MINI REVIEW article
Solving the credit assignment problem with the prefrontal cortex.
- Department of Psychology, University of California, Los Angeles, Los Angeles, CA, United States
In naturalistic multi-cue and multi-step learning tasks, where outcomes of behavior are delayed in time, discovering which choices are responsible for rewards can present a challenge, known as the credit assignment problem . In this review, I summarize recent work that highlighted a critical role for the prefrontal cortex (PFC) in assigning credit where it is due in tasks where only a few of the multitude of cues or choices are relevant to the final outcome of behavior. Collectively, these investigations have provided compelling support for specialized roles of the orbitofrontal (OFC), anterior cingulate (ACC), and dorsolateral prefrontal (dlPFC) cortices in contingent learning. However, recent work has similarly revealed shared contributions and emphasized rich and heterogeneous response properties of neurons in these brain regions. Such functional overlap is not surprising given the complexity of reciprocal projections spanning the PFC. In the concluding section, I overview the evidence suggesting that the OFC, ACC and dlPFC communicate extensively, sharing the information about presented options, executed decisions and received rewards, which enables them to assign credit for outcomes to choices on which they are contingent. This account suggests that lesion or inactivation/inhibition experiments targeting a localized PFC subregion will be insufficient to gain a fine-grained understanding of credit assignment during learning and instead poses refined questions for future research, shifting the focus from focal manipulations to experimental techniques targeting cortico-cortical projections.
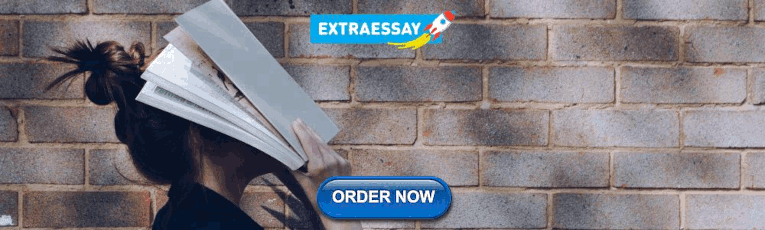
Introduction
When an animal is introduced to an unfamiliar environment, it will explore the surroundings randomly until an unexpected reward is encountered. Reinforced by this experience, the animal will gradually learn to repeat those actions that produced the desired outcome. The work conducted in the past several decades has contributed a detailed understanding of the psychological and neural mechanisms that support such reinforcement-driven learning ( Schultz and Dickinson, 2000 ; Schultz, 2004 ; Niv, 2009 ). It is now broadly accepted that dopamine (DA) signaling conveys prediction errors, or the degree of surprise brought about by unexpected rewards, and interacts with cortical and basal ganglia circuits to selectively reinforce the advantageous choices ( Schultz, 1998a , b ; Schultz and Dickinson, 2000 ; Niv, 2009 ). Yet, in naturalistic settings, where rewards are delayed in time, and where multiple cues are encountered, or where several decisions are made before the outcomes of behavior are revealed, discovering which choices are responsible for rewards can present a challenge, known as the credit assignment problem ( Mackintosh, 1975 ; Rothkopf and Ballard, 2010 ).
In most everyday situations, the rewards are not immediate consequences of behavior, but instead appear after substantial delays. To influence future choices, the teaching signal conveyed by DA release needs to reinforce synaptic events occurring on a millisecond timescale, frequently seconds before the outcomes of decisions are revealed ( Izhikevich, 2007 ; Fisher et al., 2017 ). This apparent difficulty in linking preceding behaviors caused by transient neuronal activity to a delayed feedback has been termed the distal reward or temporal credit assignment problem ( Hull, 1943 ; Barto et al., 1983 ; Sutton and Barto, 1998 ; Dayan and Abbott, 2001 ; Wörgötter and Porr, 2005 ). Credit for the reward delayed by several seconds can frequently be assigned by establishing an eligibility trace, a molecular memory of the recent neuronal activity, allowing modification of synaptic connections that participated in the behavior ( Pan et al., 2005 ; Fisher et al., 2017 ). On longer timescales, or when multiple actions need to be performed sequentially to reach a final goal, intermediate steps themselves can acquire motivational significance and subsequently reinforce preceding decisions, such as in temporal-difference (TD) learning models ( Sutton and Barto, 1998 ).
Several excellent reviews have summarized the accumulated knowledge on mechanisms that link choices and their outcomes through time, highlighting the advantages of eligibility traces and TD models ( Wörgötter and Porr, 2005 ; Barto, 2007 ; Niv, 2009 ; Walsh and Anderson, 2014 ). Yet these solutions to the distal reward problem can impede learning in multi-choice tasks, or when an animal is presented with many irrelevant stimuli prior to or during the delay. Here, I only briefly overview the work on the distal reward problem to highlight potential complications that can arise in credit assignment based on eligibility traces when learning in multi-cue environments. Instead, I focus on the structural (or spatial ) credit assignment problem, requiring animals to select and learn about the most meaningful features in the environment and ignore irrelevant distractors. Collectively, the reviewed evidence highlights a critical role for the prefrontal cortex (PFC) in such contingent learning.
Recent studies have provided compelling support for specialized functions of the orbitofrontal (OFC) and dorsolateral prefrontal (dlPFC) cortices in credit assignment in multi-cue tasks, with fewer experiments targeting the anterior cingulate cortex (ACC). For example, it has seen suggested that the dlPFC aids reinforcement-driven learning by directing attention to task-relevant cues ( Niv et al., 2015 ), the OFC assigns credit for rewards based on the causal relationship between trial outcomes and choices ( Jocham et al., 2016 ; Noonan et al., 2017 ), whereas the ACC contributes to unlearning of action-outcome associations when the rewards are available for free ( Jackson et al., 2016 ). However, this work has similarly revealed shared contributions and emphasized rich and heterogeneous response properties of neurons in the PFC, with different subregions monitoring and integrating the information about the task (i.e., current context, available options, anticipated rewards, as well as delay and effort costs) at variable times within a trial (upon stimulus presentation, action selection, outcome anticipation, and feedback monitoring; ex., Hunt et al., 2015 ; Khamassi et al., 2015 ). In the concluding section, I overview the evidence suggesting that contingent learning in multi-cue environments relies on dynamic cortico-cortical interactions during decision making and outcome valuation.
Solving the Temporal Credit Assignment Problem
When outcomes follow choices after short delays (Figure 1A ), the credit for distal rewards can frequently be assigned by establishing an eligibility trace, a sustained memory of the recent activity that renders synaptic connections malleable to modification over several seconds. Eligibility traces can persist as elevated levels of calcium in dendritic spines of post-synaptic neurons ( Kötter and Wickens, 1995 ) or as a sustained neuronal activity throughout the delay period ( Curtis and Lee, 2010 ) to allow for synaptic changes in response to reward signals. Furthermore, spike-timing dependent plasticity can be influenced by neuromodulator input ( Izhikevich, 2007 ; Abraham, 2008 ; Fisher et al., 2017 ). For example, the magnitude of short-term plasticity can be modulated by DA, acetylcholine and noradrenaline, which may even revert the sign of the synaptic change ( Matsuda et al., 2006 ; Izhikevich, 2007 ; Seol et al., 2007 ; Abraham, 2008 ; Zhang et al., 2009 ). Sustained neural activity has been observed in the PFC and striatum ( Jog et al., 1999 ; Pasupathy and Miller, 2005 ; Histed et al., 2009 ; Kim et al., 2009 , 2013 ; Seo et al., 2012 ; Her et al., 2016 ), as well as the sensory cortices after experience with consistent pairings between the stimuli and outcomes separated by predictable delays ( Shuler and Bear, 2006 ).
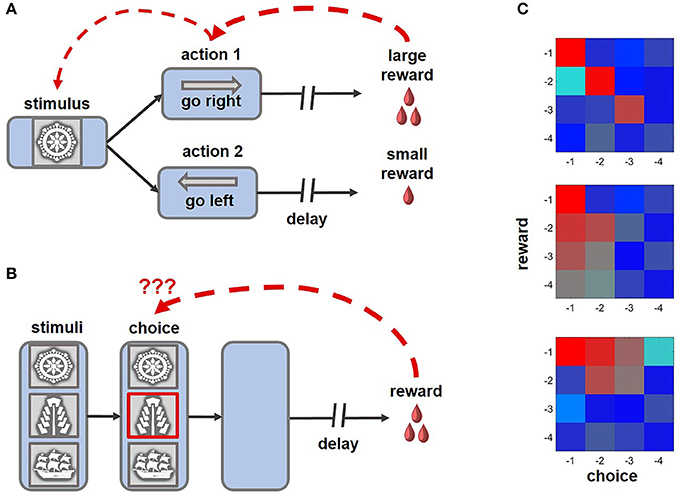
Figure 1 . Example tasks highlighting the challenge of credit assignment and learning strategies enabling animals to solve this problem. (A) An example of a distal reward task that can be successfully learned with eligibility traces and TD rules, where intermediate choices can acquire motivational significance and subsequently reinforce preceding decisions (ex., Pasupathy and Miller, 2005 ; Histed et al., 2009 ). (B) In this version of the task, multiple cues are present at the time of choice, only one of which is meaningful for obtaining rewards. After a brief presentation, the stimuli disappear, requiring an animal to solve a complex structural and temporal credit assignment problem (ex., Noonan et al., 2010 , 2017 ; Niv et al., 2015 ; Asaad et al., 2017 ; while the schematic of the task captures the challenge of credit assignment, note that in some experimental variants of the behavioral paradigm stimuli disappeared before an animal revealed its choice, whereas in others the cues remained on the screen until the trial outcome was revealed). Under such conditions, learning based on eligibility traces is suboptimal, as non-specific reward signals can reinforce visual cues that did not meaningfully contribute, but occurred close, to beneficial outcomes of behavior. (C) On reward tasks, similar to the one shown in (B) , the impact of previous decisions and associated rewards on current behavior can be assessed by performing regression analyses ( Jocham et al., 2016 ; Noonan et al., 2017 ). Here, the color of each cell in a matrix represents the magnitude of the effect of short-term choice and outcome histories, up to 4 trials into the past (red-strong influence; blue-weak influence on the current decision). Top: an animal learning based on the causal relationship between outcomes and choices (i.e., contingent learning). Middle: each choice is reinforced by a combined history of rewards (i.e., decisions are repeated if beneficial outcomes occur frequently). Bottom: the influence of recent rewards spreads to unrelated choices.
On extended timescales, when multiple actions need to be performed sequentially to reach a final goal, the distal reward problem can be solved by assigning motivational significance to intermediate choices that can subsequently reinforce preceding decisions, such as in TD learning models ( Montague et al., 1996 ; Sutton and Barto, 1998 ; Barto, 2007 ). Assigning values to these intervening steps according to expected future rewards allows to break complex temporal credit assignment problems into smaller and easier tasks. There is ample evidence for TD learning in humans and other animals that on the neural level is supported by transfer of DA responses from the time of reward delivery to preceding cues and actions ( Montague et al., 1996 ; Schultz, 1998a , b ; Walsh and Anderson, 2014 ).
Both TD learning and eligibility traces offer elegant solutions to the distal reward problem, and models based on cooperation between these two mechanisms can predict animal behavior as well as neuronal responses to rewards and predictive stimuli ( Pan et al., 2005 ; Bogacz et al., 2007 ). Yet assigning credit based on eligibility traces can be suboptimal when an animal interacts with many irrelevant stimuli prior to or during the delay (Figure 1B ). Under such conditions sensory areas remain responsive to distracting stimuli and the arrival of non-specific reward signals can reinforce intervening cues that did not meaningfully contribute, but occurred close, to the outcome of behavior ( FitzGerald et al., 2013 ; Xu, 2017 ).
The Role of the PFC in Structural Credit Assignment
Several recent studies have investigated the neural mechanisms of appropriate credit assignment in challenging tasks where only a few of the multitude of cues predict rewards reliably. Collectively, this work has provided compelling support for causal contributions of the PFC to structural credit assignment. For example, Asaad et al. (2017) examined the activity of neurons in monkey dlPFC while subjects were performing a delayed learning task. The arrangement of the stimuli varied randomly between trials and within each block either the spatial location or stimulus identity was relevant for solving the task. The monkeys' goal was to learn by trial-and-error to select one of the four options that led to rewards according to current rules. When stimulus identity was relevant for solving the task, neural activity in the dlPFC at the time of feedback reflected both the relevant cue (regardless of its spatial location) and the trial outcome, thus integrating the information necessary for credit assignment. Such responses were strategy-selective: these neurons did not encode cue identity at the time of feedback when it was not necessary for learning in the spatial location task, in which making a saccade to the same position on the screen was reinforced within a block of trials. Previous research has similarly indicated that neurons in the dlPFC respond selectively to behaviorally-relevant and attended stimuli ( Lebedev et al., 2004 ; Markowitz et al., 2015 ) and integrate information about prediction errors, choice values as well as outcome uncertainty prior to trial feedback ( Khamassi et al., 2015 ).
The activity within the dlPFC has been linked to structural credit assignment through selective attention and representational learning ( Niv et al., 2015 ). Under conditions of reward uncertainty and unknown relevant task features, human participants opt for computational efficiency and engage in a serial-hypothesis-testing strategy ( Wilson and Niv, 2011 ), selecting one cue and its anticipated outcome as the main focus of their behavior, and updating the expectations associated exclusively with that choice upon feedback receipt ( Akaishi et al., 2016 ). Niv and colleagues tested participant on a three-armed bandit task, where relevant stimulus dimensions (i.e., shape, color or texture) predicting outcome probabilities changed between block of trials ( Niv et al., 2015 ). In such multidimensional environment, reinforcement-driven learning was aided by attentional control mechanisms that engaged the dlPFC, intraparietal cortex, and precuneus.
In many tasks, the credit for outcomes can be assigned according to different rules: based on the causal relationship between rewards and choices (i.e., contingent learning), their temporal proximity (i.e., when the reward is received shortly after a response), or their statistical relationship (when an action has been executed frequently before beneficial outcomes; Jocham et al., 2016 ; Figure 1C ). The analyses presented in papers discussed above did not allow for the dissociation between these alternative strategies of credit assignment. By testing human participants on a task with continuous stimulus presentation, instead of a typical trial-by-trial structure, Jocham et al. (2016) demonstrated that the tendency to repeat choices that were immediately followed by rewards and causal learning operate in parallel. In this experiment, activity within another subregion of the PFC, the OFC, was associated with contingent learning. Complementary work in monkeys revealed that the OFC contributes causally to credit assignment ( Noonan et al., 2010 ): animals with OFC lesions were unable to associate a reward with the choice on which it was contingent and instead relied on temporal and statistical learning rules. In another recent paper, Noonan and colleagues (2017) extended these observations to humans, demonstrating causal contributions of the OFC to credit assignment across species. The participants were tested on a three-choice probabilistic learning task. The three options were presented simultaneously and maintained on the screen until the outcome of a decision was revealed, thus requiring participants to ignore irrelevant distractors. Notably, only patients with lateral OFC lesions displayed any difficulty in learning the task, whereas damage to the medial OFC or dorsomedial PFC preserved contingent learning mechanisms. However, it is presently unknown whether lesions to the dlPFC or ACC affect such causal learning.
In another test of credit assignment in learning, contingency degradation, the subjects are required to track causal relationships between the stimuli or actions and rewards. During contingency degradation sessions, the animals are still reinforced for responses, but rewards are also available for free. After experiencing non-contingent rewards, control subjects reliably decrease their choices of the stimuli. However, lesions to both the ACC and OFC inhibit contingency degradation ( Jackson et al., 2016 ). Taken together, these observations demonstrate causal contributions of the PFC to appropriate credit assignment in multi-cue environments.
Cooperation Between PFC Subregions Supports Contingent Learning in Multi-Cue Tasks
Despite the segregation of temporal and structural aspects of credit assignment in earlier sections of this review, in naturalistic settings the brains frequently need to tackle both problems simultaneously. Here, I overview the evidence favoring a network perspective, suggesting that dynamic cortico-cortical interactions during decision making and outcome valuation enable adaptive solutions to complex spatio-temporal credit assignment problems. It has been previously suggested that feedback projections from cortical areas occupying higher levels of processing hierarchy, including the PFC, can aid in attribution of outcomes to individual decisions by implementing attention-gated reinforcement learning ( Roelfsema and van Ooyen, 2005 ). Similarly, recent theoretical work has shown that even complex multi-cue and multi-step problems can be solved by an extended cascade model of synaptic memory traces, in which the plasticity is modulated not only by the activity within a population of neurons, but also by feedback about executed decisions and resulting rewards ( Urbanczik and Senn, 2009 ; Friedrich et al., 2010 , 2011 ). Contingent learning, according to these models, can be supported by the communication between neurons encoding available options, committed choices and outcomes of behavior during decision making and feedback monitoring. For example, at the time of outcome valuation, information about recent choices can be maintained as a memory trace in the neuronal population involved in action selection or conveyed by an efference copy from an interconnected brain region ( Curtis and Lee, 2010 ; Khamassi et al., 2011 , 2015 ). Similarly, reinforcement feedback is likely communicated as a global reward signal (ex., DA release) as well as projections from neural populations engaged in performance monitoring, such as those within the ACC ( Friedrich et al., 2010 ; Khamassi et al., 2011 ). The complexity of reciprocal and recurrent projections spanning the PFC ( Barbas and Pandya, 1989 ; Felleman and Van Essen, 1991 ; Elston, 2000 ) may enable this network to implement such learning rules, integrating the information about the task, executed decisions and performance feedback.
In many everyday decisions, the options are compared across multiple features simultaneously (ex., by considering current context, needs, available reward types, as well as delay and effort costs). Neurons in different subregions of the PFC exhibit rich response properties, signaling these features of the task at various time epochs within a trial. For example, reward selectivity in response to predictive stimuli emerges earlier in the OFC and may then be passed to the dlPFC that encodes both the expected outcome and the upcoming choice ( Wallis and Miller, 2003 ). Similarly, on trials where options are compared based on delays to rewards, choices are dependent on interactions between the OFC and dlPFC ( Hunt et al., 2015 ). Conversely, when effort costs are more meaningful for decisions, it is the ACC that influences choice-related activity in the dlPFC ( Hunt et al., 2015 ). The OFC is required not only for the evaluation of stimuli, but also more complex abstract rules, based on rewards they predict ( Buckley et al., 2009 ). While both the OFC and dlPFC encode abstract strategies (ex., persisting with recent choices or shifting to a new response), such signals appear earlier in the OFC and may be subsequently conveyed to the dlPFC where they are combined with upcoming response (i.e., left vs. right saccade) encoding ( Tsujimoto et al., 2011 ). Therefore, the OFC may be the first PFC subregion to encode task rules and/or potential rewards predicted by sensory cues; via cortico-cortical projections, this information may be subsequently communicated to the dlPFC or ACC ( Kennerley et al., 2009 ; Hayden and Platt, 2010 ) to drive strategy-sensitive response planning.
The behavioral strategy that the animal follows is influenced by recent reward history ( Cohen et al., 2007 ; Pearson et al., 2009 ). If its choices are reinforced frequently, the animal will make similar decisions in the future (i.e., exploit its current knowledge). Conversely, unexpected omission of expected rewards can signal a need for novel behaviors (i.e., exploration). Neurons in the dlPFC carry representations of planned as well as previous choices, anticipate outcomes, and jointly encode the current decisions and their consequences following feedback ( Seo and Lee, 2007 ; Seo et al., 2007 ; Tsujimoto et al., 2009 ; Asaad et al., 2017 ). Similarly, the ACC tracks trial-by-trial outcomes of decisions ( Procyk et al., 2000 ; Shidara and Richmond, 2002 ; Amiez et al., 2006 ; Quilodran et al., 2008 ) as well as reward and choice history ( Seo and Lee, 2007 ; Kennerley et al., 2009 , 2011 ; Sul et al., 2010 ; Kawai et al., 2015 ) and signals errors in outcome prediction ( Kennerley et al., 2009 , 2011 ; Hayden et al., 2011 ; Monosov, 2017 ). At the time of feedback, neurons in the OFC encode committed choices, their values and contingent rewards ( Tsujimoto et al., 2009 ; Sul et al., 2010 ). Notably, while the OFC encodes the identity of expected outcomes and the value of the chosen option after the alternatives are presented to an animal, it does not appear to encode upcoming decisions ( Tremblay and Schultz, 1999 ; Wallis and Miller, 2003 ; Padoa-Schioppa and Assad, 2006 ; Sul et al., 2010 ; McDannald et al., 2014 ), therefore it might be that feedback projections from the dlPFC or ACC are required for such activity to emerge at the time of reward feedback.
To capture the interactions between PFC subregions in reinforcement-driven learning, Khamassi and colleagues have formulated a computation model in which action values are stored and updated in the ACC and then communicated to the dlPFC that decides which action to trigger ( Khamassi et al., 2011 , 2013 ). This model relies on meta-learning principles ( Doya, 2002 ), flexibly adjusting the exploration-exploitation parameter based on performance history and variability in the environment that are monitored by the ACC. The explore-exploit parameter then influences action-selection mechanisms in the dlPFC, prioritizing choice repetition once the rewarded actions are discovered and encouraging switching between different options when environmental conditions change. In addition to highlighting the dynamic interactions between the dlPFC and ACC in learning, the model similarly offers an elegant solution to the credit assignment problem by restricting value updating only to those actions that were selected on a given trial. This is implemented by requiring the prediction error signals in the ACC to coincide with a motor efference copy sent by the premotor cortex. The model is endorsed with an ability to learn meta-values of novel objects in the environment based on the changes in the average reward that follow the presentation of such stimuli. While the authors proposed that such meta-value learning is implemented by the ACC, it is plausible that the OFC also plays a role in this process based on its contributions to stimulus-outcome and state learning ( Wilson et al., 2014 ; Zsuga et al., 2016 ). Intriguingly, this model could reproduce monkey behavior and neural responses on two tasks: four-choice deterministic and two-choice probabilistic paradigms, entailing a complex spatio-temporal credit assignment problem as the stimuli disappeared from the screen prior to action execution and outcome presentation ( Khamassi et al., 2011 , 2013 , 2015 ). Model-based analyses of neuronal responses further revealed that information about prediction errors, action values and outcome uncertainty is integrated both in the dlPFC and ACC, but at different timepoints: before trial feedback in the dlPFC and after feedback in the ACC ( Khamassi et al., 2015 ).
Collectively, these findings highlight the heterogeneity of responses in each PFC subregion that differ in temporal dynamics within a single trial and suggest that the cooperation between the OFC, ACC and dlPFC may support flexible, strategy- and context-dependent choices. This network perspective further suggests that individual PFC subregions may be less specialized in their functions than previously thought. For example, in primates both the ACC and dlPFC participate in decisions based on action values ( Hunt et al., 2015 ; Khamassi et al., 2015 ). And more recently, it has been demonstrated that the OFC is involved in updating action-outcome values as well ( Fiuzat et al., 2017 ). Analogously, while it has been proposed that the OFC is specialized for stimulus-outcome and ACC for action-outcome learning ( Rudebeck et al., 2008 ), lesions to the ACC have been similarly reported to impair stimulus-based reversal learning ( Chudasama et al., 2013 ), supporting shared contributions of the PFC subregions to adaptive behavior. Indeed, these brain regions communicate extensively, sharing the information about presented options, executed decisions and received rewards (Figure 2 ), which can enable them to assign credit for outcomes to choices on which they are contingent ( Urbanczik and Senn, 2009 ; Friedrich et al., 2010 , 2011 ). Attention-gated learning likely relies on the cooperation between PFC subregions as well: for example, coordinated and synchronized activity between the ACC and dlPFC aids in goal-directed attentional shifting and prioritization of task-relevant information ( Womelsdorf et al., 2014 ; Oemisch et al., 2015 ; Voloh et al., 2015 ).
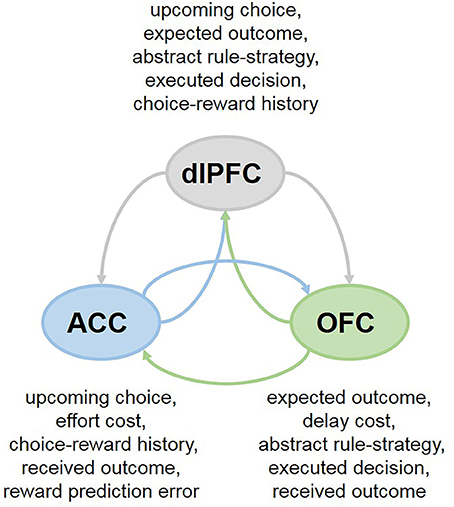
Figure 2 . Cooperation between PFC subregions in multi-cue tasks. In many everyday decisions, the options are compared across multiple features simultaneously (ex., by considering current context, needs, available reward types, as well as delay and effort costs). Neurons in different subregions of the PFC exhibit rich response properties, integrating many aspects of the task at hand. The OFC, ACC and dlPFC communicate extensively, sharing the information about presented options, executed decisions and received rewards, which can enable them to assign credit for outcomes to choices on which they are contingent.
Functional connectivity within the PFC can support contingent learning on shorter timescales (ex., across trials within the same task), when complex rules or stimulus-action-outcome mappings are switching frequently ( Duff et al., 2011 ; Johnson et al., 2016 ). Under such conditions, the same stimuli can carry different meaning depending on task context or due to changes in the environment (ex., serial discrimination-reversal problems) and the PFC neurons with heterogeneous response properties may be better targets for modification, allowing the brain to exert flexible, rapid and context-sensitive control over behavior ( Asaad et al., 1998 ; Mansouri et al., 2006 ). Indeed, it has been shown that rule and reversal learning induce plasticity in OFC synapses onto the dorsomedial PFC (encompassing the ACC) in rats ( Johnson et al., 2016 ). When motivational significance of reward-predicting cues fluctuates frequently, neuronal responses and synaptic connections within the PFC tend to update more rapidly (i.e., across block of trials) compared to subcortical structures and other cortical regions ( Padoa-Schioppa and Assad, 2008 ; Morrison et al., 2011 ; Xie and Padoa-Schioppa, 2016 ; Fernández-Lamo et al., 2017 ; Saez et al., 2017 ). Similarly, neurons in the PFC promptly adapt their responses to incoming information based on the recent history of inputs ( Freedman et al., 2001 ; Meyers et al., 2012 ; Stokes et al., 2013 ). Critically, changes in the PFC activity closely track behavioral performance ( Mulder et al., 2003 ; Durstewitz et al., 2010 ), and interfering with neural plasticity within this brain area prevents normal responses to contingency degradation ( Swanson et al., 2015 ).
When the circumstances are stable overall and the same cues or actions remain reliable predictors of rewards, long-range connections between the PFC, association and sensory areas can support contingent learning on prolonged timescales. Neurons in the lateral intraparietal area demonstrate larger post-decisional responses and enhanced learning following choices that predict final outcomes of sequential behavior in a multi-step and -cue task ( Gersch et al., 2014 ). Such changes in neuronal activity likely rely on information about task rules conveyed by the PFC directly or via interactions with neuromodulatory systems. These hypotheses could be tested in future work.
In summary, dynamic interactions between subregions of the PFC can support contingent learning in multi-cue environments. Furthermore, via feedback projections, the PFC can guide plasticity in other cortical areas associated with sensory and motor processing ( Cohen et al., 2011 ). This account suggests that lesion experiments targeting a localized PFC subregion will be insufficient to gain fine-grained understanding of credit assignment during learning and instead poses refined questions for future research, shifting the focus from focal manipulations to experimental techniques targeting cortico-cortical projections. To gain novel insights into functional connectivity between PFC subregions, it will be critical to assess neural correlates of contingent learning in the OFC, ACC, and dlPFC simultaneously in the context of the same task. In humans, functional connectivity can be assessed by utilizing coherence, phase synchronization, Granger causality and Bayes network approaches ( Bastos and Schoffelen, 2016 ; Mill et al., 2017 ). Indeed, previous studies have linked individual differences in cortico-striatal functional connectivity to reinforcement-driven learning ( Horga et al., 2015 ; Kaiser et al., 2017 ) and future work could focus on examining cortico-cortical interactions in similar paradigms. To probe causal contributions of projections spanning the PFC, future research may benefit from designing multi-cue tasks for rodents and taking advantage of recently developed techniques (i.e., chemo- and opto-genetic targeting of projection neurons followed by silencing of axonal terminals to achieve pathway-specific inhibition; Deisseroth, 2010 ; Sternson and Roth, 2014 ) that afford increasingly precise manipulations of cortico-cortical connectivity. It should be noted, however, that most experiments to date have probed the contributions of the PFC to credit assignment in primates, and functional specialization across different subregions may be even less pronounced in mice and rats. Finally, as highlighted throughout this review, the recent progress in understanding the neural mechanisms of credit assignment has relied on introduction of more complex tasks, including multi-cue and probabilistic choice paradigms. While such tasks better mimic the naturalistic problems that the brains have evolved to solve, they also produce behavioral patterns that are more difficult to analyze and interpret ( Scholl and Klein-Flügge, 2017 ). As such, computational modeling of the behavior and neuronal activity may prove especially useful in future work on credit assignment.
Author Contributions
The author confirms being the sole contributor of this work and approved it for publication.
This work was supported by UCLA's Division of Life Sciences Recruitment and Retention fund (Izquierdo), as well as the UCLA Distinguished University Fellowship (Stolyarova).
Conflict of Interest Statement
The author declares that the research was conducted in the absence of any commercial or financial relationships that could be construed as a potential conflict of interest.
Acknowledgments
The author thanks her mentor Dr. Alicia Izquierdo for helpful feedback and critiques on the manuscript, and Evan E. Hart, as well as the members of the Center for Brains, Minds and Machines and Lau lab for stimulating conversations on the topic.
Abraham, W. C. (2008). Metaplasticity: tuning synapses and networks for plasticity. Nat. Rev. Neurosci. 9:387 doi: 10.1038/nrn2356
PubMed Abstract | CrossRef Full Text | Google Scholar
Akaishi, R., Kolling, N., Brown, J. W., and Rushworth, M. (2016). Neural mechanisms of credit assignment in a multicue environment. J. Neurosci. 36, 1096–1112. doi: 10.1523/JNEUROSCI.3159-15.2016
Amiez, C., Joseph, J. P., and Procyk, E. (2006). Reward encoding in the monkey anterior cingulate cortex. Cereb. Cortex 16, 1040–1055. doi: 10.1093/cercor/bhj046
Asaad, W. F., Lauro, P. M., Perge, J. A., and Eskandar, E. N. (2017). Prefrontal neurons encode a solution to the credit assignment problem. J. Neurosci. 37, 6995–7007. doi: 10.1523/JNEUROSCI.3311-16.2017.
Asaad, W. F., Rainer, G., and Miller, E. K. (1998). Neural activity in the primate prefrontal cortex during associative learning. Neuron 21, 1399–1407. doi: 10.1016/S0896-6273(00)80658-3
Barbas, H., and Pandya, D. N. (1989). Architecture and intrinsic connections of the prefrontal cortex in the rhesus monkey. J. Comp. Neurol. 286, 353–375 doi: 10.1002/cne.902860306
Barto, A. G. (2007). Temporal difference learning. Scholarpedia J. 2:1604. doi: 10.4249/scholarpedia.1604
CrossRef Full Text | Google Scholar
Barto, A. G., Sutton, R. S., and Anderson, C. W. (1983). “Neuronlike adaptive elements that can solve difficult learning control problems,” in IEEE Transactions on Systems, Man, and Cybernetics, SMC-13 , 834–846
Google Scholar
Bastos, A. M., and Schoffelen, J. M. (2016). A tutorial review of functional connectivity analysis methods and their interpretational pitfalls. Front. Syst. Neurosci . 9:175. doi: 10.3389/fnsys.2015.00175
Bogacz, R., McClure, S. M., Li, J., Cohen, J. D., and Montague, P. R. (2007). Short-term memory traces for action bias in human reinforcement learning. Brain Res. 1153, 111–121. doi: 10.1016/j.brainres.2007.03.057
Buckley, M. J., Mansouri, F. A., Hoda, H., Mahboubi, M., Browning, P. G. F., Kwok, S. C., et al. (2009). Dissociable components of rule-guided behavior depend on distinct medial and prefrontal regions. Science 325, 52–58. doi: 10.1126/science.1172377
Chudasama, Y., Daniels, T. E., Gorrin, D. P., Rhodes, S. E., Rudebeck, P. H., and Murray, E. A. (2013). The role of the anterior cingulate cortex in choices based on reward value and reward contingency. Cereb Cortex 23, 2884–2898. doi: 10.1093/cercor/bhs266
Cohen, J. D., McClure, S. M., and Yu, A. J. (2007). Should I stay or should I go? How the human brain manages the trade-off between exploitation and exploration. Philos. Trans. R. Soc. Lond. Ser. B Biol. Sci. 362, 933–942. doi: 10.1098/rstb.2007.2098
Cohen, M. X., Wilmes, K., and Vijver, I. v. (2011). Cortical electrophysiological network dynamics of feedback learning. Trends Cogn. Sci. 15, 558–566. doi: 10.1016/j.tics.2011.10.004
Curtis, C. E., and Lee, D. (2010). Beyond working memory: the role of persistent activity in decision making. Trends Cogn. Sci. 14, 216–222. doi: 10.1016/j.tics.2010.03.006
Dayan, P., and Abbott, L. F. (2001). Theoretical Neuroscience: Computational and Mathematical Modeling of Neural Systems. Cambridge, MA: MIT Press.
Deisseroth, K. (2010). Optogenetics. Nat. Methods 8, 26–29. doi: 10.1038/nmeth.f.324
Doya, K. (2002). Metalearning and neuromodulation. Neural. Netw. 15, 495–506. doi: 10.1016/S0893-6080(02)00044-8
Duff, A., Sanchez Fibla, M., and Verschure, P. F. M. J. (2011). A biologically based model for the integration of sensory–motor contingencies in rules and plans: a prefrontal cortex based extension of the distributed adaptive control architecture. Brain Res. Bull. 85, 289–304. doi: 10.1016/j.brainresbull.2010.11.008
Durstewitz, D., Vittoz, N. M., Floresco, S. B., and Seamans, J. K. (2010). Abrupt transitions between prefrontal neural ensemble states accompany behavioral transitions during rule learning. Neuron 66, 438–448. doi: 10.1016/j.neuron.2010.03.029
Elston, G. N. (2000). Pyramidal cells of the frontal lobe: all the more spinous to think with. J. Neurosci. 20:RC95. Available online at: http://www.jneurosci.org/content/20/18/RC95.long
PubMed Abstract | Google Scholar
Felleman, D. J., and Van Essen, D. C. (1991). Distributed hierarchical processing in the primate cerebral cortex. Cereb. Cortex 1, 1–47. doi: 10.1093/cercor/1.1.1
Fernández-Lamo, I., Delgado-García, J. M., and Gruart, A. (2017). When and where learning is taking place: multisynaptic changes in strength during different behaviors related to the acquisition of an operant conditioning task by behaving rats. Cereb. Cortex 14, 1–13. doi: 10.1093/cercor/bhx011
CrossRef Full Text
Fisher, S. D., Robertson, P. B., Black, M. J., Redgrave, P., Sagar, M. A., Abraham, W. C., et al. (2017). Reinforcement determines the timing dependence of corticostriatal synaptic plasticity in vivo . Nat. Commun. 8:334. doi: 10.1038/s41467-017-00394-x
FitzGerald, T. H. B., Friston, K. J., and Dolan, R. J. (2013). Characterising reward outcome signals in sensory cortex. NeuroImage 83, 329–334. doi: 10.1016/j.neuroimage.2013.06.061
Fiuzat, E. C., Rhodes, S. E., and Murray, E. A. (2017). The role of orbitofrontal-amygdala interactions in updating action-outcome valuations in macaques. J. Neurosci. 37, 2463–2470. doi: 10.1523/JNEUROSCI.1839-16.2017
Freedman, D. J., Riesenhuber, M., Poggio, T., and Miller, E. K. (2001). Categorical representation of visual stimuli in the primate prefrontal cortex. Science 291, 312–316. doi: 10.1126/science.291.5502.312
Friedrich, J., Urbanczik, R., and Senn, W. (2010). Learning spike-based population codes by reward and population feedback. Neural. Comput. 22, 1698–1717. doi: 10.1162/neco.2010.05-09-1010
Friedrich, J., Urbanczik, R., and Senn, W. (2011). Spatio-temporal credit assignment in neuronal population learning. PLoS Comput. Biol. 7:e1002092. doi: 10.1371/journal.pcbi.1002092
Gersch, T. M., Foley, N. C., Eisenberg, I., and Gottlieb, J. (2014). Neural correlates of temporal credit assignment in the parietal lobe. PloS One , 9:e88725. doi: 10.1371/journal.pone.0088725
Hayden, B. Y., Heilbronner, S. R., Pearson, J. M., and Platt, M. L. (2011). Surprise signals in anterior cingulate cortex: neuronal encoding of unsigned reward prediction errors driving adjustment in behavior. J. Neurosci. 31, 4178–4187. doi: 10.1523/JNEUROSCI.4652-10.2011
Hayden, B. Y., and Platt, M. L. (2010). Neurons in anterior cingulate cortex multiplex information about reward and action. J. Neurosci. 30, 3339–3346. doi: 10.1523/JNEUROSCI.4874-09.2010
Her, E. S., Huh, N., Kim, J., and Jung, M. W. (2016). Neuronal activity in dorsomedial and dorsolateral striatum under the requirement for temporal credit assignment. Sci. Rep. 6:27056. doi: 10.1038/srep27056
Histed, M. H., Pasupathy, A., and Miller, E. K. (2009). Learning substrates in the primate prefrontal cortex and striatum: sustained activity related to successful actions. Neuron 63, 244–253. doi: 10.1016/j.neuron.2009.06.019
Horga, G., Maia, T. V., Marsh, R., Hao, X., Xu, D., Duan, Y., et al. (2015). Changes in corticostriatal connectivity during reinforcement learning in humans. Hum. Brain Mapp. 36, 793–803. doi: 10.1002/hbm.22665
Hull, C. (1943). Principles of Behavior . New York, NY: Appleton-Century-Crofts.
Hunt, L. T., Behrens, T. E. J., Hosokawa, T., Wallis, J. D., and Kennerley, S. W. (2015). Capturing the temporal evolution of choice across prefrontal cortex. eLife 4:e11945. doi: 10.7554/eLife.11945
Izhikevich, E. M. (2007). Solving the distal reward problem through linkage of STDP and dopamine signaling. Cereb. Cortex 17, 2443–2452. doi: 10.1093/cercor/bhl152
Jackson, S. A. W., Horst, N. K., Pears, A., Robbins, T. W., and Roberts, A. C. (2016). Role of the perigenual anterior cingulate and orbitofrontal cortex in contingency learning in the marmoset. Cereb. Cortex 26, 3273–3284. doi: 10.1093/cercor/bhw067
Jocham, G., Brodersen, K. H., Constantinescu, A. O., Kahn, M. C., Ianni, A. M., Walton, M. E., et al. (2016). Reward-guided learning with and without causal attribution. Neuron 90, 177–190. doi: 10.1016/j.neuron.2016.02.018
Jog, M. S., Kubota, Y., Connolly, C. I., Hillegaart, V., and Graybiel, A. M. (1999). Building neural representations of habits. Science 286, 1745–1749. doi: 10.1126/science.286.5445.1745
Johnson, C. M., Peckler, H., Tai, L. H., and Wilbrecht, L. (2016). Rule learning enhances structural plasticity of long-range axons in frontal cortex. Nat. Commun. 7:10785. doi: 10.1038/ncomms10785
Kaiser, R. H., Treadway, M. T., Wooten, D. W., Kumar, P., Goer, F., Murray, L., et al. (2017). Frontostriatal and dopamine markers of individual differences in reinforcement learning: a multi-modal investigation. Cereb. Cortex . doi: 10.1093/cercor/bhx281. [Epub ahead of print].
Kawai, T., Yamada, H., Sato, N., Takada, M., and Matsumoto, M. (2015). Roles of the lateral habenula and anterior cingulate cortex in negative outcome monitoring and behavioral adjustment in nonhuman primates. Neuron 88, 792–804. doi: 10.1016/j.neuron.2015.09.030
Kennerley, S. W., Behrens, T. E. J., and Wallis, J. D. (2011). Double dissociation of value computations in orbitofrontal and anterior cingulate neurons. Nat. Neurosci. 14, 1581–1589. doi: 10.1038/nn.2961
Kennerley, S. W., Dahmubed, A. F., Lara, A. H., and Wallis, J. D. (2009). Neurons in the frontal lobe encode the value of multiple decision variables. J. Cogn. Neurosci. 21, 1162–1178. doi: 10.1162/jocn.2009.21100
Khamassi, M., Enel, P., Dominey, P. F., and Procyk, E. (2013). Medial prefrontal cortex and the adaptive regulation of reinforcement learning parameters. Prog. Brain Res. 202, 441–464. doi: 10.1016/B978-0-444-62604-2.00022-8
Khamassi, M., Lallée, S., Enel, P., Procyk, E., and Dominey, P. F. (2011). Robot cognitive control with a neurophysiologically inspired reinforcement learning model. Front Neurorobot 5:1. doi: 10.3389/fnbot.2011.00001
Khamassi, M., Quilodran, R., Enel, P., Dominey, P. F., and Procyk, E. (2015). Behavioral regulation and the modulation of information coding in the lateral prefrontal and cingulate cortex. Cereb. Cortex 25, 3197–3218. doi: 10.1093/cercor/bhu114
Kim, H., Lee, D., and Jung, M. W. (2013). Signals for previous goal choice persist in the dorsomedial, but not dorsolateral striatum of rats. J. Neurosci. 33, 52–63. doi: 10.1523/JNEUROSCI.2422-12.2013
Kim, H., Sul, J. H., Huh, N., Lee, D., and Jung, M. W. (2009). Role of striatum in updating values of chosen actions. J. Neurosci. 29, 14701–14712. doi: 10.1523/JNEUROSCI.2728-09.2009
Kötter, R., and Wickens, J. (1995). Interactions of glutamate and dopamine in a computational model of the striatum. J. Comput. Neurosci. 2, 195–214. doi: 10.1007/BF00961434
Lebedev, M. A., Messinger, A., Kralik, J. D., and Wise, S. P. (2004). Representation of attended versus remembered locations in prefrontal cortex. PLoS Biol. 2:e365. doi: 10.1371/journal.pbio.0020365
Mackintosh, N. J. (1975). Blocking of conditioned suppression: role of the first compound trial. J. Exp. Psychol. 1, 335–345. doi: 10.1037/0097-7403.1.4.335
Mansouri, F. A., Matsumoto, K., and Tanaka, K. (2006). Prefrontal cell activities related to monkeys' success and failure in adapting to rule changes in a Wisconsin Card Sorting Test analog. J. Neurosci. 26, 2745–2756. doi: 10.1523/JNEUROSCI.5238-05.2006
Markowitz, D. A., Curtis, C. E., and Pesaran, B. (2015). Multiple component networks support working memory in prefrontal cortex. Proc. Natl. Acad. Sci. U.S.A. 112, 11084–11089. doi: 10.1073/pnas.1504172112
Matsuda, Y., Marzo, A., and Otani, S. (2006). The presence of background dopamine signal converts long-term synaptic depression to potentiation in rat prefrontal cortex. J. Neurosci. 26, 4803–4810. doi: 10.1523/JNEUROSCI.5312-05.2006
McDannald, M. A., Esber, G. R., Wegener, M. A., Wied, H. M., Liu, T.-L., Stalnaker, T. A., et al. (2014). Orbitofrontal neurons acquire responses to “valueless” Pavlovian cues during unblocking. eLife 3:e02653. doi: 10.7554/eLife.02653
Meyers, E. M., Qi, X. L., and Constantinidis, C. (2012). Incorporation of new information into prefrontal cortical activity after learning working memory tasks. Proc. Natl. Acad. Sci. U.S.A. 109, 4651–4656. doi: 10.1073/pnas.1201022109
Mill, R. D., Bagic, A., Bostan, A., Schneider, W., and Cole, M. W. (2017). Empirical validation of directed functional connectivity. Neuroimage 146, 275–287. doi: 10.1016/j.neuroimage.2016.11.037
Monosov, I. E. (2017). Anterior cingulate is a source of valence-specific information about value and uncertainty. Nat. Commun. 8:134. doi: 10.1038/s41467-017-00072-y
Montague, P. R., Dayan, P., and Sejnowski, T. J. (1996). A framework for mesencephalic dopamine systems based on predictive Hebbian learning. J. Neurosci. 16, 1936–1947.
Morrison, S. E., Saez, A., Lau, B., and Salzman, C. D. (2011). Different time courses for learning-related changes in amygdala and orbitofrontal cortex. Neuron 71, 1127–1140. doi: 10.1016/j.neuron.2011.07.016
Mulder, A. B., Nordquist, R. E., Orgüt, O., and Pennartz, C. M. A. (2003). Learning-related changes in response patterns of prefrontal neurons during instrumental conditioning. Behav. Brain Res. 146, 77–88. doi: 10.1016/j.bbr.2003.09.016
Niv, Y. (2009). Reinforcement learning in the brain. J. Math. Psychol. 53, 139–154. doi: 10.1016/j.jmp.2008.12.005
Niv, Y., Daniel, R., Geana, A., Gershman, S. J., Leong, Y. C., Radulescu, A., et al. (2015). Reinforcement learning in multidimensional environments relies on attention mechanisms. J. Neurosci. 35, 8145–8157. doi: 10.1523/JNEUROSCI.2978-14.2015
Noonan, M. P., Chau, B. K. H., Rushworth, M. F. S., and Fellows, L. K. (2017). Contrasting effects of medial and lateral orbitofrontal cortex lesions on credit assignment and decision-making in humans. J. Neurosci . 37, 7023–7035. doi: 10.1523/JNEUROSCI.0692-17.2017
Noonan, M. P., Walton, M. E., Behrens, T. E., Sallet, J., Buckley, M. J., and Rushworth, M. F. (2010). Separate value comparison and learning mechanisms in macaque medial and lateral orbitofrontal cortex. Proc. Natl. Acad. Sci. U.S.A. 107, 20547–20252. doi: 10.1073/pnas.1012246107
Oemisch, M., Westendorff, S., Everling, S., and Womelsdorf, T. (2015). Interareal spike-train correlations of anterior cingulate and dorsal prefrontal cortex during attention shifts. J. Neurosci. 35, 13076–13089. doi: 10.1523/JNEUROSCI.1262-15.2015
Padoa-Schioppa, C., and Assad, J. A. (2006). Neurons in the orbitofrontal cortex encode economic value. Nature 441, 223–226 doi: 10.1038/nature04676
Padoa-Schioppa, C., and Assad, J. A. (2008). The representation of economic value in the orbitofrontal cortex is invariant for changes of menu. Nat. Neurosci. 11, 95–102. doi: 10.1038/nn2020
Pan, W. X., Schmidt, R., Wickens, J. R., and Hyland, B. I. (2005). Dopamine cells respond to predicted events during classical conditioning: evidence for eligibility traces in the reward-learning network. J. Neurosci. 25, 6235–6242. doi: 10.1523/JNEUROSCI.1478-05.2005
Pasupathy, A., and Miller, E. K. (2005). Different time courses of learning-related activity in the prefrontal cortex and striatum. Nature 433, 873–876. doi: 10.1038/nature03287
Pearson, J. M., Hayden, B. Y., Raghavachari, S., and Platt, M. L. (2009). Neurons in posterior cingulate cortex signal exploratory decisions in a dynamic multioption choice task. Curr. Biol. 19, 1532–1537. doi: 10.1016/j.cub.2009.07.048
Procyk, E., Tanaka, Y. L., and Joseph, J. P. (2000). Anterior cingulate activity during routine and non-routine sequential behaviors in macaques. Nat. Neurosci. 3, 502–508. doi: 10.1038/74880
Quilodran, R., Rothe, M., and Procyk, E. (2008). Behavioral shifts and action valuation in the anterior cingulate cortex. Neuron 57, 314–325. doi: 10.1016/j.neuron.2007.11.031
Roelfsema, P. R., and van Ooyen, A. (2005). Attention-gated reinforcement learning of internal representations for classification. Neural. Comput. 17, 2176–2214. doi: 10.1162/0899766054615699
Rothkopf, C. A., and Ballard, D. H. (2010). Credit assignment in multiple goal embodied visuomotor behavior. Front. Psychol. 1:173. doi: 10.3389/fpsyg.2010.00173
Rudebeck, P. H., Behrens, T. E., Kennerley, S. W., Baxter, M. G., Buckley, M. J., Walton, M. E., et al. (2008). Frontal cortex subregions play distinct roles in choices between actions and stimuli. J. Neurosci. 28, 13775–13785. doi: 10.1523/JNEUROSCI.3541-08.2008
Saez, R. A., Saez, A., Paton, J. J., Lau, B., and Salzman, C. D. (2017). Distinct roles for the amygdala and orbitofrontal cortex in representing the relative amount of expected reward. Neuron 95, 70.e3–77.e3. doi: 10.1016/j.neuron.2017.06.012
Scholl, J., and Klein-Flügge, M. (2017). Understanding psychiatric disorder by capturing ecologically relevant features of learning and decision-making. Behav Brain Res . doi: 10.1016/j.bbr.2017.09.050. [Epub ahead of print].
Schultz, W. (1998a). Predictive reward signal of dopamine neurons. J. Neurophysiol. 80, 1–27. doi: 10.1152/jn.1998.80.1.1
Schultz, W. (1998b). The phasic reward signal of primate dopamine neurons. Adv. Pharmacol. 42, 686–690. doi: 10.1016/S1054-3589(08)60841-8
Schultz, W. (2004). Neural coding of basic reward terms of animal learning theory, game theory, microeconomics and behavioural ecology. Curr. Opin. Neurobiol. 14, 139–147. doi: 10.1016/j.conb.2004.03.017
Schultz, W., and Dickinson, A. (2000). Neuronal coding of prediction errors. Ann. Rev. Neurosci. 23, 473–500. doi: 10.1146/annurev.neuro.23.1.473
Seo, H., Barraclough, D. J., and Lee, D. (2007). Dynamic signals related to choices and outcomes in the dorsolateral prefrontal cortex. Cerebral Cortex 17(Suppl. 1), i110–i117. doi: 10.1093/cercor/bhm064
Seo, H., and Lee, D. (2007). Temporal filtering of reward signals in the dorsal anterior cingulate cortex during a mixed-strategy game. J. Neurosci. 27, 8366–8377. doi: 10.1523/JNEUROSCI.2369-07.2007
Seo, M., Lee, E., and Averbeck, B. B. (2012). Action selection and action value in frontal-striatal circuits. Neuron 74, 947–960. doi: 10.1016/j.neuron.2012.03.037
Seol, G. H., Ziburkus, J., Huang, S., Song, L., Kim, I. T., Takamiya, K., et al. (2007). Neuromodulators control the polarity of spike-timing-dependent synaptic plasticity. Neuron 55, 919–929. doi: 10.1016/j.neuron.2007.08.013
Shidara, M., and Richmond, B. J. (2002). Anterior cingulate: single neuronal signals related to degree of reward expectancy. Science 296, 1709–1711. doi: 10.1126/science.1069504
Shuler, M. G., and Bear, M. F. (2006). Reward timing in the primary visual cortex. Science 311, 1606–1609. doi: 10.1126/science.1123513
Sternson, S. M., and Roth, B. L. (2014). Chemogenetic tools to interrogate brain functions. Ann. Rev. Neurosci. 37, 387–407. doi: 10.1146/annurev-neuro-071013-014048
Stokes, M. G., Kusunoki, M., Sigala, N., Nili, H., Gaffan, D., and Duncan, J. (2013). Dynamic coding for cognitive control in prefrontal cortex. Neuron 78, 364–375. doi: 10.1016/j.neuron.2013.01.039
Sul, J. H., Kim, H., Huh, N., Lee, D., and Jung, M. W. (2010). Distinct roles of rodent orbitofrontal and medial prefrontal cortex in decision making. Neuron 66, 449–460. doi: 10.1016/j.neuron.2010.03.033
Sutton, R. S., and Barto, A. G. (1998). Reinforcement Learning: An Introduction Vol. 1 Cambridge: MIT press
Swanson, A. M., Allen, A. G., Shapiro, L. P., and Gourley, S. L. (2015). GABAAα1-mediated plasticity in the orbitofrontal cortex regulates context-dependent action selection. Neuropsychopharmacology 40, 1027–1036. doi: 10.1038/npp.2014.292
Tremblay, L., and Schultz, W. (1999). Relative reward preference in primate orbitofrontal cortex. Nature 398, 704–708. doi: 10.1038/19525
Tsujimoto, S., Genovesio, A., and Wise, S. P. (2009). Monkey orbitofrontal cortex encodes response choices near feedback time. J. Neurosci. 29, 2569–2574. doi: 10.1523/JNEUROSCI.5777-08.2009
Tsujimoto, S., Genovesio, A., and Wise, S. P. (2011). Comparison of strategy signals in the dorsolateral and orbital prefrontal cortex. J. Neurosci. 31, 4583–4592. doi: 10.1523/JNEUROSCI.5816-10.2011
Urbanczik, R., and Senn, W. (2009). Reinforcement learning in populations of spiking neurons. Nat. Neurosci. 12, 250–252. doi: 10.1038/nn.2264
Voloh, B., Valiante, T. A., Everling, S., and Womelsdorf, T. (2015). Theta-gamma coordination between anterior cingulate and prefrontal cortex indexes correct attention shifts. Proc. Natl. Acad. Sci. U.S.A. 112, 8457–8462. doi: 10.1073/pnas.1500438112
Wallis, J. D., and Miller, E. K. (2003). Neuronal activity in primate dorsolateral and orbital prefrontal cortex during performance of a reward preference task. Eur. J. Neurosci. 18, 2069–2081. doi: 10.1046/j.1460-9568.2003.02922.x
Walsh, M. M., and Anderson, J. R. (2014). Navigating complex decision spaces: problems and paradigms in sequential choice. Psychol. Bull. 140, 466–486. doi: 10.1037/a0033455
Wilson, R. C., and Niv, Y. (2011). Inferring relevance in a changing world. Front Hum. Neurosci. 5:189. doi: 10.3389/fnhum.2011.00189
Wilson, R. C., Takahashi, Y. K., Schoenbaum, G., and Niv, Y. (2014). Orbitofrontal cortex as a cognitive map of task space. Neuron 81, 267–279. doi: 10.1016/j.neuron.2013.11.005
Womelsdorf, T., Ardid, S., Everling, S., and Valiante, T. A. (2014). Burst firing synchronizes prefrontal and anterior cingulate cortex during attentional control. Curr. Biol. 24, 2613–2621. doi: 10.1016/j.cub.2014.09.046
Wörgötter, F., and Porr, B. (2005). Temporal sequence learning, prediction, and control: a review of different models and their relation to biological mechanisms. Neural. Comput. 17, 245–319. doi: 10.1162/0899766053011555
Xie, J., and Padoa-Schioppa, C. (2016). Neuronal remapping and circuit persistence in economic decisions. Nat. Neurosci. 19, 855–861. doi: 10.1038/nn.4300
Xu, Y. (2017). Reevaluating the sensory account of visual working memory storage. Trends Cogn. Sci. 21, 794–815 doi: 10.1016/j.tics.2017.06.013
Zhang, J. C., Lau, P.-M., and Bi, G.-Q. (2009). Gain in sensitivity and loss in temporal contrast of STDP by dopaminergic modulation at hippocampal synapses. Proc. Natl. Acad. Sci. U.S.A. 106, 13028–13033 doi: 10.1073/pnas.0900546106
Zsuga, J., Biro, K., Tajti, G., Szilasi, M. E., Papp, C., Juhasz, B., et al. (2016). ‘Proactive’ use of cue-context congruence for building reinforcement learning's reward function. BMC Neurosci. 17:70. doi: 10.1186/s12868-016-0302-7
Keywords: orbitofrontal, dorsolateral prefrontal, anterior cingulate, learning, reward, reinforcement, plasticity, behavioral flexibility
Citation: Stolyarova A (2018) Solving the Credit Assignment Problem With the Prefrontal Cortex. Front. Neurosci . 12:182. doi: 10.3389/fnins.2018.00182
Received: 27 September 2017; Accepted: 06 March 2018; Published: 27 March 2018.
Reviewed by:
Copyright © 2018 Stolyarova. This is an open-access article distributed under the terms of the Creative Commons Attribution License (CC BY) . The use, distribution or reproduction in other forums is permitted, provided the original author(s) and the copyright owner are credited and that the original publication in this journal is cited, in accordance with accepted academic practice. No use, distribution or reproduction is permitted which does not comply with these terms.
*Correspondence: Alexandra Stolyarova, [email protected]
Disclaimer: All claims expressed in this article are solely those of the authors and do not necessarily represent those of their affiliated organizations, or those of the publisher, the editors and the reviewers. Any product that may be evaluated in this article or claim that may be made by its manufacturer is not guaranteed or endorsed by the publisher.
Towards Practical Credit Assignment for Deep Reinforcement Learning
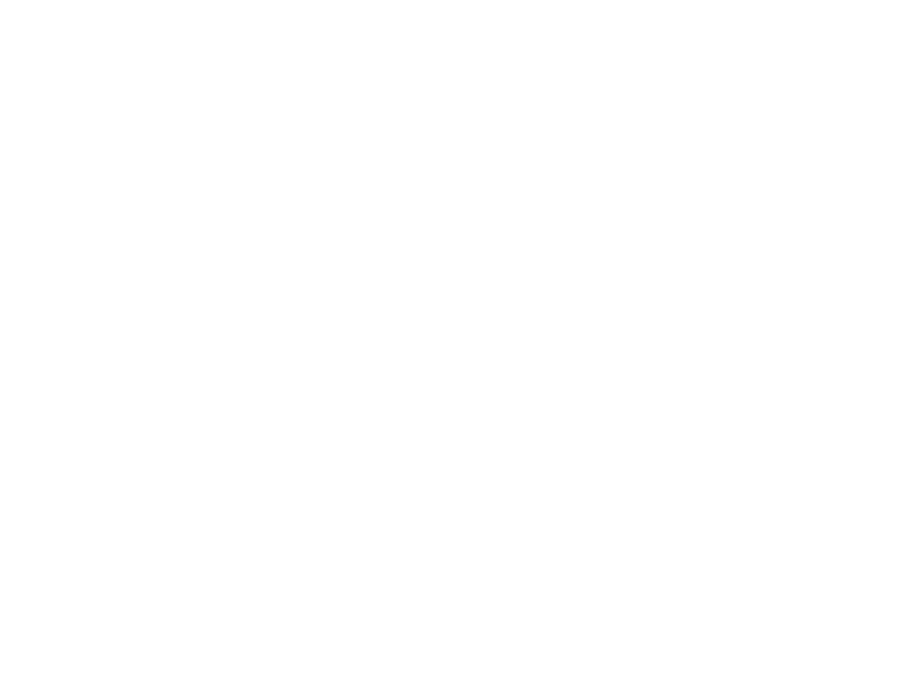
Credit assignment is a fundamental problem in reinforcement learning , the problem of measuring an action's influence on future rewards. Improvements in credit assignment methods have the potential to boost the performance of RL algorithms on many tasks, but thus far have not seen widespread adoption. Recently, a family of methods called Hindsight Credit Assignment (HCA) was proposed, which explicitly assign credit to actions in hindsight based on the probability of the action having led to an observed outcome. This approach is appealing as a means to more efficient data usage, but remains a largely theoretical idea applicable to a limited set of tabular RL tasks, and it is unclear how to extend HCA to Deep RL environments. In this work, we explore the use of HCA-style credit in a deep RL context. We first describe the limitations of existing HCA algorithms in deep RL, then propose several theoretically-justified modifications to overcome them. Based on this exploration, we present a new algorithm, Credit-Constrained Advantage Actor-Critic (C2A2C), which ignores policy updates for actions which don't affect future outcomes based on credit in hindsight, while updating the policy as normal for those that do. We find that C2A2C outperforms Advantage Actor-Critic (A2C) on the Arcade Learning Environment (ALE) benchmark, showing broad improvements over A2C and motivating further work on credit-constrained update rules for deep RL methods.

Vyacheslav Alipov
Riley Simmons-Edler
Nikita Putintsev
Pavel Kalinin
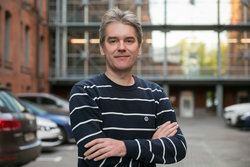
Dmitry Vetrov
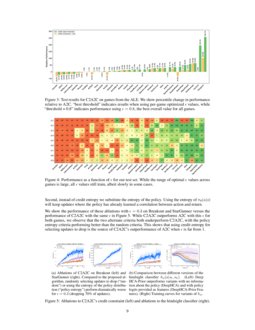
Related Research
Hindsight credit assignment, from credit assignment to entropy regularization: two new algorithms for neural sequence prediction, learning guidance rewards with trajectory-space smoothing, pairwise weights for temporal credit assignment, counterfactual credit assignment in model-free reinforcement learning, variance reduced advantage estimation with δ hindsight credit assignment, direct advantage estimation.
Please sign up or login with your details
Generation Overview
AI Generator calls
AI Video Generator calls
AI Chat messages
Genius Mode messages
Genius Mode images
AD-free experience
Private images
- Includes 500 AI Image generations, 1750 AI Chat Messages, 30 AI Video generations, 60 Genius Mode Messages and 60 Genius Mode Images per month. If you go over any of these limits, you will be charged an extra $5 for that group.
- For example: if you go over 500 AI images, but stay within the limits for AI Chat and Genius Mode, you'll be charged $5 per additional 500 AI Image generations.
- Includes 100 AI Image generations and 300 AI Chat Messages. If you go over any of these limits, you will have to pay as you go.
- For example: if you go over 100 AI images, but stay within the limits for AI Chat, you'll have to reload on credits to generate more images. Choose from $5 - $1000. You'll only pay for what you use.
Out of credits
Refill your membership to continue using DeepAI
Share your generations with friends
Credit assignment in heterogeneous multi-agent reinforcement learning for fully cooperative tasks
- Published: 26 October 2023
- Volume 53 , pages 29205–29222, ( 2023 )
Cite this article
- Kun Jiang 1 , 2 ,
- Wenzhang Liu 3 ,
- Yuanda Wang 1 ,
- Lu Dong 4 &
- Changyin Sun ORCID: orcid.org/0000-0001-9269-334X 1 , 2
629 Accesses
Explore all metrics
Credit assignment poses a significant challenge in heterogeneous multi-agent reinforcement learning (MARL) when tackling fully cooperative tasks. Existing MARL methods assess the contribution of each agent through value decomposition or agent-wise critic networks. However, value decomposition techniques are not directly applicable to control problems with continuous action spaces. Additionally, agent-wise critic networks struggle to differentiate the distinct contributions from the shared team reward. Moreover, most of these methods assume agent homogeneity, which limits their utility in more diverse scenarios. To address these limitations, we present a novel algorithm that factorizes and reshapes the team reward into agent-wise rewards, enabling the evaluation of the diverse contributions of heterogeneous agents. Specifically, we devise agent-wise local critics that leverage both the team reward and the factorized reward, alongside a global critic for assessing the joint policy. By accounting for the contribution differences resulting from agent heterogeneity, we introduce a power balance constraint that ensures a fairer measurement of each heterogeneous agent’s contribution, ultimately promoting energy efficiency. Finally, we optimize the policies of all agents using deterministic policy gradients. The effectiveness of our proposed algorithm has been validated through simulation experiments conducted in fully cooperative and heterogeneous multi-agent tasks.
This is a preview of subscription content, log in via an institution to check access.
Access this article
Price includes VAT (Russian Federation)
Instant access to the full article PDF.
Rent this article via DeepDyve
Institutional subscriptions
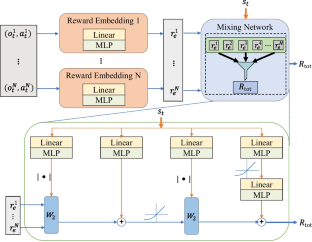
Similar content being viewed by others
VAAC: V-value Attention Actor-Critic for Cooperative Multi-agent Reinforcement Learning
A Game-Theoretic Approach to Multi-agent Trust Region Optimization
Multi-Agent Reinforcement Learning
Data availability.
The data that support the findings of this study are available on request from the first author.
Vinyals O, Babuschkin I, Czarnecki WM, Mathieu M, Dudzik A, Chung J, Choi DH, Powell R, Ewalds T, Georgiev P et al (2019) Grandmaster level in starcraft ii using multi-agent reinforcement learning. Nature, 350-354
Chen Y, Zheng Z, Gong X (2022) Marnet: Backdoor attacks against cooperative multi-agent reinforcement learning. IEEE Trans Dependable Sec Comput, 1-11
Liu X, Wang G, Chen K (2022) Option-based multi-agent reinforcement learning for painting with multiple large-sized robots. IEEE Trans Intell Transp Syst, 15707-15715
Chen YJ, Chang DK, Zhang C (2020) Autonomous tracking using a swarm of uavs: A constrained multi-agent reinforcement learning approach. IEEE Trans Veh Technol. 13702-13717
Zhou W, Chen D, Yan J, Li Z, Yin H, Ge W (2022) Multi-agent reinforcement learning for cooperative lane changing of connected and autonomous vehicles in mixed traffic. Auton Intell Syst
Dinneweth J, Boubezoul A, Mandiau R, Espié S (2022) Multi-agent reinforcement learning for autonomous vehicles: a survey. Auton Intell Syst, 27
Sun C, Liu W, Dong, L (2021) Reinforcement learning with task decomposition for cooperative multiagent systems. IEEE Trans Neural Netw Learn Syst, 2054-2065
Liu X, Tan Y (2022) Feudal latent space exploration for coordinated multi-agent reinforcement learning. IEEE Trans Neural Netw Learn Syst, 1-9
Yarahmadi H, Shiri ME, Navidi H, Sharifi A, Challenger M (2023) Bankruptcyevolutionary games based solution for the multi-agent credit assignment problem. Swarm Evol Comput, 101229
Ding S, Du W, Ding L, Guo L, Zhang J, An B (2023) Multi-agent dueling qlearning with mean field and value decomposition. Pattern Recognition, 109436
Du W, Ding S, Guo L, Zhang J, Zhang C, Ding L (2022) Value function factorization with dynamic weighting for deep multi-agent reinforcement learning. Information Sciences, 191-208
Rashid T, Samvelyan M, Schroeder C, Farquhar G, Foerster J, Whiteson S (2018) QMIX: Monotonic value function factorisation for deep multi-agent reinforcement learning. Proceedings of the 35th Int Conf Mac Learn, 4295-4304
Lowe R, Wu Y, Tamar A, Harb J, Abbeel P, Mordatch I (2017) Multiagent actor-critic for mixed cooperative-competitive environments. Adv Neural Info Process Syst, 6379-6390
Lyu X, Xiao Y, Daley B, Amato C (2021) Contrasting centralized and decentralized critics in multi-agent reinforcement learning. 20th Int Conf Auton Agents & Multiagent Syst, 844-852
Oroojlooy A, Hajinezhad D (2022) A review of cooperative multi-agent deep reinforcement learning. Appl Intell, 1-46
Wang J, Yuan M, Li Y, Zhao Z (2023) Hierarchical attention master-slave for heterogeneous multi-agent reinforcement learning. Neural Netw, 359-368
Mahajan A, Rashid T, Samvelyan M, Whiteson S (2019) MAVEN: multiagent variational exploration. In: Adv Neural Info Process Syst, pp. 7611-7622
Li W, He S, Mao X, Li B, Qiu C, Yu J, Peng F, Tan X (2023) Multiagent evolution reinforcement learning method for machining parameters optimization based on bootstrap aggregating graph attention network simulated environment. J Manuf Syst, 424-438
Qiu D, Wang J, Dong Z, Wang Y, Strbac G (2022) Mean-field multi-agent reinforcement learning for peer-to-peer multi-energy trading. IEEE Trans Power Syst, 1-13
Lee HR, Lee T (2021) Multi-agent reinforcement learning algorithm to solve a partially-observable multi-agent problem in disaster response. Euro J Oper Res, 296-308
Foerster J, Farquhar G, Afouras T, Nardelli N, Whiteson S (2018) Counterfactual multi-agent policy gradients. Proceedings of the AAAI Conf Artif Intell, 2974-2982
Guo D, Tang L, Zhang X, Liang YC (2020) Joint optimization of handover control and power allocation based on multi-agent deep reinforcement learning. IEEE Trans Veh Technol, 13124-13138
Hou Y, Sun M, Zeng Y, Ong YS, Jin Y, Ge H, Zhang Q (2023) A multi-agent cooperative learning system with evolution of social roles. IEEE Trans Evol Comput
Yang J, Nakhaei A, Isele D, Fujimura K, Zha H (2020) CM3: cooperative multi-goal multi-stage multi-agent reinforcement learning. In: 8th International Conference on Learning Representations
Nguyen DT, Kumar A, Lau HC (2017) Collective multiagent sequential decision making under uncertainty. Proceedings of the 31st Conference on Artificial Intelligence, 3036-3043
Du Y, Han L, Fang M, Liu J, Dai T, Tao D (2019) LIIR: learning individual intrinsic reward in multi-agent reinforcement learning. Adv Neural Inf Process Syst, 4405-4416
Sunehag P, Lever G, Gruslys A, Czarnecki WM, Zambaldi VF, Jaderberg M, Lanctot M, Sonnerat N, Leibo JZ, Tuyls K, Graepel T (2018) Value-decomposition networks for cooperative multi-agent learning based on team reward. Proceedings of the 17th International Conference on Autonomous Agents and MultiAgent Systems, 2085-2087
Son K, Kim D, Kang WJ, Hostallero DE, Yi Y (2019) Qtran: Learning to factorize with transformation for cooperative multi-agent reinforcement learning. Int Conf Mach Learn, p 5887-5896
Rashid T, Farquhar G, Peng B, Whiteson S (2020) Weighted qmix: Expanding monotonic value function factorisation for deep multi-agent reinforcement learning. Adv neural inf process syst, 10199-10210
Zhou D, Gayah VV (2023) Scalable multi-region perimeter metering control for urban networks: A multi-agent deep reinforcement learning approach. Transp Res Part C Emerg Technol, p 104033
Liu S, Liu W, Chen W, Tian G, Chen J, Tong Y, Cao J, Liu Y (2023) Learning multi-agent cooperation via considering actions of teammates. IEEE Trans Neural Netw Learn Syst, p 1-12
DENG H, LI Y, YIN Q (2023) Improved qmix algorithm from communication and exploration for multi-agent reinforcement learning. J Comput Appl, p 202
Zhang Y, Ma H, Wang Y (2021) Avd-net: Attention value decomposition network for deep multi-agent reinforcement learning. 25th International Conference on Pattern Recognition, p 7810-7816
Qin Z, Johnson D, Lu Y (2023) Dynamic production scheduling towards selforganizing mass personalization: A multi-agent dueling deep reinforcement learning approach. J Manuf Syst, 242-257
Wang X, Zhang L, Lin T, Zhao C,Wang K, Chen Z (2022) Solving job scheduling problems in a resource preemption environment with multi-agent reinforcement learning. Robot Comput Integr Manuf, 102324
Yu T, Huang J, Chang Q (2021) Optimizing task scheduling in human-robot collaboration with deep multi-agent reinforcement learning. J Manuf Syst, 487-499
Wu H, Ghadami A, Bayrak AE, Smereka JM, Epureanu BI (2021) Impact of heterogeneity and risk aversion on task allocation in multi-agent teams. IEEE Robotics and Automation Letters, 7065-7072
Zhao Y, Xian C, Wen G, Huang P, Ren W (2022) Design of distributed eventtriggered average tracking algorithms for homogeneous and heterogeneous multiagent systems. IEEE Transactions on Automatic Control, 1269-1284
Jiang W, Feng G, Qin S, Yum TSP, Cao G (2019) Multi-agent reinforcement learning for efficient content caching in mobile d2d networks. IEEE Trans Wirel Commun, 1610-1622
Jahn J (2020) Introduction to the theory of nonlinear optimization. Springer Nature
Download references
Author information
Authors and affiliations.
School of Automation, Southeast University, Nanjing, 210096, Jiangsu, China
Kun Jiang, Yuanda Wang & Changyin Sun
Peng Cheng Laboratory, Shenzhen, 518955, Guangdong, China
Kun Jiang & Changyin Sun
School of Artificial Intelligence, Anhui University, Hefei, 230039, Anhui, China
Wenzhang Liu
School of Cyber Science and Engineering, Southeast University, Nanjing, 211189, Jiangsu, China
You can also search for this author in PubMed Google Scholar
Corresponding author
Correspondence to Changyin Sun .
Ethics declarations
Conflicts of interest.
No potential conflict of interest was reported by the authors.
Additional information
Publisher's note.
Springer Nature remains neutral with regard to jurisdictional claims in published maps and institutional affiliations.
Rights and permissions
Springer Nature or its licensor (e.g. a society or other partner) holds exclusive rights to this article under a publishing agreement with the author(s) or other rightsholder(s); author self-archiving of the accepted manuscript version of this article is solely governed by the terms of such publishing agreement and applicable law.
Reprints and permissions
About this article
Jiang, K., Liu, W., Wang, Y. et al. Credit assignment in heterogeneous multi-agent reinforcement learning for fully cooperative tasks. Appl Intell 53 , 29205–29222 (2023). https://doi.org/10.1007/s10489-023-04866-0
Download citation
Accepted : 02 July 2023
Published : 26 October 2023
Issue Date : December 2023
DOI : https://doi.org/10.1007/s10489-023-04866-0
Share this article
Anyone you share the following link with will be able to read this content:
Sorry, a shareable link is not currently available for this article.
Provided by the Springer Nature SharedIt content-sharing initiative
- Credit assignment
- Multi-agent reinforcement learning
- Reward decomposition
- Heterogeneous agents
- Find a journal
- Publish with us
- Track your research
- Skip to primary navigation
- Skip to content
- Skip to primary sidebar
Tim Dettmers
Making deep learning accessible.
Header Right
Blog posts topics.
- PhD Life (3)
- Deep Learning (7)
- Hardware (8)
- Neuroscience (1)
Main navigation
Credit assignment in deep learning.
2017-09-16 by Tim Dettmers 15 Comments
This morning I got an email about my blog post discussing the history of deep learning which rattled me back into a time of my academic career which I rather not think about. It was a low point which nearly ended my Master studies at the University of Lugano, and it made me feel so bad about blogging that I took two long years to recover. So what has happened?
When I started my masters, I worked on blog posts for NVIDIA which featured introductions into deep learning. Part of this blog post series also discusses the history of deep learning. I hence discussed what I thought to be the historical milestones with the largest impact but in doing so, I inadvertently assigned credit to researchers that I thought had a good impact on the field. I worked on this blog post and circulated it in my deep learning class’s forums to the dismay of my then advisor who holds the opposite view of mine.
To evaluate the credit that a research idea deserves, I believe that it is not only important who has the first idea, but I also believe that it is equally important to actually make it work (the implementation). My ex-advisor believed that it only really matters who was the first who published the idea.
My advisor scolded me in class for my views since he felt very strongly that the first idea counts and that my view is plain wrong. To redeem myself and to salvage the relationship with him, I felt coerced to change my blog post to his wishes.
This quasi-censorship of my blog post eviscerated me, and in consequence, I lost all desire to blog for two years. Despite my efforts, the relationship with my then advisor deteriorated further, and I had to look for a new advisor.
Looking back at the blog post that I produced, I feel ashamed. It does not express my personal views. I value integrity, and my behavior did not reflect who I want to be.
I write this blog post to discuss my true beliefs about credit assignment and why I believe that the idea, its communication and its implementation are all equally important.
Who Deserves Credit for Deep Learning Ideas?
There has been a lot of discussion about how to assign credit to researchers, or in other words, how to determine whose work had a large impact. Note that I do not discuss here who deserves credit for discovering an idea, I look at who deserves credit for the impact that an idea has. Looking at this, there are two main camps: The first believes that ideas and implementation count equally, and, the second believes that it counts who had the ideas first.
The problem with this discussion is that it is not a scientific topic, but a philosophical one. How do we determine what has how much value? We use the scientific method. What is the scientific method in philosophy? Use reductions to arrive at simple statements, then use logic to derive other factual statements, failing that — like in this case — we make thought experiments where we isolate variables which we then take to extremes. Let’s do this now to get insight into the issue.
All Ideas, No Communication, No Implementation
Let’s imagine there exists a person that has come up with all ideas in deep learning of the past and all ideas in deep learning of the future. However, this person cannot communicate with either words or writing. This person also cannot write code. How much credit deserves such a person?
I would argue that such a person deserves zero credit. In fact, I think it is epistemologically correct that this person deserves no credit because nobody can know that he or she deserves credit.
All Ideas, 1 Communication + No Ideas, Full Communication
We have a Person 1 that invented everything in deep learning. Now this person can communicate, but he or she is so unclear that only a single Person 2 can understand these ideas.
Now, Person 2 has no creativity but is a perfect communicator. Person 2 basically just translates what Person 1 said and the entire world understands. Who deserves credit here?
It is tempting to think that Person 2 deserves all the credit because Person 1 is useless without Person 2. But similarly, Person 2 is useless without Person 1.
Both people thus deserve equal credit — no one can achieve anything without the other.
All Ideas, Full Communication, 1 Implementation
Let’s increase the complexity of the problem. Let us say the duo of Person 1 and Person 2 spread the ideas so that the entire world understands deep learning, but let us assume that all people are implementation agnostic. Nobody can make deep learning work. The world knows about all deep learning ideas but cannot solve any problem with it. In such a world, the ideas of deep learning are quickly abandoned by the large majority due to their uselessness (just like the majority of the population does not care much about pure mathematics, e.g., few care if a n + b n = c n is true for all integer n >2).
Enter Person 3. Person 3 has no creativity, cannot communicate, but he or she can implement all the deep learning ideas in a practical manner. The world looks at this person’s code and suddenly is able to solve all problems which are solvable with deep learning.
Who deserves the most credit: Person 1, Person 2, or Person 3?
As discussed before, Person 1 and Person 2 deserve equal credit, and also here, I would argue, that Person 3 deserves equal credit.
This becomes apparent when we think about the value of ideas. Ideas are useful when they have an affect. If they have no or only a small effect they just deserve no recognition or little recognition. If deep learning ideas have no practical value then they would not deserve more recognition than, say, the idea that there might be something beyond the observable universe — it is a nice idea, but it will never produce anything of much value.
Comparative Individual Value For Collective Contributions
The evaluation changes if we distribute the contributions of ideas, communication, and implementation among many individuals. If we can take the three scenarios above, expand Person 1-3 into groups of people and subject them to comparative evaluation, that is, how much value the contributions of each individual has compared to all the other people have we arrive at the following thought experiment.
1 Ideas, 1000 Communication, 1000 Implementation
We have 1 person who has all the ideas, 1000 people who can understand these ideas and communicate them to the world, and 1000 people who can implement them to yield practical value, then how do we assign credit?
As discussed it is reasonable that each of the areas, (1) ideas, (2) communication, (3) implementation deserve equal credit. If now the groups of 1000 people made contributions (communications and implementations) of equal value, it would be fair to say that:
- 1 Ideas: 1/3 credit
- 1000 Communication: 1/3000 credit each
- 1000 Implementation: 1/3000 credit each.
We see in this case the one person with the idea should receive the largest amount of credit.
Similarly, if we weight the numbers differently, and if we assume contributions of individuals in groups are equal, then this credit assignment holds for all other combinations like (1000, 1, 1000), or (10000, 1000, 1).
Timing and Relational Effects
In the real world, we have timing effects and relational effects. Not all 1000 Ideas, Communication, or Implementation people will publish their work at the same time, but they will have a specific sequence. In this sequence, they will influence and build on each other — they stand on the shoulders of giants. Who are the giants? Who deserves what amount of credit?
If we think about it, it is not much different than our first analysis. Lets take Person 1 that only has ideas and can communicate his or her ideas to only one other Person 2; Person 2, standing on Person 1’s shoulders, is only able to communicate the ideas to another person Person 3; Person 3, standing on Person 2’s shoulders, in turn, can communicate the ideas clearly to the entire world.
If we express the ability of people as numbers which represent the fraction of all value ideas, communication, and implementation we could weight Person 1, Person 2, and Person 3 in this way:
- Person1: [1, 1/10^10, 0]
- Person2: [0, 1/10^10, 0]
- Person3: [0, 1, 0]
Which means that Person 1, has all the ideas (1), could communicate these ideas to 1 person (we assume a total population of 10 billion people to make the math easier). Person 2 has no ideas, could understand Person 1’s idea but could only communicate this idea to one other person, Person 3. Person 3 has no ideas, understands the idea of Person 2 and can communicate it so that everybody understands. Note that this example is simplified so that all people are implementation agnostic.
From these fractions, we see that Person 2 has almost no fraction of contributions since Person 2 is not creative and also not a good communicator. However, if we look at the relational effects we know Person 3 would have no value without Person 2, and Person 1 would also have no value without Person 2. So how do we solve this credit assignment problem?
We can try to solve this problem by expressing it as a weighted graph which expressed relationships over time and the relationships of the fractions with respect to the world.

How we weight the contribution of each person in this case? There are many answers to this, but here PageRank would be a good fit. PageRank works exactly as we discussed above, the credit is assigned comparatively, that is if we have a (1, 1000, 1000) distribution, the largest chunk of PageRank will be distributed by the single person. Thus it reflects our evaluation system. PageRank also takes into account the relationships between nodes and their recursive weight (standing on the shoulders of giants).
Using the scenario above, we find the contributions as follows:
We see that P2 has the largest contribution despite being only the bridge between P1 and P3 who have the largest fractions (all the ideas and full communication abilities). However, P1’s success depends on P2, and P3’s success depends on P2 and as such P2 is the most critical link in the entire system.
This is quite insightful. If you understand some obscure research and communicate this to just a few researchers who, in turn, influence many other researchers then you will have made a substantial contribution to the deep learning community.
It would not feel this way because you will probably not experience any fame or recognition here. The recognition will come for P1 (having ideas) and P3 (communicating ideas). But still, the numbers do not lie here.
This experiment was quite interesting, and if you want to experiment a bit by yourself, you can download the code to see what happens if you add more people and more relationships among these people. This exercise can give quite some insight into what is valuable for research.
Response to Criticism on Reddit
There has been some sharp criticism on Reddit concerning ideas expressed in this blog post. The user metacurse makes the point that in science we credit usually those researchers who had the idea first and that communication and implementation are not valued. For example we value Albert Einstein more highly for the discovery of general relativity and the photoelectric effect and not its communication by Neil deGrasse Tyson; similarly, Cocks is credited for RSA even though he never implemented it in any way that was widely used (and he could not produce public implementations due to the classified status of RSA). However, this entire argument is rather weak and unfair:
- I do not discuss who should be credited for an idea or the usage of the idea, I discuss who should be credited for the overall impact of an idea. These are very different questions.
- He uses examples to try to prove his own hypothesis when we know that examples cannot prove anything (he uses classical philosophic techniques, which has some value, but it does not generate any reliable knowledge like analytical philosophy does). He mocks me for not using examples myself.
- He appeals to the emotion of the readers, by saying that my views endorse unethical ideas like “stealing olds ideas and rebranding them as your own” when it has nothing to do with my argument (reductio ad Hitlerum). He does this quite successfully swaying many emotional readers. I do not think this is helpful.
To make a sharper contrast why metacurse’s argument is not relevant to mine take this thought experiment.
We have a super genius who knows about all possible ideas and writes them down so that everybody can understand it easily. Then she locks these notes away in a locker and dies the next second. Over the next billions of years humanity rediscovers all ideas and uses them to build a flourishing society where all living things live in harmony and every being is fulfilled and so forth. One second before the last human dies in heat death, that human discovers the notebook.
Metacurse’s argument would look for the answer to the question: Should our super genius be credited for inventing everything? Metacurse would argue, yes, and I would totally agree.
What I discuss in this blog post: How much impact did our super genius have on the overall impact of all ideas? Very little, she never had any direct or even indirect effect with any of the ideas; the only impact she had was that one other person understood that she had the ideas before others had them. That is the total impact of her ideas. Her impact is almost zero.
Here I discussed how it is best to think about contributions in deep learning. From thought experiments, we could see that ideas, their communication, and their implementation are equally important contributions.
We also discussed how timing effects and dependencies could be modeled in a relational graph. We found that people that link ideas to communicators can make substantial contributions to the research community even if they themselves are not creative or good communicators. Creating the links between influential ideas and influential communicators (or people that implement) are important here.
Related Posts

Reader Interactions
Murray Frank says
2018-01-03 at 02:48
Giving credit is a long debated problem. Frequently someone comes up with an idea that has a huge influence. Then other people say that in reality someone else had really thought of the idea earlier. Often such claims are true. In other cases you can see the essence of the idea but not the whole thing in the earlier work. In some cases we retroactively give credit. In other cases it does not happen. For example, Kuhn and Tucker came up with a standard theorem in optimization in 1951. Eventually people realized that it was also in Karush’s 1939 master’s thesis. To this day you will see the theorem called the Kuhn-Tucker theorem, and you will also see it listed as the Karush-Kuhn-Tucker theorem. There are many such examples.
Tim Dettmers says
2018-01-15 at 22:49
There are many interesting examples indeed! Do you think this relates how past researchers communicated their work, or how “mature” their work is in general (master thesis vs full researchers).
2017-11-15 at 08:01
Hi, just found this blog, great stuff! Just a minor point – “Communication can be important even after publication. Just look at Immanuel Kant’s work, which is probably the most important philosophical work, yet it was not read for some time because nobody understood his ideas.” I find that very strange, not a good example at all. “Probably the most important philosophical work” – I don’t know what that’s based on. “Arguably”, arguably, but ‘probably’?! I’ve never heard anyone claim that. It’s news to me that Kant wasn’t read for some time. Whatever “some time” means. But I don’t think that’s right at all. And “nobody understood his ideas” is even more murky. (There’s not even a single thing you could point to and call “an understanding of his ideas”, i.e. there are a wide range of interpretations, even to this day. What one person calls an understanding, to another is gross misunderstanding, etc.) His Critiques have a repellent, almost impenetrable style, granted, maybe that’s what you meant. p.s. Gauss invented the FFT, apparently, though it seems he never told anyone, not sure how much credit he deserves. I kept expecting to see his name on these pages in that connection. 🙂
2017-11-16 at 22:49
I am talking about the “Critique of Pure Reason” here. Kant published it, and it was poorly received because people could not understand it. He rewrote it 6 years later, and suddenly people could actually understand his points, which in turn could help other people understand. Through this Kant became the most talked-about philosopher during that time.
Karthikeyan Chittayil says
2017-09-30 at 07:53
Tim, I think you have a nice way of putting complex concepts in simple words, and elementary maths. Please keep it up. As you have brought it out, communication indeed is very important. Keep blogging !
2017-10-01 at 15:25
Thank you, that means a lot of me!
Yun Teng says
2017-09-28 at 03:16
Enlightening as always! The saying “Those who can, do, those who can’t, teach” has always bothered me. Because of that, I really liked your “Timing and Relational Effects” example with the PageRank, which showed that Person 2 was the most important, and even Person 3 had 0.2305 contribution. To me, Person 2 is like a mentor/advisor and Person 3 is an instructor with many students, both roles having significant impact in the real world.
2017-09-29 at 14:52
Indeed, I think this is a good way to think about Person 2 and Person 3.
Alison B Lowndes says
2017-09-18 at 12:59
I will read this in full when I get chance but just wanted to add that if I’d listened to my Supervisor I’d have researched neural networks on CPU! I didn’t listen to him – which is lucky – because he also told me I’d never be able to recognise features in histology images!? Its a tough world out there so you just have to learn to be humble and courageous at the same time. PS My Supervisor also told me to steer clear of your (ex) Supervisor! PPS We still want to hire you!
2017-09-25 at 15:12
Thanks for your comment, Alison. I really appreciate it! Indeed it can be messy with the wrong supervisor, but I must also say that it was a good experience for me since I learned a lot from that experience. With that, I will be able to make a better choice for my PhD advisor. So in the end, it was not so bad after all!
2017-09-18 at 01:26
Hmm in other fields a lot of credit is given to the original person who came up with it, even if it wasn’t used or popularized right away. Like in computer graphics you give credit to the mathematician who came up with quaternions even if (as far as I know) they weren’t used for years any where else. It was just some obscure math. Likewise the guy who came up with plate tectonics was considered a quack when it was introduced, yet years later when we accept it we give him credit (even if he couldn’t popularize it). I think in a sense the purpose of academia and universities is to go beyond what’s necessarily useful today, to explore the far off distance, even if it isn’t worth popularizing right now (because there’s no use for it).
My understanding of Deep Learning is a lot of it got popularized due to faster computing machines, in particular GPUs. Certainly I believe the person who implemented DL on GPUs deserves a lot of credit for it, but I wouldn’t dismiss people who came beforehand with ideas because they didn’t implement it right away. (Actually this is kind of inspiring me to take a look into who first decided to use quaternions in graphics to see interesting early things they may have done.)
I was thinking maybe you’re coming from more of a corporate standpoint, where all that matters to you is utilization. But even in the corporate world credit to obscure ideas is given. An example is Apple. Popularizing GUIs and what have you. But we still give credit to Xerox, and even in interviews Steve Jobs discusses this!
In your examples you give this idea about someone being unable to communicate their ideas to the world. That makes sense to me, if they couldn’t get it out and it remained so obscure that it only remained in their minds, they probably don’t deserve much credit (like you say there wouldn’t even be proof). But if someone gets a publication out, that is no longer obscure, and I would say that’s a worthy of credit assignment.
2017-09-18 at 11:34
You talk about who to credit for an idea. This blog post does not discuss this topic. This blog post discusses how the impact of the idea is distributed among people and thus how much credit people should receive.
Xerox, of course, should be credited with the idea of the GUI. It was their original research. But who gets how much credit for the impact the idea of a GUI had over time?
Communication can be important even after publication. Just look at Immanuel Kant’s work, which is probably the most important philosophical work, yet it was not read for some time because nobody understood his ideas. It was similar for the LSTM. People just could not understand the paper and thus the significance of LSTMs.
Note that all these are mere examples which do not yield any reliable knowledge. You can look at it with the scientific method from other disciplines too, and I think this would be a better way to contribute to this discussion.
For example, in social network analysis similar effects as I describe here are well known (central nodes in a network are strong even though their only merit is their network connectivity itself). You can see similar things in some games in game theory. This can be used to describe these effects mathematically and thus I believe these theories are better than using examples which have a hard time to prove an argument.
2017-09-18 at 14:22
Ah sorry, I think I misunderstood your blog post originally, thought you were dismissing original credit. Impact isn’t something I have thought about seriously, and I think the topic is something that could easily be brushed aside for the status quo with lazy statements like “impact isn’t something I have thought about seriously” or with hostility to change. So with that said I think it’s good you’re questioning credit assignment, even if you are met with a lot of hostility. So thank you.
I agree communication is important. I am very new to deep learning, and I find the initiatives within the field for improving communication to be extremely inspiring and helpful to me. Including your own work, especially your last blog post about research direction and computational efficiency. So thank you and I hope you continue to write.
Rein Halbersma says
2017-09-16 at 21:36
Nice post! You could also interpret the credit assignment problem as a bargaining game in which each player bargains over the deployment of its assets (ideas, communication, implementation) to create something of value. Applying the tools from cooperative game theory, I would expect a solution concept like the Shapley-value to emerge as a fair credit assignment. Linking pins such as communicators connecting different communities also have great value in such bargaining games.
2017-09-16 at 21:56
Thanks for your comment — this is a very interesting analogy! I think something like the Shapley-value and its problem fit this entire problem quite well and I would expect the solutions to be quite similar.
Leave a Reply Cancel reply
Your email address will not be published. Required fields are marked *
Save my name, email, and website in this browser for the next time I comment.
Notify me of follow-up comments by email.
Notify me of new posts by email.
- Search Search Please fill out this field.
What Is Credit?
Credit in lending and borrowing, other definitions of credit, what is a letter of credit, what is a credit limit, what is a line of credit, what is revolving credit, the bottom line.
- Credit & Debt
- Definitions A - M
Credit: What It Is and How It Works
:max_bytes(150000):strip_icc():format(webp)/Group1805-3b9f749674f0434184ef75020339bd35.jpg)
Skylar Clarine is a fact-checker and expert in personal finance with a range of experience including veterinary technology and film studies.
The word "credit" has many meanings in the financial world, but it most commonly refers to a contractual agreement in which a borrower receives a sum of money or something else of value and commits to repaying the lender at a later date, typically with interest.
Credit can also refer to the creditworthiness or credit history of an individual or a company—as in "she has good credit." In the world of accounting, it refers to a specific type of bookkeeping entry.
Key Takeaways
- Credit is typically defined as an agreement between a lender and a borrower.
- Credit can also refer to an individual's or a business's creditworthiness.
- In accounting, a credit is a type of bookkeeping entry, the opposite of which is a debit.
Investopedia / Sydney Saporito
Credit represents an agreement between a creditor (lender) and a borrower (debtor). The debtor promises to repay the lender, often with interest, or risk financial or legal penalties. Extending credit is a practice that goes back thousands of years, to the dawn of human civilization, according to the anthropologist David Graeber in his book Debt: The First 5000 Years.
There are many different forms of credit. Common examples include car loans, mortgages, personal loans, and lines of credit. Essentially, when the bank or other financial institution makes a loan, it "credits" money to the borrower, who must pay it back at a future date.
Credit cards may be the most ubiquitous example of credit today, allowing consumers to purchase just about anything on credit. The card-issuing bank serves as an intermediary between buyer and seller, paying the seller in full while extending credit to the buyer, who may repay the debt over time while incurring interest charges until it is fully paid off.
Similarly, if buyers receive products or services from a seller who doesn't require payment until later, that is a form of credit. For example, when a restaurant receives a truckload of produce from a wholesaler who will bill the restaurant for it a month later, the wholesaler is providing the restaurant owner with a form of credit.
"Credit" is also used as shorthand to describe the financial soundness of businesses or individuals. Someone who has good or excellent credit is considered less of a risk to lenders than someone with bad or poor credit.
Credit scores are one way that individuals are classified in terms of risk, not only by prospective lenders but also by insurance companies and, in some cases, landlords and employers. For example, the commonly used FICO score ranges from 300 to 850. Anyone with a score of 800 or higher is considered to have exceptional credit, 740 to 799 represents very good credit, 670 to 739 is good credit, 580 to 669 is fair, and a score of 579 or less is poor.
Companies are also judged by credit rating agencies , such as Moody's and Standard and Poor's, and given letter-grade scores, representing the agency's assessment of their financial strength. Those scores are closely watched by bond investors and can affect how much interest companies will have to offer in order to borrow money. Similarly, government securities are graded based on whether the issuing government or government agency is considered to have solid credit. U.S. Treasuries, for example, are backed by "full faith and credit of the United States."
In the world of accounting, "credit" has a more specialized meaning. It refers to a bookkeeping entry that records a decrease in assets or an increase in liabilities (as opposed to a debit , which does the opposite). For example, suppose that a retailer buys merchandise on credit. After the purchase, the company's inventory account increases by the amount of the purchase (via a debit), adding an asset to the company's balance sheet. However, its accounts payable field also increases by the amount of the purchase (via a credit), adding a liability.
Often used in international trade, a letter of credit is a letter from a bank guaranteeing that a seller will receive the full amount that it is due from a buyer by a certain agreed-upon date. If the buyer fails to do so, the bank is on the hook for the money.
A credit limit represents the maximum amount of credit that a lender (such as a credit card company) will extend (such as to a credit card holder). Once the borrower reaches the limit they are unable to make further purchases until they repay some portion of their balance. The term is also used in connection with lines of credit and buy now, pay later loans .
A line of credit refers to a loan from a bank or other financial institution that makes a certain amount of credit available to the borrower for them to draw on as needed, rather than taking all at once. One type is the home equity line of credit (HELOC) , which allows owners to borrow against the value of their home for renovations or other purposes.
Revolving credit involves a loan with no fixed end date—a credit card account being a good example. As long as the account is in good standing, the borrower can continue to borrow against it, up to whatever credit limit has been established. As the borrower makes payments toward the balance, the account is replenished. These kinds of loans are often referred to open-end credit . Mortgages and car loans, by contrast, are considered closed-end credit because they come to an end on a certain date.
The word "credit" has multiple meanings in personal and business finance. Most often it refers to the ability to buy a good or service and pay for it at some future point. Credit may be arranged directly between a buyer and seller or with the assistance of an intermediary, such as a bank or other financial institution. Credit serves a vital purpose in making the world of commerce run smoothly.
Experian. " What Is a Good Credit Score? "
- Accounting Explained With Brief History and Modern Job Requirements 1 of 51
- What Is the Accounting Equation, and How Do You Calculate It? 2 of 51
- What Is an Asset? Definition, Types, and Examples 3 of 51
- Liability: Definition, Types, Example, and Assets vs. Liabilities 4 of 51
- Equity Meaning: How It Works and How to Calculate It 5 of 51
- Revenue Definition, Formula, Calculation, and Examples 6 of 51
- Expense: Definition, Types, and How Expenses Are Recorded 7 of 51
- Current Assets vs. Noncurrent Assets: What's the Difference? 8 of 51
- What Is Accounting Theory in Financial Reporting? 9 of 51
- Accounting Principles Explained: How They Work, GAAP, IFRS 10 of 51
- Accounting Standard Definition: How It Works 11 of 51
- Accounting Convention: Definition, Methods, and Applications 12 of 51
- What Are Accounting Policies and How Are They Used? With Examples 13 of 51
- How Are Principles-Based and Rules-Based Accounting Different? 14 of 51
- What Are Accounting Methods? Definition, Types, and Example 15 of 51
- What Is Accrual Accounting, and How Does It Work? 16 of 51
- Cash Accounting Definition, Example & Limitations 17 of 51
- Accrual Accounting vs. Cash Basis Accounting: What's the Difference? 18 of 51
- Financial Accounting Standards Board (FASB): Definition and How It Works 19 of 51
- Generally Accepted Accounting Principles (GAAP): Definition, Standards and Rules 20 of 51
- What Are International Financial Reporting Standards (IFRS)? 21 of 51
- IFRS vs. GAAP: What's the Difference? 22 of 51
- How Does US Accounting Differ From International Accounting? 23 of 51
- Cash Flow Statement: What It Is and Examples 24 of 51
- Breaking Down The Balance Sheet 25 of 51
- Income Statement: How to Read and Use It 26 of 51
- What Does an Accountant Do? 27 of 51
- Financial Accounting Meaning, Principles, and Why It Matters 28 of 51
- How Does Financial Accounting Help Decision-Making? 29 of 51
- Corporate Finance Definition and Activities 30 of 51
- How Financial Accounting Differs From Managerial Accounting 31 of 51
- Cost Accounting: Definition and Types With Examples 32 of 51
- Certified Public Accountant: What the CPA Credential Means 33 of 51
- What Is a Chartered Accountant (CA) and What Do They Do? 34 of 51
- Accountant vs. Financial Planner: What's the Difference? 35 of 51
- Auditor: What It Is, 4 Types, and Qualifications 36 of 51
- Audit: What It Means in Finance and Accounting, and 3 Main Types 37 of 51
- Tax Accounting: Definition, Types, vs. Financial Accounting 38 of 51
- Forensic Accounting: What It Is, How It's Used 39 of 51
- Chart of Accounts (COA) Definition, How It Works, and Example 40 of 51
- What Is a Journal in Accounting, Investing, and Trading? 41 of 51
- Double Entry: What It Means in Accounting and How It's Used 42 of 51
- Debit: Definition and Relationship to Credit 43 of 51
- Credit: What It Is and How It Works 44 of 51
- Closing Entry 45 of 51
- What Is an Invoice? It's Parts and Why They Are Important 46 of 51
- 6 Components of an Accounting Information System (AIS) 47 of 51
- Inventory Accounting: Definition, How It Works, Advantages 48 of 51
- Last In, First Out (LIFO): The Inventory Cost Method Explained 49 of 51
- The FIFO Method: First In, First Out 50 of 51
- Average Cost Method: Definition and Formula with Example 51 of 51
:max_bytes(150000):strip_icc():format(webp)/GettyImages-172163302-190cb9289927436cbe0679cb4138c085.jpg)
- Terms of Service
- Editorial Policy
- Privacy Policy
- Your Privacy Choices

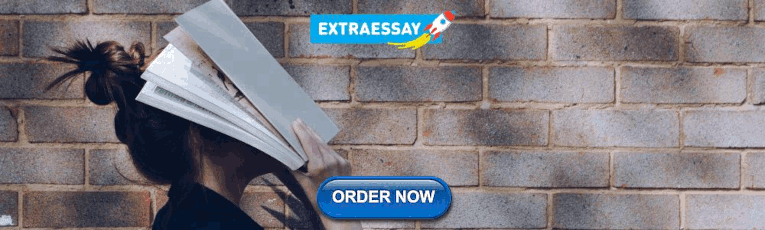
Understanding Assignments
What this handout is about.
The first step in any successful college writing venture is reading the assignment. While this sounds like a simple task, it can be a tough one. This handout will help you unravel your assignment and begin to craft an effective response. Much of the following advice will involve translating typical assignment terms and practices into meaningful clues to the type of writing your instructor expects. See our short video for more tips.
Basic beginnings
Regardless of the assignment, department, or instructor, adopting these two habits will serve you well :
- Read the assignment carefully as soon as you receive it. Do not put this task off—reading the assignment at the beginning will save you time, stress, and problems later. An assignment can look pretty straightforward at first, particularly if the instructor has provided lots of information. That does not mean it will not take time and effort to complete; you may even have to learn a new skill to complete the assignment.
- Ask the instructor about anything you do not understand. Do not hesitate to approach your instructor. Instructors would prefer to set you straight before you hand the paper in. That’s also when you will find their feedback most useful.
Assignment formats
Many assignments follow a basic format. Assignments often begin with an overview of the topic, include a central verb or verbs that describe the task, and offer some additional suggestions, questions, or prompts to get you started.
An Overview of Some Kind
The instructor might set the stage with some general discussion of the subject of the assignment, introduce the topic, or remind you of something pertinent that you have discussed in class. For example:
“Throughout history, gerbils have played a key role in politics,” or “In the last few weeks of class, we have focused on the evening wear of the housefly …”
The Task of the Assignment
Pay attention; this part tells you what to do when you write the paper. Look for the key verb or verbs in the sentence. Words like analyze, summarize, or compare direct you to think about your topic in a certain way. Also pay attention to words such as how, what, when, where, and why; these words guide your attention toward specific information. (See the section in this handout titled “Key Terms” for more information.)
“Analyze the effect that gerbils had on the Russian Revolution”, or “Suggest an interpretation of housefly undergarments that differs from Darwin’s.”
Additional Material to Think about
Here you will find some questions to use as springboards as you begin to think about the topic. Instructors usually include these questions as suggestions rather than requirements. Do not feel compelled to answer every question unless the instructor asks you to do so. Pay attention to the order of the questions. Sometimes they suggest the thinking process your instructor imagines you will need to follow to begin thinking about the topic.
“You may wish to consider the differing views held by Communist gerbils vs. Monarchist gerbils, or Can there be such a thing as ‘the housefly garment industry’ or is it just a home-based craft?”
These are the instructor’s comments about writing expectations:
“Be concise”, “Write effectively”, or “Argue furiously.”
Technical Details
These instructions usually indicate format rules or guidelines.
“Your paper must be typed in Palatino font on gray paper and must not exceed 600 pages. It is due on the anniversary of Mao Tse-tung’s death.”
The assignment’s parts may not appear in exactly this order, and each part may be very long or really short. Nonetheless, being aware of this standard pattern can help you understand what your instructor wants you to do.
Interpreting the assignment
Ask yourself a few basic questions as you read and jot down the answers on the assignment sheet:
Why did your instructor ask you to do this particular task?
Who is your audience.
- What kind of evidence do you need to support your ideas?
What kind of writing style is acceptable?
- What are the absolute rules of the paper?
Try to look at the question from the point of view of the instructor. Recognize that your instructor has a reason for giving you this assignment and for giving it to you at a particular point in the semester. In every assignment, the instructor has a challenge for you. This challenge could be anything from demonstrating an ability to think clearly to demonstrating an ability to use the library. See the assignment not as a vague suggestion of what to do but as an opportunity to show that you can handle the course material as directed. Paper assignments give you more than a topic to discuss—they ask you to do something with the topic. Keep reminding yourself of that. Be careful to avoid the other extreme as well: do not read more into the assignment than what is there.
Of course, your instructor has given you an assignment so that they will be able to assess your understanding of the course material and give you an appropriate grade. But there is more to it than that. Your instructor has tried to design a learning experience of some kind. Your instructor wants you to think about something in a particular way for a particular reason. If you read the course description at the beginning of your syllabus, review the assigned readings, and consider the assignment itself, you may begin to see the plan, purpose, or approach to the subject matter that your instructor has created for you. If you still aren’t sure of the assignment’s goals, try asking the instructor. For help with this, see our handout on getting feedback .
Given your instructor’s efforts, it helps to answer the question: What is my purpose in completing this assignment? Is it to gather research from a variety of outside sources and present a coherent picture? Is it to take material I have been learning in class and apply it to a new situation? Is it to prove a point one way or another? Key words from the assignment can help you figure this out. Look for key terms in the form of active verbs that tell you what to do.
Key Terms: Finding Those Active Verbs
Here are some common key words and definitions to help you think about assignment terms:
Information words Ask you to demonstrate what you know about the subject, such as who, what, when, where, how, and why.
- define —give the subject’s meaning (according to someone or something). Sometimes you have to give more than one view on the subject’s meaning
- describe —provide details about the subject by answering question words (such as who, what, when, where, how, and why); you might also give details related to the five senses (what you see, hear, feel, taste, and smell)
- explain —give reasons why or examples of how something happened
- illustrate —give descriptive examples of the subject and show how each is connected with the subject
- summarize —briefly list the important ideas you learned about the subject
- trace —outline how something has changed or developed from an earlier time to its current form
- research —gather material from outside sources about the subject, often with the implication or requirement that you will analyze what you have found
Relation words Ask you to demonstrate how things are connected.
- compare —show how two or more things are similar (and, sometimes, different)
- contrast —show how two or more things are dissimilar
- apply—use details that you’ve been given to demonstrate how an idea, theory, or concept works in a particular situation
- cause —show how one event or series of events made something else happen
- relate —show or describe the connections between things
Interpretation words Ask you to defend ideas of your own about the subject. Do not see these words as requesting opinion alone (unless the assignment specifically says so), but as requiring opinion that is supported by concrete evidence. Remember examples, principles, definitions, or concepts from class or research and use them in your interpretation.
- assess —summarize your opinion of the subject and measure it against something
- prove, justify —give reasons or examples to demonstrate how or why something is the truth
- evaluate, respond —state your opinion of the subject as good, bad, or some combination of the two, with examples and reasons
- support —give reasons or evidence for something you believe (be sure to state clearly what it is that you believe)
- synthesize —put two or more things together that have not been put together in class or in your readings before; do not just summarize one and then the other and say that they are similar or different—you must provide a reason for putting them together that runs all the way through the paper
- analyze —determine how individual parts create or relate to the whole, figure out how something works, what it might mean, or why it is important
- argue —take a side and defend it with evidence against the other side
More Clues to Your Purpose As you read the assignment, think about what the teacher does in class:
- What kinds of textbooks or coursepack did your instructor choose for the course—ones that provide background information, explain theories or perspectives, or argue a point of view?
- In lecture, does your instructor ask your opinion, try to prove their point of view, or use keywords that show up again in the assignment?
- What kinds of assignments are typical in this discipline? Social science classes often expect more research. Humanities classes thrive on interpretation and analysis.
- How do the assignments, readings, and lectures work together in the course? Instructors spend time designing courses, sometimes even arguing with their peers about the most effective course materials. Figuring out the overall design to the course will help you understand what each assignment is meant to achieve.
Now, what about your reader? Most undergraduates think of their audience as the instructor. True, your instructor is a good person to keep in mind as you write. But for the purposes of a good paper, think of your audience as someone like your roommate: smart enough to understand a clear, logical argument, but not someone who already knows exactly what is going on in your particular paper. Remember, even if the instructor knows everything there is to know about your paper topic, they still have to read your paper and assess your understanding. In other words, teach the material to your reader.
Aiming a paper at your audience happens in two ways: you make decisions about the tone and the level of information you want to convey.
- Tone means the “voice” of your paper. Should you be chatty, formal, or objective? Usually you will find some happy medium—you do not want to alienate your reader by sounding condescending or superior, but you do not want to, um, like, totally wig on the man, you know? Eschew ostentatious erudition: some students think the way to sound academic is to use big words. Be careful—you can sound ridiculous, especially if you use the wrong big words.
- The level of information you use depends on who you think your audience is. If you imagine your audience as your instructor and they already know everything you have to say, you may find yourself leaving out key information that can cause your argument to be unconvincing and illogical. But you do not have to explain every single word or issue. If you are telling your roommate what happened on your favorite science fiction TV show last night, you do not say, “First a dark-haired white man of average height, wearing a suit and carrying a flashlight, walked into the room. Then a purple alien with fifteen arms and at least three eyes turned around. Then the man smiled slightly. In the background, you could hear a clock ticking. The room was fairly dark and had at least two windows that I saw.” You also do not say, “This guy found some aliens. The end.” Find some balance of useful details that support your main point.
You’ll find a much more detailed discussion of these concepts in our handout on audience .
The Grim Truth
With a few exceptions (including some lab and ethnography reports), you are probably being asked to make an argument. You must convince your audience. It is easy to forget this aim when you are researching and writing; as you become involved in your subject matter, you may become enmeshed in the details and focus on learning or simply telling the information you have found. You need to do more than just repeat what you have read. Your writing should have a point, and you should be able to say it in a sentence. Sometimes instructors call this sentence a “thesis” or a “claim.”
So, if your instructor tells you to write about some aspect of oral hygiene, you do not want to just list: “First, you brush your teeth with a soft brush and some peanut butter. Then, you floss with unwaxed, bologna-flavored string. Finally, gargle with bourbon.” Instead, you could say, “Of all the oral cleaning methods, sandblasting removes the most plaque. Therefore it should be recommended by the American Dental Association.” Or, “From an aesthetic perspective, moldy teeth can be quite charming. However, their joys are short-lived.”
Convincing the reader of your argument is the goal of academic writing. It doesn’t have to say “argument” anywhere in the assignment for you to need one. Look at the assignment and think about what kind of argument you could make about it instead of just seeing it as a checklist of information you have to present. For help with understanding the role of argument in academic writing, see our handout on argument .
What kind of evidence do you need?
There are many kinds of evidence, and what type of evidence will work for your assignment can depend on several factors–the discipline, the parameters of the assignment, and your instructor’s preference. Should you use statistics? Historical examples? Do you need to conduct your own experiment? Can you rely on personal experience? See our handout on evidence for suggestions on how to use evidence appropriately.
Make sure you are clear about this part of the assignment, because your use of evidence will be crucial in writing a successful paper. You are not just learning how to argue; you are learning how to argue with specific types of materials and ideas. Ask your instructor what counts as acceptable evidence. You can also ask a librarian for help. No matter what kind of evidence you use, be sure to cite it correctly—see the UNC Libraries citation tutorial .
You cannot always tell from the assignment just what sort of writing style your instructor expects. The instructor may be really laid back in class but still expect you to sound formal in writing. Or the instructor may be fairly formal in class and ask you to write a reflection paper where you need to use “I” and speak from your own experience.
Try to avoid false associations of a particular field with a style (“art historians like wacky creativity,” or “political scientists are boring and just give facts”) and look instead to the types of readings you have been given in class. No one expects you to write like Plato—just use the readings as a guide for what is standard or preferable to your instructor. When in doubt, ask your instructor about the level of formality they expect.
No matter what field you are writing for or what facts you are including, if you do not write so that your reader can understand your main idea, you have wasted your time. So make clarity your main goal. For specific help with style, see our handout on style .
Technical details about the assignment
The technical information you are given in an assignment always seems like the easy part. This section can actually give you lots of little hints about approaching the task. Find out if elements such as page length and citation format (see the UNC Libraries citation tutorial ) are negotiable. Some professors do not have strong preferences as long as you are consistent and fully answer the assignment. Some professors are very specific and will deduct big points for deviations.
Usually, the page length tells you something important: The instructor thinks the size of the paper is appropriate to the assignment’s parameters. In plain English, your instructor is telling you how many pages it should take for you to answer the question as fully as you are expected to. So if an assignment is two pages long, you cannot pad your paper with examples or reword your main idea several times. Hit your one point early, defend it with the clearest example, and finish quickly. If an assignment is ten pages long, you can be more complex in your main points and examples—and if you can only produce five pages for that assignment, you need to see someone for help—as soon as possible.
Tricks that don’t work
Your instructors are not fooled when you:
- spend more time on the cover page than the essay —graphics, cool binders, and cute titles are no replacement for a well-written paper.
- use huge fonts, wide margins, or extra spacing to pad the page length —these tricks are immediately obvious to the eye. Most instructors use the same word processor you do. They know what’s possible. Such tactics are especially damning when the instructor has a stack of 60 papers to grade and yours is the only one that low-flying airplane pilots could read.
- use a paper from another class that covered “sort of similar” material . Again, the instructor has a particular task for you to fulfill in the assignment that usually relates to course material and lectures. Your other paper may not cover this material, and turning in the same paper for more than one course may constitute an Honor Code violation . Ask the instructor—it can’t hurt.
- get all wacky and “creative” before you answer the question . Showing that you are able to think beyond the boundaries of a simple assignment can be good, but you must do what the assignment calls for first. Again, check with your instructor. A humorous tone can be refreshing for someone grading a stack of papers, but it will not get you a good grade if you have not fulfilled the task.
Critical reading of assignments leads to skills in other types of reading and writing. If you get good at figuring out what the real goals of assignments are, you are going to be better at understanding the goals of all of your classes and fields of study.
You may reproduce it for non-commercial use if you use the entire handout and attribute the source: The Writing Center, University of North Carolina at Chapel Hill
Make a Gift
- Solutions for
- >>
Credit Assignment
Support and procedures.
Gain Fiscal advantages trough the assignment of bad debts
CRIBIS Credit Management, the CRIF’s Group business specializing in credit management and credit recovery services, draw up a procedural process that allows you to reduce the tax impact of your debts.
- Reduce the economic losses and save money with the diminution of the balance sheet-profit.
- Gain legal advantages , in respect to the law related to credit assignment
- Cut management costs on non-performing loans by employing the company internal recourses in a different way.
- Write a coherent balance sheet that represents the economic and patrimonial situation and satisfies what is required in terms of veracity and transparency in book entries.
Recommended for
Invoice Trading
How does it work?
Taking away the losses for missed credits, guarantees immediate fiscal saving, thanks to the balance income-sheet profit loss. CRIBIS Credit Management , a Crif’s Group society specialized in credit management and debt collection services, together with the most qualified financial intermediaries of the Ufficio Italiano Cambi , has come up with a procedure that allows companies to reduce the fiscal impact of irrevocable credits .
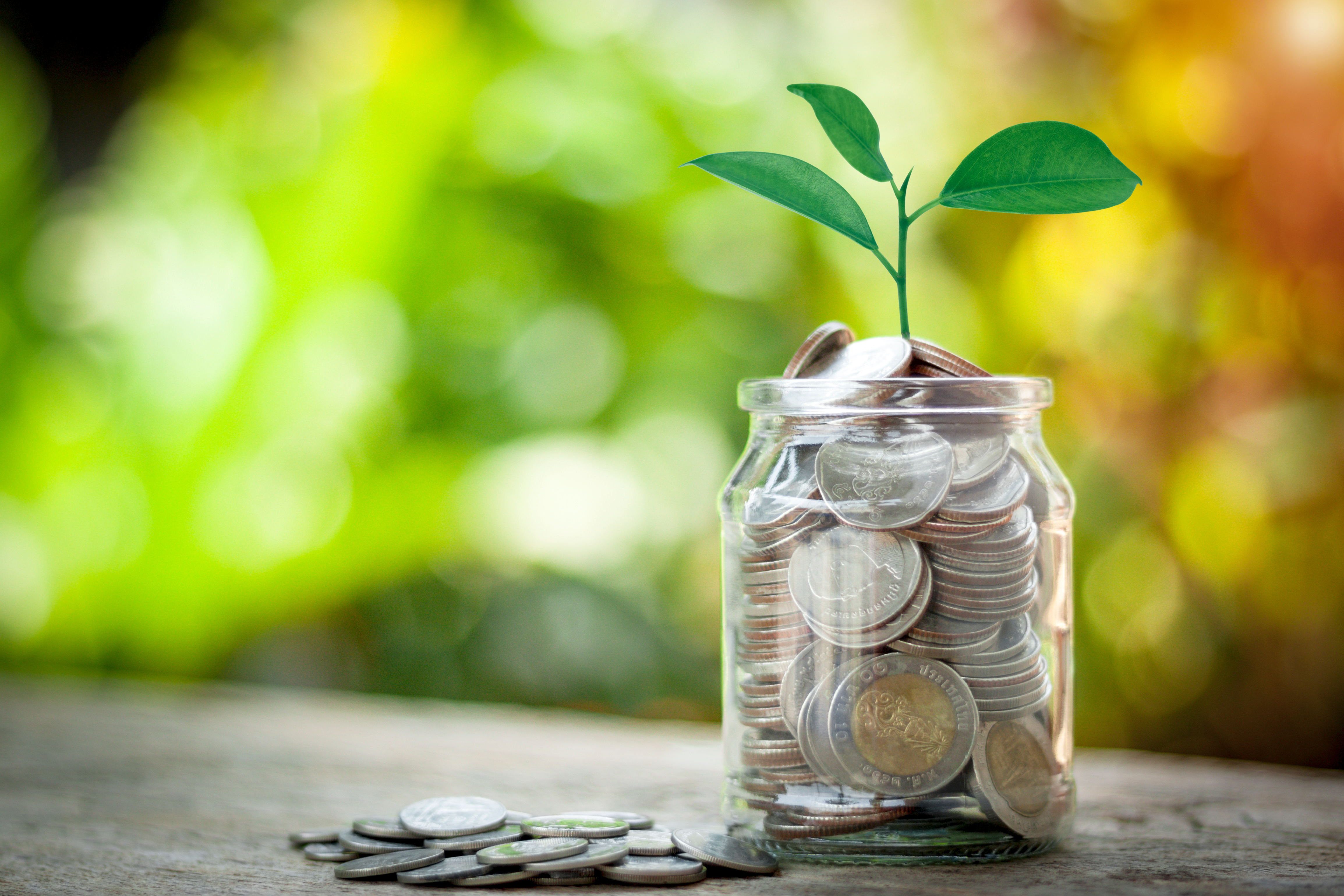
Via a non-recourse assignment, the transferer can take at cost in the income statement, the import that comes from the difference between the credit nominal value and the assignment value.
Whenever the company decides to go with the non-recourse assignment, the business must define the existence and the exact amount of the fund.
For company of people or sole proprietorship, the money saved varies depending on the taxable income (remember that irpef is tax brackets)
If the capital company goes to the loss of assigned receivables, with an empty bad debt provisions, the company has the chance to save 24% (the IRES rate) on taxes related to the amount of losses.
Do you have any questions or want to receive a quotation?
In Evidence
CRIBIS Radar
With CRIBIS Radar you can discover all best solutions and the regulations of the subsidized financing with support throughout the process and access to financing
CRIBIS CASH
Cribis Cash helps companies collect business credits right away and gain immediate liquidity thanks to business information and advance collection of invoices.
Tax Credit Place
Buy and sell fiscal credits in the first digital italian market.
Not registered yet? Register now
* Look at the complete archive of our reports * Contact support by email * Request an offer for other products more quickly * Change your registration information
Password Recovery
Already registered? Log In
We invite yoy to consult our Privacy Policy to gain more information about the treatment of your personal data before filling the form o give consents to the treatments in question
to process contact requests
The provision of the above data is necessary and does not require consent as the processing of the same is legitimized pursuant to art. 6 lett. b) of EU Regulation 679/2016. The user is free not to provide such data but in this case we will not be able to follow up on his requests. We invite you to consult our Privacy Policy for more information on the processing of your data.
for commercial communication purposes
The consent to the processing of the above data for marketing purposes, commercial communication, sending newsletters, carrying out market research on CRIBIS D&B S.r.l. products or services, is optional. Such communications may take place through the use of traditional tools (telephone with operator / paper mail) or automated (email, fax, sms etc.) also through specifically appointed external companies. We invite you to consult our Privacy Policy for more information on the processing of your data.
I agree to be contacted for marketing, commercial communication, market research purposes on the products or services of CRIBIS D&B using traditional methods (paper mail, telephone with operator) and with automated tools (sms, email, fax, etc.)
I agree to be contacted for marketing purposes, commercial communication, carrying out market research on the products or services of other Crif companies using traditional methods (paper mail, telephone with operator) and with automated tools (sms, email, fax, etc.)
I agree to receive newsletters
Search Results
Learn more.
Watch CBS News
We may receive commissions from some links to products on this page. Promotions are subject to availability and retailer terms.
Can I wipe my credit card debt without paying?
By Angelica Leicht
Edited By Matt Richardson
May 9, 2024 / 12:06 PM EDT / CBS News
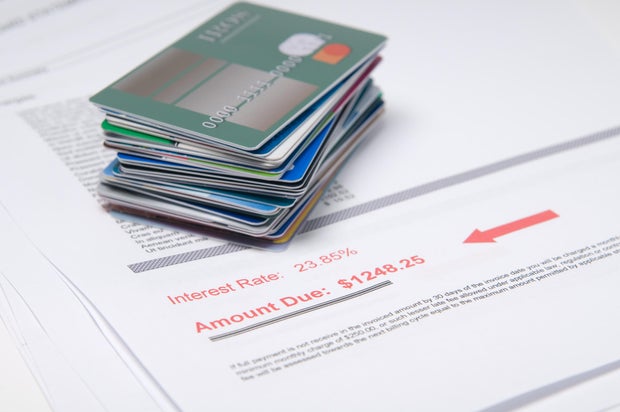
Many Americans are feeling the financial squeeze from multiple directions right now. For starters, stubbornly high inflation , which is currently running at around 3.5%, has driven up the cost of essentials like food, housing and energy. And, the Federal Reserve has been keeping its benchmark rate at a 23-year high in an effort to get inflation under control, which is causing consumer borrowing rates to be elevated, too. This high-rate environment has, in turn, made borrowing more expensive for things like mortgages , auto loans and credit cards .
For households that are already struggling to make ends meet, this combination of high inflation and elevated interest rates can have a severe impact . When the bills keep piling up but income isn't keeping pace, it can lead you to turn to credit cards as a stopgap just to cover basic living expenses. And, before you know it, you've racked up substantial credit card balances that become increasingly difficult to pay down as the interest charges compound.
It's no wonder that in this difficult economic environment, those who are saddled with mounting credit card debt may start looking for any lifeline or innovative way to get out from under that burden. And, some may even go so far as to explore the possibility of wiping out their credit card debt entirely without paying what they owe. But is that really possible? And, if so, what are the potential consequences?
Need extra help with your credit card debt? Compare your top debt relief options here .
The short answer is yes, there are a couple of ways you can technically get out of paying your credit card debt entirely. However, these options come with major downsides and should really only be considered as an absolute last resort. That said, your options for doing so include:
Filing for bankruptcy
The most straightforward way to have your credit card debt legally forgiven is to file for bankruptcy . When you file for Chapter 7 bankruptcy, commonly known as liquidation bankruptcy, your assets above certain exempt amounts are sold off to repay as much of your debt as possible. Any remaining unsecured debts, like credit cards, are then discharged, meaning you are no longer legally obligated to pay them.
While this allows you to start with a clean slate, the bankruptcy itself will remain on your credit report for seven to 10 years, making it extremely difficult to get approved for new credit or loans during that time. It can also limit your housing options or make it harder to get hired for certain jobs. Those types of consequences should make bankruptcy the last option for many people.
Find out what the right debt relief solution is for you now .
Opting for debt settlement or debt forgiveness
Another potential option to wipe out credit card debt without paying the full amount is to negotiate what's known as a debt settlement with your creditors. In this process, you stop making monthly payments and instead negotiate with the credit card companies — either directly or through a debt settlement company representing you — to pay a lump sum that is less than the full balance in exchange for them forgiving the remaining amount.
This option won't allow you to wipe away your credit card debt completely without paying anything out of pocket. That said, creditors are sometimes willing to accept these reduced payoff amounts, especially if you demonstrate a true inability to pay and the debt has gone into default status.
The catch is that the forgiven portion of the debt is treated as taxable income , so you'll likely owe income taxes on that portion of your debt. You'll also typically see a negative impact on your credit score, which will make borrowing more difficult and expensive in the future.
Other options for wiping your credit card debt
Outside of bankruptcy or debt settlement, there are really no other ways to completely wipe away credit card debt without paying. Making minimum payments and slowly chipping away at the balance is the norm for most people in debt, and that may be the best option in many situations.
However, there are some alternatives that can provide temporary relief and get you on a path to paying off the debt in full. These include:
- Debt management plans: When you enroll in a debt management plan , the debt relief agency you work with may be able to negotiate lower interest rates, waived fees or alternate payment plans with creditors on your behalf. This can make the debt more manageable to pay off in full.
- Debt consolidation loans: Debt consolidation loans allow you to combine multiple credit card balances into one new fixed-rate loan, ideally with a lower interest rate than the cards. You still pay the full principal, but the savings on interest can speed up the payoff process.
- Balance transfer cards: Balance transfer cards with 0% intro APR promotions allow you to move your debt to a new card without interest charges for the first 12 to 18 months. This interest-free window allows more of your payment to go to the principal.
The bottom line
Wiping out credit card debt entirely without any consequences or obligation to eventually pay is essentially impossible outside of bankruptcy. While that can certainly provide a fresh start, it comes with immense costs and negative impacts that can take years to recover from.
For most people, finding ways to responsibly pay off their credit card debt over time through a combination of budgeting, negotiating with creditors, debt consolidation and measured use of balance transfers is a better choice. It preserves your credit rating and avoids the financial implications of options like bankruptcy or debt settlement. And, with some diligence and perseverance, that nagging credit card debt can be overcome through commitment rather than avoidance.
Angelica Leicht is senior editor for CBS' Moneywatch: Managing Your Money, where she writes and edits articles on a range of personal finance topics. Angelica previously held editing roles at The Simple Dollar, Interest, HousingWire and other financial publications.
More from CBS News
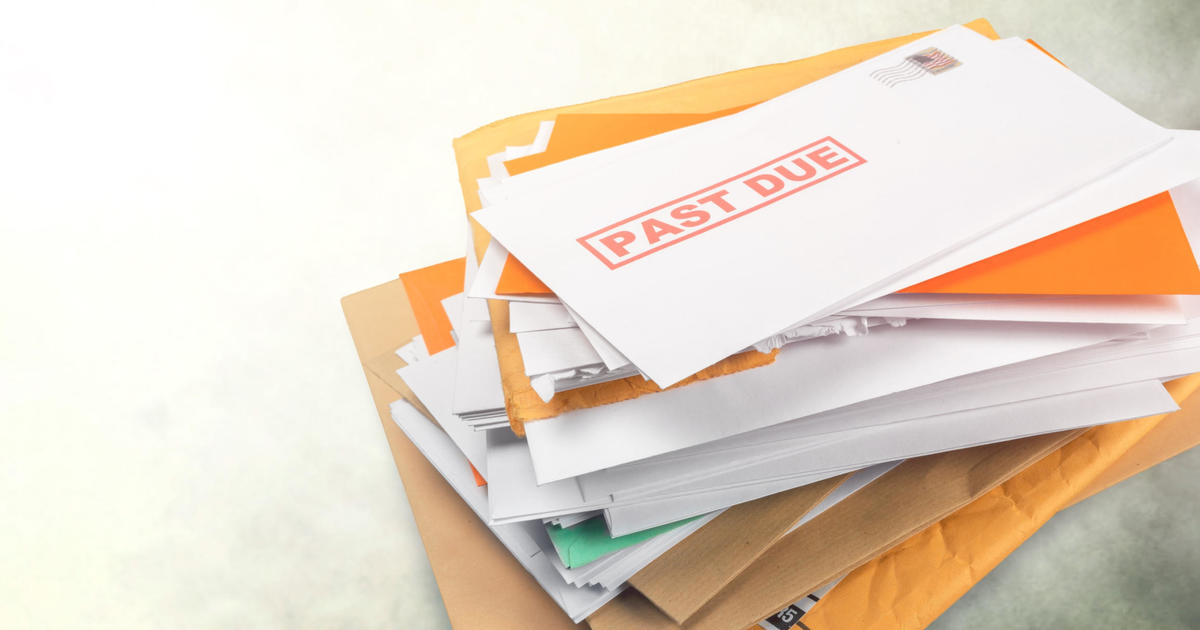
What is the lowest amount debt collectors will settle for? What experts say
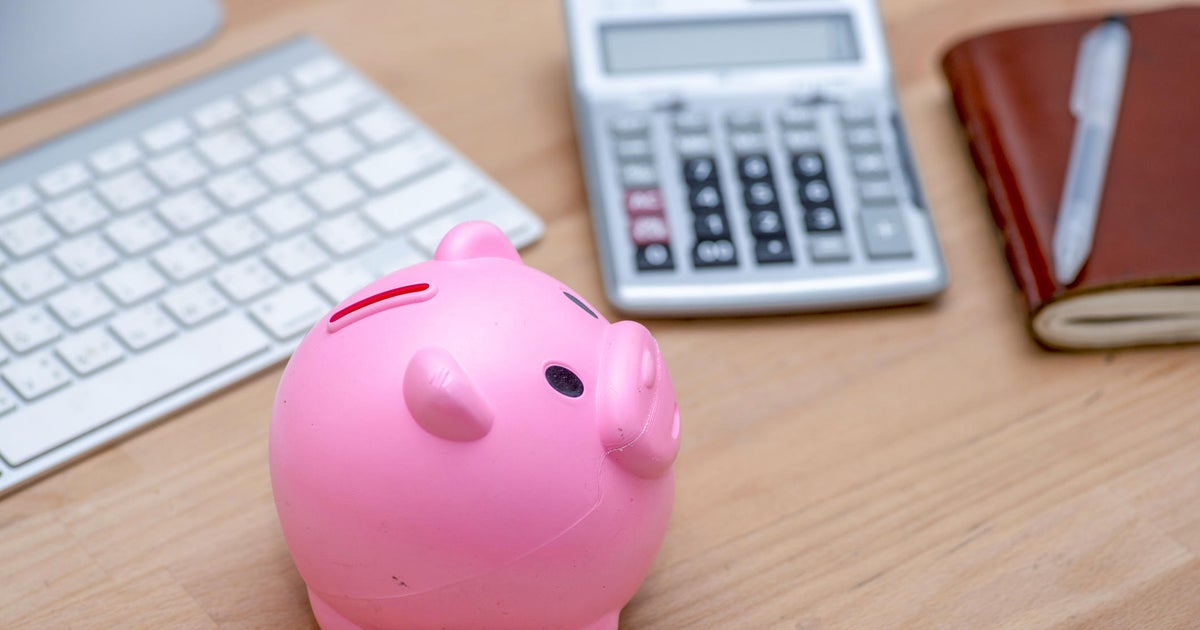
Why you should open a 6-month CD this May
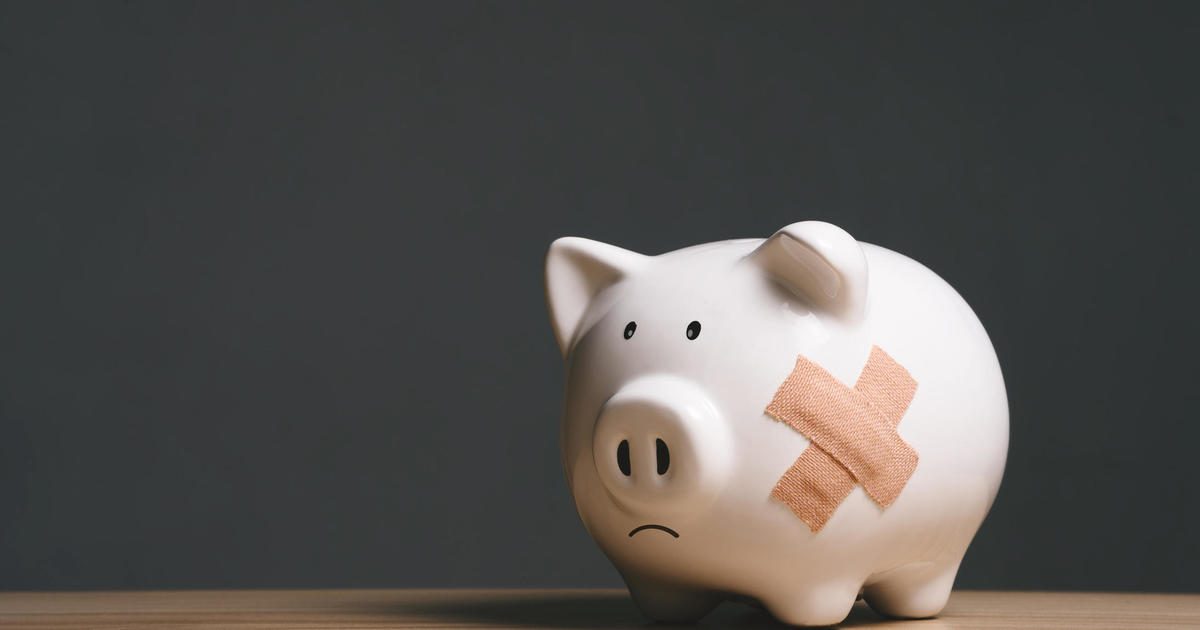
How much does it cost to file for bankruptcy?
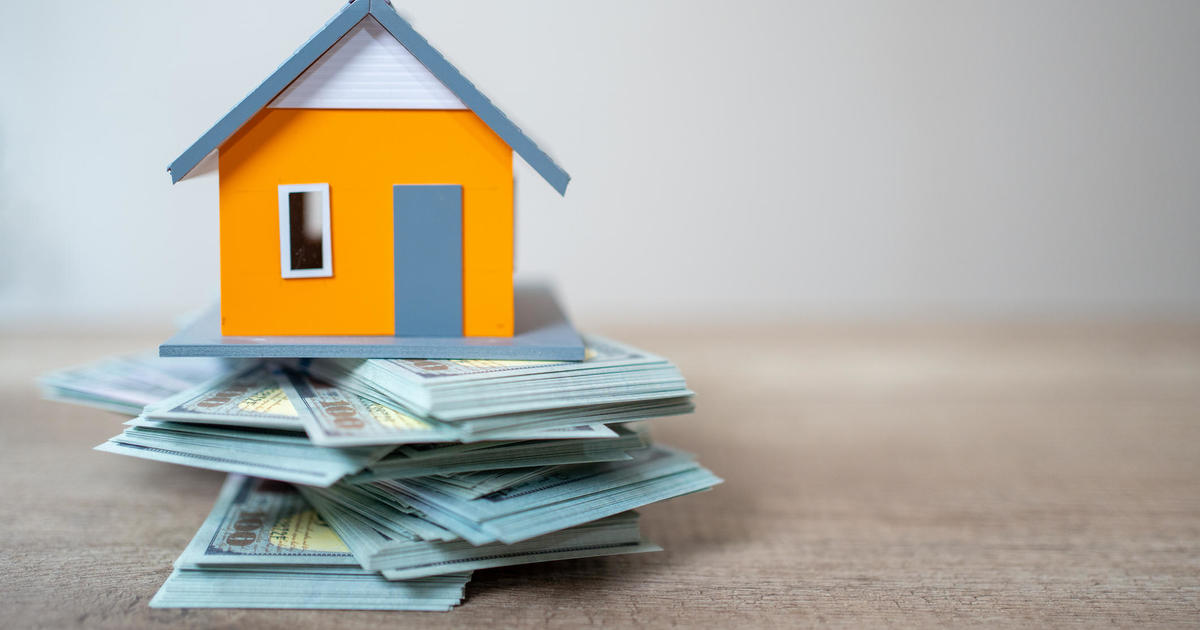
Is a $40,000 home equity loan worth it?
US judge halts rule capping credit card late fees at $8
- Medium Text
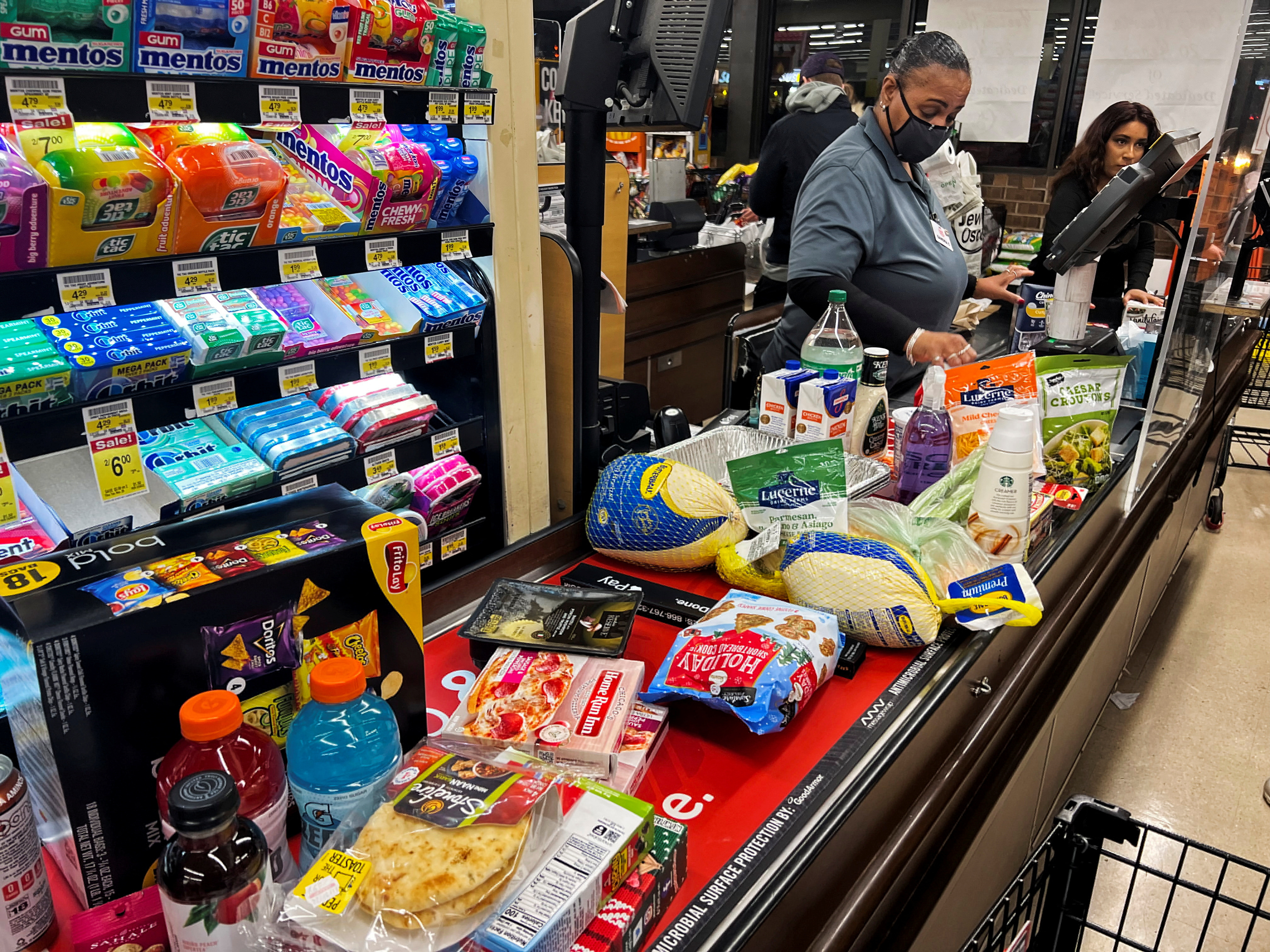
Sign up here.
Reporting by Nate Raymond in Boston; Editing by Leslie Adler, David Gregorio, Gerry Doyle and William Mallard
Our Standards: The Thomson Reuters Trust Principles. New Tab , opens new tab

Thomson Reuters
Nate Raymond reports on the federal judiciary and litigation. He can be reached at [email protected].
Read Next / Editor's Picks
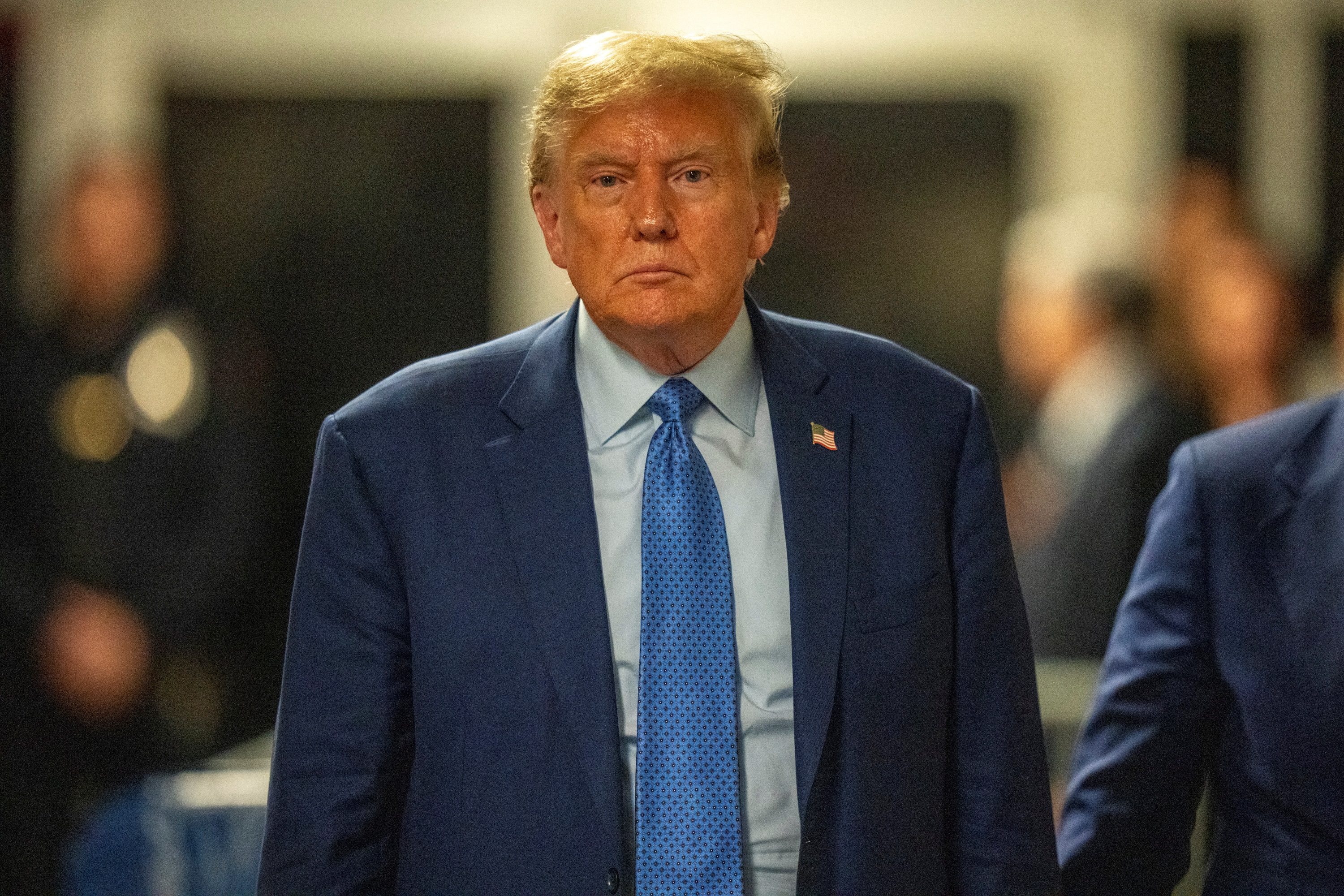
Industry Insight Chevron
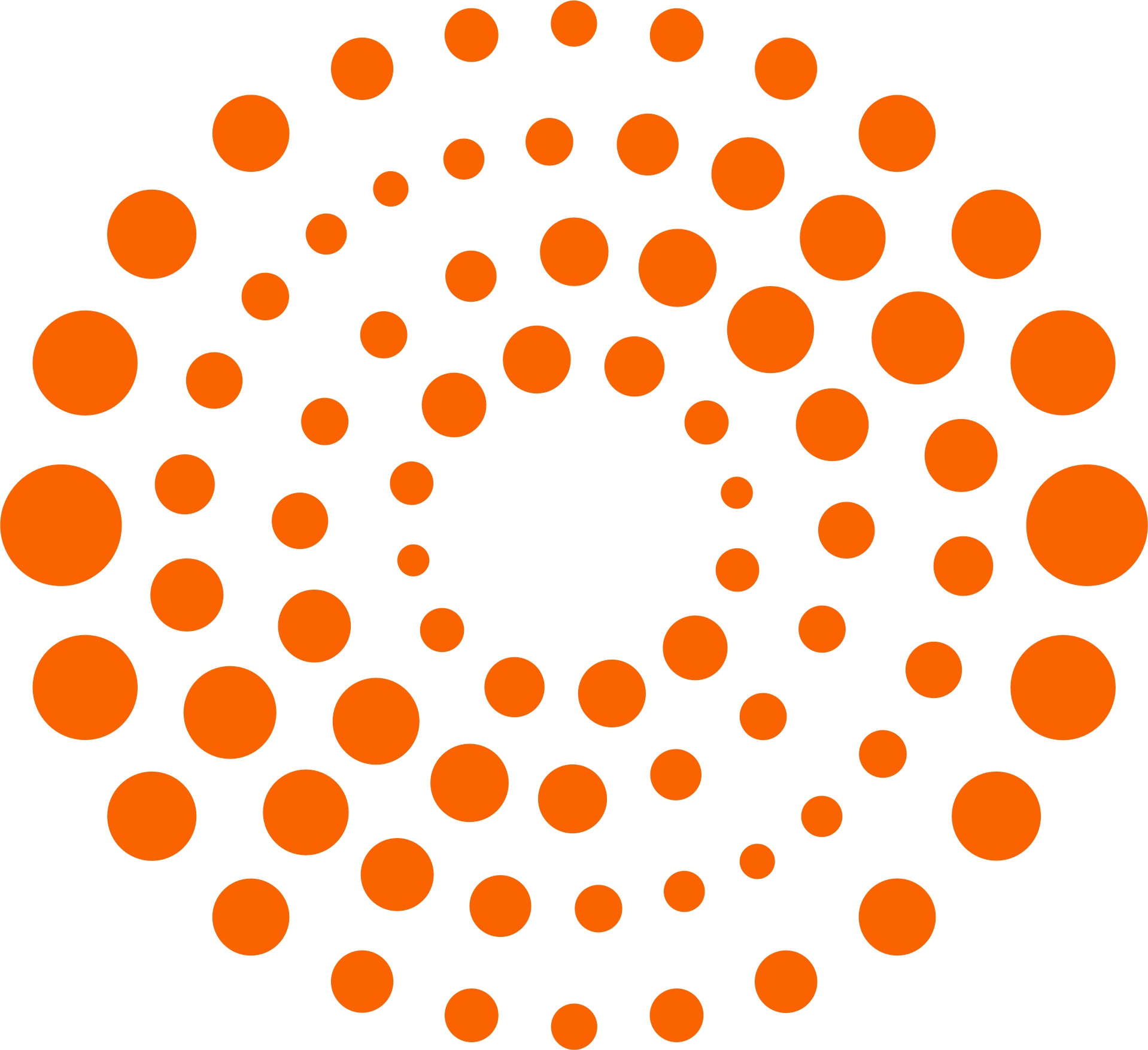
Mike Scarcella, David Thomas

Karen Sloan

Henry Engler

Diana Novak Jones
Help | Advanced Search
Computer Science > Machine Learning
Title: a survey of temporal credit assignment in deep reinforcement learning.
Abstract: The Credit Assignment Problem (CAP) refers to the longstanding challenge of Reinforcement Learning (RL) agents to associate actions with their long-term consequences. Solving the CAP is a crucial step towards the successful deployment of RL in the real world since most decision problems provide feedback that is noisy, delayed, and with little or no information about the causes. These conditions make it hard to distinguish serendipitous outcomes from those caused by informed decision-making. However, the mathematical nature of credit and the CAP remains poorly understood and defined. In this survey, we review the state of the art of Temporal Credit Assignment (CA) in deep RL. We propose a unifying formalism for credit that enables equitable comparisons of state of the art algorithms and improves our understanding of the trade-offs between the various methods. We cast the CAP as the problem of learning the influence of an action over an outcome from a finite amount of experience. We discuss the challenges posed by delayed effects, transpositions, and a lack of action influence, and analyse how existing methods aim to address them. Finally, we survey the protocols to evaluate a credit assignment method, and suggest ways to diagnoses the sources of struggle for different credit assignment methods. Overall, this survey provides an overview of the field for new-entry practitioners and researchers, it offers a coherent perspective for scholars looking to expedite the starting stages of a new study on the CAP, and it suggests potential directions for future research
Submission history
Access paper:.
- HTML (experimental)
- Other Formats

References & Citations
- Google Scholar
- Semantic Scholar
BibTeX formatted citation

Bibliographic and Citation Tools
Code, data and media associated with this article, recommenders and search tools.
- Institution
arXivLabs: experimental projects with community collaborators
arXivLabs is a framework that allows collaborators to develop and share new arXiv features directly on our website.
Both individuals and organizations that work with arXivLabs have embraced and accepted our values of openness, community, excellence, and user data privacy. arXiv is committed to these values and only works with partners that adhere to them.
Have an idea for a project that will add value for arXiv's community? Learn more about arXivLabs .
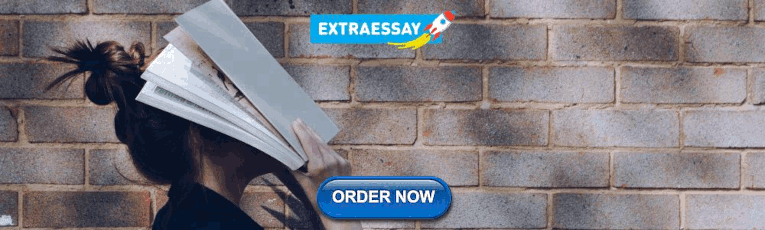
COMMENTS
The credit assignment problem (CAP) is a fundamental challenge in reinforcement learning. It arises when an agent receives a reward for a particular action, but the agent must determine which of its previous actions led to the reward. In reinforcement learning, an agent applies a set of actions in an environment to maximize the overall reward.
The (temporal) credit assignment problem (CAP) (discussed in Steps Toward Artificial Intelligence by Marvin Minsky in 1961) is the problem of determining the actions that lead to a certain outcome. For example, in football, at each second, each football player takes an action. In this context, an action can e.g. be "pass the ball", "dribbe ...
The concept of credit assignment refers to the problem of determining how much 'credit' or 'blame' a given neuron or synapse should get for a given outcome. More specifically, it is a way of determining how each parameter in the system (for example, each synaptic weight) should change to ensure that $\Delta F \ge 0$ .
Credit assignment determines the contribution of each internal decision to the final success or failure, and it has been shown to be effective in reducing the sample complexity of the training process. In this paper, we resort to a model-based reinforcement learning method to assign credits for model-free DRL methods. Since heuristic methods ...
Assigning credit or blame to those internal processes that lead to the choice of action is the structural credit assignment problem. In the case of pole balancing, the learning system will typically keep statistics such as how long, on average, the pole remained balanced after taking a particular action in a particular state, or after a failure ...
The credit assignment problem concerns determining how the success of a system's overall performance is due to the various contributions of the system's components (Minsky, 1963). "In playing a complex game such as chess or checkers, or in writing a computer program, one has a definite success criterion - the game is won or lost.
Figure 1.Example tasks highlighting the challenge of credit assignment and learning strategies enabling animals to solve this problem. (A) An example of a distal reward task that can be successfully learned with eligibility traces and TD rules, where intermediate choices can acquire motivational significance and subsequently reinforce preceding decisions (ex., Pasupathy and Miller, 2005 ...
Credit assignment is a fundamental problem in reinforcement learning, the problem of measuring an action's influence on future rewards. Explicit credit assignment methods have the potential to boost the performance of RL algorithms on many tasks, but thus far remain impractical for general use. Recently, a family of methods called Hindsight Credit Assignment (HCA) was proposed, which ...
Credit Assignment (HCA), an algorithm for credit assign-ment. HCA uses information about future events to compute updates for the policy in hindsight. HCA only modifies the probabilities of actions that affect the likelihood of reaching rewarding states, and does not update actions that have no
The credit assignment problem in reinforcement learning [Minsky, 1961, Sutton, 1985, 1988] is concerned with identifying the contribution of past actions on observed future outcomes. Of par-ticular interest to the reinforcement-learning (RL) problem [Sutton and Barto, 1998] are observed
Credit assignment is a fundamental problem in reinforcement learning, the problem of measuring an action's influence on future rewards. Improvements in credit assignment methods have the potential to boost the performance of RL algorithms on many tasks, but thus far have not seen widespread adoption. Recently, a family of methods called ...
important credit assignment challenges, through a set of illustrative tasks. 1 Introduction A reinforcement learning (RL) agent is tasked with two fundamental, interdependent problems: exploration (how to discover useful data), and credit assignment (how to incorporate it). In this work, we take a careful look at the problem of credit assignment.
Credit assignment poses a significant challenge in heterogeneous multi-agent reinforcement learning (MARL) when tackling fully cooperative tasks. Existing MARL methods assess the contribution of each agent through value decomposition or agent-wise critic networks. However, value decomposition techniques are not directly applicable to control problems with continuous action spaces. Additionally ...
this notion, which we then use to characterize when credit assignment is an ob-stacle to efficient learning. With this perspective, we outline several information-theoretic mechanisms for measuring credit under a fixed behavior policy, high-lighting the potential of information theory as a key tool towards provably-efficient credit assignment.
1000 Implementation: 1/3000 credit each. We see in this case the one person with the idea should receive the largest amount of credit. Similarly, if we weight the numbers differently, and if we assume contributions of individuals in groups are equal, then this credit assignment holds for all other combinations like (1000, 1, 1000), or (10000 ...
Credit is a contractual agreement in which a borrower receives something of value now and agrees to repay the lender at some date in the future, generally with interest. Credit also refers to an ...
What this handout is about. The first step in any successful college writing venture is reading the assignment. While this sounds like a simple task, it can be a tough one. This handout will help you unravel your assignment and begin to craft an effective response. Much of the following advice will involve translating typical assignment terms ...
Benefits. Reduce the economic losses and save money with the diminution of the balance sheet-profit. Gain legal advantages, in respect to the law related to credit assignment. Cut management costs on non-performing loans by employing the company internal recourses in a different way. Write a coherent balance sheet that represents the economic ...
A credit score is a numerical rating that shows how good one's credit is. It ranges from 300 to 850. Lenders will use his credit score to determine how likely it is that he will pay back the loan. With a score of 750, they will be confident that he will pay the money back. Greg used his credit card to buy exercise equipment.
AP Credit Policy Search Your AP scores could earn you college credit or advanced placement (meaning you could skip certain courses in college). Use this tool to find colleges that offer credit or placement for AP scores.
Biologically plausible solutions to credit assignment include those based on reinforcement learn-ing (RL) algorithms and reward-modulated STDP (Bouvier et al., 2016; Fiete et al., 2007; Fiete & Seung, 2006; Legenstein et al., 2010; Miconi, 2017). In these approaches a globally distributed reward signal provides feedback to all neurons in a network.
Google Classroom is a great app for assignments and the classroom in general, but there's some problems on the go for mobile users. I have a Google Pixel 8 (amazing phone), and when I'm on the go, no matter the network, albeit 5G, 5G UC (T-Mobile), or my Gigabit wifi at home, the app still opens really slowly and takes around 15-30 seconds to load a class page.
Credit card debt forgiveness can be easy to qualify for, assuming you meet some basic requirements. Getty Images If you have mounting credit card debt, you're not alone. The ...
A federal judge in Fort Worth, Texas, on Friday blocked a new Biden administration rule that would prohibit credit card companies from charging customers late fees higher than $8.
Filing for bankruptcy. The most straightforward way to have your credit card debt legally forgiven is to file for bankruptcy.When you file for Chapter 7 bankruptcy, commonly known as liquidation ...
Anyone who watched "The Big Bang Theory" with any regularity knew what was coming as its prequel "Young Sheldon" comes to a close, but the knock at the door that ended the most recent ...
A federal judge in Texas on Friday halted the Consumer Financial Protection Bureau's new rule capping credit card late fees at $8, a victory for business and banking groups challenging part of the ...
We propose to use information theory to define this notion, which we then use to characterize when credit assignment is an obstacle to efficient learning. With this perspective, we outline several information-theoretic mechanisms for measuring credit under a fixed behavior policy, highlighting the potential of information theory as a key tool ...
"For many families looking to finance a trip or a vacation, those [credit card] benefits are really valuable. … It's almost seen as savings - something in the bank that you will be able to ...
A Survey of Temporal Credit Assignment in Deep Reinforcement Learning. The Credit Assignment Problem (CAP) refers to the longstanding challenge of Reinforcement Learning (RL) agents to associate actions with their long-term consequences. Solving the CAP is a crucial step towards the successful deployment of RL in the real world since most ...