration and the use of the distributed knowledge inside and outside of the company (McAfee 2006 ). Kärkkäinen et al. ( 2011 ) by analysing previous literature on social media proposed that social media use has a positive effect on sharing and creation of customer information and knowledge in the case of B2B companies.
3.1.7 Corporate Credibility
Another study by Kho ( 2008 ) states the advantages of using social media by B2B companies, which include faster and more personalised communications between customer and vendor, which can improve corporate credibility and strengthen the relationships. Thanks to social media companies can provide more detailed information about their products and services. Kho ( 2008 ) also mentions that customer forums and blog comments in the B2B environment should be carefully monitored in order to make sure that inappropriate discussions are taken offline and negative eWOM communications should be addressed in a timely manner.
3.1.8 Acquiring New Customers
Meire et al. ( 2017 ) investigated the impact of social media on acquiring B2B customers. By using commercially purchased prospecting data, website data and Facebook data from beverage companies the study conducted an experiment and found that social media us an effective tool in acquiring B2B customers. Future work might assess the added value of social media pages for profitability prediction instead of prospect conversion. When a longer timeframe becomes available (e.g., after one year), the profitability of the converted prospects can be assessed.
3.1.9 Salesperson Performance
Moncrief et al. ( 2015 ) investigated the impact of social media technologies on the role of salesperson position. It was found that social media affects sales management functions (supervision, selection, training, compensation, and deployment) and salesperson performance (role, skill, and motivation). Another study by Rodriguez et al. ( 2012 ) examines the effect of social media on B2B sales performance by using social capital theory and collecting data from 1699 B2B salespeople from over 25 different industries. By employing SEM AMOS, the study found that social media usage has a positive significant relationship with selling companies’ ability to create opportunities and manage relationships. The study also found that social media usage has a positive and significant relationship with sales performance (based on relational measurers of sales that focus on behaviours that strengthen the relationship between buyers and sellers), but not with outcome-based sales performance (reflected by quota achievement, growth in average billing size, and overall revenue gain).
3.1.10 Employee Brand Management
The study by Pitt et al. ( 2018 ) focuses on employee engagement with B2B companies on social media. By using results from Glassdoor (2315 five-star and 1983 one-star reviews for the highest-ranked firms, and 1013 five star and 1025 one-star reviews for lowest ranked firms) on employee brand engagement on social media, two key drivers of employee brand engagement by using the content analysis tool DICTION were identified-optimism and commonality. Individuals working in top-ranked companies expressed a higher level of optimism and commonality in comparison with individuals working in low-ranked companies. As a result, a 2 × 2 matrix was constructed which can help managers to choose strategies in order to increase and improve employee brand engagement. Another study by Pitt et al. ( 2017 ) focused on employee engagement of B2B companies on social media. By using a conceptual framework based on a theory of word choice and verbal tone and 6300 reviews collected from Glassdoor and analysed using DICTION. The study found that employees of highly ranked B2B companies are more positive about their employer brand and talk more optimistically about these brands. For low ranked B2B companies it was found that employees express a greater level of activity, certainty, and realism. Also, it was found that they used more aggressive language.
3.1.11 Sustainability
Sustainability refers to the strategy that helps a business “to meet its current requirements without compromising its ability to meet future needs” (World Commission Report on Environment and Development 1987 , p 41). Two studies out of 70 focused on the role of social media for B2B sustainability (Sivarajah et al. 2019 ; Kasper et al. 2015 ). For example, Sivarajah et al. ( 2019 ) argued that big data and social media within a participatory web environment to enable B2B organisations to become profitable and remain sustainable through strategic operations and marketing related business activities.
Another study by Kasper et al. ( 2015 ) proposed the Social Media Matrix which helps companies to decide which social media activities to execute based on their corporate and communication goals. The matrix includes three parts. The first part is focusing on social media goals and task areas, which were identified and matched. The second part consists of five types of social media activities (content, interaction/dialog, listening and analysing, application and networking). The third part provides a structure to assess the suitability of each activity type on each social media platform for each goal. The matrix was successfully tested by assessing the German B2B sector by using expert interviews with practitioners.
Based on the reviewed studies, it can be seen that if used appropriately social media have positive effect on B2B companies before and after sales, such as customer satisfaction, value creation, intention to buy and sales, customer relationships, brand awareness, knowledge creation, corporate credibility, acquiring new customers, salesperson performance, employee brand management, and sustainability. However, limited research is done on the negative effect of social media on b2b companies.
3.2 Adoption of Social Media
Some scholars investigated factors affecting the adoption of social media by B2B companies (Buratti et al. 2018 ; Gáti et al. 2018 ; Gazal et al. 2016 ; Itani et al. 2017 ; Kumar and Möller 2018 ; Lacka and Chong 2016 ). For instance, Lacka and Chong ( 2016 ) investigated factors affecting the adoption of social media by B2B companies from different industries in China. The study collected the data from 181 respondents and used the technology acceptance model with Nielsen’s model of attributes of system acceptability as a theoretical framework. By using SEM AMOS for analysis the study found that perceived usability, perceived usefulness, and perceived utility positively affect adoption and use of social media by B2B marketing professionals. The usefulness is subject to the assessment of whether social media sites are suitable means through which marketing activities can be conducted. The ability to use social media sites for B2B marketing purposes, in turn, is due to those sites learnability and memorability attributes.
Another study by Müller et al. ( 2018 ) investigated factors affecting the usage of social media. By using survey data from 100 Polish and 39 German sensor suppliers, it was found that buying frequency, the function of a buyer, the industry sector and the country does not affect the usage of social media in the context of sensor technology from Poland and Germany. The study used correlation analysis and ANOVA.
Lashgari et al. ( 2018 ) studied the adoption and use of social media by using face-to-face interviews with key managers of four multinational corporations and observations from companies’ websites and social media platforms. It was found that that the elements essential in forming the B2B firm’s social media adoption strategies are content (depth and diversity), corresponding social media platform, the structure of social media channels, the role of moderators, information accessibility approaches (public vs. gated-content), and online communities. These elements are customized to the goals and target group the firm sets to pursue. Similarly, integration of social media into other promotional channels can fall under an ad-hoc or continuous approach depending on the scope and the breadth of the communication plan, derived from the goal.
Similar to Lashgari et al. ( 2018 ), Shaltoni ( 2017 ) used data from managers. The study applied technology organisational environmental framework and diffusion of innovations to investigate factors affecting the adoption of social media by B2B companies. By using data from marketing managers or business owners of 480 SMEs, the study found that perceived relative advance, perceive compatibility, organizational innovativeness, competitor pressure, and customer pressure influence the adoption of social media by B2B companies. The findings also suggest that many decision-makers in B2B companies think that Internet marketing is not beneficial, as it is not compatible with the nature of B2B markets.
Buratti et al. ( 2018 ) investigated the adoption of social media by tanker shipping companies and ocean carriers. By using data from 60 companies the following was found. LinkedIn is the most used tool, with a 93.3% adoption rate. Firm size emerges as a predictor of Twitter’s adoption: big companies unveil a higher attitude to use it. Finally, the country of origin is not a strong influential factor in the adoption rate. Nonetheless, Asian firms clearly show a lower attitude to join SM tools such as Facebook (70%) and LinkedIn (86.7%), probably also due to governmental web restrictions imposed in China. External dimensions such as the core business, the firm size, the geographic area of origin, etc., seem to affect network wideness. Firm size, also, discriminates the capacity of firms to build relational networks. Bigger firms create networks larger than small firms do. Looking at geographical dimensions, Asian firms confirm to be far less active on SM respect to European and North American firms. Finally, the study analyzed the format of the contents disclosed by sample firms, observing quite limited use of photos and videos: in the sample industries, informational contents seem more appropriate for activating a dialogue with stakeholders and communication still appears formulated in a very traditional manner. Preliminary findings suggest that companies operating in conservative B2B services pursue different strategic approaches toward SMM and develop ad hoc communication tactics. Nonetheless, to be successful in managing SM tools, a high degree of commitment and a clear vision concerning the role of SM within communication and marketing strategy is necessary.
Gazal et al. ( 2016 ) investigated the adoption and measuring of the effectiveness of social media in the context of the US forest industry by using organisational-level adoption framework and TAM. By using data from 166 companies and performing regression analysis, the following results were received. Years in business, new sales revenue, product type, amount of available information on a company website, perceived importance of e-commerce and perceived ease of use of social media significantly affected social media use. Also, it was found that companies’ strategies and internal resources and capabilities and influence a company’s decision to adopt social media. Also, it was found that 94 of respondents do not measure the ROI from social media use. The reason is that the use of social media in marketing is relatively new and companies do not possess the knowledge of measuring ROI from the use of social media. Companies mostly use quantitative metrics (number of site visits, number of social network friends, number of comments and profile views) and qualitative metrics (growth of relationships with the key audience, audience participation, moving from monologue to dialogue with consumers. Facebook was found to be the most effective social media platform reported by the US forest industry.
The study by Kumar and Möller ( 2018 ) investigated the role of social media for B2B companies in their recruitment practices. By using data from international B2B company with headquarter in Helsinki, Finland comprised of 139 respondents it was found that brand familiarity encourages them to adopt social media platforms for a job search; however, the effect of the persuasiveness of recruitment messages on users’ adoption of social media platforms for their job search behavior is negative. The study used correlation analysis and descriptive analysis to analyse the data.
Nunan et al. ( 2018 ) identified areas for future research such as patterns of social media adoption, the role of social media platforms within the sales process, B2B consumer engagement and social media, modeling the ROI of social media, and the risks of social media within B2B sales relationships.
The study by Pascucci et al. ( 2018 ) conducted a systematic literature review on antecedents affecting the adoption and use of social media by B2B companies. By reviewing 29 studies published in academic journal and conferences from 2001 to 2017, the study identified external (pressure from customers, competitors, availability of external information about social media) and internal factors (personal characteristics -managers age, individual commitment, perceptions of social media-perceived ease of use, perceived usefulness, perceived utility), which can affect adoption of social media.
The study by Siamagka et al. ( 2015 ) aims to investigate factors affecting the adoption of social media by B2B organisations. The conceptual model was based on the technology acceptance model and the resource-based theory. AMOS software and Structural equation modelling were employed to test the proposed hypotheses. By using a sample of 105 UK companies, the study found that perceived usefulness of social media is influenced by image, perceived ease of use and perceived barriers. Also, it was found that social media adoption is significantly determined by organisational innovativeness and perceived usefulness. Additionally, the study tested the moderating role of organisational innovativeness and found that it does not affect the adoption of social media by B2B organisations. The study also identified that perceived barriers to SNS (uncertainty about how to use SNS to achieve objectives, employee’s lack of knowledge about SNS, high cost of investment needed to adopt the technology) have a negative impact on perceived usefulness of social media by B2B organisations. The study also used nine in-depth interviews with B2B senior managers and social media specialists about adoption of social media by B2B. It was found that perceived pressure from stakeholders influences B2B organisations’ adoption intention of social media. Future research should test it by using quantitative methods.
While most of the studies focused on the antecedents of social media adoption by B2B companies, Michaelidou et al. ( 2011 ) investigated the usage, perceived barriers and measuring the effectiveness of social media. By using data from 92 SMEs the study found that over a quarter of B2B SMEs in the UK are currently using SNS to achieve brand objectives, the most popular of which is to attract new customers. The barriers that prevent SMEs from using social media to support their brands were lack of staff familiarity and technical skills. Innovativeness of a company determined the adoption of social media. It was found that most of the companies do not evaluate the effectiveness of their SNS in supporting their brand. The most popular measures were the number of users joining the groups/discussion and the number of comments made. The findings showed that the size of the company does not influence the usage of social media for small and medium-sized companies. Future research should investigate the usage of social media in large companies and determine if the size can have and influence on the use. The benefits of using social media include increasing awareness and communicating the brand online. B2B companies can employ social media to create customer value in the form of interacting with customers, as well as building and fostering customer relationships. Future research should investigate the reasons why most of the users do not assess the effectiveness of their SNS. Future research should also investigate how the attitude towards technology can influence the adoption of social media.
Based on the reviewed studies it can be seen that the main factors affecting the adoption of social media by B2B companies are perceived usability, technical skills of employees, pressure from stakeholders, perceived usefulness and innovativeness.
3.3 Social Media Strategies
Another group of studies investigated types of strategies B2B companies apply (Cawsey and Rowley 2016 ; Huotari et al. 2015 ; Kasper et al. 2015 ; McShane et al. 2019 ; Mudambi et al. 2019 ; Swani et al. 2013 ; Swani et al. 2014 ; Swani et al. 2017 ; Watt 2010 ). For example, Cawsey and Rowley ( 2016 ) focused on the social media strategies of B2B companies. By conducting semi-structured interviews with marketing professionals from France, Ireland, the UK and the USA it was found that enhancing brand image, extending brand awareness and facilitating customer engagement were considered the most common social media objective. The study proposed the B2B social media strategy framework, which includes six components of a social media strategy: 1) monitoring and listening 2) empowering and engaging employees 3) creating compelling content 4) stimulating eWOM 5) evaluating and selecting channels 6) enhancing brand presence through integrating social media.
Chirumalla et al. ( 2018 ) focused on the social media engagement strategies of manufacturing companies. By using semi-structured interviews (36), observations (4), focus group meetings (6), and documentation, the study developed the process of social media adoption through a three-phase engagement strategy which includes coordination, cooperation, and co-production.
McShane et al. ( 2019 ) proposed social media strategies to influence online users’ engagement with B2B companies. Taking into consideration fluency lens the study analysed Twitter feeds of top 50 social B2B brands to examine the influence of hashtags, text difficulty embedded media and message timing on user engagement, which was evaluated in terms of likes and retweets. It was found that hashtags and text difficulty are connected to lower levels of engagement while embedded media such as images and videos improve the level of engagement.
Swani et al. ( 2014 ) investigate the use of Twitter by B2B and B2C companies and predict factors that influence message strategies. The study conducted a longitudinal content analysis by collecting 7000 tweets from Fortune 500 companies. It was found that B2B and B2C companies used different message appeals, cues, links and hashtags. B2B companies tend to use more emotional than functional appeals. It was found that B2B and B2C companies do not use hard-sell message strategies.
Another study by Swani et al. ( 2013 ) aimed to investigate message strategies that can help in promoting eWOM activity for B2B companies. By applying content analysis and hierarchical linear modeling the study analysed 1143 wall post messages from 193 fortune 500 Facebook accounts. The study found that B2B account posts will be more effective if they include corporate brand names and avoid hard sell or explicitly commercial statement. Also, companies should use emotional sentiment in Facebook posts.
Huotari et al. ( 2015 ) aimed to investigate how B2B marketers can influence content creation in social media. By conducting four face-to-face interviews with B2B marketers, it was found that a B2B company can influence content creation in social media directly by adding new content, participating in a discussion and removing content through corporate user accounts and controlling employees social media behaviour. Also, it can influence it indirectly by training employees to create desired content and perfuming marketing activities that influence other users to create content that is favorable for the company.
Most of the studies investigated the strategies and content of social media communications of B2B companies. However, the limited number of studies investigated the importance of CEO engagement on social media in the company’s strategies. Mudambi et al. ( 2019 ) emphasise the importance of the CEO of B2B companies to be present and active on social media. The study discusses the advantages of social media presence for the CEO and how it will benefit the company. For example, one of the benefits for the CEO can be perceived as being more trustworthy and effective than non-social CEOs, which will benefit the company in increased customer trust. Mudambi et al. ( 2019 ) also discussed the platforms the CEO should use and posting frequencies depending on the content of the post.
From the above review of the studies, it can be seen that B2B companies social media strategies include enhancing brand image, extending brand awareness and facilitating customer engagement. Companies use various message strategies, such as using emotional appeal, use of brand names, and use of hashtags. Majority of the companies avoid hard sell or explicitly commercial statement.
3.4 Social Media Use
Studies investigated the way how companies used social media and factors affecting the use of social media by B2B (Andersson et al. 2013 ; Bernard 2016 ; Bolat et al. 2016 ; Denktaş-Şakar and Sürücü 2018 ; Dyck 2010 ; Guesalaga 2016 ; Habibi et al. 2015 ). For example, Vasudevan and Kumar ( 2018 ) investigated how B2B companies use social media by analysing 325 brand posts of Canon India, Epson India, and HP India on Linkedin, Facebook, and Twitter. By employing content analysis the study found that most of the posts had a combination of text and message. More than 50% of the posts were about product or brand-centric. The study argued that likes proved to be an unreliable measure of engagement, while shares were considered a more reliable metric. The reason was that likes had high spikes when brand posts were boosted during promotional activities.
Andersson and Wikström ( 2017 ) used case studies of three B2B companies to investigate reasons for using social media. It was found that companies use social media to enhance customer relationships, support sales and build their brands. Also, social media is used as a recruiting tool, a seeking tool, and a product information and service tool.
Bell and Shirzad ( 2013 ) aimed to conduct social media use analysis in the context of pharmaceutical companies. The study analysed 54,365 tweets from the top five pharmaceutical companies. The study analysed the popular time slots, the average number of positive and negative tweets and its content by using Nvivo9.
Bernard ( 2016 ) aims to examine how chief marketing officers use social media. By using case studies from IBM experience with social media it was found that B2B CMO’s are not ready to make use of social media. It was proposed that social media can be used for after-sales service, getting sales leads, engaging with key influencers, building the company’s reputation and enhancing the industry status of key individuals. B2B firms need to exploit the capabilities of processing massive amounts of data to get the most from social media.
Bolat et al. ( 2016 ) explore how companies apply mobile social media. By employing a grounded theory approach to analyse interviews from 26 B2B company representatives from UK advertising and marketing sector companies. It was found that companies use social media for branding, sensing market, managing relationships, and developing content.
Denktaş-Şakar and Sürücü ( 2018 ) investigated how social media usage influence stakeholder engagement focusing on the corporate Facebook page of 30 3PLs companies. In total 1532 Facebook posts were analysed. It was found that the number of followers, post sharing frequency, negatively affect stakeholder engagement. It was found that content including photos facilitates more stakeholder engagement (likes, comment, share) in comparison with other forms. Vivid posts and special day celebration posts strengthen relationships with stakeholders.
Dyck ( 2010 ) discussed the advantages of using social media for the device industry. Social media can be used for product innovation and development, to build a team and collaborate globally. Also, there is an opportunity to connect with all of the stakeholders needed in order to deliver the device to the market. Additionally, it provides to receive feedback from customers (doctors, hospitals) in real-time.
The study by Guesalaga ( 2016 ) draws on interactional psychology theory to propose and test a model of usage of social media in sales, analysing individual, organizational, and customer-related factors. It was found that organizational competence and commitment to social media are key determinants of social media usage in sales, as well as individual commitment. Customer engagement with social media also predicts social media usage in sales, both directly and (mostly) through the individual and organizational factors analysed, especially organizational competence and commitment. Finally, the study found evidence of synergistic effects between individual competence and commitment, which is not found at the organizational level. The data obtained by surveying 220 sales executives in the United States were analysed using regression analysis.
Habibi et al. ( 2015 ) proposed a conceptual model for the implementation of social media by B2B companies. Based on existing B2B marketing, social media and organisational orientational literature the study proposed that four components of electronic market orientation (philosophical, initiation, implementation and adoption) address different implementation issues faced in implementing social media.
Katona and Sarvary ( 2014 ) presented a case of using social media by Maersk-the largest container shipping company in the world. The case provided details on the program launch and the integration strategy which focused on integrating the largest independent social media operation into the company’s broader marketing efforts.
Moore et al. ( 2013 ) provided insights into the understanding of the use of social media by salespersons. 395 salespeople in B2B and B2C markets, utilization of relationship-oriented social media applications are presented and examined. Overall, findings show that B2B practitioners tend to use media targeted at professionals whereas their B2C counterparts tend to utilize more sites targeted to the general public for engaging in one-on-one dialogue with their customers. Moreover, B2B professionals tend to use relationship-oriented social media technologies more than B2C professionals for the purpose of prospecting, handling objections, and after-sale follow-up.
Moore et al. ( 2015 ) investigated the use of social media between B2B and B2C salespeople. By using survey data from 395 sales professionals from different industries they found that B2B sales managers use social selling tools significantly more frequently than B2C managers and B2C sales representatives while conducting sales presentations. Also, it was found that B2B managers used social selling tools significantly more frequently than all sales representatives while closing sales.
Müller et al. ( 2013 ) investigated social media use in the German automotive market. By using online analysis of 10 most popular car manufacturers online social networks and surveys of six manufacturers, 42 car dealers, 199 buyers the study found that social media communication relations are widely established between manufacturers and (prospective) buyers and only partially established between car dealers and prospective buyers. In contrast to that, on the B2B side, social media communication is rarely used. Social Online Networks (SONs) are the most popular social media channels employed by businesses. Manufacturers and car dealers focus their social media engagement, especially on Facebook. From the perspective of prospective buyers, however, forums are the most important source of information.
Sułkowski and Kaczorowska-Spychalska ( 2016 ) investigated the adoption of social media by companies in the Polish textile-clothing industry. By interviewing seven companies representatives of small and medium-sized enterprises the study found that companies started implementing social media activities in their marketing activities.
Vukanovic ( 2013 ) by reviewing previous literature on social media outlined advantages of using social media for B2B companies, which include: increase customer loyalty and trust, building and improving corporate reputation, facilitating open communications, improvement in customer engagement to name a few.
Keinänen and Kuivalainen ( 2015 ) investigated factors affecting the use of social media by B2B customers by conducting an online survey among 82 key customer accounts of an information technology service company. Partial least squares path modelling was used to analysed the proposed hypotheses. It was found that social media private use, colleague support for using SM, age, job position affected the use of social media by B2B customers. The study also found that corporate culture, gender, easiness to use, and perception of usability did not affect the use of social media by B2B customers.
By using interviews and survey social media research found that mostly B2B companies use social media to enhance customer relationships, support sales, build their brands, sense market, manage relationships, and develop content. Additionally, some companies use it social media as a recruitment tool. The main difference between B2B and B2C was that B2B sales managers use social selling tools significantly more frequently than B2C managers.
3.5 Measuring the Effectiveness of Social Media
It is important for a business to be able to measure the effectiveness of social media by calculating return on investment (ROI). ROI is the relationship between profit and the investment that generate that profit. Some studies focused on the ways B2B companies can measure ROI and the challenges they face (Gazal et al. 2016 ; Michaelidou et al. 2011 ; Vasudevan and Kumar 2018 ). For example, Gazal et al. ( 2016 ) investigated the adoption and measuring of the effectiveness of social media in the context of the US forest industry by using organisational-level adoption framework and TAM. By using data from 166 companies it was found that 94% of respondents do not measure the ROI from social media use. The reason is that the use of social media in marketing is relatively new and companies do not possess the knowledge of measuring ROI from the use of social media. Companies mostly use quantitative metrics (number of site visits, number of social network friends, number of comments and profile views) and qualitative metrics (growth of relationships with the key audience, audience participation, moving from monologue to dialogue with consumers).
Another study by Michaelidou et al. ( 2011 ) found that most of the companies do not evaluate the effectiveness of their SNS in supporting their brand. The most popular measures were the number of users joining the groups/discussion and the number of comments made.
Vasudevan and Kumar ( 2018 ) investigated how B2B companies use social media and measure ROI from social media by analysing 325 brand posts of Canon India, Epson India, and HP India on Linkedin, Facebook, and Twitter. By employing content analysis the study found that most of the post has a combination of text and message. More than 50% of the posts were about product or brand-centric. The study argued that likes proved to be an unreliable measure of engagement, while shares were considered a more reliable metric. The reason was that likes had high spikes when brand posts were boosted during promotional activities. Future research should conduct longitudinal studies.
By reviewing the above studies, it can be concluded that companies still struggle to find ways of measuring ROI and applying correct metrics. By gaining knowledge in how to measure ROI from social media activities, B2B companies will be able to produce valuable insights leading to better marketing strategies (Lal et al. 2020 ).
3.6 Social Media Tools
Some studies proposed tools that could be employed by companies to advance their use of social media. For example, Mehmet and Clarke ( 2016 ) proposed a social semiotic multimodal (SSMM) framework that improved the analysis of social media communications. This framework employs multimodal extensions to systemic functional linguistics enabling it to be applying to analysing non-language as well as language constituents of social media messages. Furthermore, the framework also utilises expansion theory to identify, categorise and analyse various marketing communication resources associated with marketing messages and also to reveal how conversations are chained together to form extended online marketing conversations. This semantic approach is exemplified using a Fairtrade Australia B2B case study demonstrating how marketing conversations can be mapped and analysed. The framework emphasises the importance of acknowledging the impact of all stakeholders, particularly messages that may distract or confuse the original purpose of the conversation.
Yang et al. ( 2012 ) proposed the temporal analysis technique to identify user relationships on social media platforms. The experiment was conducted by using data from Digg.com . The results showed that the proposed techniques achieved substantially higher recall but not very good at precision. This technique will help companies to identify their future consumers based on their user relationships.
Based on the literature review, it can be seen that B2B companies can benefit by using the discussed tools. However, it is important to consider that employee should have some technical skills and knowledge to use these tools successfully. As a result, companies will need to invest some resources in staff training.
4 Weight Analysis
Weight analysis enables scrutiny of the predictive power of independent variables in studied relationships and the degree of effectiveness of the relationships (Jeyaraj et al. 2006 ; Rana et al. 2015 ; Ismagilova et al. 2020a ). The results of weight analysis are depicted in Table 3 providing information about an independent variable, dependent variable, number of significant relationships, number of non-significant relationships, the total number of relationships and weight. To perform weight analysis, the number of significant relationships was divided by the total number of analysed relationships between the independent variable and the dependent variable (Jeyaraj et al. 2006 ; Rana et al. 2015 ). For example, the weight for the relationship between attitude towards social media and social media is calculated by dividing ‘1’ (the number of significant relationships) by ‘2’ (the total number of relationships) which equals 0.5.
A predictor is defined as well-utilised if it was examined five or more times, otherwise, it is defined as experimental. It can be seen from Table 3 that all relationships were examined less than five times. Thus all studied predictors are experimental. The predictor is defined as promising when it has been examined less than five times by existing studies but has a weight equal to ‘1’ (Jeyaraj et al. 2006 ). From the predictors affecting the adoption of social media, it can be seen that two are promising, technical skills of employees and pressure from stakeholders. Social media usage is a promising predictor for acquiring new customers, sales, stakeholder engagement and customer satisfaction. Perceived ease of use and age of salesperson are promising predictors of social media usage. Even though this relationship was found to be significant every time it was examined, it is suggested that this variable, which can also be referred to as experimental, will need to be further tested in order to qualify as the best predictor. Another predictor, average rating of product/service, was examined less than five times with a weight equal to 0.75, thus it is considered as an experimental predictor.
Figure 1 shows the diagrammatic representation of the factors affecting different relationships in B2B social media with their corresponding weights, based on the results of weight analysis. The findings suggest that promising predictors should be included in further empirical studies to determine their overall performance.
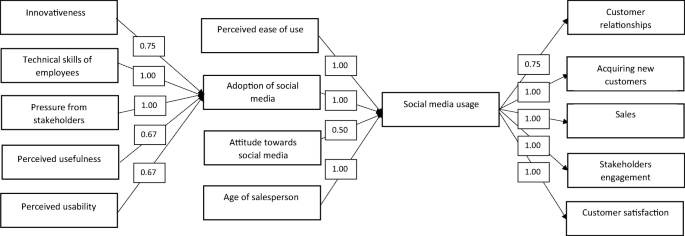
Diagrammatic representation of results of weight analysis. Note: experimental predictors
It can be seen from Fig. 1 that social media usage is affected by internal (e.g. attitude towards social media, technical skills of employees) and external factors (e.g. pressure from stakeholders) of the company. Also, the figure depicts the effect of social media on the business (e.g. sales) and society (e.g. customer satisfaction).
5 Discussion
In reviewing the publications gathered for this paper, the following themes were identified. Some studies investigated the effect of social media use by B2B companies. By using mostly survey to collect the data from salespeople and managers, the studies found that social media has a positive effect on number of outcomes important for the business such as customer satisfaction, value creation, intention to buy and sales, customer relationships, brand awareness, knowledge creation, corporate credibility, acquiring new customers, salespersons performance, employee brand management, and sustainability. Most of the outcomes are similar to the research on social media in the context of B2C companies. However, some of the outcomes are unique for B2B context (e.g. employee brand management, company credibility). Just recently, studies started investigating the impact of the use of social media on sustainability.
Another group of studies looked at the adoption of social media by B2B companies (Buratti et al. 2018 ; Gáti et al. 2018 ; Gazal et al. 2016 ; Itani et al. 2017 ; Kumar and Möller 2018 ). The studies investigated it mostly from the perspectives of salespersons and identify some of the key factors which affect the adoption, such as innovativeness, technical skills of employees, pressure from stakeholders, perceived usefulness, and perceived usability. As these factors are derived mostly from surveys conducted with salespersons findings can be different for other individuals working in the organisation. This it is important to conduct studies that will examine factors affecting the adoption of social media across the entire organisation, in different departments. Using social media as part of the digital transformation is much bigger than sales and marketing, it encompasses the entire company. Additionally, most of the studies were cross-sectional, which limits the understanding of the adoption of social media by B2B over time depending on the outcomes and environment (e.g. competitors using social media).
Some studies looked at social media strategies of B2B companies (Cawsey and Rowley 2016 ; Huotari et al. 2015 ; Kasper et al. 2015 ; McShane et al. 2019 ; Mudambi et al. 2019 ). By employing interviews with companies’ managers and analysing its social media platforms (e.g. Twitter) it was found that most of the companies follow the following strategies: 1) monitoring and listening 2) empowering and engaging employees 3) creating compelling content 4) stimulating eWOM 5) evaluating and selecting channels 6) enhancing brand presence through integrating social media (Cawsey and Rowley 2016 ). Some studies investigated the difference between social media strategies of B2B and B2C companies. For example, a study by Swani et al. ( 2017 ) focused on effective social media strategies. By applying psychological motivation theory the study examined the key differences in B2B and B2C social media message strategies in terms of branding, message appeals, selling, and information search. The study used Facebook posts on brand pages of 280 Fortune companies. In total, 1467 posts were analysed. By using Bayesian models, the results showed that the inclusion of corporate brand names, functional and emotional appeals and information search cues increases the popularity of B2B messages in comparison with B2C messages. Also, it was found that readers of B2B content show a higher message liking rate and lower message commenting rate in comparison with readers of B2C messages.
The next group of studies looked at social media use by B2B companies (Andersson et al. 2013 ; Bernard 2016 ; Bolat et al. 2016 ; Denktaş-Şakar and Sürücü 2018 ; Dyck 2010 ; Guesalaga 2016 ; Habibi et al. 2015 ). B2B companies use social media for enhancing and managing customer relationships (Andersson and Wikström 2017 ; Bolat et al. ( 2016 ); branding (Andersson and Wikström 2017 ; Bolat et al. 2016 ), sensing market (Bolat et al. 2016 ) and co-production (Chirumalla et al. 2018 ). Additionally, it was mentioned that some of the B2B companies use social media as a recruiting tool, and tool which helps to collaborate globally (Andersson and Wikström 2017 ; Dyck 2010 ).
It is important for companies to not only use social media to achieve positive business outcomes but also it is important to measure their achievements. As a result, some of the studies focused on the measuring effectiveness of social media (Gazal et al. 2016 ; Michaelidou et al. 2011 ; Vasudevan and Kumar 2018 ). Surprisingly, it was found that not so many companies measure ROI from social media (Gazal et al. 2016 ; Michaelidou et al. 2011 ). The ones who do it mostly use quantitative metrics (number of site visits, number of social network friends, number of comments and profile views) and qualitative metrics (growth of relationships with key audience, audience participation, moving from monologue to dialogue with consumers) (Gazal et al. 2016 ). Some future studies should investigate how ROI influences the strategy of B2B companies over period of time.
The last group of studies focused on social media tools used by B2B companies (Keinänen and Kuivalainen 2015 ; Mehmet and Clarke 2016 ; Yang et al. 2012 ). By using number of social media tools (Social Semiotic Multimodal) companies are able to improve their analysis of social media communications and identify their future consumers based on their user relationships. Studies investigating barriers and factors adoption of various social media tools by B2B companies are needed.
After reviewing studies on b2B social media, weight analysis was performed. Based on the results of weight analysis the conceptual model for future studies was proposed (Fig. 2 ). It is important to note that a limited number of studies focused and empirically tested factors affecting the adoption, use, and effect of social media. As a result, identified factors were considered as experimental (examined less than five times). It is too early to label these experimental predictors as worst or best, thus their further investigation is encouraged.
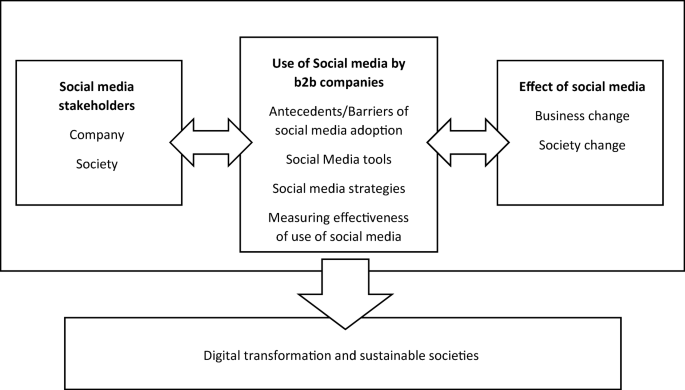
Social media impact on digital transformation and sustainable societies
Additionally, our review of the literature on B2B social media identified dominant research methods used by scholars. Qualitative and quantitative techniques were used by most of these studies. Closer analysis of 70 publications reviewed in this study revealed the multiple techniques applied for gathering data. Quantitative methods used in the studies mostly used surveys (see Table 4 ).
The data was mostly gathered from salespersons, managers and data from social media platforms (e.g. Twitter, Facebook). Just a limited number of studies employed consumer reported data (see Table 5 ).
On the other hand, publications using qualitative methods mainly used interviews and web scraping for the collection of the required data. To analyse the data studies used a variety of techniques including SEM, regression analysis and content analysis being one of the most used (see Table 6 ).
5.1 Digital Transformation and Sustainability Model
Based on the conducted literature review and adapting the model by Pappas et al. ( 2018 ) Fig. 2 presents the digital transformation and sustainability model in the context of B2B companies, which conceptualise the social media ecosystems, and the factors that need to collaborate to enable the use of social media towards the achievement of digital transformation and the creation of sustainable societies. The model comprises of social media stakeholders, the use of social media by B2B companies, and effect of social media on business and society.
5.1.1 Social Media Stakeholders
Building on the discussion and model provided by Pappas et al. ( 2018 ), this paper posits that the social media ecosystem comprises of the data stakeholders (company, society), who engage on social media (posting, reading, using information from social media). The use of social media by different stakeholders will lead to different effects affecting companies, customers and society. This is an iterative process based on which the stakeholders use their experience to constantly improve and evolve their use of social media, which has impacts on both, business and society. The successful implementation of this process is key to digital transformation and the creation of sustainable societies. Most of the current studies (Andersson et al. 2013 ; Bernard 2016 ; Bolat et al. 2016 ; Denktaş-Şakar and Sürücü 2018 ; Dyck 2010 ; Guesalaga 2016 ) focus mostly on the company as a stakeholder. However, more research is needed on other types of stakeholders (e.g. society).
5.1.2 Use of Social Media by B2B Companies
Social media affects not only ways how companies connect with their clients, but it is also changing their business models, the way how the value is delivered and profit is made. To successfully implement and use social media, B2B companies need to consider various social media tools, antecedents/barriers of its adoption, identify suitable social media strategies which are in line with the company’s overall strategy, and measure effectiveness of the use of social media. There are various factors that affect the use of social media by B2B companies. The study found that social media usage is influenced by perceived ease of use, adoption of social media, attitude towards social media and age of salesperson.
The majority of the studies focus on the management of the marketing department. However, digital transformation is much bigger than just marketing as it encompasses the entire organisation. As a result, future studies should look like the entire organisation and investigate barriers and factors affecting the use of social media.
It is crucial for companies to design content which will be noticed on social media by their potential, actual and former customers. Social media content should be interesting and offer some beneficial information, rather than just focus on services the company provides. Companies could use fresh views on relevant industry news, provide information how they are contributing to society and environment, include humour in their posts, share information about the team, make it more personal. It is also useful to use images, infographics, and video content.
It is also important for companies to measure digital marketing actions. More studies are needed on how to isolate the impact of specific media marketing actions to demonstrate their impact on the desired business outcomes (Salo 2017 ). Thus, future studies can consider how particular social media channels (e.g. Facebook, LinkedIn) in a campaign of a new product/ service influence brand awareness and sales level. Also, a limited number of studies discussed the way B2B companies can measure ROI. Future research should investigate how companies can measure intangible ROI, such as eWOM, brand awareness, and customer engagement (Kumar and Mirchandani 2012 ). Also, future research should investigate the reasons why most of the users do not assess the effectiveness of their SNS. Furthermore, most of the studies focused on likes, shares, and comments to evaluate social media engagement. Future research should focus on other types of measures. More research needs considering the impact of legislation on the use of social media by companies. Recent B2B studies did not consider recent legislation (General Data Protection Regulation 2018 ) in the context B2B (Sivarajah et al. 2019 ).
5.1.3 Effect of Social Media on Business and Society
Social media plays an important part in the company’s decision-making process. Social media can bring positive changes into company, which will result in improving customer satisfaction, value creation, increase in sales, building relationships with customers, knowledge creation, improve the perception of corporate credibility, acquisition of new customers, and improve employment brand engagement. Using information collected from social media can help companies to have a set of reliable attributes that comprise social, economic and environmental aspects in their decision-making process (Tseng 2017 ). Additionally, by using social media B2B companies can provide information to other stakeholders on their sustainability activities. By using data from social media companies will be able to provide products and services which are demanded by society. It will improve the quality of life and result in less waste. Additionally, social media can be considered as a tool that helps managers to integrate business practices with sustainability (Sivarajah et al. 2019 ). As a result, social media use by B2B companies can lead to business and societal changes.
A limited number of studies investigated the effect of social media on word of mouth communications in the B2B context. Future research should investigate the differences and similarities between B2C and B2B eWOM communications. Also, studies should investigate how these types of communications can be improved and ways to deal with negative eWOM. It is important for companies to respond to comments on social media. Additionally, future research should investigate its perceived helpfulness by customers.
Majority of studies (Agnihotri et al. 2016 ; Ancillai et al. 2019 ; Rossmann and Stei 2015 ; Agnihotri et al. 2012 ; Agnihotri et al. 2017 ; Itani et al. 2017 ; Salo 2017 ; Bhattacharjya and Ellison 2015 ; Gáti et al. 2018 ; Gruner and Power 2018 ; Hollebeek 2019 ) investigated positive effect of social media such consumer satisfaction, consumer engagement, and brand awareness. However, it will be interesting to consider the dark side of social media use such as an excessive number of requests on social media to salespeople (Agnihotri et al. 2016 ), which can result in the reduction of the responsiveness; spread of misinformation which can damage the reputation of the company.
Studies were performed in China (Lacka and Chong 2016 ; Niedermeier et al. 2016 ), the USA (Guesalaga 2016 ; Iankova et al. 2018 ; Ogilvie et al. 2018 ), India (Agnihotri et al. 2017 ; Vasudevan and Kumar 2018 ), the UK (Bolat et al. 2016 ; Iankova et al. 2018 ; Michaelidou et al. 2011 ). It is strongly advised that future studies conduct research in other countries as findings can be different due to the culture and social media adoption rates. Future studies should pay particular attention to other emerging markets (such as Russia, Brazil, and South Africa) as they suffer from the slow adoption rate of social media marketing. Some companies in these countries still rely more on traditional media for advertising of their products and services, as they are more trusted in comparison with social media channels (Olotewo 2016 ). The majority of studies investigate the effect of social media in B2B or B2C context. Future studies should pay attention to other contexts (e.g. B2B2B, B2B2C). Another limitation of the current research on B2B companies is that most of the studies on social media in the context of B2B focus on the effect of social media use only on business outcomes. It is important for future research to focus on societal outcomes.
Lastly, most of the studies on social media in the context of B2B companies use a cross-sectional approach to collect the data. Future research can use the longitudinal approach in order to advance understanding of social media use and its impact over time.
5.2 Research Propositions
Based on the social media research in the context of B2B companies and the discussion above the following is proposed, which could serve as a foundation for future empirical work.
Social media is a powerful tool to deliver information to customers. However, social media can be used to get consumer and market insights (Kazienko et al. 2013 ). A number of studies highlighted how information obtained from a number of social media platforms could be used for various marketing purposes, such as understanding the needs and preferences of consumers, marketing potential for new products/services, and current market trends (Agnihotri et al. 2016 ; Constantinides et al. 2008 ). It is advised that future research employs a longitudinal approach to study the impact of social media use on understanding customers. Therefore, the following proposition can be formulated:
Proposition 1
Social media usage of B2B companies has a positive influence on understanding its customers.
By using social media companies can examiner valuable information on competitors. It can help to understand competitors’ habits and strategies, which can lead to the competitive advantage and help strategic planning (Dey et al. 2011 ; Eid et al. 2019 ; Teo and Choo 2001 ). It is advised that future research employs a longitudinal approach to study the impact of social media use on understanding its competitors. As a result, using social media to understand customers and competitors can create business value (Mikalef et al. 2020a ) for key stakeholders and lead to positive changes in the business and societies. The above discussion leads to the following proposition:
Proposition 2
Social media usage of B2B companies has a positive influence on understanding its competitors.
Proposition 3
Culture influences the adoption and use of social media by B2B companies.
Usage of social media can result in some positive marketing outcomes such as building new customer relationships, increasing brand awareness, and level of sales to name a few (Agnihotri et al. 2016 ; Ancillai et al. 2019 ; Dwivedi et al. 2020 ; Rossmann and Stei 2015 ). However, when social media is not used appropriately it can lead to negative consequences. If a company does not have enough resources to implement social media tools the burden usually comes on a salesperson. A high number of customer inquiries, the pressure to engage with customers on social media, and monitor communications happening on various social media platforms can result in the increased workload of a salesperson putting extra pressure (Agnihotri et al. 2016 ). As a result, a salesperson might not have enough time to engage with all the customers online promptly or engage in reactive and proactive web care. As a result, customer satisfaction can be affected as well as company reputation. To investigate the negative impact of social media research could apply novel methods for data collection and analysis such as fsQCA (Pappas et al. 2020 ), or implying eye-tracking (Mikalef et al. 2020b ). This leads to the following proposition:
Proposition 4
Inappropriate use of social media by B2B companies has a negative effect on a) customer satisfaction and b) company reputation.
According to Technology-Organisation-Environment (TOE) framework environmental context significantly affects a company’s use of innovations (Abed 2020 ; Oliveira and Martins 2011 ). Environment refers to the factors which affect companies from outside, including competitors and customers. Adopting innovation can help companies to change the rules of the competition and reach a competitive advantage (Porter and Millar 1985 ). In a competitive environment, companies have a tendency to adopt an innovation. AlSharji et al. ( 2018 ) argued that the adoption of innovation can be extended to social media use by companies. A study by AlSharji et al. ( 2018 ) by using data from 1700 SMEs operating in the United Arab Emirates found that competitive pressure significantly affects the use of social media by SMEs. It can be explained by the fact that companies could feel pressure when other companies in the industry start adopting a particular technology and as a result adopt it to remain competitive (Kuan and Chau 2001 ). Based on the above discussion, the following proposition can be formulated:
Proposition 5
Competitive pressure positively affects the adoption of social media by B2B companies.
Companies might feel that they are forced to adopt and use IT innovations because their customers would expect them to do so. Meeting customers’ expectations could result in adoption of new technologies by B2B companies. Some research studies investigated the impact of customer pressure on companies (AlSharji et al. 2018 ; Maduku et al. 2016 ). For example, a study by Maduku et al. ( 2016 ) found that customer pressure has a positive effect on SMEs adoption of mobile marketing in the context of South Africa. Future research could implement longitudinal approach to investigate how environment affects adoption of social media by B2B companies. This leads to the formulation of the following proposition:
Proposition 6
Customer pressure positively affects the adoption of social media by B2B companies.
6 Conclusion
The aim of this research was to provide a comprehensive systematic review of the literature on social media in the context of B2B companies and propose the framework outlining the role of social media in the digital transformation of B2B companies. It was found that B2B companies use social media, but not all companies consider it as part of their marketing strategies. The studies on social media in the B2B context focused on the effect of social media, antecedents, and barriers of adoption of social media, social media strategies, social media use, and measuring the effectiveness of social media. Academics and practitioners can employ the current study as an informative framework for research on the use of social media by B2B companies. The summary of the key observations provided from this literature review is the following: [i] Facebook, Twitter, and LinkedIn are the most famous social media platforms used by B2B companies, [ii] Social media has a positive effect on customer satisfaction, acquisition of new customers, sales, stakeholder engagement, and customer relationships, [iii] In systematically reviewing 70 publications on social media in the context of B2B companies it was observed that most of the studies use online surveys and online content analysis, [iv] Companies still look for ways to evaluate the effectiveness of social media, [v] Innovativeness, pressure from stakeholders, perceived usefulness, and perceived usability have a significant positive effect on companies’ adoption to use social media, [vi] Lack of staff familiarity and technical skills are the main barriers that affect the adoption of social media by B2B, [vii] Social media has an impact not only on business but also on society, [viii] There is a dark side of social media: fake online reviews, an excessive number of requests on social media to salespeople, distribution of misinformation, negative eWOM, [ix] Use of social media by companies has a positive effect on sustainability, and [x] For successful digital transformation social media should change not only the way how companies integrate it into their marketing strategies but the way how companies deliver values to their customers and conduct their business. This research has a number of limitations. First, only publications from the Scopus database were included in literature analysis and synthesis. Second, this research did not use meta-analysis. To provide a broader picture of the research on social media in the B2B context and reconcile conflicting findings of the existing studies future research should conduct a meta-analysis (Ismagilova et al. 2020c ). It will advance knowledge of the social media domain.
Abed, S. S. (2020). Social commerce adoption using TOE framework: An empirical investigation of Saudi Arabian SMEs. International Journal of Information Management, 53 , 102118.
Article Google Scholar
Agnihotri, R., Kothandaraman, P., Kashyap, R., & Singh, R. (2012). Bringing “social” into sales: The impact of salespeople’s social media use on service behaviors and value creation. Journal of Personal Selling and Sales Management, 32 (3), 333–348. https://doi.org/10.2753/PSS0885-3134320304 .
Agnihotri, R., Dingus, R., Hu, M. Y., & Krush, M. T. (2016). Social media: Influencing customer satisfaction in B2B sales. Industrial Marketing Management, 53 , 172–180. https://doi.org/10.1016/j.indmarman.2015.09.003 .
Agnihotri, R., Trainor, K. J., Itani, O. S., & Rodriguez, M. (2017). Examining the role of sales-based CRM technology and social media use on post-sale service behaviors in India. Journal of Business Research, 81 , 144–154. https://doi.org/10.1016/j.jbusres.2017.08.021 .
Alalwan, A. A., Rana, N. P., Dwivedi, Y. K., & Algharabat, R. (2017). Social media in marketing: A review and analysis of the existing literature. Telematics and Informatics, 34 (7), 1177–1190.
AlSharji, A., Ahmad, S. Z., & Bakar, A. R. A. (2018). Understanding social media adoption in SMEs. Journal of Entrepreneurship in Emerging Economies, 10 (2), 302–328.
Ancillai, C., Terho, H., Cardinali, S., & Pascucci, F. (2019). Advancing social media driven sales research: Establishing conceptual foundations for B-to-B social selling. Industrial Marketing Management, 82 , 293–308. https://doi.org/10.1016/j.indmarman.2019.01.002 .
Andersson, S., & Wikström, N. (2017). Why and how are social media used in a B2B context, and which stakeholders are involved? Journal of Business and Industrial Marketing, 32 (8), 1098–1108. https://doi.org/10.1108/JBIM-07-2016-0148 .
Andersson, S., Evers, N., & Griot, C. (2013). Local and international networks in small firm internationalization: Cases from the Rhône-Alpes medical technology regional cluster. Entrepreneurship & Regional Development, 25 (9–10), 867–888.
Andzulis, J. M., Panagopoulos, N. G., & Rapp, A. (2012). A review of social media and implications for the sales process. Journal of Personal Selling & Sales Management, 32 (3), 305–316.
Barreda, A. A., Bilgihan, A., Nusair, K., & Okumus, F. (2015). Generating brand awareness in online social networks. Computers in Human Behavior, 50 , 600–609.
Bell, D., & Shirzad, S, R. (2013). Social media domain analysis (SoMeDoA): A pharmaceutical study. 9th international conference on web information systems and technologies, 561–570. https://doi.org/10.5220/0004499105610570 .
Bernard, M. (2016). The impact of social media on the B2B CMO. Journal of Business and Industrial Marketing, 31 (8), 955–960. https://doi.org/10.1108/JBIM-10-2016-268 .
Bhattacharjya, J., & Ellison, A, B. (2015). Building business resilience with social media in B2B environments: The emergence of responsive customer relationship management processes on twitter. Working Conference on Virtual Enterprises doi: https://doi.org/10.1007/978-3-319-24141-8_15 .
Bolat, E., Kooli, K., & Wright, L. T. (2016). Businesses and mobile social media capability. Journal of Business and Industrial Marketing, 31 (8), 971–981. https://doi.org/10.1108/JBIM-10-2016-270 .
Buratti, N., Parola, F., & Satta, G. (2018). Insights on the adoption of social media marketing in B2B services. TQM Journal, 30 (5), 490–529. https://doi.org/10.1108/TQM-11-2017-0136 .
Cawsey, T., & Rowley, J. (2016). Social media brand building strategies in B2B companies. Marketing Intelligence and Planning, 34 (6), 754–776. https://doi.org/10.1108/MIP-04-2015-0079 .
Chatterjee, S., & Kar, A. K. (2020). Why do small and medium enterprises use social media marketing and what is the impact: Empirical insights from India. International Journal of Information Management, 53 , 102103.
Chen, H., Chiang, R. H., & Storey, V. C. (2012). Business intelligence and analytics: From big data to big impact. MIS Quarterly, 36 , 1165–1188.
Chirumalla, K., Oghazi, P., & Parida, V. (2018). Social media engagement strategy: Investigation of marketing and R&D interfaces in manufacturing industry. Industrial Marketing Management, 74 , 138–149. https://doi.org/10.1016/j.indmarman.2017.10.001 .
Constantinides, E., Romero, C. L., & Boria, M. A. G. (2008). Social media: A new frontier for retailers?. In European retail research (pp. 1–28) . Wiesbaden: Gabler Verlag.
Google Scholar
Denktaş-Şakar, G., & Sürücü, E. (2018). Stakeholder engagement via social media: An analysis of third-party logistics companies. Service Industries Journal, 40 , 866–889. https://doi.org/10.1080/02642069.2018.1561874 .
Dey, L., Haque, S, M., Khurdiya, A., & Shroff, G. (2011). Acquiring competitive intelligence from social media. In proceedings of the 2011 joint workshop on multilingual OCR and analytics for noisy unstructured text data (pp. 1-9) . https://doi.org/10.1145/2034617.2034621 .
Dwivedi, Y. K., Rana, N. P., Jeyaraj, A., Clement, M., & Williams, M. D. (2019a). Re-examining the unified theory of acceptance and use of technology (UTAUT): Towards a revised theoretical model. Information Systems Frontiers, 21 (3), 719–734.
Dwivedi, Y, K., Hughes, L., Ismagilova, E., Aarts, G., Coombs, C., Crick, T., ... & Galanos, V. (2019b). Artificial Intelligence (AI): Multidisciplinary perspectives on emerging challenges, opportunities, and agenda for research, practice and policy. International Journal of Information Management , 101994, doi: https://doi.org/10.1016/j.ijinfomgt.2019.08.002 .
Dwivedi, Y, K., Ismagilova, E., Hughes, D. L., Carlson, J., Filieri, R., Jacobson, J., ... & Kumar, V. (2020). Setting the future of digital and social media marketing research: Perspectives and research propositions. International Journal of Information Management , 102168, doi: https://doi.org/10.1016/j.ijinfomgt.2020.102168 .
Dyck, P. V. (2010). As social media evolves, the device industry must also. Medical Device and Diagnostic Industry, 32 (8).
Eid, R., Abdelmoety, Z., & Agag, G. (2019). Antecedents and consequences of social media marketing use: An empirical study of the UK exporting B2B SMEs. Journal of Business & Industrial Marketing., 35 , 284–305.
Gáti, M., Mitev, A., & Bauer, A. (2018). Investigating the impact of salespersons’ use of technology and social media on their customer relationship performance in B2B settings. Trziste, 30 (2), 165–176. https://doi.org/10.22598/mt/2018.30.2.165 .
Gazal, K., Montague, I., Poudel, R., & Wiedenbeck, J. (2016). Forest products industry in a digital age: Factors affecting social media adoption. Forest Products Journal, 66 (5-6), 343–353. https://doi.org/10.13073/FPJ-D-15-00007 .
General Data Protection Regulation (2018). Guide to the General Data Protection Regulation. Available at https://www.gov.uk/government/publications/guide-to-the-general-data-protection-regulation . Accessed 28 Jan 2021.
Gregorio, J. (2017). 10 reasons to diversify your digital marketing efforts. Digital marketing Philippines. Available at https://digitalmarketingphilippines.com/10-reasons-to-diversify-your-digital-marketing-efforts// Accessed on April , 27 , 2019.
Gruner, R. L., & Power, D. (2018). To integrate or not to integrate? Understanding B2B social media communications. Online Information Review, 42 (1), 73–92. https://doi.org/10.1108/OIR-04-2016-0116 .
Guesalaga, R. (2016). The use of social media in sales: Individual and organizational antecedents, and the role of customer engagement in social media. Industrial Marketing Management, 54 , 71–79. https://doi.org/10.1016/j.indmarman.2015.12.002 .
Gupta, P., Chauhan, S., & Jaiswal, M. P. (2019). Classification of smart city research-a descriptive literature review and future research agenda. Information Systems Frontiers, 21 (3), 661–685.
Habibi, F., Hamilton, C. A., Valos, M. J., & Callaghan, M. (2015). E-marketing orientation and social media implementation in B2B marketing. European Business Review, 27 (6), 638–655. https://doi.org/10.1108/EBR-03-2015-0026 .
Harrigan, P., Miles, M. P., Fang, Y., & Roy, S. K. (2020). The role of social media in the engagement and information processes of social CRM. International Journal of Information Management, 54 , 102151.
Hollebeek, L. D. (2019). Developing business customer engagement through social media engagement-platforms: An integrative SD logic/RBV-informed model. Industrial Marketing Management, 81 , 89–98.
Hsiao, S. H., Wang, Y. Y., Wang, T., & Kao, T. W. (2020). How social media shapes the fashion industry: The spillover effects between private labels and national brands. Industrial Marketing Management, 86 , 40–51.
Huotari, L., Ulkuniemi, P., Saraniemi, S., & Mäläskä, M. (2015). Analysis of content creation in social media by B2B companies. Journal of Business and Industrial Marketing, 30 (6), 761–770. https://doi.org/10.1108/JBIM-05-2013-0118 .
Iankova, S., Davies, I., Archer-Brown, C., Marder, B., & Yau, A. (2018). A comparison of social media marketing between B2B, B2C and mixed business models. Industrial Marketing Management, 81 , 169–179. https://doi.org/10.1016/j.indmarman.2018.01.001 .
Iannacci, F., Fearon, C., & Pole, K. (2020). From acceptance to adaptive acceptance of social media policy change: A set-theoretic analysis of b2b SMEs. Information Systems Frontiers , 1-18. https://doi.org/10.1007/s10796-020-09988-1 .
Ismagilova, E., Dwivedi, Y. K., Slade, E., & Williams, M. D. (2017). Electronic word of mouth (eWOM) in the marketing context: A state of the art analysis and future directions . Cham: Springer International Publishing. https://doi.org/10.1007/978-3-319-52459-7 .
Ismagilova, E., Hughes, L., Dwivedi, Y. K., & Raman, K. R. (2019). Smart cities: Advances in research—An information systems perspective. International Journal of Information Management, 47 , 88–100.
Ismagilova, E., Slade, E. L., Rana, N. P., & Dwivedi, Y. K. (2020a). The effect of electronic word of mouth communications on intention to buy: A meta-analysis. Information Systems Frontiers, 22 , 1203–1226. https://doi.org/10.1007/s10796-019-09924-y .
Ismagilova, E., Slade, E., Rana, N. P., & Dwivedi, Y. K. (2020b). The effect of characteristics of source credibility on consumer behaviour: A meta-analysis. Journal of Retailing and Consumer Services, 53 , 101736.
Ismagilova, E., Rana, N, P., Slade, E., & Dwivedi, Y, K. (2020c). A meta-analysis of the factors affecting eWOM providing behaviour. European Journal of Marketing. doi: https://doi.org/10.1108/EJM-07-2018-0472 , ahead-of-print.
Itani, O. S., Agnihotri, R., & Dingus, R. (2017). Social media use in B2b sales and its impact on competitive intelligence collection and adaptive selling: Examining the role of learning orientation as an enabler. Industrial Marketing Management, 66 , 64–79. https://doi.org/10.1016/j.indmarman.2017.06.012 .
Jeyaraj, A., & Dwivedi, Y. K. (2020). Meta-analysis in information systems research: Review and recommendations. International Journal of Information Management, 55 , 102226.
Jeyaraj, A., Rottman, J. W., & Lacity, M. C. (2006). A review of the predictors, linkages, and biases in IT innovation adoption research. Journal of Information Technology, 21 (1), 1–23.
Juntunen, M., Ismagilova, E., & Oikarinen, E. L. (2020). B2B brands on twitter: Engaging users with a varying combination of social media content objectives, strategies, and tactics. Industrial Marketing Management, 89 , 630–641.
Jussila, J. J., Kärkkäinen, H., & Leino, M. (2011). Benefits of social media in business-to-business customer interface in innovation. 15th International Academic MindTrek Conference: Envisioning Future Media Environments . MindTrek, 2011 , 167–174. https://doi.org/10.1145/2181037.2181065 .
Kamboj, S., Sarmah, B., Gupta, S., & Dwivedi, Y. (2018). Examining branding co-creation in brand communities on social media: Applying the paradigm of stimulus-organism-response. International Journal of Information Management, 39 , 169–185.
Kapoor, K. K., Tamilmani, K., Rana, N. P., Patil, P., Dwivedi, Y. K., & Nerur, S. (2018). Advances in social media research: Past, present and future. Information Systems Frontiers, 20 (3), 531–558.
Kärkkäinen, H., Jussila, J., & Janhonen, J. (2011). Managing customer information and knowledge with social media in business-to-business companies. ACM International Conference Proceeding Series, doi: https://doi.org/10.1145/2024288.2024309 .
Kasper, H., Koleva, I., & Kett, H. (2015). Social media matrix matching corporate goals with external social media activities doi: https://doi.org/10.1007/978-3-662-46641-4_17 .
Katona, Z., & Sarvary, M. (2014). Maersk line: B2B social media-“it’s communication, not marketing. California Management Review , 56(3), 142–156. doi: https://doi.org/10.1525/cmr.2014.56.3.142 .
Kazienko, P., Szozda, N., Filipowski, T., & Blysz, W. (2013). New business client acquisition using social networking sites. Electronic Markets, 23 (2), 93–103. https://doi.org/10.1007/s12525-013-0123-9 .
Keinänen, H., & Kuivalainen, O. (2015). Antecedents of social media B2B use in industrial marketing context: Customers’ view. Journal of Business and Industrial Marketing, 30 (6), 711–722. https://doi.org/10.1108/JBIM-04-2013-0095 .
Kho, N. D. (2008). B2B gets social media. EContent, 31 (3), 26–30.
Kovac, M. (2016). Social media works for B2B sales, too. Harvard Business Review. Retrieved from https://hbr.org/2016/01/social-media-worksfor-b2b-sales-too . Accessed on April , 27 , 2020 .
Kuan, K. K., & Chau, P. Y. (2001). A perception-based model for EDI adoption in small businesses using a technology–organization–environment framework. Information & Management, 38 (8), 507–521.
Kumar, V., & Mirchandani, R. (2012). Increasing the ROI of social media marketing. MIT Sloan Management Review, 54 (1), 55–61.
Kumar, A., & Möller, K. (2018). Extending the boundaries of corporate branding: An exploratory study of the influence of brand familiarity in recruitment practices through social media by B2B firms. Corporate Reputation Review, 21 (3), 101–114. https://doi.org/10.1057/s41299-018-0046-7 .
Kunsman, T. (2018). Internal marketing: Why your company should prioritize it. https://everyonesocial.com/blog/internal-marketing/ . Accessed on September, 25, 2019.
Lacka, E., & Chong, A. (2016). Usability perspective on social media sites' adoption in the B2B context. Industrial Marketing Management, 54 , 80–91. https://doi.org/10.1016/j.indmarman.2016.01.001 .
Lal, B., Ismagilova, E., Dwivedi, Y.,. K., & Kwayu, S. (2020). Return on Investment in Social Media Marketing: Literature review and suggestions for future research. In Digital and Social Media Marketing (pp. 3–17) . Cham: Springer.
Lashgari, M., Sutton-Brady, C., Solberg Søilen, K., & Ulfvengren, P. (2018). Adoption strategies of social media in B2B firms: A multiple case study approach. Journal of Business and Industrial Marketing, 33 (5), 730–743. https://doi.org/10.1108/JBIM-10-2016-0242 .
Loebbecke, C., & Picot, A. (2015). Reflections on societal and business model transformation arising from digitization and big data analytics: A research agenda. The Journal of Strategic Information Systems, 24 (3), 149–157.
Maduku, D. K., Mpinganjira, M., & Duh, H. (2016). Understanding mobile marketing adoption intention by south African SMEs: A multi-perspective framework. International Journal of Information Management, 36 (5), 711–723.
Mahrous, A, A. (2013). Social media marketing: Prospects for marketing theory and practice on the social web. E-marketing in developed and developing countries: Emerging practices (pp. 56-68) doi: https://doi.org/10.4018/978-1-4666-3954-6.ch004 Retrieved from www.scopus.com .
McAfee, A. P. (2006). Enterprise 2.0: The dawn of emergent collaboration. Enterprise, 2 , 15–26.
McKinsey & Company (2015). Transforming the business through social tools. McKinsey.com , ( http://www.mckinsey.com/industries/high-tech/our-insights/transformingthe-business-through-social-tools ).
McShane, L., Pancer, E., & Poole, M. (2019). The influence of B to B social media message features on brand engagement: A fluency perspective. Journal of Business-to-Business Marketing, 26 (1), 1–18. https://doi.org/10.1080/1051712X.2019.1565132 .
Mehmet, M. I., & Clarke, R. J. (2016). B2B social media semantics: Analysing multimodal online meanings in marketing conversations. Industrial Marketing Management, 54 , 92–106. https://doi.org/10.1016/j.indmarman.2015.12.006 .
Meire, M., Ballings, M., & Van den Poel, D. (2017). The added value of social media data in B2B customer acquisition systems: A real-life experiment. Decision Support Systems, 104 , 26–37. https://doi.org/10.1016/j.dss.2017.09.010 .
Michaelidou, N., Siamagka, N. T., & Christodoulides, G. (2011). Usage, barriers and measurement of social media marketing: An exploratory investigation of small and medium B2B brands. Industrial Marketing Management, 40 (7), 1153–1159. https://doi.org/10.1016/j.indmarman.2011.09.009 .
Mikalef, P., Pappas, I. O., Krogstie, J., & Pavlou, P. A. (2020a). Big data and business analytics: A research agenda for realizing business value. Information & Management, 57 (1), 103237.
Mikalef, P., Sharma, K., Pappas, I, O., & Giannakos, M. (2020b). Seeking information on social commerce: An examination of the impact of user-and marketer-generated content through an eye-tracking study. Information Systems Frontiers , 1-14, doi: https://doi.org/10.1007/s10796-020-10034-3 .
Minsky, L., & Quesenberry, K, A. (2016). How B2B sales can benefit from social selling . Harvard Business Review. Available at https://hbr.org/2016/11/84-of-b2b-sales-start-with-a-referral-not-a-salesperson . Accessed 28 Jan 2021.
Moncrief, W. C., Marshall, G. W., & Rudd, J. M. (2015). Social media and related technology: Drivers of change in managing the contemporary sales force. Business Horizons, 58 (1), 45–55. https://doi.org/10.1016/j.bushor.2014.09.009 .
Moore, J. N., Hopkins, C. D., & Raymond, M. A. (2013). Utilization of relationship-oriented social media in the selling process: A comparison of consumer (B2C) and industrial (B2B) salespeople. Journal of Internet Commerce, 12 (1), 48–75. https://doi.org/10.1080/15332861.2013.763694 .
Moore, J. N., Raymond, M. A., & Hopkins, C. D. (2015). Social selling: A comparison of social media usage across process stage, markets, and sales job functions. Journal of Marketing Theory and Practice, 23 (1), 1–20. https://doi.org/10.1080/10696679.2015.980163 .
Mudambi, S. M., Sinha, J. I., & Taylor, D. S. (2019). Why B-to-B CEOs should be more social on social media. Journal of Business-to-Business Marketing, 26 (1), 103–105. https://doi.org/10.1080/1051712X.2019.1565144 .
Muhammad, S. S., Dey, B. L., & Weerakkody, V. (2018). Analysis of factors that influence customers’ willingness to leave big data digital footprints on social media: A systematic review of literature. Information Systems Frontiers, 20 (3), 559–576.
Müller, L., Griesbaum, J., & Mandl, T. (2013). Social media relations in the german automotive market. Paper presented at the proceedings of the IADIS international conference ICT, society and human beings 2013, Proceedings of the IADIS International Conference e-Commerce 2013, 19–26.
Müller, J. M., Pommeranz, B., Weisser, J., & Voigt, K. I. (2018). Digital, social media, and Mobile marketing in industrial buying: Still in need of customer segmentation? Empirical evidence from Poland and Germany. Industrial Marketing Management, 73 , 70–83. https://doi.org/10.1016/j.indmarman.2018.01.033 .
Niedermeier, K. E., Wang, E., & Zhang, X. (2016). The use of social media among business-to-business sales professionals in China: How social media helps create and solidify guanxi relationships between sales professionals and customers. Journal of Research in Interactive Marketing, 10 (1), 33–49. https://doi.org/10.1108/JRIM-08-2015-0054 .
Nunan, D., Sibai, O., Schivinski, B., & Christodoulides, G. (2018). Reflections on “social media: Influencing customer satisfaction in B2B sales” and a research agenda. Industrial Marketing Management, 75 , 31–36. https://doi.org/10.1016/j.indmarman.2018.03.009 .
Ogilvie, J., Agnihotri, R., Rapp, A., & Trainor, K. (2018). Social media technology use and salesperson performance: A two study examination of the role of salesperson behaviors, characteristics, and training. Industrial Marketing Management, 75 , 55–65. https://doi.org/10.1016/j.indmarman.2018.03.007 .
Oliveira, T., & Martins, M. F. (2011). Literature review of information technology adoption models at firm level. Electronic Journal of Information Systems Evaluation, 14 (1), 110.
Olotewo, J. (2016). Social media marketing in emerging markets. International Journal of Online Marketing Research, 2 (2), 10–18.
Pappas, I. O., Mikalef, P., Giannakos, M. N., Krogstie, J., & Lekakos, G. (2018). Big data and business analytics ecosystems: Paving the way towards digital transformation and sustainable societies. Information Systems and e-Business Management, 16 , 479–491.
Pappas, I. O., Papavlasopoulou, S., Mikalef, P., & Giannakos, M. N. (2020). Identifying the combinations of motivations and emotions for creating satisfied users in SNSs: An fsQCA approach. International Journal of Information Management, 53 , 102128.
Pascucci, F., Ancillai, C., & Cardinali, S. (2018). Exploring antecedents of social media usage in B2B: A systematic review. Management Research Review, 41 (6), 629–656. https://doi.org/10.1108/MRR-07-2017-0212 .
Pitt, C. S., Plangger, K. A., Botha, E., Kietzmann, J., & Pitt, L. (2017). How employees engage with B2B brands on social media: Word choice and verbal tone. Industrial Marketing Management, 81 , 130–137. https://doi.org/10.1016/j.indmarman.2017.09.012 .
Pitt, C. S., Botha, E., Ferreira, J. J., & Kietzmann, J. (2018). Employee brand engagement on social media: Managing optimism and commonality. Business Horizons, 61 (4), 635–642. https://doi.org/10.1016/j.bushor.2018.04.001 .
Porter, M. E., & Millar, V. E. (1985). How information gives you competitive advantage. Harvard Business Review, 63 (4), 149–160.
Pulizzi, J., & Handley, A. (2017). B2C content marketing-2018 benchmarks, budgets, and trends—North America. Available at https://contentmarketinginstitute.com/wp-content/uploads/2016/09/2017_B2B_Research_FINAL.pdf Accessed on April , 27 , 2020.
Rana, N. P., Dwivedi, Y. K., & Williams, M. D. (2015). A meta-analysis of existing research on citizen adoption of e-government. Information Systems Frontiers, 17 (3), 547–563.
Rana, N. P., Luthra, S., Mangla, S. K., Islam, R., Roderick, S., & Dwivedi, Y. K. (2019). Barriers to the development of smart cities in Indian context. Information Systems Frontiers, 21 (3), 503–525.
Rodriguez, M., Peterson, R. M., & Krishnan, V. (2012). Social media's influence on business-to-business sales performance. Journal of Personal Selling and Sales Management, 32 (3), 365–378. https://doi.org/10.2753/PSS0885-3134320306 .
Rossmann, A., & Stei, G. (2015). Sales 2.0 in business-to-business (B2B) networks: Conceptualization and impact of social media in B2B sales relationships . Paper presented at the Lecture Notes in Informatics (LNI), Proceedings - Series of the Gesellschaft Fur Informatik (GI), 244 67–78. Available at https://subs.emis.de/LNI/Proceedings/Proceedings244/67.pdf . Accessed 28 Jan 2021.
Salo, J. (2017). Social media research in the industrial marketing field: Review of literature and future research directions. Industrial Marketing Management, 66 , 115–129. https://doi.org/10.1016/j.indmarman.2017.07.013 .
Shaltoni, A. M. (2017). From websites to social media: Exploring the adoption of internet marketing in emerging industrial markets. Journal of Business and Industrial Marketing, 32 (7), 1009–1019. https://doi.org/10.1108/JBIM-06-2016-0122 .
Siamagka, N. T., Christodoulides, G., Michaelidou, N., & Valvi, A. (2015). Determinants of social media adoption by B2B organizations. Industrial Marketing Management, 51 , 89–99. https://doi.org/10.1016/j.indmarman.2015.05.005 .
Sivarajah, U., Irani, Z., Gupta, S., & Mahroof, K. (2019). Role of big data and social media analytics for business to business sustainability: A participatory web context. Industrial Marketing Management . https://doi.org/10.1016/j.indmarman.2019.04.005 .
Sobal, A. (2017). 30 statistics about B2B social media usage. Available at https://www.weidert.com/blog/statistics-about-b2b-social-media-usage Accessed on April , 27 , 2020.
Stelzner, M. (2011). 2012 social media marketing industry report. Social media examiner . Available at https://www.socialmediaexaminer.com/socialmedia-marketing-industry-report-2012/ . Accessed 28 Jan 2021.
Sułkowski, Ł., & Kaczorowska-Spychalska, D. (2016). Social media in the process of marketing evolution in polish textile-clothing industry. Fibres and Textiles in Eastern Europe, 24 (5), 15–20. https://doi.org/10.5604/12303666.1215521 .
Swani, K., Milne, G., & Brown, B. P. (2013). Spreading the word through likes on facebook: Evaluating the message strategy effectiveness of fortune 500 companies. Journal of Research in Interactive Marketing, 7 (4), 269–294. https://doi.org/10.1108/JRIM-05-2013-0026 .
Swani, K., Brown, B. P., & Milne, G. R. (2014). Should tweets differ for B2B and B2C? An analysis of fortune 500 companies' twitter communications. Industrial Marketing Management, 43 (5), 873–881. https://doi.org/10.1016/j.indmarman.2014.04.012 .
Swani, K., Milne, G. R., Brown, B. P., Assaf, A. G., & Donthu, N. (2017). What messages to post? Evaluating the popularity of social media communications in business versus consumer markets. Industrial Marketing Management, 62 , 77–87. https://doi.org/10.1016/j.indmarman.2016.07.006 .
Tedeschi, B. (2006). Like shopping? Social networking? Try social shopping. New York Times, 11 , 09.
Teo, T. S., & Choo, W. Y. (2001). Assessing the impact of using the internet for competitive intelligence. Information & Management, 39 (1), 67–83.
Tseng, M. L. (2017). Using social media and qualitative and quantitative information scales to benchmark corporate sustainability. Journal of Cleaner Production, 142 , 727–738.
Vasudevan, S., & Kumar, F. J. P. (2018). Social media and B2B brands: An Indian perspective. International Journal of Mechanical Engineering and Technology, 9 (9), 767–775.
Vukanovic, Z. (2013). Managing social media value networks: From publisher (broadcast) to user-centric (broadband-narrowcast) business models. Handbook of social media management: Value chain and business models in changing media markets (pp. 269-288) doi: https://doi.org/10.1007/978-3-642-28897-5_16 .
Wang, Y., Rod, M., Ji, S., & Deng, Q. (2017). Social media capability in B2B marketing: Toward a definition and a research model. Journal of Business and Industrial Marketing, 32 (8), 1125–1135. https://doi.org/10.1108/JBIM-10-2016-0250 .
Watt, I. (2010). Changing visions of parliamentary libraries: From the enlightenment to Facebook. IFLA Journal, 36 (1), 47–60. https://doi.org/10.1177/0340035209359574 .
Webster, J., & Watson, R.,. T. (2002). Analyzing the past to prepare for the future: Writing a literature review. MIS quarterly, 26 (2), xiii–xxiii.
Westerman, G., Bonnet, D., & McAfee, A. (2014). Leading digital: Turning technology into business transformation . Boston, MA: Harvard Business Press.
Williams, M. D., Rana, N. P., & Dwivedi, Y. K. (2015). The unified theory of acceptance and use of technology (UTAUT): A literature review. Journal of Enterprise Information Management, 28 (3), 443–488.
World Commission Report on Environment and Development. (1987). Our Common Future . Oxford: Oxford University Press.
Yang, C. C., Yang, H., Tang, X., & Jiang, L. (2012). Identifying implicit relationships between social media users to support social commerce. In ACM international conference proceeding series (pp. 41–47). https://doi.org/10.1145/2346536.2346544 .
Chapter Google Scholar
Download references
Author information
Authors and affiliations.
Emerging Markets Research Centre (EMaRC), School of Management, Swansea University, Bay Campus, Swansea, UK
Yogesh K. Dwivedi
School of Management, University of Bradford, Bradford, UK
Elvira Ismagilova & Nripendra P. Rana
Symbiosis Institute of Business Management, Pune & Symbiosis International, Deemed University, Pune, India
Ramakrishnan Raman
You can also search for this author in PubMed Google Scholar
Corresponding author
Correspondence to Yogesh K. Dwivedi .
Additional information
Publisher’s note.
Springer Nature remains neutral with regard to jurisdictional claims in published maps and institutional affiliations.
Rights and permissions
Open Access This article is licensed under a Creative Commons Attribution 4.0 International License, which permits use, sharing, adaptation, distribution and reproduction in any medium or format, as long as you give appropriate credit to the original author(s) and the source, provide a link to the Creative Commons licence, and indicate if changes were made. The images or other third party material in this article are included in the article's Creative Commons licence, unless indicated otherwise in a credit line to the material. If material is not included in the article's Creative Commons licence and your intended use is not permitted by statutory regulation or exceeds the permitted use, you will need to obtain permission directly from the copyright holder. To view a copy of this licence, visit http://creativecommons.org/licenses/by/4.0/ .
Reprints and permissions
About this article
Dwivedi, Y.K., Ismagilova, E., Rana, N.P. et al. Social Media Adoption, Usage And Impact In Business-To-Business (B2B) Context: A State-Of-The-Art Literature Review. Inf Syst Front 25 , 971–993 (2023). https://doi.org/10.1007/s10796-021-10106-y
Download citation
Accepted : 07 January 2021
Published : 02 February 2021
Issue Date : June 2023
DOI : https://doi.org/10.1007/s10796-021-10106-y
Share this article
Anyone you share the following link with will be able to read this content:
Sorry, a shareable link is not currently available for this article.
Provided by the Springer Nature SharedIt content-sharing initiative
- Business-to-business
- Digital transformation
- Information systems
- Literature review
- Social media
Advertisement
- Find a journal
- Publish with us
- Track your research
- Open access
- Published: 16 March 2020
Exploring the role of social media in collaborative learning the new domain of learning
- Jamal Abdul Nasir Ansari 1 &
- Nawab Ali Khan 1
Smart Learning Environments volume 7 , Article number: 9 ( 2020 ) Cite this article
376k Accesses
178 Citations
19 Altmetric
Metrics details
This study is an attempt to examine the application and usefulness of social media and mobile devices in transferring the resources and interaction with academicians in higher education institutions across the boundary wall, a hitherto unexplained area of research. This empirical study is based on the survey of 360 students of a university in eastern India, cognising students’ perception on social media and mobile devices through collaborative learning, interactivity with peers, teachers and its significant impact on students’ academic performance. A latent variance-based structural equation model approach was followed for measurement and instrument validation. The study revealed that online social media used for collaborative learning had a significant impact on interactivity with peers, teachers and online knowledge sharing behaviour.
Additionally, interactivity with teachers, peers, and online knowledge sharing behaviour has seen a significant impact on students’ engagement which consequently has a significant impact on students’ academic performance. Grounded to this finding, it would be valuable to mention that use of online social media for collaborative learning facilitate students to be more creative, dynamic and research-oriented. It is purely a domain of knowledge.
Introduction
The explosion of Information and Communication Technology (ICT) has led to an increase in the volume and smoothness in transferring course contents, which further stimulates the appeasement of Digital Learning Communities (DLCs). The millennium and naughtiness age bracket were Information Technology (IT) centric on web space where individual and geopolitical disperse learners accomplished their e-learning goals. The Educause Center for Applied Research [ECAR] ( 2012 ) surveyed students in higher education mentioned that students are pouring the acceptance of mobile computing devices (cellphones, smartphones, and tablet) in Higher Education Institutions (HEIs), roughly 67% surveyed students accepted that mobile devices and social media play a vital role in their academic performance and career enhancement. Mobile devices and social media provide excellent educational e-learning opportunities to the students for academic collaboration, accessing in course contents, and tutors despite the physical boundary (Gikas & Grant, 2013 ). Electronic communication technologies accelerate the pace of their encroachment of every aspect of life, the educational institutions incessantly long decades to struggle in seeing the role of such devices in sharing the contents, usefulness and interactivity style. Adoption and application of mobile devices and social media can provide ample futuristic learning opportunities to the students in accessing course contents as well as interaction with peers and experts (Cavus & Ibrahim, 2008 , 2009 ; Kukulska-Hulme & Shield, 2008 ; Nihalani & Mayrath, 2010 ; Richardson & Lenarcic, 2008 , Shih, 2007 ). Recently Pew Research Center reported that 55% American teenage age bracket of 15–17 years using online social networking sites, i.e. Myspace and Facebook (Reuben, 2008 ). Social media, the fast triggering the mean of virtual communication, internet-based technologies changed the life pattern of young youth.
Use of social media and mobile devices presents both advantages as well as challenges, mostly its benefits seen in terms of accessing course contents, video clip, transfer of the instructional notes etc. Overall students feel that social media and mobile devices are the cheap and convenient tools of obtaining relevant information. Studies in western countries have confronted that online social media use for collaborative learning has a significant contribution to students’ academic performance and satisfaction (Zhu, 2012 ). The purpose of this research project was to explore how learning and teaching activities in higher education institutions were affected by the integration and application of mobile devices in sharing the resource materials, interaction with colleagues and students’ academic performance. The broad goal of this research was to contemporise the in-depth perspectives of students’ perception of mobile devices and social media in learning and teaching activities. However, this research paper paid attention to only students’ experiences, and their understanding of mobile devices and social media fetched changes and its competency in academic performance. The fundamental research question of this research was, what are the opinions of students on social media and mobile devices when it is integrating into higher education for accessing, interacting with peers.
A researcher of the University of Central Florida reported that electronic devices and social media create an opportunity to the students for collaborative learning and also allowed the students in sharing the resource materials to the colleagues (Gikas & Grant, 2013 ). The result of the eight Egyptian universities confirmed that social media have the significant impact on higher education institutions especially in term of learning tools and teaching aids, faculty members’ use of social media seen at a minimum level due to several barriers (internet accessibility, mobile devices etc.).
Social media and mobile devices allow the students to create, edit and share the course contents in textual, video or audio forms. These technological innovations give birth to a new kind of learning cultures, learning based on the principles of collective exploration and interaction (Selwyn, 2012 ). Social media the phenomena originated in 2005 after the Web2.0 existence into the reality, defined more clearly as “a group of Internet-based applications that build on the ideological and technological foundation of web 2.0 and allow creation and exchange of user-generated contents (Kaplan & Haenlein, 2010 ). Mobile devices and social media provide opportunities to the students for accessing resources, materials, course contents, interaction with mentor and colleagues (Cavus & Ibrahim, 2008 , 2009 ; Richardson & Lenarcic, 2008 ).
Social media platform in academic institutions allows students to interact with their mentors, access their course contents, customisation and build students communities (Greenhow, 2011a , 2011b ). 90% school going students currently utilise the internet consistently, with more than 75% teenagers using online networking sites for e-learning (DeBell & Chapman, 2006 ; Lenhart, Arafeh, & Smith, 2008 ; Lenhart, Madden, & Hitlin, 2005 ). The result of the focus group interview of the students in 3 different universities in the United States confirmed that use of social media created opportunities to the learners for collaborative learning, creating and engaging the students in various extra curriculum activities (Gikas & Grant, 2013 ).
Research background and hypotheses
The technological innovation and increased use of the internet for e-learning by the students in higher education institutions has brought revolutionary changes in communication pattern. A report on 3000 college students in the United States revealed that 90% using Facebook while 37% using Twitter to share the resource materials as cited in (Elkaseh, Wong, & Fung, 2016 ). A study highlighted that the usage of social networking sites in educational institutions has a practical outcome on students’ learning outcomes (Jackson, 2011 ). The empirical investigation over 252 undergraduate students of business and management showed that time spent on twitter and involvement in managing social lives and sharing information, course-related influences their performance (Evans, 2014 ).
Social media for collaborative learning, interactivity with teachers, interactivity with peers
Many kinds of research confronted on the applicability of social media and mobile devices in higher education for interaction with colleagues.90% of faculty members use some social media in courses they were usually teaching or professional purposes out of the campus life. Facebook and YouTube are the most visited sites for the professional outcomes, around 2/3rd of the all-faculty use some medium fora class session, and 30% posted contents for students engagement in reading, view materials (Moran, Seaman, & Tinti-Kane, 2011 ). Use of social media and mobile devices in higher education is relatively new phenomena, completely hitherto area of research. Research on the students of faculty of Economics at University of Mortar, Bosnia, and Herzegovina reported that social media is already used for the sharing the materials and exchanges of information and students are ready for active use of social networking site (slide share etc.) for educational purposes mainly e-learning and communication (Mirela Mabić, 2014 ).
The report published by the U.S. higher education department stated that the majority of the faculty members engaged in different form of the social media for professional purposes, use of social media for teaching international business, sharing contents with the far way students, the use of social media and mobile devices for sharing and the interactive nature of online and mobile technologies build a better learning environment at international level. Responses on 308 graduate and postgraduate students in Saudi Arabia University exhibited that positive correlation between chatting, online discussion and file sharing and knowledge sharing, and entertainment and enjoyment with students learning (Eid & Al-Jabri, 2016 ). The quantitative study on 168 faculty members using partial least square (PLS-SEM) at Carnegie classified Doctoral Research University in the USA confirmed that perceived usefulness, external pressure and compatibility of task-technology have positive effect on social media use, the higher the degree of the perceived risk of social media, the less likely to use the technological tools for classroom instruction, the study further revealed that use of social media for collaborative learning has a positive effect on students learning outcome and satisfaction (Cao, Ajjan, & Hong, 2013 ). Therefore, the authors have hypothesized:
H1: Use of social media for collaborative learning is positively associated with interactivity with teachers.
Additionally, Madden and Zickuhr ( 2011 ) concluded that 83% of internet user within the age bracket of 18–29 years adopting social media for interaction with colleagues. Kabilan, Ahmad, and Abidin ( 2010 ) made an empirical investigation on 300 students at University Sains Malaysia and concluded that 74% students found to be the same view that social media infuses constructive attitude towards learning English (Fig. 1 ).
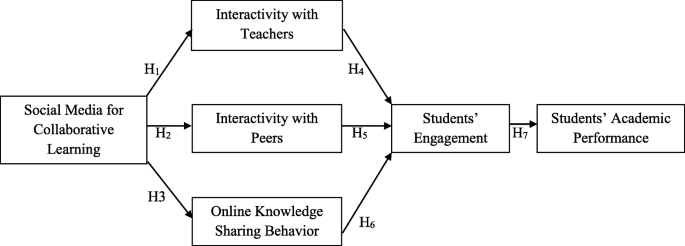
Research Model
Reuben ( 2008 ) concluded in his study on social media usage among professional institutions revealed that Facebook and YouTube used over half of 148 higher education institutions. Nevertheless, a recent survey of 456 accredited United States institutions highlighted 100% using some form of social media, notably Facebook 98% and Twitter 84% for e-learning purposes, interaction with mentors (Barnes & Lescault, 2011 ).
Information and communication technology (ICT), such as web-based application and social networking sites enhances the collaboration and construction of knowledge byway of instruction with outside experts (Zhu, 2012 ). A positive statistically significant relationship was found between student’s use of a variety of social media tools and the colleague’s fellow as well as the overall quality of experiences (Rutherford, 2010 ). The potential use of social media leads to collaborative learning environments which allow students to share education-related materials and contents (Fisher & Baird, 2006 ). The report of 233 students in the United States higher educations confirmed that more recluse students interact through social media, which assist them in collaborative learning and boosting their self-confidence (Voorn & Kommers, 2013 ). Thus hypotheses as
H2: Use of social media for collaborative learning is positively associated with interactivity with peers.
Social media for collaborative learning, interactivity with peers, online knowledge sharing behaviour and students’ engagement
Students’ engagement in social media and its types represent their physical and mental involvement and time spent boost to the enhancement of educational Excellency, time spent on interaction with peers, teachers for collaborative learning (Kuh, 2007 ). Students’ engagement enhanced when interacting with peers and teacher was in the same direction, shares of ideas (Chickering & Gamson, 1987 ). Engagement is an active state that is influenced by interaction or lack thereof (Leece, 2011 ). With the advancement in information technology, the virtual world becomes the storehouse of the information. Liccardi et al. ( 2007 ) concluded that 30% students were noted to be active on social media for interaction with their colleagues, tutors, and friends while more than 52% used some social media forms for video sharing, blogs, chatting, and wiki during their class time. E-learning becomes now sharp and powerful tools in information technology and makes a substantial impact on the student’s academic performance. Sharing your knowledge will make you better. Social network ties were shown to be the best predictors of online knowledge sharing intention, which in turn associated with knowledge sharing behaviour (Chen, Chen, & Kinshuk, 2009 ). Social media provides the robust personalised, interactive learning environment and enhances in self-motivation as cited in (Al-Mukhaini, Al-Qayoudhi, & Al-Badi, 2014 ). Therefore, it was hypothesised that:
H3: Use of social media for collaborative learning is positively associated with online knowledge sharing behaviour.
Broadly Speaking social media/sites allow the students to interact, share the contents with colleagues, also assisting in building connections with others (Cain, 2008 ). In the present era, the majority of the college-going students are seen to be frequent users of these sophisticated devices to keep them informed and updated about the external affair. Facebook reported per day 1,00,000 new members join; Facebook is the most preferred social networking sites among the students of the United States as cited in (Cain, 2008 ). The researcher of the school of engineering, Swiss Federal Institute of Technology Lausanne, Switzerland, designed and developed Grasp, a social media platform for their students’ collaborative learning, sharing contents (Bogdanov et al., 2012 ). The utility and its usefulness could be seen in the University of Geneva and Tongji University at both two educational places students were satisfied and accept ‘ Grasp’ to collect, organised and share the contents. Students use of social media will interact ubiquity, heterogeneous and engaged in large groups (Wankel, 2009 ). So we hypotheses
H4: More interaction with teachers leads to higher students’ engagement.
However, a similar report published on 233 students revealed that social media assisted in their collaborative learning and self-confidence as they prefer communication technology than face to face communication. Although, the students have the willingness to communicate via social media platform than face to face (Voorn & Kommers, 2013 ). The potential use of social media tools facilitates in achieving higher-level learning through collaboration with colleagues and other renewed experts in their field (Junco, Heiberger, & Loken, 2011 ; Meyer, 2010 ; Novak, Razzouk, & Johnson, 2012 ; Redecker, Ala-Mutka, & Punie, 2010 ). Academic self-efficacy and optimism were found to be strongly related to performance, adjustment and consequently both directly impacted on student’s academic performance (Chemers, Hu, & Garcia, 2001 ). Data of 723 Malaysian researchers confirmed that both male and female students were satisfied with the use of social media for collaborative learning and engagement was found positively affected with learning performance (Al-Rahmi, Alias, Othman, Marin, & Tur, 2018 ). Social media were seen as a powerful driver for learning activities in terms of frankness, interactivity, and friendliness.
Junco et al. ( 2011 ) conducted research on the specific purpose of the social media; how Twitter impacted students’ engagement, found that it was extent discussion out of class, their participation in panel group (Rodriguez, 2011 ). A comparative study conducted by (Roblyer, McDaniel, Webb, Herman, & Witty, 2010 ) revealed that students were more techno-oriented than faculty members and more likely using Facebook and such similar communication technology to support their class-related task. Additionally, faculty members were more likely to use traditional techniques, i.e. email. Thus hypotheses framed is that:
H5: More interaction with peers ultimately leads to better students’ engagement.
Social networking sites and social media are closely similar, which provide a platform where students can interact, communicate, and share emotional intelligence and looking for people with other attitudes (Gikas & Grant, 2013 ). Facebook and YouTube channel use also increased in the skills/ability and knowledge and outcomes (Daniel, Isaac, & Janet, 2017 ). It was highlighted that 90% of faculty members were using some sort of social media in their courses/ teaching. Facebook was the most visited social media sites as per study, 40% of faculty members requested students to read and views content posted on social media; majority reports that videos, wiki, etc. the primary source of acquiring knowledge, social networking sites valuable tool/source of collaborative learning (Moran et al., 2011 ). However, more interestingly, in a study which was carried out on 658 faculty members in the eight different state university of Turkey, concluded that nearly half of the faculty member has some social media accounts.
Further reported that adopting social media for educational purposes, the primary motivational factor which stimulates them to use was effective and quick means of communication technology (Akçayır, 2017 ). Thus hypotheses formulated is:
H6: Online knowledge sharing behaviour is positively associated with the students’ engagement.
Using multiple treatment research design, following act-react to increase students’ academic performance and productivity, it was observed when self–monitoring record sheet was placed before students and seen that students engagement and educational productivity was increased (Rock & Thead, 2007 ). Student engagement in extra curriculum activities promotes academic achievement (Skinner & Belmont, 1993 ), increases grade rate (Connell, Spencer, & Aber, 1994 ), triggering student performance and positive expectations about academic abilities (Skinner & Belmont, 1993 ). They are spending time on online social networking sites linked to students engagement, which works as the motivator of academic performance (Fan & Williams, 2010 ). Moreover, it was noted in a survey of over 236 Malaysian students that weak association found between the online game and student’s academic performance (Eow, Ali, Mahmud, & Baki, 2009 ). In a survey of 671 students in Jordan, it was revealed that student’s engagement directly influences academic performance, also seen the indirect effect of parental involvement over academic performance (Al-Alwan, 2014 ). Engaged students are perceptive and highly active in classroom activities, ready to participate in different classroom extra activities and expose motivation to learn, which finally leads in academic achievement (Reyes, Brackett, Rivers, White, & Salovey, 2012 ). A mediated role of students engagement seen in 1399 students’ classroom emotional climate and grades (Reyes et al., 2012 ). A statistically significant relation was noticed between online lecture and exam performance.
Nonetheless, intelligence quotient, personality factors, students must be engaged in learning activities as cited in (Bertheussen & Myrland, 2016 ). The report of the 1906 students at 7 universities in Colombia confirmed that the weak correlation between collaborative learning, students faculty interaction with academic performance (Pineda-Báez et al., 2014 ) Thus, the hypothesis
H7: Student's Engagement is positively associated with the student's academic performance.
Methodology
To check the students’ perception on social media for collaborative learning in higher education institutions, Data were gathered both offline and online survey administered to students from one public university in Eastern India (BBAU, Lucknow). For the sake of this study, indicators of interactivity with peers and teachers, the items of students engagement, the statement of social media for collaborative learning, and the elements of students’ academic performance were adopted from (AL-Rahmi & Othman, 2013 ). The statement of online knowledge sharing behaviour was taken from (Ma & Yuen, 2011 ).
The indicators of all variables which were mentioned above are measured on the standardised seven-point Likert scale with the anchor (1-Strongly Disagree, to 7-Strongly Agree). Interactivity with peers was measured using four indicators; the sample items using social media in class facilitates interaction with peers ; interactivity with teachers was measured using four symbols, the sample item is using social media in class allows me to discuss with the teacher. ; engagement was measured using three indicators by using social media I felt that my opinions had been taken into account in this class ; social media for collaborative learning was measured using four indicators collaborative learning experience in social media environment is better than in a face-to-face learning environment ; students’ academic performance was measured using five signs using social media to build a student-lecturer relationship with my lecturers, and this improves my academic performance ; online knowledge sharing behaviour was assessed using five symbols the counsel was received from other colleague using social media has increased our experience .
Procedure and measurement
A sample of 360 undergraduate students was collected by convenience sampling method of a public university in Eastern India. The proposed model of study was measured and evaluated using variance based structured equation model (SEM)-a latent multi variance technique which provides the concurrent estimation of structural and measurement model that does not meet parametric assumption (Coelho & Duarte, 2016 ; Haryono & Wardoyo, 2012 ; Lee, 2007 ; Moqbel, Nevo, & Kock, 2013 ; Raykov & Marcoulides, 2000 ; Williams, Rana, & Dwivedi, 2015 ). The confirmatory factor analysis (CFA) was conducted to ensure whether the widely accepted criterion of discriminate and convergent validity met or not. The loading of all the indicators should be 0.50 or more (Field, 2011 ; Hair, Anderson, Tatham, & Black, 1992 ). And it should be statistically significant at least at the 0.05.
Demographic analysis (Table 1 )
The majority of the students in this study were females (50.8%) while male students were only 49.2% with age 15–20 years (71.7%). It could be pointed out at this juncture that the majority of the students (53.9%) in BBAU were joined at least 1–5 academic pages for their getting information, awareness and knowledge. 46.1% of students spent 1–5 h per week on social networking sites for collaborative learning, interaction with teachers at an international level. The different academic pages followed for accessing material, communication with the faculty members stood at 44.4%, there would be various forms of the social networking sites (LinkedIn, Slide Share, YouTube Channel, Researchgate) which provide the facility of online collaborative learning, a platform at which both faculty members and students engaged in learning activities.
As per report (Nasir, Khatoon, & Bharadwaj, 2018 ), most of the social media user in India are college-going students, 33% girls followed by 27% boys students, and this reports also forecasted that India is going to become the highest 370.77 million internet users in 2022. Additionally, the majority of the faculty members use smartphone 44% to connect with the students for sharing material content. Technological advantages were the pivotal motivational force which stimulates faculty members and students to exploits the opportunities of resource materials (Nasir & Khan, 2018 ) (Fig. 2 ).
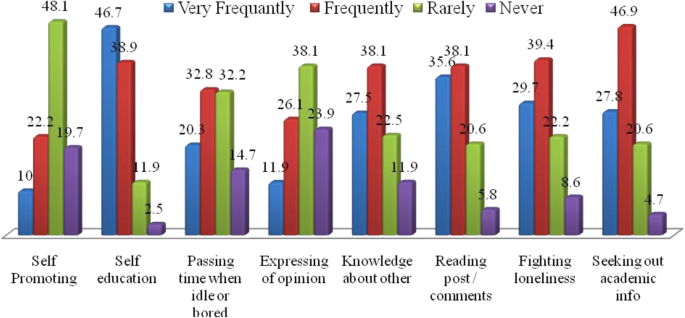
Reasons for Using Social Media
When the students were asked for what reason did they use social media, it was seen that rarely using for self-promotion, very frequently using for self-education, often used for passing the time with friends, and so many fruitful information the image mentioned above depicting.
Instrument validation
The structural model was applied to scrutinize the potency and statistically significant relationship among unobserved variables. The present measurement model was evaluated using Confirmatory Factor Analysis (CFA), and allied procedures to examine the relationship among hypothetical latent variables has acceptable reliability and validity. This study used both SPSS 20.0 and AMOS to check measurement and structural model (Field, 2013 ; Hair, Anderson, et al., 1992 ; Mooi & Sarstedt, 2011 ; Norusis, 2011 ).
The Confirmatory Factor Analysis (CFA) was conducted to ensure whether the widely accepted criterion of discriminant and convergent validity met or not. The loading of all the indicators should be 0.70 or more it should be statistically significant at least at the 0.05 (Field, 2011 ; Hair, Anderson, et al., 1992 ).
CR or CA-based tests measured the reliability of the proposed measurement model. The CA provides an estimate of the indicators intercorrelation (Henseler & Sarstedt, 2013 . The benchmark limits of the CA is 0.7 or more (Nunnally & Bernstein, 1994 ). As per Table 2 , all latent variables in this study above the recommended threshold limit. Although, Average Variance Extracted (AVE) has also been demonstrated which exceed the benchmark limit 0.5. Thus all the above-specified values revealed that our instrument is valid and effective. (See Table 2 for the additional information) (Table 3 ).
In a nutshell, the measurement model clear numerous stringent tests of convergent validity, discriminant validity, reliability, and absence of multi-collinearity. The finding demonstrated that our model meets widely accepted data validation criteria. (Schumacker & Lomax, 2010 ).
The model fit was evaluated through the Chi-Square/degree of freedom (CMIN/DF), Root Mean Residual (RMR), Root Mean Square Error of Approximation (RMSEA), Comparative Fit Index (CFI), and Goodness of fit index (GFI) and Tucker-Lewis Index (TLI). The benchmark limit of the CFI, TLI, and GFI 0.90or more (Hair et al., 2016 ; Kock, 2011 ). The model study demonstrated in the table, as mentioned above 4 that the minimum threshold limit was achieved (See Table 4 for additional diagnosis).
Path coefficient of several hypotheses has been demonstrated in Fig. 3 , which is a variable par relationship. β (beta) Coefficients, standardised partial regression coefficients signify the powers of the multivariate relationship among latent variables in the model. Remarkably, it was observed that seven out of the seven proposed hypotheses were accepted and 78% of the explained variance in students’ academic performance, 60% explained variance in interactivity with teachers, 48% variance in interactivity with peers, 43% variance in online knowledge sharing behaviour and 79% variance in students’ engagement. Social media collaborative learning has a significant association with teacher interactivity(β = .693, P < 0.001), demonstrating that there is a direct effect on interaction with the teacher by social media when other variables are controlled. On the other hand, use of social media for collaborative learning has noticed statistically significant positive relationship with peers interactivity (β = .704, p < 0.001) meaning thereby, collaborative learning on social media by university students, leads to the high degree of interaction with peers, colleagues. Implied 10% rise in social media use for learning purposes, expected 7.04% increase in interaction with peers.
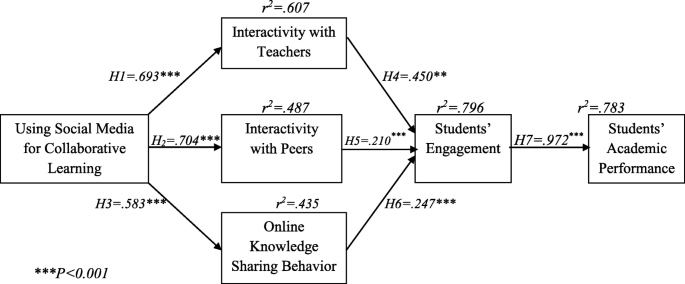
Path Diagram
Use of social media for collaborating learning has a significant positive association with online knowledge sharing behaviour (β = .583, p < 0.001), meaning thereby that the more intense use of social media for collaborative learning by university students, the more knowledge sharing between peers and colleagues. Also, interaction with the teacher seen the significant statistical positive association with students engagement (β = .450, p < 0.001), telling that the more conversation with teachers, leads to a high level of students engagement. Similarly, the practical interpretation of this result is that there is an expected 4.5% increase in student’s participation for every 10% increase in interaction with teachers. Interaction with peers has a significant positive association with students engagement (β = .210, p < 0.001). Practically, the finding revealed that 10% upturn in student’s involvement, there is a 2.1% increase in peer’s interaction. There is a significant positive association between online knowledge sharing behaviour and students engagement (β = 0.247, p < 0.001), and finally students engagement has been a statistically significant positive relationship with students’ academic performance (β = .972, p < 0.001), this is the clear indication that more engaged students in collaborative learning via social media leads to better students’ academic performance.
Discussion and implication
There is a continuing discussion in the academic literature that use of such social media and social networking sites would facilitate collaborative learning. It is human psychology generally that such communication media technology seems only for entertainment, but it should be noted here carefully that if such communication technology would be followed with due attention prove productive. It is essential to acknowledge that most university students nowadays adopting social media communication to interact with colleagues, teachers and also making the group be in touch with old friends and even a convenient source of transferring the resources. In the present era, the majority of the university students having diversified social media community groups like Whatsapp, Facebook pages following different academic web pages to upgrade their knowledge.
Practically for every 10% rise in students’ engagement, expected to be 2.1% increase in peer interaction. As the study suggested that students engage in different sites, they start discussing with colleagues. More engaged students in collaborative learning through social media lead better students’ academic performance. The present study revealed that for every 10% increase in student’s engagement, there would be an expected increase in student academic performance at a rate of 9.72. This extensive research finding revealed that the application of online social media would facilitate the students to become more creative, dynamics and connect to the worldwide instructor for collaborative learning.
Accordingly, the use of online social media for collaborative learning, interaction with mentors and colleagues leadbetter student’s engagement which consequently affects student’s academic performance. The higher education authority should provide such a platform which can nurture the student’s intellectual talents. Based on the empirical investigation, it would be said that students’ engagement, social media communication devices facilitate students to retrieve information and interact with others in real-time regarding sharing teaching materials contents. Additionally, such sophisticated communication devices would prove to be more useful to those students who feel too shy in front of peers; teachers may open up on the web for the collaborative learning and teaching in the global scenario and also beneficial for physically challenged students. It would also make sense that intensive use of such sophisticated technology in teaching pedagogical in higher education further facilitates the teachers and students to interact digitally, web-based learning, creating discussion group, etc. The result of this investigation confirmed that use of social media for collaborative learning purposes, interaction with peers, and teacher affect their academic performance positively, meaning at this moment that implementation of such sophisticated communication technology would bring revolutionary, drastic changes in higher education for international collaborative learning (Table 5 ).
Limitations and future direction
Like all the studies, this study is also not exempted from the pitfalls, lacunas, and drawbacks. The first and foremost research limitation is it ignores the addiction of social media; excess use may lead to destruction, deviation from the focal point. The study only confined to only one academic institution. Hence, the finding of the project cannot be generalised as a whole. The significant positive results were found in this study due to the fact that the social media and mobile devices are frequently used by the university going students not only as a means of gratification but also for educational purposes.
Secondly, this study was conducted on university students, ignoring the faculty members, it might be possible that the faculty members would not have been interested in interacting with the students. Thus, future research could be possible towards faculty members in different higher education institutions. To the authors’ best reliance, this is the first and prime study to check the usefulness and applicability of social media in the higher education system in the Indian context.
Concluding observations
Based on the empirical investigation, it could be noted that application and usefulness of the social media in transferring the resource materials, collaborative learning and interaction with the colleagues as well as teachers would facilitate students to be more enthusiastic and dynamic. This study provides guidelines to the corporate world in formulating strategies regarding the use of social media for collaborative learning.
Availability of data and materials
The corresponding author declared here all types of data used in this study available for any clarification. The author of this manuscript ready for any justification regarding the data set. To make publically available of the data used in this study, the seeker must mail to the mentioned email address. The profile of the respondents was completely confidential.
Akçayır, G. (2017). Why do faculty members use or not use social networking sites for education? Computers in Human Behavior, 71 , 378–385.
Article Google Scholar
Al-Alwan, A. F. (2014). Modeling the relations among parental involvement, school engagement and academic performance of high school students. International Education Studies, 7 (4), 47–56.
Al-Mukhaini, E. M., Al-Qayoudhi, W. S., & Al-Badi, A. H. (2014). Adoption of social networking in education: A study of the use of social networks by higher education students in Oman. Journal of International Education Research, 10 (2), 143–154.
Google Scholar
Al-Rahmi, W. M., Alias, N., Othman, M. S., Marin, V. I., & Tur, G. (2018). A model of factors affecting learning performance through the use of social media in Malaysian higher education. Computers & Education, 121 , 59–72.
Al-Rahmi, W. M., & Othman, M. S. (2013). Evaluating student’s satisfaction of using social media through collaborative learning in higher education. International Journal of Advances in Engineering & Technology, 6 (4), 1541–1551.
Arbuckle, J. (2008). Amos 17.0 user's guide . Chicago: SPSS Inc..
Barnes, N. G., & Lescault, A. M. (2011). Social media adoption soars as higher-ed experiments and reevaluates its use of new communications tools . North Dartmouth: Center for Marketing Research. University of Massachusetts Dartmouth.
Bertheussen, B. A., & Myrland, Ø. (2016). Relation between academic performance and students’ engagement in digital learning activities. Journal of Education for Business, 91 (3), 125–131.
Bogdanov, E., Limpens, F., Li, N., El Helou, S., Salzmann, C., & Gillet, D. (2012). A social media platform in higher education. In Proceedings of the 2012 IEEE Global Engineering Education Conference (EDUCON) (pp. 1–8). IEEE.
Byrne, B. M. (1994). Structural equation modeling with EQS and EQS/windows: basic concepts, applications, and programming . Thousand Oaks: Sage.
Cain, J. (2008). Online social networking issues within academia and pharmacy education. American Journal of Pharmaceutical Education. https://doi.org/10.5688/aj720110 .
Cao, Y., Ajjan, H., & Hong, P. (2013). Using social media applications for educational outcomes in college teaching: a structural equation analysis. British Journal of Educational Technology, 44 (4), 581–593. https://doi.org/10.1111/bjet.12066 .
Cavus, N., & Ibrahim, D. (2008). A mobile tool for learning English words, Online Submission (pp. 6–9) Retrieved from http://libezproxy.open.ac.uk/login?url=http://search.ebscohost.com/login.aspx?direct=true&db=eric&AN=ED504283&site=ehost-live&scope=site .
Cavus, N., & Ibrahim, D. (2009). M-learning: An experiment in using SMS to support learning new English language words. British Journal of Educational Technology, 40 (1), 78–91.
Chemers, M. M., Hu, L. T., & Garcia, B. F. (2001). Academic self-efficacy and first-year college student performance and adjustment. Journal of Educational Psychology, 93 (1), 55–64. https://doi.org/10.1037/0022-0663.93.1.55 .
Chen, I. Y. L., Chen, N.-S., & Kinshuk. (2009). International forum of Educational Technology & Society Examining the factors influencing participants’ knowledge sharing behavior in virtual learning communities published by : International forum of Educational Technology & Society Examining the factor. Educational Technology & Society, 12 (1), 134–148.
Chickering, A. W., & Gamson, Z. F. (1987). Seven principles for good practise in undergraduate education. AAHE bulletin, 3 , 7.
Coelho, J., & Duarte, C. (2016). A literature survey on older adults' use of social network services and social applications. Computers in Human Behavior, 58 , 187–205.
Connell, J. P., Spencer, M. B., & Aber, J. L. (1994). Educational risk and resilience in African-American youth: Context, self, action, and outcomes in school. Child Development, 65 (2), 493–506.
Daniel, E. A., Isaac, E. N., & Janet, A. K. (2017). Influence of Facebook usage on employee productivity: A case of university of cape coast staff. African Journal of Business Management, 11 (6), 110–116. https://doi.org/10.5897/AJBM2017.8265 .
DeBell, M., & Chapman, C. (2006). Computer and internet use by students in 2003. Statistical analysis report. NCES 2006-065. National Center for education statistics.
Dziuban, C., & Walker, J. D. (2012). ECAR Study of Undergraduate Students and Information Technology, 2012 (Research Report) . Louisville: EDUCAUSE Centre for Applied Research.
Eid, M. I. M., & Al-Jabri, I. M. (2016). Social networking, knowledge sharing, and student learning: The case of university students. Computers and Education, 99 , 14–27. https://doi.org/10.1016/j.compedu.2016.04.007 .
Elkaseh, A. M., Wong, K. W., & Fung, C. C. (2016). Perceived ease of use and perceived usefulness of social media for e-learning in Libyan higher education: A structural equation modeling analysis. International Journal of Information and Education Technology, 6 (3), 192.
Eow, Y. L., Ali, W. Z. b. W., Mahmud, R. b., & Baki, R. (2009). Form one students’ engagement with computer games and its effect on their academic achievement in a Malaysian secondary school. Computers and Education, 53 (4), 1082–1091. https://doi.org/10.1016/j.compedu.2009.05.013 .
Evans, C. (2014). Twitter for teaching: Can social media be used to enhance the process of learning? British Journal of Educational Wiley Online Library, 45 (5), 902–915. https://doi.org/10.1111/bjet.12099 .
Fan, W., & Williams, C. M. (2010). The effects of parental involvement on students’ academic self-efficacy, engagement and intrinsic motivation. Educational Psychology, 30 (1), 53–74. https://doi.org/10.1080/01443410903353302 .
Field, A. (2011). Discovering statistics using SPSS: (and sex and drugs and rock'n'roll) (Vol. 497). London: Sage.
Field, A. (2013). Factor analysis using SPSS. Scientific Research and Essays, 22 (June), 1–26. https://doi.org/10.1016/B978-0-444-52272-6.00519-5 .
Fisher, M., & Baird, D. E. (2006). Making mLearning work: Utilizing mobile technology for active exploration, collaboration, assessment, and reflection in higher education. Journal of Educational Technology Systems, 35 (1), 3–30.
Gikas, J., & Grant, M. M. (2013). Mobile computing devices in higher education: Student perspectives on learning with cellphones, smartphones & social media. Internet and Higher Education Mobile, 19 , 18–26. https://doi.org/10.1016/j.iheduc.2013.06.002 .
Greenhow, C. (2011a). Online social networks and learning. On the horizon, 19 (1), 4–12.
Greenhow, C. (2011b). Youth, learning, and social media. Journal of Educational Computing Research, 45 (2), 139–146. https://doi.org/10.2190/EC.45.2.a .
Hair Anderson, R. E., Tatham, R. L., & Black, W. C. (1992). Multivariate data analysis. International Journal of Pharmaceutics . https://doi.org/10.1016/j.ijpharm.2011.02.019 .
Hair Jr., J. F., Sarstedt, M., Matthews, L. M., & Ringle, C. M. (2016). Identifying and treating unobserved heterogeneity with FIMIX-PLS: part I–method. European Business Review.
Harrington, D. (2009). Confirmatory factor analysis . Oxford university press.
Haryono, S., & Wardoyo, P. (2012). Structural Equation Modeling (Vol. 331).
Henseler, J., & Sarstedt, M. (2013). Goodness-of-fit indices for partial least squares path modeling. Computational Statistics, 28 (2), 565–580.
Jackson, C. (2011). Your students love social media… and so can you. Teaching Tolerance, 39 , 38–41.
Junco, R., Heiberger, G., & Loken, E. (2011). The effect of twitter on college student engagement and grades. Journal of Computer Assisted Learning, 27 (2), 119–132.
Kabilan, M. K., Ahmad, N., & Abidin, M. J. Z. (2010). Facebook: An online environment for learning of English in institutions of higher education? The Internet and Higher Education, 13 (4), 179–187.
Kaplan, A. M., & Haenlein, M. (2010). Users of the world, unite! The challenges and opportunities of social media. Business Horizons, 53 (1), 59–68.
Kock, N. (2011). Using WarpPLS in e-collaboration studies: Mediating effects, control and second order variables, and algorithm choices. International Journal of e-Collaboration (IJeC), 7 (3), 1–13.
Kuh, G. D. (2007). What student engagement data tell us about college readiness. Peer Review, 9 (1), 4–8.
Kukulska-Hulme, A., & Shield, L. (2008). An overview of mobile assisted language learning: From content delivery to supported collaboration and interaction. ReCALL, 20 (3), 271–289.
Lee, S.-Y. (2007). Structural equation modeling: A Bayesian approach (Wiley series in probability and statistics). Ecotoxicology and Environmental Safety, 73 . https://doi.org/10.1016/j.ecoenv.2009.09.012 .
Leece, R. (2011). Engaging students through social media. Journal of the Australian and New Zealand Student Services Association, 38 , 10–14 Retrieved from https://www.researchgate.net/profile/Anthony_Jorm/publication/235003484_Introduction_to_guidelines_for_tertiary_education_institutions_to_assist_them_in_supporting_students_with_mental_health_problems/links/0c96052ba5314e1202000000.pdf#page=67 .
Lenhart, A., Arafeh, S., & Smith, A. (2008). Writing, technology and teens . Pew Internet & American Life Project.
Lenhart, A., Madden, M., & Hitlin, P. (2005). Teens and technology (p. 2008). Washington, DC: Pew Charitable Trusts Retrieved September 29.
Liccardi, I., Ounnas, A., Pau, R., Massey, E., Kinnunen, P., Lewthwaite, S., …, Sarkar, C. (2007). The role of social networks in students’ learning experiences. In ACM Sigcse Bulletin (39, 4, 224–237).
Ma, W. W. K., & Yuen, A. H. K. (2011). Understanding online knowledge sharing: An interpersonal relationship perspective. Computers & Education, 56 (1), 210–219.
Madden, M., & Zickuhr, K. (2011). 65% of online adults use social networking sites. Pew Internet & American Life Project, 1 , 14.
Meyer, K. A. (2010). A comparison of web 2.0 tools in a doctoral course. The Internet and Higher Education, 13 (4), 226–232.
Mirela Mabić, D. G. (2014). Facebook as a learning tool. Igarss, 2014 (1), 1–5. https://doi.org/10.1007/s13398-014-0173-7.2 .
Mooi, E., & Sarstedt, M. (2011). A concise guide to market research: The process, data, and methods using IBM SPSS statistics . Springeringer. https://doi.org/10.1007/978-3-642-12541-6 .
Moqbel, M., Nevo, S., & Kock, N. (2013). Organizational members’ use of social networking sites and job performance. Information Technology & People, 26 (3), 240–264. https://doi.org/10.1108/ITP-10-2012-0110 .
Moran, M., Seaman, J., & Tinti-Kane, H. (2011). Teaching, learning, and sharing: How Today’s higher education faculty use social media (pp. 1–16). Babson survey research group, (April. https://doi.org/10.1016/j.chb.2013.06.015 .
Nasir, J. A., & Khan, N. A. (2018). Faculty member usage of social media and mobile devices in higher education institution. International Journal of Advance and Innovative Research, 6 (1), 17–25.
Nasir, J. A., Khatoon, A., & Bharadwaj, S. (2018). Social media users in India: A futuristic approach. International Journal of Research and Analytical Reviews, 5 (4), 762–765 Retrieved from http://ijrar.com/ .
Nihalani, P. K., & Mayrath, M. C. (2010). Statistics I. Findings from using an iPhone app in a higher education course. In White Paper .
Norusis, M. (2011). IBM SPSS statistics 20 brief guide (pp. 1–170). IBM Corporation Retrieved from http://www.ibm.com/support .
Novak, E., Razzouk, R., & Johnson, T. E. (2012). The educational use of social annotation tools in higher education: A literature review. The Internet and Higher Education, 15 (1), 39–49.
Nunnally, J. C., & Bernstein, I. H. (1994). Psychological theory .
Pineda-Báez, C., José-Javier, B. A., Rubiano-Bello, Á., Pava-García, N., Suárez-García, R., & Cruz-Becerra, F. (2014). Student engagement and academic performance in the Colombian University context. RELIEVE-Revista Electrónica de Investigación y Evaluación Educativa, 20 (2), 1–19.
Raykov, T., & Marcoulides, G. A. (2000). A First Course in Structural Equation Modeling .
Redecker, C., Ala-Mutka, K., & Punie, Y. (2010). Learning 2.0-the impact of social media on learning in Europe. Policy brief. JRC scientific and technical report. EUR JRC56958 EN, Available from http://bit.ly/cljlpq . Accessed 6 Feb 2011.
Reuben, B. R. (2008). The use of social Media in Higher Education for marketing and communications : A guide for professionals in higher education (Vol. 5) Retrieved from httpdoteduguru comwpcontentuploads200808socialmediainhighereducation pdf)). https://doi.org/10.1108/S2044-9968(2012)0000005018 .
Book Google Scholar
Reyes, M. R., Brackett, M. A., Rivers, S. E., White, M., & Salovey, P. (2012). Classroom emotional climate, student engagement, and academic achievement. Journal of Educational Psychology, 104 (3), 700–712. https://doi.org/10.1037/a0027268 .
Richardson, J., & Lenarcic, J. (2008). Text Messaging as a Catalyst for Mobile Student Administration: The “Trigger” Experience. International Journal of Emerging Technologies & Society, 6 (2), 140–155.
Roblyer, M. D., McDaniel, M., Webb, M., Herman, J., & Witty, J. V. (2010). Findings on Facebook in higher education: A comparison of college faculty and student uses and perceptions of social networking sites. The Internet and Higher Education, 13 (3), 134–140.
Rock, M. L., & Thead, B. K. (2007). The effects of fading a strategic self-monitoring intervention on students’ academic engagement, accuracy, and productivity. Journal of Behavioral Education, 16 (4), 389–412. https://doi.org/10.1007/s10864-007-9049-7 .
Rodriguez, J. E. (2011). Social media use in higher education : Key areas to consider for educators. MERLOT Journal of Online Learning and Teaching, 7 (4), 539–550 https://doi.org/ISSN1558-9528 .
Rutherford, C. (2010). Using online social media to support Preservice student engagement. MERLOT Journal of Online Learning and Teaching, 6 (4), 703–711 Retrieved from http://jolt.merlot.org/vol6no4/rutherford_1210.pdf .
Schumacker, R. E., & Lomax, R. G. (2010). A Beginner’s Guide to structural equation modeling (3rd ed.). New York: Taylor & Francis Group.
Selwyn, N. (2012). Making sense of young people, education and digital technology: The role of sociological theory. Oxford Review of Education, 38 (1), 81–96.
Shih, Y. E. (2007). Setting the new standard with mobile computing in online learning. The International Review of Research in Open and Distributed Learning, 8 (2), 1–16.
Skinner, E. A., & Belmont, M. J. (1993). Motivation in the classroom: Reciprocal effects of teacher behavior and student engagement across the school year. Journal of educational psychology, 85 (4), 571.
Tabachnick, B. G., Fidell, L. S., & Ullman, J. B. (2007). Using multivariate statistics (Vol. 5). Boston: Pearson.
Voorn, R. J., & Kommers, P. A. (2013). Social media and higher education: Introversion and collaborative learning from the student’s perspective. International Journal of Social Media and Interactive Learning Environments, 1 (1), 59–73.
Wankel, C. (2009). Management education using social media. Organization Management Journal, 6 (4), 251–262.
Williams, M. D., Rana, N. P., & Dwivedi, Y. K. (2015). The unified theory of acceptance and use of technology (UTAUT): a literature review. Journal of Enterprise Information Management, 28 (3), 443–488.
Zhu, C. (2012). Student satisfaction, performance, and knowledge construction in online collaborative learning. Journal of Educational Technology & Society, 15 (1), 127–136.
Download references
Acknowledgements
We want to express our special gratitude to the Almighty who has blessed us with such hidden talent to give the shape of this research paper.
The authors of this manuscript, solemnly declared that no funding agency was supported to execute this research project.
Author information
Authors and affiliations.
Department of Commerce, Aligarh Muslim University, Aligarh, 202002, India
Jamal Abdul Nasir Ansari & Nawab Ali Khan
You can also search for this author in PubMed Google Scholar
Contributions
Jamal Abdul Nasir Ansari: The first author of this manuscript has performed all sorts of necessary works like the collection of data from respondents, administration of the questionnaire. Collection of information from the respondents was quite challenging. The author faced a lot of difficulties while collecting data. The main contribution of the author in this manuscript is that the entire work, like data analysis and its interpretation performed by him. Additionally, the author has tried to explore and usefulness of social media and its applicability in transferring the course contents. Nawab Ali Khan: The second author of this manuscript has checked all types of grammatical issues, and necessary corrections wherever required. The author(s) read and approved the final manuscript.
Corresponding author
Correspondence to Jamal Abdul Nasir Ansari .
Ethics declarations
Competing interests.
The authors declare that they have no competing interests.
Additional information
Publisher’s note.
Springer Nature remains neutral with regard to jurisdictional claims in published maps and institutional affiliations.
Rights and permissions
Open Access This article is distributed under the terms of the Creative Commons Attribution 4.0 International License ( http://creativecommons.org/licenses/by/4.0/ ), which permits unrestricted use, distribution, and reproduction in any medium, provided you give appropriate credit to the original author(s) and the source, provide a link to the Creative Commons license, and indicate if changes were made.
Reprints and permissions
About this article
Cite this article.
Ansari, J.A.N., Khan, N.A. Exploring the role of social media in collaborative learning the new domain of learning. Smart Learn. Environ. 7 , 9 (2020). https://doi.org/10.1186/s40561-020-00118-7
Download citation
Received : 27 November 2019
Accepted : 18 February 2020
Published : 16 March 2020
DOI : https://doi.org/10.1186/s40561-020-00118-7
Share this article
Anyone you share the following link with will be able to read this content:
Sorry, a shareable link is not currently available for this article.
Provided by the Springer Nature SharedIt content-sharing initiative
- Social media
- Higher education
- Faculty members
Subscribe to the PwC Newsletter
Join the community, edit social preview.

Add a new code entry for this paper
Remove a code repository from this paper, mark the official implementation from paper authors, add a new evaluation result row, remove a task, add a method.
- LABEL SMOOTHING
Remove a method
- LABEL SMOOTHING -
Edit Datasets
Enhancing suicide risk detection on social media through semi-supervised deep label smoothing.
9 May 2024 · Matthew Squires , Xiaohui Tao , Soman Elangovan , U Rajendra Acharya , Raj Gururajan , Haoran Xie , Xujuan Zhou · Edit social preview
Suicide is a prominent issue in society. Unfortunately, many people at risk for suicide do not receive the support required. Barriers to people receiving support include social stigma and lack of access to mental health care. With the popularity of social media, people have turned to online forums, such as Reddit to express their feelings and seek support. This provides the opportunity to support people with the aid of artificial intelligence. Social media posts can be classified, using text classification, to help connect people with professional help. However, these systems fail to account for the inherent uncertainty in classifying mental health conditions. Unlike other areas of healthcare, mental health conditions have no objective measurements of disease often relying on expert opinion. Thus when formulating deep learning problems involving mental health, using hard, binary labels does not accurately represent the true nature of the data. In these settings, where human experts may disagree, fuzzy or soft labels may be more appropriate. The current work introduces a novel label smoothing method which we use to capture any uncertainty within the data. We test our approach on a five-label multi-class classification problem. We show, our semi-supervised deep label smoothing method improves classification accuracy above the existing state of the art. Where existing research reports an accuracy of 43\% on the Reddit C-SSRS dataset, using empirical experiments to evaluate our novel label smoothing method, we improve upon this existing benchmark to 52\%. These improvements in model performance have the potential to better support those experiencing mental distress. Future work should explore the use of probabilistic methods in both natural language processing and quantifying contributions of both epistemic and aleatoric uncertainty in noisy datasets.
Code Edit Add Remove Mark official
Tasks edit add remove, datasets edit, results from the paper edit, methods edit add remove.
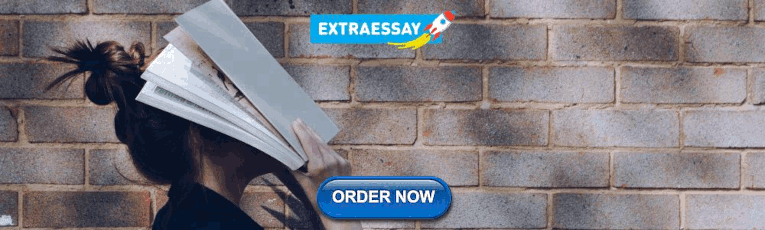
COMMENTS
PDF | Social media is the collective of online communications channels dedicated to community-based input, interaction, content-sharing and... | Find, read and cite all the research you need on ...
The growth in social media use has given rise to concerns about the impacts it may have on users' psychological well-being. This paper's main objective is to shed light on the effect of social media use on psychological well-being. Building on contributions from various fields in the literature, it provides a more comprehensive study of the ...
Abstract. Social media are responsible for aggravating mental health problems. This systematic study summarizes the effects of social network usage on mental health. Fifty papers were shortlisted from google scholar databases, and after the application of various inclusion and exclusion criteria, 16 papers were chosen and all papers were ...
Social media comprises communication websites that facilitate relationship forming between users from diverse backgrounds, resulting in a rich social structure. User generated content encourages inquiry and decision-making. Given the relevance of social media to various stakeholders, it has received significant attention from researchers of various fields, including information systems. There ...
The purpose of this study is to understand the role of social media content on users' engagement behavior. More specifically, we investigate: (i)the direct effects of format and platform on users' passive and active engagement behavior, and (ii) we assess the moderating effect of content context on the link between each content type (rational, emotional, and transactional content) and ...
Introduction. The use of social media has grown substantially in recent years (Leong et al., 2019; Kemp, 2020).Social media refers to "the websites and online tools that facilitate interactions between users by providing them opportunities to share information, opinions, and interest" (Swar and Hameed, 2017, p. 141).Individuals use social media for many reasons, including entertainment ...
Introduction. The term "social media" (SM) was first used in 1994 on a Tokyo online media environment, called Matisse. 1 It was in these early days of the commercial Internet that the first SM platforms were developed and launched. Over time, both the number of SM platforms and the number of active SM users have increased significantly, making it one of the most important applications of ...
However, evidence was found that the association of passive (but not active) social media use with well-being differed from adolescent to adolescent (Model 1B), with effect sizes ranging from − ...
In a review of 43 studies in young people, many benefits of social media were cited, including increased self-esteem and opportunities for self-disclosure (Best et al. 2014). Yet, reported negative effects were an increased exposure to harm, social isolation, depres-sive symptoms, and bullying (Best et al. 2014).
In this article, the authors present the results from a structured review of the literature, identifying and analyzing the most quoted and dominant definitions of social media (SM) and alternative terms that were used between 1994 and 2019 to identify their major applications. Similarities and differences in the definitions are highlighted to provide guidelines for researchers and managers who ...
Kaplan and Haenlein (2010) defined social media as "… a group of Internet-based applications that build on the ideological and technological foundations of Web 2.0, and that allow the creation and exchange of User Generated Content" (p. 61). The emergence of social media technologies has been embraced by a growing number of users who post text messages, pictures, and videos online ...
The spread of social media platforms enhanced academic and professional debate on social media engagement that attempted to better understand its theoretical foundations and measurements. This paper aims to systematically contribute to this academic debate by analysing, discussing, and synthesising social media engagement literature in the perspective of social media metrics. Adopting a ...
Access to personal devices, the Internet, and social media platforms among adolescents is increasing, to the point of being ubiquitous in some nations (Kwan et al., 2020).Social media is a central means by which adolescents interact, and therefore, an increased proportion of adolescents' psychosocial development takes place online (O'Keeffe & Clarke-Pearson, 2011).
For many young adults, accessing social media has become a normal part of their daily lives (Park and Lee, 2014).As of 2015, 90% of young adults regularly used social media sites such as Facebook, Instagram, and Twitter (Perrin, 2015).Researchers estimate that university students spend about 8-10 hours per day browsing, liking posts, and posting on social media sites ().
Social media has been defined as web-based communication platforms with three distinct features, in which the platform 1) allows users to create unique profiles and content to share with other users, 2) creates a visible network connection between users that can be navigated by other users, and 3) provides users with a space to broadcast ...
Social media. The term 'social media' refers to the various internet-based networks that enable users to interact with others, verbally and visually (Carr & Hayes, Citation 2015).According to the Pew Research Centre (Citation 2015), at least 92% of teenagers are active on social media.Lenhart, Smith, Anderson, Duggan, and Perrin (Citation 2015) identified the 13-17 age group as ...
Social media plays an important part in the digital transformation of businesses. This research provides a comprehensive analysis of the use of social media by business-to-business (B2B) companies. The current study focuses on the number of aspects of social media such as the effect of social media, social media tools, social media use, adoption of social media use and its barriers, social ...
Research about social media has highlighted its potential value to raise awareness, provide public education, and highlight unmet needs of patients and their families; however, there are also major concerns with representativeness and quality of online content. More research is needed into the downstream impact of social media in oncology, as ...
1 INTRODUCTION. The exponential growth of social media during the last decade has drastically changed the dynamics of firm-customer interactions and transformed the marketing environment in many profound ways.1 For example, marketing communications are shifting from one to many to one to one, as customers are changing from being passive observers to being proactive collaborators, enabled by ...
1.Introduction. Social competence refers to the ability to engage in meaningful interactions with others (Junge et al., 2020).To successfully accomplish this, children and adolescents must have a range of social skills —the building blocks of social competence (Junge et al., 2020; Rose-Krasnor, 1997).Throughout childhood and adolescence, social skills develop in interaction with the social ...
2.1. The identification of research questions. Sentiment analysis techniques have been shown to enable individuals, organizations and governments to benefit from the wealth of meaningful information contained in the unstructured data of social media, and there has been a great deal of research devoted to the design of high-performance sentiment classifiers and their applications [1], [4], [5 ...
This study is an attempt to examine the application and usefulness of social media and mobile devices in transferring the resources and interaction with academicians in higher education institutions across the boundary wall, a hitherto unexplained area of research. This empirical study is based on the survey of 360 students of a university in eastern India, cognising students' perception on ...
Social media use confers many benefits by providing access to a wide range of information sources, which facilitate learning (Greenhow and Robelia, 2009). However, instead of using social networking sites for academic purposes, students tend to be actively involved in online shopping, gaming, and entertainment during the day and at night.
Abstract. Social media platforms are popular venues for sharing personal experiences, seeking information, and offering peer-to-peer support among individuals living with mental illness. With significant shortfalls in the availability, quality, and reach of evidence-based mental health services across the United States and globally, social ...
Social media is very helpful in educational fields, and it has some flaws too. As due to excessive media usage people are have weaker relationships with their loved ones. Individuals are facing several mental and physical issues. Results of the study can also be helpful for creating new strategies to limit social media use in daily life.
A 2018 Common Sense Media report found that 81 percent of teens use social media, and more than a third report using social media sites multiple times an hour. These statistics have risen dramatically over the past six years, likely driven by increased access to mobile devices. Rising along with these stats is a growing interest in the impact ...
With the popularity of social media, people have turned to online forums, such as Reddit to express their feelings and seek support. This provides the opportunity to support people with the aid of artificial intelligence. Social media posts can be classified, using text classification, to help connect people with professional help.
Kaplan and Haenlein (2010) de ne social media as "a group of Internet-based. applications that build on the ideological and technological foundations of Web. 2.0, and that allow the creation and ...
In recent years, several studies have been conducted to explore the potential effects of social media on students' affective traits, such as stress, anxiety, depression, and so on. The present paper reviews the findings of the exemplary published works of research to shed light on the positive and negative potential effects of the massive use ...
Students earlier claimed on social media, especially Facebook groups, that the "AS Level Mathematics Paper" was leaked online just before they were scheduled to take the exam on April 2.