Click through the PLOS taxonomy to find articles in your field.
For more information about PLOS Subject Areas, click here .
Loading metrics
Open Access
Peer-reviewed
Research Article
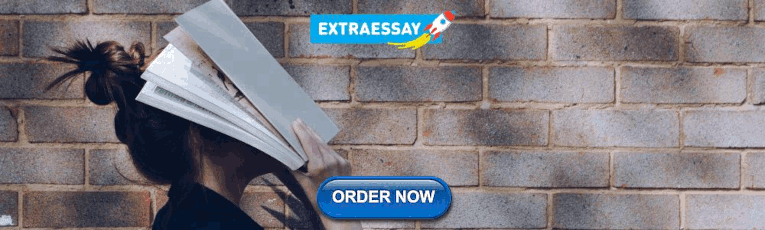
Pharmacological treatment of depression: A systematic review comparing clinical practice guideline recommendations
Roles Conceptualization, Data curation, Formal analysis, Funding acquisition, Investigation, Methodology, Project administration, Resources, Software, Supervision, Validation, Visualization, Writing – original draft, Writing – review & editing
* E-mail: [email protected]
Affiliation Departamento de Farmácia, Faculdade de Ciências Farmacêuticas, Universidade de São Paulo, São Paulo, São Paulo, Brasil

Roles Conceptualization, Data curation, Formal analysis, Investigation, Methodology, Project administration, Resources, Software, Supervision, Validation, Visualization, Writing – original draft, Writing – review & editing
Affiliation Departamento de Ciências Farmacêuticas, Instituto de Ciências Ambientais, Químicas e Farmacêuticas, Universidade Federal de São Paulo, Diadema, São Paulo, Brasil
Roles Conceptualization, Data curation, Formal analysis, Investigation, Methodology, Validation, Visualization, Writing – original draft, Writing – review & editing
Affiliation Departamento e Instituto de Psiquiatria, Hospital das Clínicas, Faculdade de Medicina da Universidade de São Paulo (IPq-HC-FM-USP); Divisão de Psiquiatria e Psicologia Hospital Universitário (HU) USP. Laboratório de Investigações Médicas – 21 (LIM 21) FM-USP, São Paulo, Brasil
Roles Conceptualization, Data curation, Methodology, Writing – review & editing
Roles Conceptualization, Data curation, Writing – review & editing
- Franciele Cordeiro Gabriel,
- Daniela Oliveira de Melo,
- Renério Fráguas,
- Nathália Celini Leite-Santos,
- Rafael Augusto Mantovani da Silva,
- Eliane Ribeiro
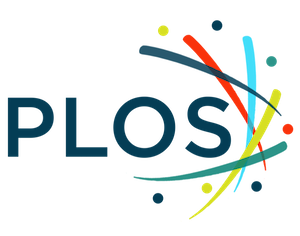
- Published: April 21, 2020
- https://doi.org/10.1371/journal.pone.0231700
- Peer Review
- Reader Comments
Depression affects over 300 million individuals worldwide and is responsible for most of the 800,000 annual suicides. Clinical practice guidelines (CPGs) for treatment of depression, founded on scientific evidence, are essential to improve patient care. However, economic and sociocultural factors may influence CPG elaboration, potentially leading to divergences in their recommendations. Consequently, we analyzed pharmacological recommendations for the treatment of depression from the most relevant CPGs. We included four CPGs with scores ≥ 80% for Domain 3 (rigor of development) on the Appraisal of Guidelines for Research and Evaluation and two other commonly used CPGs. The recommendations, their strengths, and the level of evidence were extracted from each CPG by two independent researchers and grouped as follows: (1) general recommendations for the pharmacological treatment for depression (suicide risk, acute treatment, continuation and maintenance phases, and treatment discontinuation); (2) treatment of non-responsive or partially responsive patients; and (3) treatment for subtypes of depression (chronic, psychotic, catatonic, melancholic, seasonal, somatic, mixed, and atypical). Only 50% of CPGs included recommendations for the risk of suicide associated with pharmacotherapy. All CPGs included serotonin selective reuptake inhibitors (SSRIs) as first-line treatment; however, one CPG also included agomelatine, milnacipran, and mianserin as first-line alternatives. Recommendations for depression subtypes (catatonic, atypical, melancholic) were included in three CPGs. The strength of recommendation and level of evidence clearly differed among CPGs, especially regarding treatment augmentation strategies. We conclude that, although CPGs converged in some recommendations (e.g., SSRIs as first-line treatment), they diverged in cardinal topics including the absence of recommendations regarding the risk of suicide associated with pharmacotherapy. Consequently, the recommendations listed in a specific CPG should be followed with caution.
Citation: Gabriel FC, de Melo DO, Fráguas R, Leite-Santos NC, Mantovani da Silva RA, Ribeiro E (2020) Pharmacological treatment of depression: A systematic review comparing clinical practice guideline recommendations. PLoS ONE 15(4): e0231700. https://doi.org/10.1371/journal.pone.0231700
Editor: Gabriele Fischer, Medizinische Universitat Wien, AUSTRIA
Received: June 8, 2019; Accepted: March 31, 2020; Published: April 21, 2020
Copyright: © 2020 Gabriel et al. This is an open access article distributed under the terms of the Creative Commons Attribution License , which permits unrestricted use, distribution, and reproduction in any medium, provided the original author and source are credited.
Data Availability: All relevant data are within the manuscript and its Supporting Information files.
Funding: This study was financed by the Coordenação de Aperfeiçoamento de Pessoal de Nível Superior - Brasil (CAPES) - Finance Code 001 to FCG and NCL. The funder had no role in study design, data collection and analysis, decision to publish, or preparation of the manuscript. No additional external funding was provided for this study.
Competing interests: The authors have declared that no competing interests exist.
Introduction
Globally, mental illness affects approximately 22% of the population [ 1 ]. Depression is the most prevalent psychiatric disorder, which affects more than 300 million individuals [ 2 ]. It is an incapacitating disorder, responsible for most of the 800,000 annual suicides [ 2 ]. Along with population growth and aging, the number of individuals with depression has also increased considerably and led to overloaded healthcare systems, thereby generating the need for resource optimization [ 1 , 3 ]. A primary challenge in the field of mental health is the development of health interventions based on scientific evidence to combat depression [ 4 ].
Carefully developed clinical practice guidelines (CPGs) can improve patient healthcare by outlining practices recommended based on scientific research [ 5 – 7 ].
CPGs should ensure that potential biases are properly approached during the development process and established recommendations have the viability to be implemented [ 8 ]. The development process of original high-quality CPGs demands time, resources, and an experienced team [ 9 ]. Scarcity of resources, particularly in developing countries, restricts the development of CPGs, potentially compromising their quality and validity [ 9 ]. Additionally, potential biases might result from cultural issues, even in developed countries. Recently, there has been an increase in the number of CPG publications, and problems concerning their quality have been highlighted in various studies [ 10 – 12 ].
The current study aimed to analyze the most relevant CPGs for the pharmacological treatment of depression and clarify a matrix of recommendations including agreements and potential disagreements among CPGs. Such a matrix can contribute to the development of a critical view of CPGs for practitioners and possibly help the development and adaptation of CPGs.
Materials and methods
Identification of clinical practice guidelines.
We recently reported CPGs for the pharmacological treatment of noncommunicable diseases that could be considered “high-quality” [ 12 ]. In that study, we conducted individual systematic reviews for each included disease; and using the second version of the Appraisal of Guidelines for Research and Evaluation (AGREE II) , evaluated 421 CPGs to establish the quality of their protocol registered on PROSPERO (CRD42016043364) [ 12 ]. In this study, we focus specifically on the part of that systematic review about the pharmacological treatment of depression [ 12 ].
We conducted a comprehensive search in MEDLINE, Embase, and the Cochrane Library, as well as in 12 specific websites for CPGs, because all such databases are well-recognized guideline repositories that have been cited frequently in previous studies of systematic reviews [ 12 , 13 ]. The CPG searched were published between 2011 and 2016 (details of search strategies are in S1 Appendix ). In April 2019, we searched the literature to update the included CPGs. Two independent reviewers screened the records regarding the eligibility criteria and conducted the data extraction. Discrepancies were solved by consensus.
To be included in this study, a CPG for the treatment of depression should have recommendations concerning the pharmacological treatment of depression in adults. CPGs published in English, Portuguese, or Spanish with free or restricted access to updated versions were eligible. To be included in the analysis of recommendations the CPG should have been considered of high quality (see next paragraph or had to be one of the most well-known and widely accepted CPGs [ 14 ]. All CPGs included in the analysis of the recommendations was evaluated to verify the presence of their updated version until April 2019. CPGs were excluded if they were specific for inpatients; specific for local use; focused on specific treatment approaches, such as psychotherapy or neuromodulation; or were for specific groups including pregnant women and children. CPGs addressing comorbidities in depression were also excluded.
CPG quality was judged by three independent appraisers on the basis of the six domains of the AGREE-II , as described previously [ 12 ]. Of these six domains, domain 3 (rigor of development) is considered the most relevant for the reliability of the recommendations[ 15 – 17 ]. The AGREE II does not suggest a cutoff value denoting acceptable or high quality; instead, cutoff values were determined by groups assessing CPG quality [ 17 ]. The domain 3 cut-off of ≥80% was adopted for this study to indicate high quality, as proposed in prior studies [ 18 – 20 ]. Details of quality appraisal are shown in S3 Appendix .
Extraction and analysis of recommendations
All recommendations regarding pharmacological treatment and the classification of the level of evidence from the included CPGs (when this information was available) were independently extracted by two researchers. Disagreements between the researchers (FCG and NCLS) were resolved by consensus; in the absence of a consensus, a senior investigator (ER) was included to solve the disagreement.
To perform the analyses, recommendations were classified based on their type and organized into tables by main topics. One of the authors (FCG) developed the first version of the classification, which was discussed with professors of pharmacy (ER) and psychiatry (RF).
The final version of the comparative tables of recommendations were achieved after three rounds of discussion. The recommendations were grouped by the main topics: 1) general recommendations for pharmacological treatment of depression (acute suicide risk and treatment, continuation phase treatment, maintenance phase treatment, and treatment discontinuation), 2)recommendations for treatment for those who did not respond or partially responded to therapy, and 3) recommendations for the treatment of depression subtypes (chronic depression or dysthymia, psychotic depression, catatonic depression, atypical conditions, melancholic depression, seasonal depression, somatic depression, and mixed depression).
In our initial search, we identified 947 citations and abstracts after removing duplicates. Thereafter, by reading the full text and applying the eligibility criteria, we selected 27 CPGs for this study ( Fig 1 ). ( S2 Appendix includes the reason for excluding 105 full records).
- PPT PowerPoint slide
- PNG larger image
- TIFF original image
https://doi.org/10.1371/journal.pone.0231700.g001
For the analysis of recommendations, 6 CPGs were included. Four CPGs presented a score ≥ 80% for Domain 3 and were considered high-quality [ 21 – 24 ]. In addition to these selected CPGs, two others were included based on their widespread acceptance [ 14 ]: the Canadian Network for Mood and Anxiety Treatments (CANMAT) and the American Psychiatric Association (APA) guidelines [ 25 , 26 ]. The six CPGs selected for analysis of their recommendation, based on their AGREE II Domain 3 score or on their acceptability, were as follows: Guía Clínica AUGE [ 21 ], score = 89%; Guía de Práctica Clínica [ 22 ], score = 86%; Depression in adults [ 23 ], score = 84%; Depression, adult in primary care [ 24 ], score = 81%; Practice guideline for the treatment of patients with major depressive disorder [ 26 ], score = 46%; and CANMAT [ 25 ], score = 54%. Table 1 briefly describes their characteristics.
https://doi.org/10.1371/journal.pone.0231700.t001
General recommendations according to the main topic for pharmacological treatment of depression
Only three CPGs provided information regarding the evaluation and management of the risk of suicide with pharmacological treatment, and all recommendations about this subject were considered strong [ 22 , 23 , 26 ]. Four CPGs recommended non-pharmacological treatment as first-line treatment for mild depression [ 21 – 23 , 25 ]. From the CPGs that mentioned recommendations about the indication of pharmacological treatment for patients with moderate or severe depression and a combination of pharmacological and psychotherapy treatments [ 21 – 23 , 26 ], such recommendations were considered strong ( Table 2 ).
https://doi.org/10.1371/journal.pone.0231700.t002
All CPGs indicated serotonin selective reuptake inhibitors (SSRIs) as an option for first-line treatment for depression, and the recommendations were based on high-quality studies; however, most CPGs did not cite specific drugs. Mirtazapine was considered as first-line treatment by four CPGs [ 22 , 24 – 26 ]. The tricyclic amitriptyline, fluoxetine, sertraline, and mirtazapine were considered first-line drugs by the Colombian CPG—amitriptyline for patients without contraindications and the others for patients with contraindications to tricyclics. The recommendations were based on pharmacoeconomic studies. Sertraline and mirtazapine were considered owing to cost per quality-adjusted life years [ 22 ]. The CANMAT CPG recommended agomelatine, mianserin, and milnacipran as first-line treatment [ 25 ]. More details are available in S1 Table .
Recommendations for treatment of depression in those who did not respond or partially responded
All CPGs included recommendations for the treatment of those who did not respond or partially responded to first-line therapy. Such recommendations are synthesized in Table 3 and details are presented in S2 Table . Almost all recommendations were considered strong regarding the adjustment of drug dosages when there was a lack of response to the initial pharmacological treatment. Moreover, antipsychotic agents were recommended as an augmentation strategy by five CPGs.
https://doi.org/10.1371/journal.pone.0231700.t003
However, considering the differences found among the CPGs, the CANMAT was the only one that grouped the recommendations for adjuvant treatment (i.e., combination and augmentation strategies), in first-, second-, and third-line strategy. Moreover, the level of evidence and strength of recommendations for the combination and augmentation strategies varied among the CPGs. Although the same evidence scale was not precisely used to classify among the five CPGs, the evidence for recommendation of antipsychotic agents for augmentation was considered with substantial clinical safety by the APA CPG, weakly by the Colombian CPG. The remaining CPGs did not specify the level of the evidence or the strength of the recommendations.
Among the five CPGs that included lithium as an augmentation strategy, only three clearly indicated the strength of recommendation; however, discrepancies existed among them. While the Chilean CPG strongly recommended it, the Colombian CPG recommendation was weak, and the APA CPG recommended it, based on moderate clinical safety. Considering thyroid hormones as an augmentation strategy, two CPGs included it; however, they disagreed on the strength of the recommendation [ 21 , 26 ]. The Chilean CPG strongly recommended thyroid hormones, while the APA CPG indicated that there was moderate evidence of clinical safety.
Recommendations for treatment of depression considering its subtypes
Except for the Chilean CPG, all included recommendations per depression subtypes ( Table 4 ). Moreover, most CPGs [ 22 , 23 , 25 , 26 ] provided recommendations for treating psychotic depression, except the ICSI and the Chilean CPG. The recommendations for treating depression with psychotic features was the use of antipsychotic and antidepressants agents. Regardless, the classification method applied to the significance of the recommendation or the quality of the evidence, using these drugs was considered a strong recommendation or was based on high-quality evidence.
https://doi.org/10.1371/journal.pone.0231700.t004
No high-quality CPG mentioned the treatment of catatonic depression. On the other hand, the most employed guidelines in clinical practice—CANMAT and APA CPG—recommended using benzodiazepines for the treatment of catatonic depression; however, they disagreed on the classification of the quality of the evidence [ 25 , 26 ]. Moreover, depression with atypical characteristics was considered in the CPGs most used in clinical practice and in the ICSI CPG [ 24 – 26 ]. Depression with melancholic characteristics was contemplated only in the CPGs most used in clinical practice [ 25 , 26 ]. Seasonal, with somatic symptoms, and mixed depression disorders were only addressed in the CANMAT CPG [ 25 ]. More details are shown in S3 Table .
First, it should be noted that pharmacological treatment of depression is one of the strategies that should be considered to ensure adequate patient care. Pharmacotherapy should be prescribed only after a careful evaluation of the patient, including risk of suicide, requirement of hospitalization, indication of psychotherapy, and existence of comorbidities among other clinical and psychosocial aspects.
Relevant findings of this study include the absence of specific recommendations regarding suicide risk associated with pharmacotherapy in some CPGs [ 21 , 24 , 25 ]; convergence on SSRIs as first-line antidepressants by all CPGs, aside with indication of some peculiar antidepressants as first-line by two CPGs [ 22 , 25 ]; specific strategies for treatment of a partial response are missing in various CPGs; and treatment specificities for depression subtypes being covered by all CPGs except one [ 21 ].
Recommendations for pharmacological treatment of depression.
Depression is considered the principal risk factor for suicide, and suicide risk peaks in the first weeks of antidepressant treatment [ 27 ]. However, only three of the 6 CPGs included a topic concerning the risk of suicide associated with pharmacotherapy [ 22 , 23 , 26 ]. Thus, our findings highlight that future CPGs should consider the risk of suicide associated with pharmacological treatment.
First-line treatment
All CPGs considered SSRIs as a first-line antidepressant treatment. However, we identified two important discrepancies. Besides SSRIS, as options, the CANMAT CPG recommended the use of agomelatine, milnacipran, and mianserin [ 25 ]; and the Colombian CPG recommended the use of amitriptyline [ 22 ] as first-line treatment based on pharmacoeconomic studies.
Discrepancies among recommendations can negatively affect healthcare professionals’ confidence in CPGs [ 28 ]. We found such differences even among high-quality CPGs. CPGs may provide different recommendations according to cultural differences or based on the availability and infrastructure of a healthcare system [ 29 ]. Consequently, values shared by developers and patients, aside from cost issues, may influence the choice of a recommended treatment and reduce the reliability of the recommendation, particularly when scientific evidence is weak [ 30 – 32 ].
According to the CANMAT CPG [ 25 ], agomelatine demonstrated favorable efficacy and tolerability in a network meta-analysis of new-generation antidepressants conducted by Khoo et al (2015) [ 33 ]. The advantage of network meta-analysis is that multiple treatments can be subjected to both direct (i.e., among randomized controlled trials) and indirect (i.e., across trials based on a common variable) comparisons of interventions; the effects of different interventions that have not been investigated in trials can be compared, and the analysis of all interventions enables ranking of therapeutic alternatives with regard to a given outcome. However, other aspects must be taken into account: To assess the level of evidence or strength of the recommendation, it is important to consider not only the type of study but also its quality or risk of bias, or both, as recommended in the GRADE method, for example [ 29 , 34 ]. Network meta-analysis should be conducted under strict and specific methodology conditions (transitivity and consistency criteria met); the inclusion of studies at risk of bias may negatively compromise the validity of the findings [ 35 ]. In their network meta-analysis, Khoo et al did not describe the result of the risk of bias assessment for any of the eight clinical trials included in the meta-analysis, but in the general assessment, most of the primary studies included were judged as having high or unclear risk of bias in at least one domain of the Cochrane Collaboration risk of bias tool [ 33 ]. In a 2013 Cochrane review of 13 trials in a pairwise meta-analysis [ 36 ] and in a 2018 network meta-analysis conducted by Cipriani et al [ 3 ], agomelatine did not show robust advantages over the other antidepressants for effectiveness, but it appeared to be better tolerated. In both studies, the limitation was that the primary studies had biases ([ 3 ]). In addition, agomelatine was not approved by the U.S. Food and Drug Administration to treat depression, and its safety and efficacy have been questioned [ 36 ]. The Colombian CPG explicitly recommends not using agomelatine because of insufficient evidence on its effectiveness [ 22 ].
The CANMAT CPG recommendation of milnacipran as a first-line treatment is based more on its tolerability than on its efficacy [ 37 ], which does not follow the recommended sequence for the rational use of medicines: efficacy, availability, and safety [ 38 ]. Moreover, in Cipriani et al’s study [ 3 ], milnacipran did not stand out in terms of either effectiveness or safety [ 3 ]. Milnacipran is also not approved by the U.S. Food and Drug Administration to treat depression [ 39 ].
The recommendation of mianserin as a first-line option is controversial because the CANMAT CPG itself cites the results of a network meta-analysis that demonstrated few differences in response, although SSRIs and TCAs were superior to mianserin/mirtazapine and moclobemide [ 25 , 40 ]. Indeed, Linde et al [ 40 ] affirmed that physicians should be aware that SSRIs and TCAs have a somewhat more solid evidence base than do other pharmacological classes. Cipriani et al [ 3 ] did not include mianserin in network meta-analysis [ 3 ]. Arroll et al [ 41 ] conducted a meta-analysis of two trials in which mianserin was compared with placebo and concluded that mianserin was effective for continuous outcomes but did not affect rates of remission and response [ 41 ].
The Colombian CPG inclusion of amitriptyline (for patients without a contraindication to CTAs) as a first-line option was based on its good cost-effectiveness profile. In the pharmacoeconomic study conducted by the Colombian CPG, sertraline was considered more cost-effective than amitriptyline regarding outcome quality–adjusted life years. With regard to its efficacy, a meta-analysis supported the superiority of amitriptyline over other tricyclic/heterocyclic antidepressants and over SSRIs; however, the effect size was clinically not relevant [ 42 ]. Cipriani et al [ 3 ] confirmed the effectiveness of amitriptyline but revealed that it had one of the highest rates of treatment abandonment owing to adverse events [ 3 ]. In addition, evidence regarding the tolerability and risk of CTAs is plentiful and includes a narrow safety margin and considerable risk of death in cases of overdose [ 43 ]. With regard to individuals at risk for adverse events, such as older adults (i.e., the Beers criteria), the ICSI CPG stresses that tricyclics must not be used by older adults because of their anticholinergic effects and their capacity to induce orthostatic hypotension and stimulation of the central nervous system [ 44 ].
Despite recommending SSRIs as first-line treatments, most CPGs did not cite specific SSRIs. Only three CPGs specifically indicated the SSRIs used as the first-line treatment [ 22 , 24 , 25 ]. Although only a few controlled randomized trials have compared SSRIs head-to-head [ 3 ], it is difficult to explain why some CPGs recommend specific SSRIs while others recommend a group of SSRIs.
Partial and non-responders
The initial pharmacological treatment for depression had a response rate ranging from 40–60% [ 45 ], and only around 30% achieved remission [ 45 , 46 ]. Consequently, recommendations to non-responders should be an essential part of any CPG. In fact, all the present CPG recommendations addressed first-line therapy and non-responders. Adjustment of the dosage with strong evidence was a consensus among the CPGs.
It is worth noting that many CPGs describe the alternatives of replacing the antidepressant for those not responding to first line treatment without specifying which should be considered the second, the third or the fourth-line therapy. Additionally, among those specifying a sequence of strategies, the concept and support to define each line of therapy varies across the CPGs, leading to considerable discrepancies. For example, the Colombian CPG, instead of including other antidepressants, recommends using as second-line treatment, first-line alternatives such as fluoxetine, sertraline, amitriptyline or mirtazapine, that had not been prescribed. Regarding third-line treatment, the Colombian CPG recommends imipramine, clomipramine, paroxetine, escitalopram, citalopram, fluvoxamine, venlafaxine, duloxetine, desvenlafaxine, trazodone, and bupropion [ 22 ], while third line alternatives for the Canadian CPG (CANMAT) are Monoamine oxidase inhibitors (i.e. Phenelzine and tranylcypromine) and reboxetine [ 25 ]. On the other hand, for the APA CPG, MAOIs are considered fourth line therapy [ 26 ].
Also recommended by most CPGs was the augmentation with antipsychotics for partial responders. However, some CPGs did not report, while others disagreed, about the strength of recommendation or level of evidence for augmentation strategies. Furthermore, the CANMAT CPG was the only one recommending an order for the augmentation strategies.
Subtypes of depression
Depression with psychotic features was the subtype receiving most consistent recommendations across the CPGs. Four CPGs recommended antipsychotic agents in combination with antidepressants for the treatment of depression with psychotic features [ 22 , 23 , 25 , 26 ]. Various studies, including meta-analyses, have supported the advantage of associating antidepressants with antipsychotics for the treatment of depression with psychotic features [ 47 ]. The goal of the ICSI CPG was primary-care attention, which explains its absence of recommendations for depression with psychotic features. The Chilean CPG included only comments about the effectiveness of antidepressants and antipsychotics for psychotic depression; however, it is outside the topic of recommendations [ 21 ].
Data supporting distinct antidepressant response of atypical depression to monoamine oxidase inhibitors (MAOIs; i.e., tranylcypromine) have been reported since the 1960s [ 48 ]. The presence of melancholic or atypical features was considered respectively by two and three CPGs. The APA CPG was the only CPG that addressed specific treatment for melancholic depression—serotonin and noradrenaline reuptake inhibitors (SSRIs) and tricyclic antidepressants; the CANMAT CPG stated that no antidepressant has been proven to be superior for melancholic depression. For depression with atypical features, the APA CPG and the ICSI CPG recommended MAOIs, while the CANMAT CPG stated that no antidepressant has been proven to be superior. Such discrepancies are in line with controversies in the literature. Notwithstanding the traditional view of superiority of MAOIs for depression with atypical features, the iSPOT-D Trial compared the efficacy of venlafaxine, escitalopram, and sertraline and found similar symptom reduction trajectories among depression subtypes including melancholic, atypical, anxious depression, and subtype combinations [ 49 ].
Considering catatonic depression, benzodiazepines may lead to a rapid relive for some patients [ 50 ]; however, only the APA and the CANMAT CPGs included such recommendation. Although, it can be argued that catatonic depression is not frequent, it has a high morbidity and our view is that consideration to specificities of its treatment should be included in CPGs.
Strengths and limitations
We included CPGs published in English, Portuguese, and Spanish; thus, the results might not reflect relevant CPGs in other languages. The intrinsic subjectivity limitation of the AGREE II should also be considered; to minimize this, we included three evaluators and disagreements among them were solved by discussion until consensus was reached. We also included two additional CPGs based on their wild acceptability (CANMAT and APA CPG) [ 25 , 26 ] instead of basing the study only on the AGREE II evaluation. Another point to consider is that some recommendations might have not been considered because they were placed outside the topic of pharmacological treatment or it was not clear if they were recommendations or mere dissecting of evidence.
Among the strengths of this study, we cite the comprehensive search and the careful training of appraisers. In describing the recommendations, we offer a comparative view of distinct CPGs that provide physicians and patients a more comprehensive understanding of pharmacological approaches to the treatment of depression. Our findings could help in the elaboration/adaptation of a CPG because we identified important divergences among existing CPGs to which stakeholders (patients and professionals) should give special attention. Moreover, the identification of the points at which CPGs converge fully and that have been well addressed in certain CPGs may also be helpful for the elaboration/adaptation of a CPG for local contexts, as well as contribute to clinical decisions about treatment for this severe mental health problem.
We found that the various CPGs were typically consistent with each other; however, they presented some vital differences. The use of SSRIs as first-line pharmacological treatment and its dose adjustment for non-responsive patients were consistent among all the included CPGs. Largely consistent was the use of antipsychotics as augmentation (except for the ICSI CPG, which addressed primary care) for non-responders. Importantly, only 50% of the CPGs addressed the risk of suicide associated with pharmacotherapy. Considering the increased risk of suicide associated with the first few weeks of antidepressant treatment, recommendations regarding this topic should be mandatory in all CPGs. Moreover, specificities for some subtypes of depression (e.g., catatonic and atypical) were addressed by some but not all CPGs. Differences in the level of evidence or strength of recommendation were very frequent among the CPGs, and some of them presented unique recommendations. These findings support that, when using a specific guideline for the treatment of depression, caution is needed to provide the most appropriate treatment to each patient.
Supporting information
S1 appendix. systematic review strategy: research terms used to identify clinical practice guidelines in the medline, embase, and the cochrane library databases..
https://doi.org/10.1371/journal.pone.0231700.s001
S2 Appendix. Reasons for the inclusion and exclusion of clinical practice guidelines.
https://doi.org/10.1371/journal.pone.0231700.s002
S3 Appendix. Quality assessment of the included clinical practice guidelines.
https://doi.org/10.1371/journal.pone.0231700.s003
S1 Table. Recommendations for the treatment of depression, extracted from clinical practice guidelines, listed for elaborating the synthesis published between January 2011 and April 2019: Indications and strategies of pharmacological treatment.
https://doi.org/10.1371/journal.pone.0231700.s004
S2 Table. Recommendations for the treatment of depression, extracted from clinical practice guidelines, listed for elaborating the synthesis published between January 2011 and April 2019: Patients who did not respond or partially responded.
https://doi.org/10.1371/journal.pone.0231700.s005
S3 Table. Recommendations for the treatment of depression, extracted from clinical practice guidelines, listed for the elaboration of the synthesis published between January 2011 and April 2019: Treatment for subtypes.
https://doi.org/10.1371/journal.pone.0231700.s006
S1 Checklist. PRISMA 2009 checklist.
https://doi.org/10.1371/journal.pone.0231700.s007
Acknowledgments
We would like to acknowledge everyone who contributed their time and knowledge to this study without any financial support. We would like to thank CHRONIDE for their support in the appraisal of CPGs. We also appreciate the assistance of Professor Carlota de Oliveira Rangel Yagui in manuscript preparation. We are indebted to Caroline Molino, Luciana Vasconcelos, and Sheila Kalb Wainberg for their invaluable assistance in helping us understand the method of appraisal of CPGs. We also recognize Andrea Fernandes Larruscain and Aliandra Fantinell de Oliveira for their help with manuscript formatting. Finally, we are grateful to Alfredo Jose Neto for his assistance in discussion of data synthesis and Carlos Eduardo Moscato Fuzaro for his support in data.
- View Article
- PubMed/NCBI
- Google Scholar
- 2. World Health Organization. Depression and other common mental disorders: global health estimates. [cited 2017 December 8; Internet]. Geneva: World Health Organization 2017 [21 p.] http://apps.who.int/iris/bitstream/10665/254610/1/WHO-MSD-MER-2017.2-eng.pdf .
- 5. Institute of Medicine (US). Clinical practice guidelines we can trust. Washington (DC): National Academies Press; 2011. https://doi.org/10.17226/13058 pmid:24983061
- 8. AGREE Collaboration. Appraisal of guidelines for research and evaluation (AGREE) instrument [cited 2017 December 8; Internet]. London: St. Georges Hospital Medical School; 2001. https://www.paho.org/hq/dmdocuments/2012/AGREEworksheet-guideline-appraisal-sheet.pdf .
- 21. Chile, Ministerio de Salud (MINSAL). Guía clínica AUGE depresion en personas de 15 años y mas [Internet]. [Santiago]: MINSAL; 2013 [cited 2017 June 30]. [Serie Guías Clínicas]. http://supersalud.gob.cl/difusion/572/articles-652_recurso_1.pdf .
- 22. Colombia, Ministerio de Salud. Guía de Práctica clínica: detección temprana y diagnóstico del episodio depresivo y trastorno depresivo recurrente en adultos: atención integral de los adultos con diagnóstico de episodio depresivo o trastorno depresivo recurrente. c2013 [cited 2017 June 30; Internet]. http://gpc.minsalud.gov.co/gpc_sites/Repositorio/Conv_500/GPC_td/gpc_td.aspx . Spanish.
- 23. National Institute for Health and Care Excellence. Depression in adults: recognition and management. 2009 October [last updated 2018 April; cited 2017 June 30; Internet]. https://www.nice.org.uk/guidance/cg90/evidence .
- 24. Trangle M, Gursky J, Haight R, Hardwig J, Hinnenkamp T, Kessler D, et al. Adult depression in primary care. [Bloomington]: Institute for Clinical Systems Improvement; Updated March 2016.
- 26. Gelenberg AJ, Freeman MP, Markowitz JC, Rosenbaum JF, Thase ME, Trivedi MH, et al. Practice guideline for the treatment of patients with major depressive disorder, third edition. [Washington, D.C.]: American Psychiatry Association; 2010.
- 27. World Health Organization. Suicide prevention: a resource for counsellors. Geneva: World Health Organization; 2006.
- 34. GRADE Work Group: Grading of Recommendations Assessment, Development, and Evaluation (GRADE) [Internet]. [n.p.]: GRADE Work Group; [cited 2007 Feb 21]. www.gradeworkinggroup.org .
- 35. Higgins JPT, Altman DG. Assessing risk of bias in included studies. In: Higgins JPT, Green S, editors. Cochrane handbook for systematic reviews of interventions Version 5.1.0 [Internet]. [Chichester (UK)]: Cochrane; 2011 [update 2011 Mar; cited 2012 Oct 3]. www.training.cochrane.org/handbook .
- 38. Brasil, Ministério da Saúde, Secretaria de Ciência, Tecnologia e Insumos Estratégicos. [Rational use of medicines: selected subject]. Brasília: MS, 2012. [Internet] http://bvsms.saude.gov.br/bvs/publicacoes/uso_racional_medicamentos_temas_selecionados.pdf .
Thank you for visiting nature.com. You are using a browser version with limited support for CSS. To obtain the best experience, we recommend you use a more up to date browser (or turn off compatibility mode in Internet Explorer). In the meantime, to ensure continued support, we are displaying the site without styles and JavaScript.
- View all journals
- Explore content
- About the journal
- Publish with us
- Sign up for alerts
- Published: 18 June 2020
Advances in depression research: second special issue, 2020, with highlights on biological mechanisms, clinical features, co-morbidity, genetics, imaging, and treatment
- Julio Licinio 1 &
- Ma-Li Wong 1
Molecular Psychiatry volume 25 , pages 1356–1360 ( 2020 ) Cite this article
10k Accesses
11 Citations
4 Altmetric
Metrics details
The current speed of progress in depression research is simply remarkable. We have therefore been able to create a second special issue of Molecular Psychiatry , 2020, focused on depression, with highlights on mechanisms, genetics, clinical features, co-morbidity, imaging, and treatment. We are also very proud to present in this issue a seminal paper by Chottekalapanda et al., which represents some of the last work conducted by the late Nobel Laureate Paul Greengard [ 1 ]. This brings to four the number of papers co-authored by Paul Greengard and published in our two 2020 depression special issues [ 1 , 2 , 3 , 4 ].
The research content of this special depression issue starts with Chottekalapanda et al.’s outstanding contribution aimed at determining whether neuroadaptive processes induced by antidepressants are modulated by the regulation of specific gene expression programs [ 1 ]. That team identified a transcriptional program regulated by activator protein-1 (AP-1) complex, formed by c-Fos and c-Jun that is selectively activated prior to the onset of the chronic SSRI response. The AP-1 transcriptional program modulated the expression of key neuronal remodeling genes, including S100a10 (p11), linking neuronal plasticity to the antidepressant response. Moreover, they found that AP-1 function is required for the antidepressant effect in vivo. Furthermore, they demonstrated how neurochemical pathways of BDNF and FGF2, through the MAPK, PI3K, and JNK cascades, regulate AP-1 function to mediate the beneficial effects of the antidepressant response. This newly identified molecular network provides “a new avenue that could be used to accelerate or potentiate antidepressant responses by triggering neuroplasticity.”
A superb paper by Schouten et al. showed that oscillations of glucocorticoid hormones (GC) preserve a population of adult hippocampal neural stem cells in the aging brain [ 5 ]. Moreover, major depressive disorder (MDD) is characterized by alterations in GC-related rhythms [ 6 , 7 ]. GC regulate neural stem/precursor cells (NSPC) proliferation [ 8 , 9 ]. The adrenals secrete GC in ultradian pulses that result in a circadian rhythm. GC oscillations control cell cycle progression and induce specific genome-wide DNA methylation profiles. Schouten et al. studied primary hippocampal NSPC cultures and showed that GC oscillations induced lasting changes in the methylation state of a group of gene promoters associated with cell cycle regulation and the canonical Wnt signaling pathway. Furthermore, in a mouse model of accelerated aging, they showed that disruption of GC oscillations induced lasting changes in dendritic complexity, spine numbers and morphology of newborn granule neurons. Their results indicate that GC oscillations preserve a population of GR-expressing NSPC during aging, preventing their activation possibly by epigenetic programming through methylation of specific gene promoters. These important observations suggest a novel mechanism mediated by GC that controls NSPC proliferation and preserves a dormant NSPC pool, possibly contributing to neuroplasticity reserve in the aging brain.
MDD has a critical interface with addiction and suicide, which is of immense clinical and research importance [ 10 ]. Peciña et al. have reviewed a growing body of research indicating that the endogenous opioid system is directly involved in the regulation of mood and is dysregulated in MDD [ 11 ]. Halikere et al. provide evidence that addiction associated N40D mu-opioid receptor variant modulates synaptic function in human neurons [ 12 ].
Two papers by Amare et al. and Coleman et al. examine different genetic substrates for MDD, identifying novel depression-related loci as well as studying the interface with trauma [ 13 , 14 ].
The dissection of MDD clinical phenotypes, including their interface with other illnesses is a topic of several articles in this special issue. Belvederi Murri et al. examined the symptom network structure of depressive symptoms in late-life in a large European population in the 19 country Survey of Health, Ageing, and Retirement in Europe (SHARE) (mean age 74 years, 59% females, n = 8557) [ 15 ]. They showed that the highest values of centrality were in the symptoms of death wishes, depressed mood, loss of interest, and pessimism. Another article focused on a specific feature of MDD, namely changes in appetite. Simmons et al. aimed at explaining why some individuals lose their appetite when they become depressed, while others eat more, and brought together data on neuroimaging, salivary cortisol, and blood markers of inflammation and metabolism [ 16 ]. Depressed participants experiencing decreased appetite had higher cortisol levels than other subjects, and their cortisol values correlated inversely with the ventral striatal response to food cues. In contrast, depressed participants experiencing increased appetite exhibited marked immunometabolic dysregulation, with higher insulin, insulin resistance, leptin, c-reactive protein (CRP), interleukin 1 receptor antagonist (IL-1RA), and IL-6, and lower ghrelin than subjects in other groups, and the magnitude of their insulin resistance correlated positively with the insula response to food cues. Their findings support the existence of pathophysiologically distinct depression subtypes for which the direction of appetite change may be an easily measured behavioral marker.
Mulugeta et al. studied the association between major depressive disorder and multiple disease outcomes in the UK Biobank ( n = 337,536) [ 17 ]. They performed hypothesis-free phenome-wide association analyses between MDD genetic risk score (GRS) and 925 disease outcomes. MDD was associated with several inflammatory and hemorrhagic gastrointestinal diseases, and intestinal E. coli infections. MDD was also associated with disorders of lipid metabolism and ischemic heart disease. Their results indicated a causal link between MDD and a broad range of diseases, suggesting a notable burden of co-morbidity. The authors concluded that “early detection and management of MDD is important, and treatment strategies should be selected to also minimize the risk of related co-morbidities.” Further information on the shared mechanisms between coronary heart disease and depression in the UK Biobank ( n = 367,703) was explored by Khandaker et al. [ 18 ]. They showed that family history of heart disease was associated with a 20% increase in depression risk; however, a genetic risk score that is strongly associated with CHD risk was not associated with depression. Their data indicate that comorbidity between depression and CHD arises largely from shared environmental factors.
In a systematic review and meta-analysis of cohort studies, Wang et al. examined the interface of depression and anxiety in relation to cancer incidence and mortality [ 19 ]. Their analyses suggest that depression and anxiety may have an etiologic role and prognostic impact on cancer, although there is potential reverse causality.
Several papers in this issue examine imaging in MDD, either to unravel the underlying disease processes or to identify imaging biomarkers of treatment response. Let us first look at the studies focused on elucidating brain circuitry alterations in MDD. Arterial spin labeling (ASL) was used by Cooper et al. to measure cerebral blood flow (CBF; perfusion) in order to discover and replicate alterations in CBF in MDD [ 20 ]. Their analyses revealed reduced relative CBF (rCBF) in the right parahippocampus, thalamus, fusiform, and middle temporal gyri, as well as the left and right insula, for those with MDD. They also revealed increased rCBF in MDD in both the left and the right inferior parietal lobule, including the supramarginal and angular gyri. According to the authors, “these results (1) provide reliable evidence for ASL in detecting differences in perfusion for multiple brain regions thought to be important in MDD, and (2) highlight the potential role of using perfusion as a biosignature of MDD.” Further data on imaging in MDD was provided by a coordinated analysis across 20 international cohorts in the ENIGMA MDD working group. In that paper, van Velzen et al. showed that in a coordinated and harmonized multisite diffusion tensor imaging study there were subtle, but widespread differences in white matter microstructure in adult MDD, which may suggest structural disconnectivity [ 21 ].
Four articles in this special issue examine imaging biomarkers of treatment response. Greenberg et al. studied reward-related ventral striatal activity and differential response to sertraline versus placebo in depressed using functional magnetic resonance imaging while performing a reward task [ 22 ]. They found that ventral striatum (VS) dynamic response to reward expectancy (expected outcome value) and prediction error (difference between expected and actual outcome), likely reflecting serotonergic and dopaminergic deficits, was associated with better response to sertraline than placebo. Their conclusion was that treatment measures of reward-related VS activity may serve as objective neural markers to advance efforts to personalize interventions by guiding individual-level choice of antidepressant treatment. Utilizing whole-brain functional connectivity analysis to identify neural signatures of remission following antidepressant treatment, and to identify connectomic predictors of treatment response, Korgaonkar et al. showed that intrinsic connectomes are a predictive biomarker of remission in major depressive disorder [ 23 ]. Based on their results that team proposed that increased functional connectivity within and between large-scale intrinsic brain networks may characterize acute recovery with antidepressants in depression. Repple et al. created connectome matrices via a combination of T1-weighted magnetic resonance imaging (MRI) and tractography methods based on diffusion-weighted imaging severity of current depression and remission status in 464 MDD patients and 432 healthy controls [ 24 ]. Reduced global fractional anisotropy (FA) was observed specifically in acute depressed patients compared to fully remitted patients and healthy controls. Within the MDD patients, FA in a subnetwork including frontal, temporal, insular, and parietal nodes was negatively associated with symptom intensity, an effect remaining when correcting for lifetime disease severity. Their findings provide new evidence of MDD to be associated with structural, yet dynamic, state-dependent connectome alterations, which covary with current disease severity and remission status after a depressive episode. The effects of electroconvulsive therapy (ECT), the most effective treatment for depression, on the dentate gyrus (DG) were studied by Nuninga et al. through an optimized MRI scan at 7-tesla field strength, allowing sensitive investigation of hippocampal subfields [ 25 , 26 ]. They documented a large and significant increase in DG volume after ECT, while other hippocampal subfields were unaffected. Furthermore, an increase in DG volume was related to a decrease in depression scores, and baseline DG volume predicted clinical response. These findings suggest that the volume change of the DG is related to the antidepressant properties of ECT, possibly reflecting neurogenesis.
Three articles report new directions for antidepressant therapeutics. Papakostas et al. presented the results of a promising phase 2, double-blind, placebo-controlled study of NSI-189 phosphate, a novel neurogenic compound, in MDD patients [ 27 ]. As the endogenous opioid system is thought to play an important role in the regulation of mood, Fava et al. studied the buprenorphine/samidorphan combination as an investigational opioid system modulator for adjunctive treatment of MDD in two phase 3, randomized, double-blind, placebo-controlled studies that utilized the same sequential parallel-comparison design [ 28 ]. One of the studies achieved the primary endpoint, namely change from baseline in Montgomery–Åsberg Depression Rating Scale (MADRS)-10 at week 5 versus placebo) and the other study did not achieve the primary endpoint. However, the pooled analysis of the two studies demonstrated consistently greater reduction in the MADRS-10 scores from baseline versus placebo at multiple timepoints, including end of treatment. These data provide cautious optimism and support further controlled trials for this potential new treatment option for patients with MDD who have an inadequate response to currently available antidepressants. Fava et al. also report the results of a double-blind, placebo-controlled, dose-ranging trial of intravenous (IV) ketamine as adjunctive therapy in treatment-resistant depression, using four doses of ketamine and a control [ 29 , 30 ]. They show that there was evidence for the efficacy of the two higher doses of IV ketamine and no clear or consistent evidence for clinically meaningful efficacy of the two lower doses studied.
Overall, in this issue, immense progress in depression research is provided by outstanding studies that highlight advances in our understanding of MDD biology, clinical features, co-morbidity, genetics, brain imaging (including imaging biomarkers), and treatment. Building on the groundbreaking articles from our previous 2020 special issues on stress and behavior [ 31 , 32 , 33 , 34 , 35 , 36 , 37 , 38 , 39 , 40 , 41 , 42 , 43 , 44 , 45 , 46 , 47 , 48 , 49 ] and on depression [ 2 , 3 , 4 , 50 , 51 , 52 , 53 , 54 , 55 , 56 , 57 , 58 , 59 , 60 , 61 , 62 ], we are proud that the stunning progress presented here found its home in our pages. From inception in 1996, we have aimed at making Molecular Psychiatry promote the integration of molecular medicine and clinical psychiatry [ 63 ]. It is particularly rewarding to see that goal achieved so spectacularly in this second 2020 special issue on MDD, a disorder of gene-environment interactions that represents a pressing public health challenge, with an ever increasing impact on society [ 64 , 65 , 66 ]. We are privileged to have in these two 2020 depression special issues four remarkable papers from Paul Greengard’s teams that provide substantial new data on the mechanisms of antidepressant action [ 1 , 2 , 3 , 4 ]. Such profound advances in basic science are needed to facilitate and guide future translational efforts needed to advance therapeutics [ 67 , 68 ].
Chottekalapanda R, et al. AP-1 controls the p11-dependent antidepressant response. Mol Psychiatry. 2020. https://doi.org/10.1038/s41380-020-0767-8 .
Sagi Y, et al. Emergence of 5-HT5A signaling in parvalbumin neurons mediates delayed antidepressant action. Mol Psychiatry. 2019. https://doi.org/10.1038/s41380-019-0379-3 .
Oh SJ, et al. Hippocampal mossy cell involvement in behavioral and neurogenic responses to chronic antidepressant treatment. Mol Psychiatry. 2019. https://doi.org/10.1038/s41380-019-0384-6 .
Shuto T, et al. Obligatory roles of dopamine D1 receptors in the dentate gyrus in antidepressant actions of a selective serotonin reuptake inhibitor, fluoxetine. Mol Psychiatry. 2018. https://doi.org/10.1038/s41380-018-0316-x .
Schouten M, et al. Circadian glucocorticoid oscillations preserve a population of adult hippocampal neural stem cells in the aging brain. Mol Psychiatry. 2019. https://doi.org/10.1038/s41380-019-0440-2 .
Kling MA, et al. Effects of electroconvulsive therapy on the CRH-ACTH-cortisol system in melancholic depression: preliminary findings. Psychopharmacol Bull. 1994;30:489–94.
CAS PubMed Google Scholar
Sternberg EM, Licinio J. Overview of neuroimmune stress interactions. Implications for susceptibility to inflammatory disease. Ann NY Acad Sci. 1995;771:364–71.
Article CAS Google Scholar
Bornstein SR, et al. Stress-inducible-stem cells: a new view on endocrine, metabolic and mental disease? Mol Psychiatry. 2019;24:2–9. https://doi.org/10.1038/s41380-018-0244-9 .
Article CAS PubMed Google Scholar
Rubin de Celis MF, et al. The effects of stress on brain and adrenal stem cells. Mol Psychiatry. 2016;21:590–3. https://doi.org/10.1038/mp.2015.230 .
Soares-Cunha C, et al. Nucleus accumbens medium spiny neurons subtypes signal both reward and aversion. Mol Psychiatry. 2019. https://doi.org/10.1038/s41380-019-0484-3 .
Pecina M, et al. Endogenous opioid system dysregulation in depression: implications for new therapeutic approaches. Mol Psychiatry. 2019;24:576–87, https://doi.org/10.1038/s41380-018-0117-2 .
Halikere A, et al. Addiction associated N40D mu-opioid receptor variant modulates synaptic function in human neurons. Mol Psychiatry. 2019. https://doi.org/10.1038/s41380-019-0507-0 .
Amare AT, et al. Bivariate genome-wide association analyses of the broad depression phenotype combined with major depressive disorder, bipolar disorder or schizophrenia reveal eight novel genetic loci for depression. Mol Psychiatry. 2019. https://doi.org/10.1038/s41380-018-0336-6 .
Coleman JRI, et al. Genome-wide gene-environment analyses of major depressive disorder and reported lifetime traumatic experiences in UK Biobank. Mol Psychiatry. 2020 https://doi.org/10.1038/s41380-019-0546-6 .
Belvederi Murri M, Amore M, Respino M, Alexopoulos GS. The symptom network structure of depressive symptoms in late-life: results from a European population study. Mol Psychiatry. 2018. https://doi.org/10.1038/s41380-018-0232-0 .
Article PubMed Google Scholar
Simmons WK, et al. Appetite changes reveal depression subgroups with distinct endocrine, metabolic, and immune states. Mol Psychiatry. 2018. https://doi.org/10.1038/s41380-018-0093-6 .
Article PubMed PubMed Central Google Scholar
Mulugeta A, Zhou A, King C, Hypponen E. Association between major depressive disorder and multiple disease outcomes: a phenome-wide Mendelian randomisation study in the UK Biobank. Mol Psychiatry. 2019. https://doi.org/10.1038/s41380-019-0486-1 .
Khandaker GM, et al. Shared mechanisms between coronary heart disease and depression: findings from a large UK general population-based cohort. Mol Psychiatry. 2019. https://doi.org/10.1038/s41380-019-0395-3 .
Wang YH, et al. Depression and anxiety in relation to cancer incidence and mortality: a systematic review and meta-analysis of cohort studies. Mol Psychiatry. 2019. https://doi.org/10.1038/s41380-019-0595-x .
Cooper CM, et al. Discovery and replication of cerebral blood flow differences in major depressive disorder. Mol Psychiatry. 2019. https://doi.org/10.1038/s41380-019-0464-7 .
van Velzen LS, et al. White matter disturbances in major depressive disorder: a coordinated analysis across 20 international cohorts in the ENIGMA MDD working group. Mol Psychiatry. 2019. https://doi.org/10.1038/s41380-019-0477-2 .
Greenberg T, et al. Reward related ventral striatal activity and differential response to sertraline versus placebo in depressed individuals. Mol Psychiatry. 2019. https://doi.org/10.1038/s41380-019-0490-5 .
Korgaonkar MS, Goldstein-Piekarski AN, Fornito A & Williams, LM Intrinsic connectomes are a predictive biomarker of remission in major depressive disorder. Mol Psychiatry. 2019. https://doi.org/10.1038/s41380-019-0574-2 .
Repple J, et al. Severity of current depression and remission status are associated with structural connectome alterations in major depressive disorder. Mol Psychiatry. 2019. https://doi.org/10.1038/s41380-019-0603-1 .
Nuninga JO, et al. Volume increase in the dentate gyrus after electroconvulsive therapy in depressed patients as measured with 7T. Mol Psychiatry. 2019. https://doi.org/10.1038/s41380-019-0392-6 .
Koch SBJ, Morey RA & Roelofs K. The role of the dentate gyrus in stress-related disorders. Mol Psychiatry. 2019. https://doi.org/10.1038/s41380-019-0572-4 .
Papakostas GI, et al. A phase 2, double-blind, placebo-controlled study of NSI-189 phosphate, a neurogenic compound, among outpatients with major depressive disorder. Mol Psychiatry. 2019. https://doi.org/10.1038/s41380-018-0334-8 .
Fava M, et al . Opioid system modulation with buprenorphine/samidorphan combination for major depressive disorder: two randomized controlled studies. Mol Psychiatry. 2018. https://doi.org/10.1038/s41380-018-0284-1 .
Fava M, et al. Correction: double-blind, placebo-controlled, dose-ranging trial of intravenous ketamine as adjunctive therapy in treatment-resistant depression (TRD). Mol Psychiatry. 2019. https://doi.org/10.1038/s41380-018-0311-2 .
Fava M, et al. Double-blind, placebo-controlled, dose-ranging trial of intravenous ketamine as adjunctive therapy in treatment-resistant depression (TRD). Mol Psychiatry. 2018. https://doi.org/10.1038/s41380-018-0256-5 (2018).
Licinio J. Advances in research on stress and behavior: special issue, 2020. Mol Psychiatry 2020;25:916–7. https://doi.org/10.1038/s41380-020-0741-5 .
Martinez ME, et al. Thyroid hormone overexposure decreases DNA methylation in germ cells of newborn male mice. Mol Psychiatry. 2020;25:915 https://doi.org/10.1038/s41380-020-0732-6 .
Martinez ME, et al. Thyroid hormone influences brain gene expression programs and behaviors in later generations by altering germ line epigenetic information. Mol Psychiatry. 2020;25:939–50. https://doi.org/10.1038/s41380-018-0281-4 .
Le-Niculescu H, et al. Towards precision medicine for stress disorders: diagnostic biomarkers and targeted drugs. Mol Psychiatry. 2020;25:918–38. https://doi.org/10.1038/s41380-019-0370-z .
Torres-Berrio A, et al. MiR-218: a molecular switch and potential biomarker of susceptibility to stress. Mol Psychiatry. 2020;25:951–64. https://doi.org/10.1038/s41380-019-0421-5
Sillivan SE, et al. Correction: MicroRNA regulation of persistent stress-enhanced memory. Mol Psychiatry. 2020;25:1154 https://doi.org/10.1038/s41380-019-0452-y .
Sillivan SE, et al. MicroRNA regulation of persistent stress-enhanced memory. Mol Psychiatry. 2020;25:965–76. https://doi.org/10.1038/s41380-019-0432-2 .
Shi MM, et al. Hippocampal micro-opioid receptors on GABAergic neurons mediate stress-induced impairment of memory retrieval. Mol Psychiatry. 2020;25:977–92. https://doi.org/10.1038/s41380-019-0435-z .
Mayo LM, et al. Protective effects of elevated anandamide on stress and fear-related behaviors: translational evidence from humans and mice. Mol Psychiatry. 2020;25:993–1005. https://doi.org/10.1038/s41380-018-0215-1 .
Qu N, et al. A POMC-originated circuit regulates stress-induced hypophagia, depression, and anhedonia. Mol Psychiatry. 2020;25:1006–21. https://doi.org/10.1038/s41380-019-0506-1 .
Fox ME, et al. Dendritic remodeling of D1 neurons by RhoA/Rho-kinase mediates depression-like behavior. Mol Psychiatry. 2020;25:1022–34. https://doi.org/10.1038/s41380-018-0211-5 .
Jin J, et al. Ahnak scaffolds p11/Anxa2 complex and L-type voltage-gated calcium channel and modulates depressive behavior. Mol Psychiatry. 2020;25:1035–49. https://doi.org/10.1038/s41380-019-0371-y .
Ben-Yehuda H, et al. Maternal Type-I interferon signaling adversely affects the microglia and the behavior of the offspring accompanied by increased sensitivity to stress. Mol Psychiatry. 2020;25:1050–67. https://doi.org/10.1038/s41380-019-0604-0 .
Pearson-Leary J, et al. The gut microbiome regulates the increases in depressive-type behaviors and in inflammatory processes in the ventral hippocampus of stress vulnerable rats. Mol Psychiatry. 2020;25:1068–79. https://doi.org/10.1038/s41380-019-0380-x .
Walker WH 2nd, et al. Acute exposure to low-level light at night is sufficient to induce neurological changes and depressive-like behavior. Mol Psychiatry. 2020;25:1080–93. https://doi.org/10.1038/s41380-019-0430-4 .
Lei Y, et al. SIRT1 in forebrain excitatory neurons produces sexually dimorphic effects on depression-related behaviors and modulates neuronal excitability and synaptic transmission in the medial prefrontal cortex. Mol Psychiatry. 2020;25:1094–111. https://doi.org/10.1038/s41380-019-0352-1 .
Sargin D, et al. Mapping the physiological and molecular markers of stress and SSRI antidepressant treatment in S100a10 corticostriatal neurons. Mol Psychiatry. 2020;25:1112–29. https://doi.org/10.1038/s41380-019-0473-6 .
Article Google Scholar
Iob E, Kirschbaum C, Steptoe A. Persistent depressive symptoms, HPA-axis hyperactivity, and inflammation: the role of cognitive-affective and somatic symptoms. Mol Psychiatry. 2020;25:1130–40. https://doi.org/10.1038/s41380-019-0501-6 .
Cabeza de Baca T, et al. Chronic psychosocial and financial burden accelerates 5-year telomere shortening: findings from the Coronary Artery Risk Development in Young Adults Study. Mol Psychiatry. 2020;25:1141–53. https://doi.org/10.1038/s41380-019-0482-5 .
Licinio J & Wong ML. Advances in depression research: special issue, 2020, with three research articles by Paul Greengard. Mol Psychiatry. 2020;25:1156–58. https://doi.org/10.1038/s41380-020-0781-x .
Teissier A, et al. Early-life stress impairs postnatal oligodendrogenesis and adult emotional behaviour through activity-dependent mechanisms. Mol Psychiatry. 2019. https://doi.org/10.1038/s41380-019-0493-2 .
Zhang Y, et al. CircDYM ameliorates depressive-like behavior by targeting miR-9 to regulate microglial activation via HSP90 ubiquitination. Mol Psychiatry. 2018. https://doi.org/10.1038/s41380-018-0285-0 .
Tan A, et al. Effects of the KCNQ channel opener ezogabine on functional connectivity of the ventral striatum and clinical symptoms in patients with major depressive disorder. Mol Psychiatry. 2018. https://doi.org/10.1038/s41380-018-0283-2 .
Kin K, et al. Cell encapsulation enhances antidepressant effect of the mesenchymal stem cells and counteracts depressive-like behavior of treatment-resistant depressed rats. Mol Psychiatry. 2018. https://doi.org/10.1038/s41380-018-0208-0 .
Orrico-Sanchez A, et al. Antidepressant efficacy of a selective organic cation transporter blocker in a mouse model of depression. Mol Psychiatry. 2019. https://doi.org/10.1038/s41380-019-0548-4 .
Han Y, et al. Systemic immunization with altered myelin basic protein peptide produces sustained antidepressant-like effects. Mol Psychiatry. 2019. https://doi.org/10.1038/s41380-019-0470-9 .
Wittenberg GM, et al. Effects of immunomodulatory drugs on depressive symptoms: a mega-analysis of randomized, placebo-controlled clinical trials in inflammatory disorders. Mol Psychiatry. 2019. https://doi.org/10.1038/s41380-019-0471-8 .
Beydoun MA, et al. Systemic inflammation is associated with depressive symptoms differentially by sex and race: a longitudinal study of urban adults. Mol Psychiatry. 2019. https://doi.org/10.1038/s41380-019-0408-2 .
Felger JC, et al. What does plasma CRP tell us about peripheral and central inflammation in depression? Mol Psychiatry. 2018. https://doi.org/10.1038/s41380-018-0096-3 .
Clark SL, et al. A methylation study of long-term depression risk. Mol Psychiatry. 2019. https://doi.org/10.1038/s41380-019-0516-z .
Aberg KA, et al. Methylome-wide association findings for major depressive disorder overlap in blood and brain and replicate in independent brain samples. Mol Psychiatry. 2018. https://doi.org/10.1038/s41380-018-0247-6 .
Wei YB, et al. A functional variant in the serotonin receptor 7 gene (HTR7), rs7905446, is associated with good response to SSRIs in bipolar and unipolar depression. Mol Psychiatry. 2019. https://doi.org/10.1038/s41380-019-0397-1 .
Licinio J. Molecular Psychiatry: the integration of molecular medicine and clinical psychiatry. Mol Psychiatry. 1996;1:1–3.
Steenblock C, et al. Severe acute respiratory syndrome coronavirus 2 (SARS-CoV-2) and the neuroendocrine stress axis. Mol Psychiatry. 2020. https://doi.org/10.1038/s41380-020-0758-9 .
Wong ML, Dong C, Andreev V, Arcos-Burgos M, Licinio J. Prediction of susceptibility to major depression by a model of interactions of multiple functional genetic variants and environmental factors. Mol Psychiatry. 2012;17:624–33. https://doi.org/10.1038/mp.2012.13 .
Article CAS PubMed PubMed Central Google Scholar
Lee SH, Paz-Filho G, Mastronardi C, Licinio J, Wong ML. Is increased antidepressant exposure a contributory factor to the obesity pandemic? Transl Psychiatry. 2016;6:e759 https://doi.org/10.1038/tp.2016.25 .
Bornstein SR, Licinio J. Improving the efficacy of translational medicine by optimally integrating health care, academia and industry. Nat Med. 2011;17:1567–9. https://doi.org/10.1038/nm.2583 .
Licinio J, Wong ML. Launching the ‘war on mental illness’. Mol Psychiatry. 2014;19:1–5. https://doi.org/10.1038/mp.2013.180 .
Download references
Author information
Authors and affiliations.
State University of New York, Upstate Medical University, Syracuse, NY, 13210, USA
Julio Licinio & Ma-Li Wong
You can also search for this author in PubMed Google Scholar
Corresponding author
Correspondence to Julio Licinio .
Additional information
Publisher’s note Springer Nature remains neutral with regard to jurisdictional claims in published maps and institutional affiliations.
Rights and permissions
Reprints and permissions
About this article
Cite this article.
Licinio, J., Wong, ML. Advances in depression research: second special issue, 2020, with highlights on biological mechanisms, clinical features, co-morbidity, genetics, imaging, and treatment. Mol Psychiatry 25 , 1356–1360 (2020). https://doi.org/10.1038/s41380-020-0798-1
Download citation
Published : 18 June 2020
Issue Date : July 2020
DOI : https://doi.org/10.1038/s41380-020-0798-1
Share this article
Anyone you share the following link with will be able to read this content:
Sorry, a shareable link is not currently available for this article.
Provided by the Springer Nature SharedIt content-sharing initiative
This article is cited by
Upregulation of carbonic anhydrase 1 beneficial for depressive disorder.
Acta Neuropathologica Communications (2023)
Introducing a depression-like syndrome for translational neuropsychiatry: a plea for taxonomical validity and improved comparability between humans and mice
- Iven-Alex von Mücke-Heim
- Lidia Urbina-Treviño
- Jan M. Deussing
Molecular Psychiatry (2023)
Reply to: “The serotonin theory of depression: a systematic umbrella review of the evidence” published by Moncrieff J, Cooper RE, Stockmann T, Amendola S, Hengartner MP, Horowitz MA in Molecular Psychiatry (2022 Jul 20. doi: 10.1038/s41380-022-01661-0)
- Lucie Bartova
- Rupert Lanzenberger
- Siegfried Kasper
Is the serotonin hypothesis/theory of depression still relevant? Methodological reflections motivated by a recently published umbrella review
- Hans-Jürgen Möller
- Peter Falkai
European Archives of Psychiatry and Clinical Neuroscience (2023)
The heterogeneity of late-life depression and its pathobiology: a brain network dysfunction disorder
- Kurt A. Jellinger
Journal of Neural Transmission (2023)
Quick links
- Explore articles by subject
- Guide to authors
- Editorial policies

- Open access
- Published: 28 November 2023
Prospective study of antidepressant treatment of psychiatric patients with depressive disorders: treatment adequacy and outcomes
- Johanna von Knorring 1 ,
- Ilya Baryshnikov 1 ,
- Pekka Jylhä 1 ,
- Tiina Talaslahti 1 ,
- Martti Heikkinen 1 &
- Erkki Isometsä 1
BMC Psychiatry volume 23 , Article number: 888 ( 2023 ) Cite this article
1021 Accesses
12 Altmetric
Metrics details
Despite numerous national depression care guidelines (DCGs), suboptimal antidepressant treatment may occur. We examined DCG concordance and depression treatment outcomes in psychiatric settings.
We evaluated treatment received and outcomes of 128 psychiatric out- and inpatients participating in the PEGAD (Pharmacoepidemiology and Pharmacogenetics of Antidepressant Treatment for Depressive Disorders) study at baseline, two weeks, and eight weeks using interviews and questionnaires. Inclusion criteria were ICD-10 diagnosis of a depressive disorder, a Patient Health Questionnaire-9 symptom (PHQ-9) score ≥ 10, and a new antidepressant prescribed. The primary outcome of the study was within-individual change in PHQ-9 scores.
At baseline, patients had predominately recurrent (83%) and in 19% treatment-resistant depression (TRD). The median preceding duration of the current episode was 6.5 months. At eight weeks, 85% of the patients (n = 107) used a DCG-concordant antidepressant dose. However, due to the scarcity of antidepressant combinations and augmentations, fewer TRD than non-TRD patients (25% vs. 84%, p < 0.005) received adequate antidepressant treatment. Additionally, one-third of the patients received inadequate follow-up. Overall, only 53% received treatment compatible with DCG recommendations for adequate pharmacotherapy and follow-up. The mean decline in PHQ-9 scores (-3.8 ± SD 5.7) was significant ( p < 0.0005). Nearly 40% of the patients reached a subthreshold level of depression (PHQ-9 < 10), predicted by a lower baseline PHQ-9 score, recurrent depression, and female sex. However, 45% experienced no significant clinical improvement (PHQ-9 score reduction < 20%).
Conclusions
Our findings suggest that inadequate treatment continues to occur in psychiatric care settings, particularly for TRD patients.
Peer Review reports
Introduction
Depression treatment should strive for full remission. The essential treatment option of antidepressants (ADs) is endorsed by numerous national depression care guidelines (DCGs), e.g. [ 1 , 2 , 3 , 4 ], particularly in moderate to severe depression, as robust evidence indicates their effectiveness and safety [ 5 ]. AD monotherapy is an evidence-based treatment option for acute depression and relapse prevention, which, following Finnish DCG recommendations [ 6 ], is predominately carried out in primary care. Patients with psychotic or treatment-resistant depression (TRD, defined as depression not responding to two or more adequately conducted AD monotherapy trials; [ 7 ]) and significant psychiatric comorbidity, functional disability, or suicidality should be referred to psychiatric care as they often require more complex pharmacotherapy, including AD combinations and augmentation with lithium or an antipsychotic [ 8 , 9 , 10 , 11 , 12 , 13 , 14 ]. To facilitate therapeutic decisions based on outcome predictors and observed treatment responses [ 15 , 16 , 17 ], DCGs endorse measurement-based care (MBC), i.e., routine monitoring of clinician- or patient-rated depression measures [ 18 , 19 , 20 ]. Thus, MBC provides clinicians with evidence-based tools for individualized depression treatment, additionally associated with better outcomes [ 19 , 20 , 21 ].
However, DCG-discordant or individually sub-optimized depression treatment is still an acknowledged problem in primary and psychiatric care settings [ 22 , 23 , 24 , 25 , 26 , 27 , 28 ]. Vigo et al. [ 29 ] concluded that only 10% of patients with major depressive disorder (MDD) received an adequate combination and implementation of pharmacotherapy and psychotherapy. Specifically, the main shortcomings of pharmacotherapy were underutilization and inadequate clinical monitoring of responses and side effects. A nationally representative survey study from the USA [ 30 ] found that less than one-third of non-remitted, AD-treated patients received augmentation treatment. One naturalistic European study [ 31 ] evaluating DCG adherence in outpatient care noted a scarcity of AD dose and medication changes, regardless of treatment outcome. Moreover, primary and specialized psychiatric care patients have been described as unexpectedly similar in depressive symptoms and depression severity [ 27 , 32 , 33 ], indicating possible clinical practice conflicting with DCG recommendations concerning referral to specialized care. Previously reported rates of depression treatment adequacy in psychiatric care have shown a wide variation between 31.0% and 94.4% [ 23 , 24 , 34 , 35 , 36 ]. However, there are very few studies investigating the concordance of treatments provided in psychiatric settings with DCG recommendations during the current era of guidelines and widespread AD use.
Earlier Finnish studies have also shown quality-of-care problems in depression treatment, e.g., treatment initiation delays, suboptimal treatment intensity, continuity challenges, and an indistinct division of labour between primary and psychiatric care [ 37 , 38 , 39 , 40 ]. Lähteenvuo et al. [ 41 ] recently reported on a nationwide register-based cohort that AD monotherapy was the most frequently initiated treatment, even among those TRD patients progressing to a fifth treatment trial after four previous monotherapies. This finding indicates likely non-adherence to DCG recommendations, as pharmacological combination or augmentation strategies are recommended after two failed monotherapy trials. Therefore, a more detailed study in a smaller sample is vital to broadening the understanding of current depression treatment practices and outcomes in psychiatric care settings. The Finnish DCG, first published in 2004, was recently updated in 2020. However, most studies published on the clinical practice of depression treatment in the Finnish public health care system date back more than a decade. Little is known about how treatment practices may have changed over the years. Additionally, when considering factors impacting depression care practice, we cannot ignore the 40% reduction in Finnish psychiatric hospital beds in recent years [ 42 ] and the increasing shortage of psychiatrists and other healthcare professionals in the public sector.
In this observational eight-week follow-up study, we aimed to examine Finnish DCG adherence in the psychiatric care settings of Helsinki University Hospital, Finland’s largest hospital district. Specifically, we aimed to describe (1) the clinical characteristics, prior course of illness, and treatment history of patients currently referred to psychiatric care, (2) the treatment received, focusing on AD use, and (3) the short-term treatment outcome. We expected treatment practice to align with the current recommendations, including appropriate AD dosage, treatment duration, and follow-up.
Study design and setting
This eight-week observational prospective cohort study is part of the PEGAD (Pharmacoepidemiology and pharmacogenetics of antidepressant treatment for depressive disorders) project, conducted within Helsinki University Hospital´s divisions of Acute Psychiatry, Mood Disorders, and Geropsychiatry. The Helsinki University Hospital catchment area provides adult psychiatric out- and inpatient services to Espoo, Kauniainen, Kirkkonummi, Vantaa, and Kerava, and geropsychiatric services to Helsinki and neighbouring cities, facilities from which clinicians recruited study patients between August 2018 and November 2019. The Ethics Committee of Helsinki University Hospital and the Finnish Medicines Agency (FIMEA) approved the study protocol. All recruited patients gave written informed consent. The study was based on clinical diagnoses by attending psychiatrists responsible for providing patients’ usual care. During follow-up patients received treatment as usual for their depressive disorder.
Inclusion and exclusion criteria
The inclusion criteria were as follows: (1) an ICD-10 (International Statistical Classification of Diseases and Related Health Problems 10th Revision) [ 43 ] diagnosis of Depressive episode (F32) or Recurrent depressive disorder (F33), (2) a Patient Health Questionnaire-9 (PHQ-9) [ 44 ] score ≥ 10, (3) a new AD prescribed, and (4) age ≥ 18 years. The exclusion criteria were (1) a principal clinical diagnosis other than depression, (2) current psychotic symptoms, (3) immediate suicide risk, and (4) involuntary hospitalization.
Evaluation and scales
We evaluated patients at three time points: baseline, two weeks, and eight weeks.
At baseline , research nurses collected sociodemographic and clinical data using interviews and instructed patients to answer the self-report questionnaires. Symptom and treatment history of current major depressive episode (MDE) was based on patients’ recollection. Patients were asked structured questions to estimate the time points for initial signs and symptoms reaching the level of clinical depression. For each AD reportedly used before study participation, we enquired when the patients had used it, the duration of its use, its highest dosage, and the reason for its discontinuation.
The Columbia–Suicide Severity Rating Scale (C-SSRS) [ 45 ] was used to identify and rate suicidal ideation and behaviour occurring after the initial screening and admittance to the study.
The self-report scales included the PHQ-9, the Overall Anxiety and Impairment Scale (OASIS) [ 46 ], the Mood Disorder Scale (MDQ) [ 47 ], the Snaith-Hamilton Pleasure scale (SHAPS) [ 48 ], the Sheehan Disability Scale (SDS) [ 49 ], the Alcohol Use Disorders Identification Test (AUDIT) [ 50 ], questions on illegal drug use, the Fagerström Test for Nicotine Dependence (FTND) [ 51 ], and the McLean Screening Instrument for Borderline Personality Disorder (MSI-BPD) [ 52 ].
At two weeks , patients received an email inquiry asking if they still used the AD described at baseline. Additionally, patients were asked to fill in the PHQ-9, OASIS, and MDQ.
A t eight weeks , research nurses conducted interviews concerning treatment received and patient adherence during follow-up. Drug use was specified by asking about all current psychiatric and somatic medication (dosage and time of last use) and psychiatric medication used during the preceding week. The patients also filled in the PHQ-9, OASIS, SHAPS, SDS, and MDQ.
Primary outcome
The primary outcome of depression treatment was the within-individual change in PHQ-9 symptom scores between baseline and the eight-week time point. We also divided patients into groups according to baseline depression severity, examining the change in PHQ-9 symptom scores between baseline and the eight-week time point specifically in each group.
Minimally adequate treatment
The overall treatment received during the study was classified as “minimally adequate” for non-TRD patients when the following criteria were met: (1) receiving AD for two months and having an adequate treatment dose (defined by the Finnish DCG) at the eight-week time point and (2) including at least two follow-up visits at the treating facility. “Minimally adequate” treatment for TRD patients had the additional criterion of receiving AD combination or pharmacological augmentation treatment.
Statistical analysis
As the amount of missing symptom scale data was small (data available on request), we used the mean substitution method to address missing data. We used the Chi-square and Fisher Exact tests to examine associations between categorical variables and the Mann-Whitney test to compare continuous variables between the ICD-10 specific diagnosis groups. The effects of independent baseline variables on the likelihood of reaching a PHQ-9 value < 10 at the eight-week time point were examined using a multivariate logistic regression model. We performed all analyses using the SPSS program [ 53 ].
Patient sampling
We excluded three of the 131 patients recruited for technical reasons, resulting in a baseline patient number of 128. Ninety-one patients (71.1%) provided data at two weeks and 107 (83.6%) at eight weeks. Most (n = 113; 88.3%) were outpatients at the time of recruitment, and the rest (n = 15; 11.7%) were inpatients. All follow-ups were conducted in outpatient care. A minority of participants (n = 16; 12.5%) were psychogeriatric patients (age > 65 years).
Patient characteristics
The majority (n = 85; 66.4%) of the 128 patients were women, predominantly (n = 54; 63.5%) unmarried, separated, or widowed. Women had a higher educational level (p = 0.047) and were more likely to be a part of the active workforce ( p < 0.001) than men (Table 1 ). Patients who dropped out after the baseline evaluation (n = 21) did not differ in their baseline clinical characteristics from those remaining in follow-up (data available on request).
Prior course of Illness
Most (n = 106; 82.8%) of the 128 patients were diagnosed with recurrent depression with a median of three episodes (Table 2 ). The median age for all patients’ first MDE was 17 years (range 6 to 83 years, mean 24 years). Patients had suffered from their current MDE for a median of 6.5 months, but 19.5% (n = 25) had a chronic index episode of two years or longer. Patients with recurrent depression reported a shorter duration of symptoms (median 5.5 months vs. 16.0 months, p = 0.005) and had sought treatment faster (median five months vs. six months, p = 0.048) than first-episode patients. First-episode depression was also associated with a likelihood of a chronic index episode (p = 0.014) but not with a more severe level of depression at baseline. TRD patients did not differ from others regarding the baseline severity of depression, anxiety symptoms, or functional impairment, nor were they associated with chronic medical problems (data available on request).
Treatment received for current depressive episode (index episode) before baseline
Ninety-one (71.1%) of the 128 patients had used ADs for their current episode before study participation, half of whom (n = 46; 50.5%) had only one AD, with no significant difference between recurrent and first-episode depression patient groups. Commonly used ADs were escitalopram (n = 43), bupropion (n = 23), mirtazapine (n = 21), and venlafaxine (n = 17). Among the 128 patients, 18.8% (n = 24) had not responded to at least two adequately conducted AD trials before entering the study and were identified as TRD patients.
Before baseline, 21.1% (n = 27) of the patients attended psychotherapy, and over half (n = 72; 56.3%) reported attending supportive discussions with a health care professional. However, 25% (n = 32) had no regular treatment contact for their current depression before referral to psychiatric care.
Treatment received during the study
We present an overview of the treatment received during follow-up in Table 3 . In accordance with the inclusion criteria, all 128 study patients were prescribed an AD at baseline. The most prescribed ADs were escitalopram (n = 22), bupropion and venlafaxine (n = 18 each), duloxetine (n = 17), and sertraline (n = 16). At eight weeks, 90.7% (n = 97) of the 107 patients remaining in the follow-up reported currently using ADs, 93.8% (n = 91) of whom had a dosage within the therapeutic range recommended by the Finnish DCG. Thirty (30.9%) of the 97 patients using ADs used an AD combination. Quetiapine was the only atypical antipsychotic used for AD augmentation, prescribed to nine patients (9.3%), one of whom also received augmentation with lamotrigine.
Of the 107 patients finishing the study, roughly 1/3 had no or only one follow-up visit, 1/3 had two visits, and 1/3 had more than two visits (median for all patients was 2 visits, range 0 to 24 visits). Psychiatrists met the study patients on average once during the follow-up, but 30.8% (n = 33) of patients did not meet their treating psychiatrist after the initial meeting. However, three patients had multiple follow-up visits (18, 20, and 24 visits), mainly with a psychiatric nurse, together constituting 17.2% of all follow-up visits among study patients. All three patients suffered recurrent depression, classified as severe for one, moderate for one, and mild for one. None of these three patients reported acute suicidality. The TRD and non-TRD patients did not differ in the frequency of follow-up visits.
Altogether 53.3% of all patients received overall treatment classified as “minimally adequate”, including adequate pharmacological treatment and follow-up. As the Finnish DCG recommendations differ for the TRD and non-TRD patients, we examined the adequacy of treatment received separately for these two patient groups.
Treatment of non-TRD patients
Eighty-seven non-TRD patients (83.7%) finished the study, at which point most (n = 79; 90.8%) used ADs. Of the 79 non-TRD patients using ADs, 92.4% (n = 73) had a DCG-concordant treatment dosage, 32.9% (n = 26) used an AD combination, and 10.1% (n = 8) received AD augmentation with quetiapine, one additionally with lamotrigine. Furthermore, one non-TRD patient received electroconvulsive therapy (ECT) during follow-up.
Accounting for suboptimal follow-up, while 83.9% (n = 73) of all non-TRD patients completing the study received DCG-recommended AD treatment, only 60.9% (n = 53) received overall treatment meeting our definition of “minimally adequate”.
Treatment of TRD patients
Four TRD patients dropped out of the study, resulting in 83.3% (n = 20) being evaluated at eight weeks. Most (n = 18; 90%) used ADs, all of whom had a DCG-concordant AD treatment dosage. However, 77.8% (n = 14/18) used AD monotherapy. Consequently, as only four TRD patients used AD combinations, and one received augmentation using quetiapine, this resulted in 25% (n = 5) of all TRD patients receiving DCG-recommended pharmacological treatment for depression. Compared with the non-TRD patients, a significantly smaller proportion received adequate pharmacotherapy for their depression (25% vs. 83.9%, p < 0.005).
Altogether 20% (n = 4) of the 20 TRD patients evaluated at eight weeks had received treatment meeting the criteria for “minimally adequate,” i.e., having a DCG-concordant AD dosage at eight weeks, using pharmacological augmentation or an AD combination, and having had two or more follow-up visits at the treating facility. Overall treatment adequacy was therefore significantly lower (p = 0.002) than in non-TRD patients.
Patient outcomes
Our primary outcome was the within-individual change in PHQ-9 scores (Fig. 1 ). A significant difference ( p < 0.0005) of 3.8 points emerged between PHQ-9 scores at baseline and eight weeks. At eight weeks, 10.3% (n = 11) of patients remaining in the study had a PHQ-9 score of ≤ 4 points, considered full remission, and 39.3% (n = 42) had a score of < 10 points, indicating subthreshold symptoms or remission.
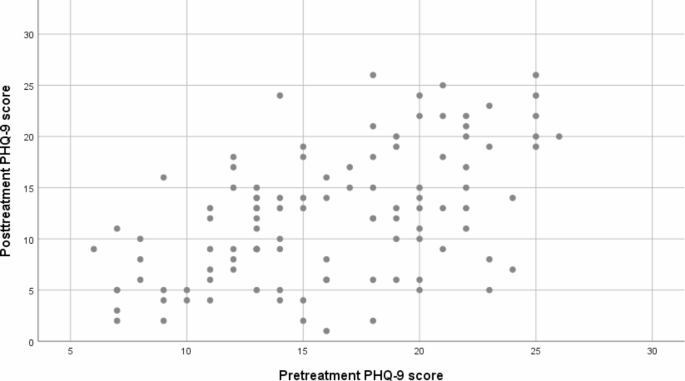
Scatter plot of PHQ-9 scores before and after treatment in psychiatric patients with depressive disorders. PHQ-9, Patient Health Questionnaire
Self-report scale results are presented in Table 4 . Mean PHQ-9 scores at baseline indicated moderately severe depressive symptoms (16 ± 5.1), decreasing to a mild level (12.2 ± 6.3) over the eight-week follow-up ( p = 0.005). However, when patients were divided into groups according to baseline depression severity, wide variations emerged. At baseline, 12.5% (n = 16) reported subthreshold depressive symptoms (PHQ-9 < 10), 31.3% (n = 40) reported mild symptoms (PHQ-9 10–14), 28.9% (n = 37) reported moderate symptoms (PHQ 15–19), and 27.3% (n = 35) reported severe symptoms (PHQ-9 ≥ 20). The PHQ-9 score change at eight weeks reached statistical significance for all baseline depression severity groups with a baseline PHQ-9 score ≥ 10 (Fig. 2 ).
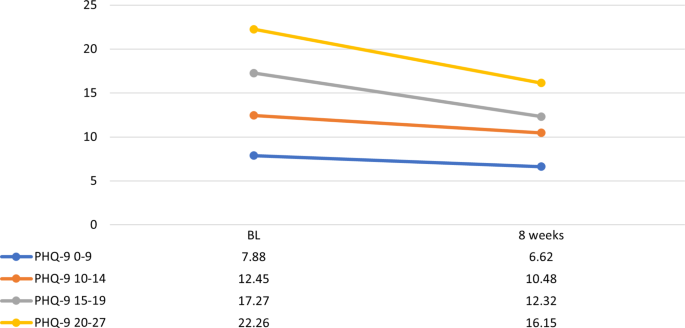
Change in PHQ-9 scores in psychiatric patients with depressive disorders. Group division based on baseline depression severity. Y-axis: PHQ-9 scores, X-axis: time points at baseline (BL) and 8 weeks. PHQ-9, Patient Health Questionnaire; PHQ-9 0–9, mild depression; PHQ-9 10–14, moderate depression; PHQ-9 15–19, moderately severe depression; PHQ-9 ≥ 20, severe depression
We ran a multivariate logistic regression model to ascertain the effects of eight independent variables (age, sex, baseline values of PHQ-9, OASIS, AUDIT, and MSI, the variables indicating either first episode or recurrent depression, and AD dosage as either adequate or not) on the likelihood of reaching a PHQ-9 value < 10. The model was statistically significant, X^2(8) = 31.080, p < 0.005, explaining 39.2% of the variance and correctly classifying 79.6% of the cases (sensitivity 62.5%, specificity 88.5%, PPV 74.1%, and NPV 81.8%). Among the independent variables, only three were statistically significant: baseline PHQ-9 (B=-0.251, p = 0.001), the variable marking first episode vs. recurrent depression (F32 or F33) (B = 2.904, p = 0.009), and sex (B=-1.436, p = 0.032). Better odds of reaching a subthreshold level of depression symptoms (PHQ-9 < 10) were associated with a lower PHQ-9 value at baseline (OR 0.778, 95% CI: 0.669–0.906), having a diagnosis of recurrent depression (OR 18.246, 95% CI: 2.038- 163.345), and being female (OR 0.238, 95% CI: 0.064–0.885).
Finally, we examined the effect of treatment adequacy on PHQ-9 score change for TRD and non-TRD patients separately. Despite some numerical differences, no significant PHQ-9 score reduction was seen in favour of adequate treatment in either patient group ( p = 0.12 for TRD patients and p = 0.09 for non-TRD patients).
This study examined current treatment practice of depression, focusing on AD use in adult psychiatry units of Helsinki University Hospital, Finland. At baseline, most study patients had recurrent, moderately severe depression, and 19% had TRD. Roughly one-third had not received any AD treatment for their current MDE before referral to psychiatric care. During the eight-week follow-up, half of all patients received treatment meeting our minimum requirements for medication and follow-up. However, only few TRD patients received DCG-recommended AD combinations or pharmacological augmentation. Despite reported symptom relief and a significant mean decline in PHQ-9 scores, individual score reductions indicated modest treatment outcomes.
Patient characteristics and treatment history
Our typical patient was an educated female in her late thirties, referred to psychiatric care for her third, moderately severe depressive episode. Most of our study patients were diagnosed with recurrent depression, 19% with TRD, and 20%, predominately first-episode patients, had a chronic index episode with depression lasting two years or longer.
The mean baseline level of depression was less severe than expected. Initially, all patients had a PHQ-9 score ≥ 10. However, the study screening and the baseline evaluation did not always coincide, resulting in treatment initiation before evaluation in some patients. Baseline symptom ratings may also have been affected by pharmacological treatment initiated shortly before referral, causing symptom reductions while waiting for access to specialized psychiatric care.
Comparing patient characteristics with previously reported findings of patients in Finnish psychiatric care settings [ 40 , 54 ], we find broadly similar sex and age distributions and mean severity of depression. We also see noteworthy differences compared with these previous studies. First, our patients seemed to suffer from a more persistent course of depression. Our study patients had their first depressive episode at a younger age, suffered more likely from recurrent depression, and had a longer symptomatic period of their current MDE before the baseline interview. Second, prior AD use was rarer than in Vuorilehto et al. [ 40 ], as nearly one-third of patients had not used any ADs for their current MDE before referral to psychiatric care.
Our finding of prolonged delay in referral to psychiatric care could reflect Finnish DCG recommendations of staging between different levels of care settings. However, it does not justify the lack of treatment trials in primary care. We did not find an explanation, such as a more severe mean level of depression or acute suicidality, for why so many patients were referred to psychiatric care without prior AD treatment. We do not know whether the failure to meet DCG recommendations is related to their not being appropriately conveyed, or to a scarcity of health care resources. We found some patient-related characteristics possibly influencing help-seeking behaviour, e.g., housing type (living alone vs. others) and lower employment rate, compared with earlier findings [ 40 , 54 ].
While expecting a higher proportion of TRD among patients in psychiatric care, 19% is still somewhat higher than the 11% suggested in a Finnish nationwide register cohort [ 41 ], and it is within the range of prevalences found in other recent studies (e.g., [ 55 , 56 , 57 , 58 , 59 ]). Also, as some patients failed to provide information on AD dosing or duration, affecting the identification of prior adequate treatment trials, the actual proportion of TRD may have been higher. Hence, our ratio of TRD patients indicates that those deemed challenging to treat in primary care are at least partly correctly referred to psychiatric care.
Treatment during follow-up
Depression chronicity and recurrence increase the probability of poor outcomes, both acute and long-term [ 60 , 61 , 62 , 63 , 64 ]. Therefore, characteristics associated with poor outcomes, namely TRD, should alert clinicians to consider treatment enhancement. However, our findings indicate that this may have been overlooked in our study patients’ treatment planning and follow-up.
The results regarding mere AD use or the bare minimum of follow-up visits appear acceptable. However, due to non-implemented DCG-recommended AD combination and augmentation strategies, the observed pharmacological treatment of TRD patients can be considered substandard. Follow-up visits were unevenly distributed among patients. One-third did not meet their psychiatrist in follow-up, yet a few patients received disproportionally many visits overall, which was not explained by depression severity or acute suicidality. Half of all patients and only 20% of TRD patients received treatment considered “minimally adequate”, meaning adequately combined pharmacological treatment and follow-up.
Our findings of current depression treatment indicate a clear gap between guideline recommendations and clinical practice but resonate with earlier results [ 27 , 36 , 41 , 65 ]. Nevertheless, the now-observed quality-of-care problems warrant attention. Concerning treatment augmentation strategies, the DCG presents AD combinations or pharmacological augmentation as plausible options when treating TRD in psychiatric care. However, as patients are referred from primary to psychiatric care in severe situations requiring expertise, we consider the expectation of using AD combinations or pharmacological augmentation in treating TRD justified. Although some TRD patients may benefit from additional AD monotherapy trials [ 66 ], medication augmentation strategies are recommended to enhance outcomes [ 67 , 68 , 69 , 70 , 71 ]. In our study, AD combinations were equally likely to be administered to TRD and non-TRD patients, and augmentation pharmacotherapy was strikingly sparse in both patient groups. We do not know whether symptom rating scales were used during each visit to guide clinicians’ treatment decisions, but clearly, TRD patients did not receive DCG-recommended drug enhancement as a rule. Simply put, a similar treatment regimen was used for all patients, regardless of their medical history.
Explanations for DCG-discordant treatment practice might include unawareness of patients’ symptom and treatment history and, therefore, failure to recognize TRD. Staff shortages may affect the quality of care, and the clinical supervision of doctors in training, decreasing the awareness of DCG recommendations in primary and psychiatric care. Also, patients may be wary of using multiple pharmaceuticals or substances not primarily used for MDD for fear of side effects and stigmatization. However, patients and caregivers must be educated to recognize the risks of prolonged ineffective treatment.
Therefore, proper psychoeducation should not be overlooked as a stepping stone in depression treatment. We need to share information with patients on disabilities and poor outcomes connected to depression chronicity and recurrence, justifying enhanced treatment options when considered necessary.
Overall, symptom rating scores indicated significant improvement in all measured entities. However, measured by PHQ-9 score reductions, only 10% of patients reached depression remission and 30% treatment response. Notably, nearly half of the patients experienced no treatment response. These findings of suboptimal treatment outcomes cannot be overlooked despite the relatively short study duration of eight weeks and the uncertainty of effective dose adjustment for a maximum response during follow-up.
The finding of a substantial proportion of non-responders may relate to clinical characteristics, such as depression chronicity in the form of a longer symptomatic period, evident at baseline. Chronicity was seen notably in the first-episode patients. In contrast, patients with recurrent depression showed faster initiation of treatment and better treatment results. Our result suggests an accentuation of chronicity compared with earlier findings from a Finnish psychiatric care cohort [ 54 ]. In this earlier study, treatment response was mainly seen within six months of treatment initiation in psychiatric care, after which recovery was sparse. Other studies also stress the effectiveness of treatment early in illness [ 63 , 72 ], that a greater illness burden reflects on the treatment required, and that results diminish for each treatment step needed [ 60 , 66 ].
We observed PHQ-9 scores decreasing more between baseline and the two-week time point than in the last six weeks of follow-up. The reason was not clarified but may relate to an unspecified reaction to treatment initiation. However, as early treatment response has been associated with better outcomes [ 16 , 73 ], the possible predictive nature of this clinical factor should be noted in follow-up. Nevertheless, this finding highlights the importance of repeated symptom and response tracking throughout the first weeks and months of acute depression treatment to ensure treatment intensification if needed.
Study strengths and limitations
This study aimed at a representative sample of patients with depressive disorders referred to psychiatric care with a PHQ-9 score ≥ 10 and a new AD initiated. Detailed data were collected during an eight-week prospective study using in-person interviews and standardized evaluation scales. This study highlights the flow of patients from primary to specialized psychiatric care and follows patients in usual treatment. Therefore, we describe current, real-life patient characteristics and depression treatment strategies used in psychiatric care.
There are some limitations to consider. First, the number of patients recruited was moderate (n = 131). Second, we did not collect data on the number of patients declining participation or the reason for this, both limitations affecting the generalizability of the results. However, given the similarity of characteristics between our cohort and other comparable psychiatric cohorts within the Helsinki University Hospital area [ 40 , 54 ], we find it unlikely that this would have resulted in a marked selection bias concerning the AD treatment provided to our study patients. In our view, possible selection bias may have enriched adherent doctors and patients, and patients without characteristics necessitating urgent clinical measures such as imminent suicide risk. Also, similar patient characteristics and suboptimal treatment practices have been reported in a recent nationwide register-based cohort study [ 41 ], further supporting our findings. Third, data collection on the prior course of illness and treatment received relied on patients’ recollections, a source for possible data inaccuracy. For example, missing data on AD dosing and duration of use affected the recognition of adequate treatment trials, likely resulting in a slight underestimation of TRD prevalence. Fourth, despite longitudinal follow-up, data collection focused on the current cross-sectional state of two and eight weeks, limiting the information available on daily medication adherence, planned and realized dose changes between research time points. Therefore, even if meeting the therapeutic dose criteria of the DCG, the AD dosage could be suboptimal at an individual level. Finally, we also compared outcomes between patients with adequate vs. inadequate treatment and found no significant difference. However, this was a non-randomized, observational study from which causal inferences from the role of treatment on the observed outcome are not justified.
Our results suggest that inadequate treatment of depression continues to occur in psychiatric care settings. Observed treatment outcomes were modest, and only 10% of patients reached remission. AD treatment was lacking particularly for TRD patients, as only 25% received DCG-recommended AD combinations or pharmacological augmentation. The intensity of treatment monitoring was inadequate for one-third of all patients.
Considering the escalating health burden caused by depressive disorders impacting the economy and personal loss, these findings of suboptimal treatment practices warrant attention. Based on our findings, we stress the importance of structured data collection and use of MBC to ensure quality of all treatment for depression.
Data Availability
The datasets generated and analysed during the current study are not publicly available due to restrictions imposed by the Finnish data protection legislation and research permits but are available from the corresponding author on reasonable request.
Abbreviations
- Antidepressant
The Alcohol Use Disorders Identification Test
The Columbia–Suicide Severity Rating Scale
Depression care guideline
The Fagerström Test for Nicotine Dependence
International Statistical Classification of Diseases and Related Health Problems 10th Revision
Measurement-based care
Major depressive disorder
Major depressive episode
The Mood Disorder Scale
The McLean Screening Instrument for Borderline Personality Disorder
The Overall Anxiety and Impairment Scale
The Patient Health Questionnaire-9
The Sheehan Disability Scale
The Snaith-Hamilton Pleasure scale
Treatment resistant depression
Association AP, APA. (2010) Practice guidelines for the treatment of patients with major depressive disorder. Am Psychiatric Association. http://www.psychiatryonline.org/pb/assets/raw/sitewide/practice_guidelines/guidelines/mdd.pdf .
Kennedy SH, Lam RW, Mcintyre RS, Tourjman SV, Bhat V, Blier P, et al. Canadian Network for Mood and anxiety treatments (CANMAT) 2016 clinical guidelines for the management of adults with major depressive disorder. Can J Psychiatry. 2016;61(9):540–60.
Article PubMed PubMed Central Google Scholar
Malhi GS, Bell E, Bassett D, Boyce P, Bryant R, Hazell P, et al. The 2020 Royal Australian and New Zealand College of Psychiatrists clinical practice guidelines for mood disorders. Australian & New Zealand Journal of Psychiatry. 2021;55(1):7–117.
Article Google Scholar
[NG222] Ng. Depression in adults: treatment and management [Available from: https://www.nice.org.uk/guidance/ng222 .
Cipriani A, Furukawa TA, Salanti G, Chaimani A, Atkinson LZ, Ogawa Y, et al. Comparative efficacy and acceptability of 21 antidepressant Drugs for the acute treatment of adults with major depressive disorder: a systematic review and network meta-analysis. The Lancet. 2018;391(10128):1357–66.
Article CAS Google Scholar
Duodecim TFMS, Working group set up by the Finnish Medical Society Duodecim and the Finnish Psychiatric Association. Depression. Current Care Guidelines. Helsinki: The Finnish Medical Society Duodecim, 2022 (referred 13.2.2023). Available online at: www.kaypahoito.fi.
Gaynes BN, Lux L, Gartlehner G, Asher G, Forman-Hoffman V, Green J, et al. Defining treatment‐resistant Depression Depression and Anxiety. 2020;37(2):134–45.
PubMed Google Scholar
Gaynes BN, Rush AJ, Trivedi MH, Wisniewski SR, Spencer D, Fava M. The STAR*D study: treating depression in the real world. Cleve Clin J Med. 2008;75(1):57–66.
Article PubMed Google Scholar
Gaynes BN, Dusetzina SB, Ellis AR, Hansen RA, Farley JF, Miller WC, et al. Treating Depression after initial treatment failure: directly comparing switch and augmenting strategies in STAR*D. J Clin Psychopharmacol. 2012;32(1):114–9.
Henssler J, Alexander D, Schwarzer G, Bschor T, Baethge C. Combining antidepressants vs antidepressant monotherapy for treatment of patients with Acute Depression. JAMA Psychiatry. 2022;79(4):300–12.
Mishra A, Sarangi SC, Maiti R, Sood M, Reeta K. Efficacy and safety of adjunctive serotonin-dopamine activity modulators in Major Depression: a Meta‐Analysis of Randomized controlled trials. J Clin Pharmacol. 2022;62(6):721–32.
Article CAS PubMed Google Scholar
Scott F, Hampsey E, Gnanapragasam S, Carter B, Marwood L, Taylor RW et al. Systematic review and meta-analysis of augmentation and combination treatments for early-stage treatment-resistant depression. J Psychopharmacol. 2022;2698811221104058.
Taylor RW, Marwood L, Oprea E, DeAngel V, Mather S, Valentini B, et al. Pharmacological augmentation in Unipolar Depression: a guide to the guidelines. Int J Neuropsychopharmacol. 2020;23(9):587–625.
Vázquez GH, Bahji A, Undurraga J, Tondo L, Baldessarini RJ. Efficacy and tolerability of combination treatments for Major Depression: antidepressants plus Second-Generation antipsychotics vs. esketamine vs. Lithium J Psychopharmacol. 2021;35(8):890–900.
De Carlo V, Calati R, Serretti A. Socio-demographic and clinical predictors of non-response/non-remission in treatment resistant depressed patients: a systematic review. Psychiatry Res. 2016;240:421–30.
Perlman K, Benrimoh D, Israel S, Rollins C, Brown E, Tunteng J-F, et al. A systematic meta-review of predictors of antidepressant treatment outcome in major depressive disorder. J Affect Disord. 2019;243:503–15.
Simon GE, Perlis RH. Personalized Medicine for Depression: can we Match patients with treatments? Am J Psychiatry. 2010;167(12):1445–55.
Trivedi MH. Tools and strategies for Ongoing Assessment of Depression. J Clin Psychiatry. 2009;70(suppl 6):26–31.
Xiao L, Qi H, Zheng W, Xiang Y-T, Carmody TJ, Mayes TL, et al. The effectiveness of enhanced evidence-based care for depressive disorders: a meta-analysis of randomized controlled trials. Translational Psychiatry. 2021;11(1):531.
Zhu M, Hong RH, Yang T, Yang X, Wang X, Liu J, et al. The efficacy of measurement-based Care for Depressive disorders. J Clin Psychiatry. 2021;82(5):21r14034.
Guo T, Xiang Y-T, Xiao L, Hu C-Q, Chiu HFK, Ungvari GS, et al. Measurement-based Care Versus Standard Care for Major Depression: a Randomized Controlled Trial with Blind Raters. Am J Psychiatry. 2015;172(10):1004–13.
Duhoux A, Fournier L, Nguyen CT, Roberge P, Beveridge R. Guideline concordance of treatment for depressive disorders in Canada. Soc Psychiatry Psychiatr Epidemiol. 2009;44(5):385–92.
Fernández A, Haro Jm Fau - Codony M, Codony M, Fau - Vilagut G, Vilagut G, Fau - Martínez-Alonso M, Martínez-Alonso MF, Autonell J, Autonell JF, Salvador-Carulla L, et al. Treatment adequacy of anxiety and depressive disorders: primary versus specialised care in Spain. J Affect Disord. 2006;96(1–2):9–20.
Kessler RC, Berglund P, Demler O, Jin R, Koretz D, Merikangas KR, et al. The epidemiology of major depressive disorder. JAMA. 2003;289(23):3095–105.
Lisinski A, Hieronymus F, Eriksson E, Wallerstedt SM. Low SSRI dosing in clinical practice—a register-based longitudinal study. Acta Psychiatrica Scandinavica. 2021;143(5):434–43.
Schneider F, Kratz S, Bermejo I, Menke R, Mulert C, Hegerl U, et al. Insufficient depression treatment in outpatient settings. Ger Med Sci. 2004;2:Doc01.
PubMed PubMed Central Google Scholar
Simon GE, Von Korff M, Rutter CM, Peterson DA. Treatment process and outcomes for Managed Care patients receiving New Antidepressant prescriptions from psychiatrists and Primary Care Physicians. Arch Gen Psychiatry. 2001;58(4):395–401.
Thornicroft G, Chatterji S, Evans-Lacko S, Gruber M, Sampson N, Aguilar-Gaxiola S, et al. Undertreatment of people with major depressive disorder in 21 countries. Br J Psychiatry. 2017;210(2):119–24.
Vigo D, Haro JM, Hwang I, Aguilar-Gaxiola S, Alonso J, Borges G, et al. Toward measuring effective treatment coverage: critical bottlenecks in quality- and user-adjusted coverage for major depressive disorder. Psychol Med. 2022;52(10):1948–58.
Mojtabai R, Amin-Esmaeili M, Spivak S, Olfson M. Remission and treatment augmentation of Depression in the United States. J Clin Psychiatry. 2021;82(6):21m13988.
Herzog DP, Wagner S, Ruckes C, Tadic A, Roll SC, Härter M, et al. Guideline adherence of antidepressant treatment in outpatients with major depressive disorder: a naturalistic study. Eur Arch Psychiatry Clin NeuroSci. 2017;267(8):711–21.
Gaynes BN, Rush AJ, Trivedi M, Wisniewski SR, Balasubramani GK, Spencer DC, et al. A direct comparison of presenting characteristics of depressed outpatients from primary vs. specialty care settings: preliminary findings from the STAR*D clinical trial. Gen Hosp Psychiatry. 2005;27(2):87–96.
Gaynes BN, Rush AJ, Trivedi MH, Wisniewski SR, Balasubramani GK, Spencer DC, et al. Major depression symptoms in Primary Care and Psychiatric Care settings: a cross-sectional analysis. The Annals of Family Medicine. 2007;5(2):126–34.
Castro-Rodríguez JI, Olariu E, Garnier-Lacueva C, Martín-López LM, Pérez-Solà V, Alonso J, et al. Diagnostic accuracy and adequacy of treatment of depressive and anxiety disorders: a comparison of primary care and specialized care patients. J Affect Disord. 2015;172:462–71.
Gabilondo A, Rojas-Farreras S, Rodráguez A, Ferníndez A, Pinto-Meza A, Vilagut G, et al. Use of primary and specialized Mental Health Care for a major depressive episode in Spain by ESEMeD respondents. Psychiatric Serv. 2011;62(2):152–61.
Wang PS, Lane M, Olfson M, Pincus HA, Wells KB, Kessler RC. Twelve-Month Use of Mental Health Services in the United States. Arch Gen Psychiatry. 2005;62(6):629–40.
Kasteenpohja T, Marttunen M, Aalto-Setälä T, Perälä J, Saarni SI, Suvisaari J. Treatment received and treatment adequacy of depressive disorders among young adults in Finland. BMC Psychiatry. 2015;15(1):47.
Melartin TK, Rytsälä HJ, Leskelä US, Lestelä-Mielonen PS, Sokero TP, Isometsä ET. Continuity is the main challenge in treating major depressive disorder in psychiatric care. J Clin Psychiatry. 2005;66:220–7.
Rytsälä HJ, Melartin MD, Leskelä TKMD, US MA, Lestelä-Mielonen PS, Sokero MAT, Isometsä PMD. ET, M.D., Ph.D. A Record-Based Analysis of 803 Patients Treated for Depression in Psychiatric Care. J Clin Psychiatry. 2001;62:701-6.
Vuorilehto MS, Melartin TK, Rytsälä HJ, Isometsä ET. Do characteristics of patients with major depressive disorder differ between primary and psychiatric care? Psychol Med. 2007;37(6):893–904.
Lähteenvuo M, Taipale H, Tanskanen A, Rannanpää S, Tiihonen J. Courses of treatment and risk factors for treatment-resistant depression in Finnish primary and special healthcare: a nationwide cohort study. J Affect Disord. 2022;308:236–42.
Linnaranta O. Selvitys psykiatristen sairaalapaikkojen määrästä. Terveyden ja hyvinvoinnin laitos THL; 2022. https://urn.fi/URN:ISBN:978-952-343-820-0 .
WHO. World Health Organization. International classification of Disease. 10th ed. Geneva: WHO; 1992.
Google Scholar
Kroenke K, Spitzer RL, Williams JBW. The PHQ-9. J Gen Intern Med. 2001;16(9):606–13.
Article CAS PubMed PubMed Central Google Scholar
Posner K, Brown GK, Stanley B, Brent DA, Yershova KV, Oquendo MA, et al. The Columbia–Suicide severity rating scale: initial validity and internal consistency findings from three Multisite studies with adolescents and adults. Am J Psychiatry. 2011;168(12):1266–77.
Norman SB, Hami Cissell S, Means-Christensen AJ, Stein MB. Development and validation of an overall anxiety severity and impairment scale (OASIS). Depress Anxiety. 2006;23(4):245–9.
Hirschfeld RMA. The Mood Disorder Questionnaire. The primary care companion to the. J Clin Psychiatry. 2002;04(01):9–11.
Snaith RP, Hamilton M, Morley S, Humayan A, Hargreaves D, Trigwell P. A scale for the Assessment of Hedonic Tone the Snaith–Hamilton pleasure scale. Br J Psychiatry. 1995;167(1):99–103.
Sheehan KH, Sheehan DV. Assessing treatment effects in clinical trials with the discan metric of the Sheehan disability scale. Int Clin Psychopharmacol. 2008;23(2):70–83.
de Meneses-Gaya C, Zuardi AW, Loureiro SR, Crippa JAS. Alcohol Use Disorders Identification Test (AUDIT): an updated systematic review of psychometric properties. Psychol Neurosci. 2009;2:83–97.
Heatherton TF, Kozlowski LT, Frecker RC, Fagerstrom K-O. The Fagerstrom Test for Nicotine Dependence: a revision of the Fagerstrom Tolerance Questionnaire. Addiction. 1991;86(9):1119–27.
CAS Google Scholar
Zanarini MC, Vujanovic AA, Parachini EA, Boulanger JL, Frankenburg FR, Hennen J. A screening measure for BPD: the Mclean Screening Instrument for Borderline personality disorder (MSI-BPD). J Personal Disord. 2003;17(6):568–73.
Statistics IS, for Windows V, Corp I. (Released 2017). IBM SPSS statistics for Windows, Version 25.0. Armonk, NY: IBM Corp. In.
Melartin TK, Rytsälä HJ, Leskelä US, Lestelä-Mielonen PS, Sokero TP, Isometsä ET. Severity and comorbidity predict episode duration and recurrence of DSM-IV major depressive disorder. J Clin Psychiatry. 2004;65(6):810–9.
Fife D, Feng Y, Wang MY-H, Chang C-J, Liu C-Y, Juang H-T, et al. Epidemiology of pharmaceutically treated depression and treatment resistant depression in Taiwan. Psychiatry Res. 2017;252:277–83.
Gronemann FH, Jorgensen MB, Nordentoft M, Andersen PK, Osler M. Incidence of, risk factors for, and Changes over Time in Treatment-Resistant Depression in Denmark: a Register-based Cohort Study. J Clin Psychiatry. 2018;79(4):17m11845.
Hägg D, Brenner P, Reutfors J, Li G, Dibernardo A, Bodén R, et al. A register-based approach to identifying treatment-resistant depression—comparison with clinical definitions. PLoS ONE. 2020;15(7):e0236434.
Mahlich J, Tsukazawa S, Wiegand F. Estimating prevalence and Healthcare utilization for treatment-resistant depression in Japan: a retrospective claims database study. Drugs - Real World Outcomes. 2018;5(1):35–43.
Gronemann FH, Jorgensen MB, Nordentoft M, Andersen PK, Osler M. Treatment-resistant depression and risk of all-cause mortality and suicidality in Danish patients with major depression. J Affect Disord. 2021;287:204–13.
Fekadu A, Wooderson SC, Markopoulo K, Donaldson C, Papadopoulos A, Cleare AJ. What happens to patients with treatment-resistant depression? A systematic review of medium to long term outcome studies. J Affect Disord. 2009;116(1–2):4–11.
Jonsson U, Bohman H, von Knorring L, Olsson G, Paaren A, von Knorring AL. Mental health outcome of long-term and episodic adolescent depression: 15-year follow-up of a community sample. J Affect Disord. 2011;130(3):395–404.
Pettit JW, Lewinsohn PM, Roberts RE, Seeley JR, Monteith L. The long-term course of depression: development of an empirical index and identification of early adult outcomes. Psychol Med. 2009;39(3):403–12.
Rush AJ, Wisniewski SR, Zisook S, Fava M, Sung SC, Haley CL, et al. Is prior course of Illness relevant to acute or longer-term outcomes in depressed out-patients? A STAR*D report. Psychol Med. 2012;42(6):1131–49.
Satyanarayana S, Enns MW, Cox BJ, Sareen J. Prevalence and correlates of Chronic Depression in the Canadian Community Health Survey: Mental Health and Well-Being. Can J Psychiatry. 2009;54(6):389–98.
Fernández A, Haro JM, Martinez-Alonso M, Demyttenaere K, Brugha TS, Autonell J, et al. Treatment adequacy for anxiety and depressive disorders in six European countries. Br J Psychiatry. 2007;190(2):172–3.
Rush AJ, Trivedi MH, Wisniewski SR, Nierenberg AA, Stewart JW, Warden D, et al. Acute and longer-term outcomes in depressed outpatients requiring one or several treatment steps: a STAR*D report. Am J Psychiatry. 2006;163(11):1905–17.
Bennabi D, Charpeaud T, Yrondi A, Genty J-B, Destouches S, Lancrenon S, et al. Clinical guidelines for the management of treatment-resistant depression: French recommendations from experts, the French Association for Biological Psychiatry and Neuropsychopharmacology and the fondation FondaMental. BMC Psychiatry. 2019;19(1):262.
Nuñez NA, Joseph B, Pahwa M, Kumar R, Resendez MG, Prokop LJ, et al. Augmentation strategies for treatment resistant major depression: a systematic review and network meta-analysis. J Affect Disord. 2022;302:385–400.
Strawbridge R, Carter B, Marwood L, Bandelow B, Tsapekos D, Nikolova VL, et al. Augmentation therapies for treatment-resistant depression: systematic review and meta-analysis. Br J Psychiatry. 2019;214(1):42–51.
Zhou X, Keitner GI, Qin B, Ravindran AV, Bauer M, Del Giovane C, et al. Atypical antipsychotic augmentation for treatment-resistant depression: a systematic review and network Meta-analysis. Int J Neuropsychopharmacol. 2015;18(11):pyv060.
Zhou X, Ravindran AV, Qin B, Del Giovane C, Li Q, Bauer M, et al. Comparative efficacy, acceptability, and tolerability of augmentation agents in treatment-resistant depression: systematic review and network meta-analysis. J Clin Psychiatry. 2015;76(4):e487–98.
Kaymaz N, van Os J, Loonen AJ, Nolen WA. Evidence that patients with single versus recurrent depressive episodes are differentially sensitive to treatment discontinuation: a meta-analysis of placebo-controlled randomized trials. J Clin Psychiatry. 2008;69(9):1423–36.
Szegedi A, Jansen WT, van Willigenburg AP, van der Meulen E, Stassen HH, Thase ME. Early improvement in the first 2 weeks as a predictor of treatment outcome in patients with major depressive disorder: a meta-analysis including 6562 patients. J Clin Psychiatry. 2009;70(3):344–53.
Download references
Acknowledgements
We would like to thank Drs. Ritva Arajärvi, Hannu Koponen, Katariina Lönnfors and Kari Raaska plus Lic. Psychol Petri Näätänen for their help in collecting the PEGAD data, and professors Janne Backman, Mikko Niemi and Tiina Paunio for their contributions to the PEGAD study design.
Open Access funding provided by University of Helsinki (including Helsinki University Central Hospital).
The PEGAD was funded by a grant (TYH2018307) from the Helsinki University Hospital.
Author information
Authors and affiliations.
Department of Psychiatry, University of Helsinki and Helsinki University Hospital, P.O. Box 22, Helsinki, FI-00014, Finland
Johanna von Knorring, Ilya Baryshnikov, Pekka Jylhä, Tiina Talaslahti, Martti Heikkinen & Erkki Isometsä
You can also search for this author in PubMed Google Scholar
Contributions
EI conceptualized the study design and data collection and is the PI of the study. JvK and IB analyzed and interpreted the patient data. JvK drafted the manuscript, to which IB and EI provided critical revisions and gave valuable input on the interpretation of results.PJ, TT and MH participated in collecting data. All authors have critically commented and approved the final manuscript.
Corresponding author
Correspondence to Erkki Isometsä .
Ethics declarations
Ethics approval and consent to participate.
The Ethics Committee of Helsinki University Hospital and the Finnish Medicines Agency (FIMEA) approved the study protocol in 2018. All recruited patients gave written informed consent.
Consent for publication
Not applicable.
Competing interests
Dr. Heikkinen has participated as a researcher in clinical trials of mood disorder pharmacotherapies sponsored by Janssen. The other authors declare that they have no conflicts of interest.
Additional information
Publisher’s note.
Springer Nature remains neutral with regard to jurisdictional claims in published maps and institutional affiliations.
Rights and permissions
Open Access This article is licensed under a Creative Commons Attribution 4.0 International License, which permits use, sharing, adaptation, distribution and reproduction in any medium or format, as long as you give appropriate credit to the original author(s) and the source, provide a link to the Creative Commons licence, and indicate if changes were made. The images or other third party material in this article are included in the article’s Creative Commons licence, unless indicated otherwise in a credit line to the material. If material is not included in the article’s Creative Commons licence and your intended use is not permitted by statutory regulation or exceeds the permitted use, you will need to obtain permission directly from the copyright holder. To view a copy of this licence, visit http://creativecommons.org/licenses/by/4.0/ . The Creative Commons Public Domain Dedication waiver ( http://creativecommons.org/publicdomain/zero/1.0/ ) applies to the data made available in this article, unless otherwise stated in a credit line to the data.
Reprints and permissions
About this article
Cite this article.
von Knorring, J., Baryshnikov, I., Jylhä, P. et al. Prospective study of antidepressant treatment of psychiatric patients with depressive disorders: treatment adequacy and outcomes. BMC Psychiatry 23 , 888 (2023). https://doi.org/10.1186/s12888-023-05390-8
Download citation
Received : 15 February 2023
Accepted : 21 November 2023
Published : 28 November 2023
DOI : https://doi.org/10.1186/s12888-023-05390-8
Share this article
Anyone you share the following link with will be able to read this content:
Sorry, a shareable link is not currently available for this article.
Provided by the Springer Nature SharedIt content-sharing initiative
- Psychiatric care
- Treatment adequacy
- Treatment outcome
BMC Psychiatry
ISSN: 1471-244X
- Submission enquiries: [email protected]
- General enquiries: [email protected]
- Registered report
- Open access
- Published: 05 June 2020
Psychotherapy or medication for depression? Using individual symptom meta-analyses to derive a Symptom-Oriented Therapy (SOrT) metric for a personalised psychiatry
- Nils Kappelmann ORCID: orcid.org/0000-0002-2923-4455 1 , 2 ,
- Martin Rein 1 ,
- Julia Fietz 1 , 2 ,
- Helen S. Mayberg 3 , 4 ,
- W. Edward Craighead 4 , 5 ,
- Boadie W. Dunlop 4 ,
- Charles B. Nemeroff 6 ,
- Martin Keller 7 ,
- Daniel N. Klein 8 ,
- Bruce A. Arnow 9 ,
- Nusrat Husain 10 ,
- Robin B. Jarrett 11 ,
- Jeffrey R. Vittengl 12 ,
- Marco Menchetti 13 ,
- Gordon Parker 14 ,
- Jacques P. Barber 15 ,
- Andre G. Bastos 16 ,
- Jack Dekker 17 ,
- Jaap Peen 17 ,
- Martin E. Keck 1 &
- Johannes Kopf-Beck 1
BMC Medicine volume 18 , Article number: 170 ( 2020 ) Cite this article
21k Accesses
19 Citations
46 Altmetric
Metrics details
Antidepressant medication (ADM) and psychotherapy are effective treatments for major depressive disorder (MDD). It is unclear, however, if treatments differ in their effectiveness at the symptom level and whether symptom information can be utilised to inform treatment allocation. The present study synthesises comparative effectiveness information from randomised controlled trials (RCTs) of ADM versus psychotherapy for MDD at the symptom level and develops and tests the Symptom-Oriented Therapy (SOrT) metric for precision treatment allocation.
First, we conducted systematic review and meta-analyses of RCTs comparing ADM and psychotherapy at the individual symptom level. We searched PubMed Medline, PsycINFO, and the Cochrane Central Register of Controlled Trials databases, a database specific for psychotherapy RCTs, and looked for unpublished RCTs. Random-effects meta-analyses were applied on sum-scores and for individual symptoms for the Hamilton Rating Scale for Depression (HAM-D) and Beck Depression Inventory (BDI) measures.
Second, we computed the SOrT metric, which combines meta-analytic effect sizes with patients’ symptom profiles. The SOrT metric was evaluated using data from the Munich Antidepressant Response Signature (MARS) study ( n = 407) and the Emory Predictors of Remission in Depression to Individual and Combined Treatments (PReDICT) study ( n = 234).
The systematic review identified 38 RCTs for qualitative inclusion, 27 and 19 for quantitative inclusion at the sum-score level, and 9 and 4 for quantitative inclusion on individual symptom level for the HAM-D and BDI, respectively. Neither meta-analytic strategy revealed significant differences in the effectiveness of ADM and psychotherapy across the two depression measures. The SOrT metric did not show meaningful associations with other clinical variables in the MARS sample, and there was no indication of utility of the metric for better treatment allocation from PReDICT data.
Conclusions
This registered report showed no differences of ADM and psychotherapy for the treatment of MDD at sum-score and symptom levels. Symptom-based metrics such as the proposed SOrT metric do not inform allocation to these treatments, but predictive value of symptom information requires further testing for other treatment comparisons.
Peer Review reports
“Major depressive disorder (MDD) is a debilitating disease” is one of the most frequent introductory phrases in psychiatric literature and rightfully so (e.g. [ 1 , 2 , 3 , 4 , 5 ]). Its lifetime prevalence varies across countries between 5.5 and 21% [ 6 , 7 ], and it is estimated to be the second leading cause for years lived with disability [ 8 ], leaving no doubt about the importance and urgency of developing effective treatments. While research on potential new treatments such as esketamine and anti-inflammatory drugs is underway [ 9 , 10 , 11 ], antidepressant medication (ADM) and psychotherapy offer effective treatments for a majority of patients as shown in recent high-quality meta-analyses [ 12 , 13 , 14 ]. As evidence is mainly based on between-group comparisons (e.g. drug versus placebo), the specific patient-treatment match often remains unclear. Specifically, as patients react differently towards specific treatments in clinical practice, they often need to “cycle through” different types of ADM and/or psychotherapy to find the treatment that will eventually help them personally [ 15 , 16 , 17 ]. Consequently, there is an increasing awareness in psychiatric and psychotherapy research for the necessity of more personalised treatment approaches that align with Paul’s old but important question: “What works for whom?” [ 18 ].
Attempts have been made and are underway towards establishing such personalised psychiatric care. These cover a wide range of different approaches such as using big data sets and machine learning models to predict the MDD course from baseline self-reports [ 19 , 20 ], explorative data-mining strategies in order to define decision trees for the treatment of depression [ 21 ], algorithm-based treatments associated with shorter treatment time [ 15 ], imaging-based functional connectivity indices for treatment selection [ 22 ], or statistical strategies to examine superiority between treatments depending on stratification variables [ 23 , 24 ]. Among the latter is a promising attempt by DeRubeis and colleagues who developed the Personalised Advantage Index (PAI) by re-analysing data from a randomised controlled trial (RCT) of cognitive behavioural therapy (CBT) versus ADM [ 25 ]. The PAI constitutes the predicted difference in how patients would have benefitted from the treatment they received to the treatment they did not receive. In order to estimate the PAI for each patient across treatments, the authors used regression-based models with prognostic and prescriptive (treatment-moderating) variables as predictor variables and depression sum-scores at study endpoint (8 weeks) as outcome.
Four more recent studies on the treatment of depression have also estimated the PAI in RCTs of sertraline versus placebo [ 26 ], cognitive therapy versus interpersonal therapy [ 27 ], CBT versus psychodynamic therapy [ 28 ], and continuation-phase cognitive therapy versus fluoxetine for relapse prevention [ 29 ]. Throughout these studies, findings indicate that patients randomised to their optimal treatment (as suggested by the PAI) had clinically significantly better improvements in depression symptoms. Variables moderating treatment effects differed between studies and ranged from sociodemographic factors over life events up to personality and specific problems, symptoms, temperament, and comorbid conditions [ 25 , 26 , 27 , 28 , 29 ]. Beyond the PAI and analyses of single RCTs, individual patient data (IPD) meta-analysis work is currently underway by Weitz and colleagues who are trying to identify treatment moderators that indicate a combination of ADM and psychotherapy as more effective than monotherapy [ 30 ]. These studies thus describe strategies of using pre-treatment variables to inform treatment allocation. Nevertheless, the range of different, hardly overlapping variables between different studies stresses the sample dependency of results. It further reveals the necessity of replication in a prospective design to determine the clinical utility of stratified treatment allocation.
Besides the lack of prospective studies, we argue that previous studies potentially miss out on making use of the heterogeneity present within the construct of MDD. Specifically, all aforementioned studies using the PAI defined reductions in sum-scores on depression scales as the singular outcome variable to indicate treatment efficacy. Yet, individual depressive symptoms load differently on overall psychosocial impairment [ 31 ], so that clinical severity might not be best expressed by summing over individual depression scores [ 32 ]. This phenomenon gets aggravated when considering how low the content overlap in symptoms is between prominent depression scales [ 33 ]. Additionally, symptom combinations in MDD, for instance as defined by the Diagnostic and Statistical Manual of Mental Disorders 5th edition (DSM-5), allow two patients to present with a completely diverging symptom profile when considering the diametrically opposed symptoms such as insomnia and hypersomnia [ 34 ] within that compound symptom. This is not only of concern theoretically but a recent descriptive study in 3703 patients has estimated that of 1030 unique symptom combinations the most frequent symptom combination was only present in 1.8% of patients [ 35 ].
Utilising heterogeneity in symptom expression could offer additional, complementary insights for personalised treatment allocation of main treatments for depression. Empirically, two recent investigations have attempted to use information on symptom expression to differentiate effects of main treatments in re-analyses of RCTs comparing ADM and psychotherapy. In the first study, the authors compared treatment effects on distinct symptom clusters and results showed that cognitive therapy was better than both ADM and placebo in improving atypical vegetative symptoms (weight gain/increased appetite and hypersomnia) while there was no difference in clusters of (i) mood, (ii) cognitive/suicidal, (iii) anxiety, and (iv) typical vegetative symptoms (weight loss/decreased appetite and insomnia) [ 36 ]. These analysed symptom clusters still rely on assumptions of common factors underlying MDD, however, which has been criticised in recent research [ 37 ] and is suggestive of a move towards individual symptom analyses. Here, another re-analysis of individual symptom data indicated that ADM was better at reducing suicidality than CBT [ 38 ]. This latter study, however, was potentially underpowered for individual symptom analyses, and meta-analytic strategies could offer a more thorough approach. Nonetheless, these studies constitute promising attempts at delineating more symptom-specific treatment effects and are aided by other findings that show, for instance, that ADM efficacy as compared to placebo is most pronounced for the “depressed mood” symptom specifically [ 39 ].
In the current investigation, we wanted to develop and test what we term a Symptom-Oriented Therapy (SOrT) metric that aspires to quantify potential preference (or not) of ADM or psychotherapy similar to the PAI. Contrary to the PAI, however, the SOrT metric is not based on pre-treatment (moderating) variables but instead makes use of patients’ heterogeneity in symptom expression. Additionally, the SOrT metric is not based on re-analyses of individual RCT data but instead on effect size estimates obtained in meta-analyses across a number of RCTs to avoid dependency of results on individual study samples. In particular, we wanted to conduct a systematic review and meta-analysis with the aim of synthesising existing evidence from RCTs comparing ADM and psychotherapy in the treatment of depression. While we also aimed to update existing meta-analytic evidence of these treatments on depression sum-scores, a previously used strategy [ 40 , 41 , 42 ], our primary aim was to analyse treatment efficacy on individual depressive symptoms. As prior RCTs have largely measured depressive symptoms using the Hamilton Depression Rating Scale (here, HAM-D; also commonly abbreviated as HRSD or HDRS) and/or Beck’s Depression Inventory (BDI) [ 40 ], we focussed our investigation on these two scales.
Following this overview of symptom-based treatment differences and similarities, we then aimed to compute the meta-analysis-based SOrT metric to indicate preference (or not) for a specific treatment type (i.e. ADM or psychotherapy) at the individual-patient level. This was evaluated using both data from an existing depressed inpatient sample, the Munich Antidepressant Response Signature study [ 43 ], and data from a previous RCT of ADM versus psychotherapy, the Emory Predictors of Remission in Depression to Individual and Combined Treatments (PReDICT) study [ 44 ]. Here, the primary test of our metric was to compare patients allocated to optimal versus non-optimal treatment in the PReDICT study as defined using the SOrT metric. Our primary hypothesis was that patients receiving their optimal treatment had significantly better outcomes on depressive symptoms than those receiving non-optimal treatment. We hoped that developing the SOrT metric based on our results would be particularly useful for future researchers (and ultimately clinicians) to test and individualise treatments for patients suffering from MDD. In this way, our results could provide a more refined view on heterogeneity of MDD and hopefully move the field closer towards personalised medicine.
Step 1: Systematic review and meta-analysis of RCTs of ADM versus psychotherapy
Protocol registration.
The protocol for this systematic review was registered on PROSPERO (identifier: CRD42019123905) [ 45 ].
Search strategy and study selection
We systematically searched PubMed Medline, PsycINFO, and Cochrane Central Register of Controlled Trials (CENTRAL) databases for randomised controlled trials (RCTs) of psychotherapy versus ADM in the treatment of depression. The search terms are presented in detail in Additional file 1 : Table S1 in respective database grammar and align with the following search terms for PubMed Medline:
(depression[MeSH Terms] OR depressive disorder[MeSH Terms] OR mood disorder[MeSH Terms] OR affective* OR depress*) AND (psychotherapy [mh] OR (Cogniti* AND (technique* OR therap* OR restructur* OR challeng*)) AND (Antidepressive Agents [Pharmacological Action] OR agents, antidepressive[MeSH Terms] OR SSRI OR SNRI OR TCA OR “selective serotonin reuptake inhibitor” OR “selective norepinephrine inhibitor” OR “tricyclic antidepressant”) AND (randomized controlled trial[pt] OR controlled clinical trial[pt] OR randomized [tiab] OR randomly [tiab] OR trial [tiab] OR groups [tiab])
In addition to search of databases, we searched 352 RCTs mentioned on http://www.evidencebasedpsychotherapies.org/ , a database created for psychotherapy RCTs and comparative trials [ 46 ]; hand-searched reference lists of retrieved RCTs and conference abstracts; searched for and, if necessary, contacted authors with published trial protocols; and wrote to prominent authors for unpublished data.
NK and JKB screened titles and abstracts of articles for the following inclusion and exclusion criteria: RCTs were required to (i) have at least one arm each for (individual and/or group) psychotherapy and ADM during part of the trial (e.g. crossover studies were allowed if data can be extracted before crossover), (ii) measure depressive symptoms using the Beck Depression Inventory (BDI) or Hamilton Depression Rating Scale (HAM-D), (iii) investigate adult depression, (iv) investigate major depression as a primary diagnosis without major medical comorbidity, (v) describe ethical approval and ascertainment of written informed consent, and (vi) include patients aged 18–75 years. For studies in languages other than English, we decided on a case-by-case basis whether we had the resources for translation of articles, which did not lead to further exclusions.
Risk of bias assessment
In order to assess the quality of RCTs and risk of bias, we evaluated included studies using the gold standard Cochrane Risk of Bias tool [ 47 ] while making specific adaptations of the tool to the context of psychotherapy research as was recently suggested by Munder and Barth [ 48 ]. The Cochrane Risk of Bias tool includes assessments of (i) random sequence generation, (ii) allocation concealment, (iii) blinding of participants and personnel, (iv) blinding of outcome assessment, (v) incomplete outcome data, (vi) selective reporting, and (vii) other biases. As blinding of participants and personnel is impossible in ADM versus psychotherapy comparisons, we assessed how differential expectations of patients and personnel about treatment were handled. Here, we focussed on bias being introduced by way of the study design, because wait-list control and combined treatment (i.e. ADM and psychotherapy) might bias participants towards expecting less or more treatment effects than single treatments (ADM or psychotherapy), respectively. Additionally, we assessed “bias due to deviations from intended interventions” (e.g. changes in treatment adherence or integrity), which has recently been added to the Cochrane Risk of Bias tool 2.0 and is particularly relevant for complex interventions like psychotherapy [ 48 , 49 ]. First, NK and JKB independently assessed included studies for their risk of bias. In a second step, assessments were compared and inconsistencies discussed and resolved.
Data acquisition and extraction
The main outcome for systematic review and meta-analyses were individual symptom data. Of note, individual symptom data—as required for our analyses—are not equal to individual patient data (IPD). While IPD have one row per participant, the format we required for our analyses is similar to frequency tables per symptom per treatment group; that is, one row per symptom, one column per symptom severity increment (e.g. 0, 1, 2, and 3 for all 21 BDI items) per treatment group, and cells indicating the number of patients indicating this symptom severity at end of treatment.
As depression outcome is usually not reported on the described individual symptom level, which was, however, required for our analyses, we only expected to be able to obtain a subset of data through extraction from manuscripts. The extracted variables from manuscripts were (i) study design and population, (ii) type and dosage of psychotherapy and ADM, (iii) study duration and follow-up, (iv) depression questionnaires used, (v) sample sizes of treatment arms at baseline and study endpoint, (vi) means and standard deviations of depression (sum-scores) at least at baseline and study endpoint (BDI and/or HAM-D), (vii) comorbidities (axis I and II disorders), (viii) age and sex of study participants, (ix) inclusion and exclusion criteria, (x) handling of missing data, dropouts, and use of intention-to-treat analysis, and (xi) researcher allegiance (i.e. main investigators’ training). NK and JKB independently extracted these data and subsequently discussed and resolved inconsistencies. If relevant data were only present in graph format and we did not get a response from authors, data were extracted using a reliable software tool [ 50 ].
Contacting authors for data (esp. individual symptom data) was also done in a standardised manner to maximise available data for this investigation (Additional file 2 ).
Statistical analysis
The effect size for meta-analysis of depression is usually the standardised mean difference (SMD) when using sum-scores of depression scales. Although we wanted to provide an updated quantitative synthesis of depression sum-scores in the comparison of psychotherapy and pharmacotherapy using SMDs, our primary aim was to evaluate treatment differences at the individual symptom level. The SMD, however, does not address the potential non-normality of individual symptom items, which are on an ordinal but not necessarily interval scale. To that end, we have chosen to calculate proportional odds ratios (pORs) as effect sizes of first choice; these are appropriate for ordinal data if the proportionality assumption is met (i.e. if steps in symptom severity are similar) [ 51 , 52 ]. pORs might be thought of as average ORs of all possible item severity steps (e.g. from 0 to 1, from 1 to 2, etc.).
In addition to pORs, we also presented analyses based on SMDs and "normal" ORs (after median split of symptom items) as sensitivity analysis. While SMDs and normal ORs are not ideal for this type of meta-analysis due to potentially violated assumptions and loss of power, respectively, they are more commonly used than pORs, so might offer additional insights to readers and highlight potential method dependency and robustness of the results. To allow comparability between metrics, we converted between (p)ORs and SMDs (referred to in results as converted (p)ORs and converted SMDs) according to the formula SMD = ln(OR)/1.81 [ 53 ]. As BDI and HAM-D have partially overlapping symptoms, we also performed a “spill over” sensitivity analysis by comparing individual symptom effect sizes between these scales for all comparable items. For instance, meta-analyses of “feelings of guilt” items in BDI and HAM-D should show comparable effect sizes towards ADM, psychotherapy, or neither. Additional file 3 : Tables S2-S3 highlight scale differences and similarities on the item level, which authors NK and JKB have evaluated with reference to a prior content analysis of depression scales [ 33 ]. In addition to BDI and HAM-D comparability, we also expected differences in the BDI, depending on whether BDI-I or BDI-II was used. Comparable items of BDI-I and BDI-II were aggregated if possible but separated if necessary (Additional file 3 : Table S5).
Meta-analyses were conducted using the metafor package [ 54 ] in R [ 55 ]. For meta-analyses, effects were weighted based on study sample size (i.e. inverse variance method) and we used a random-effects approach. Of note, we only analysed available data at the study endpoint (i.e. completer data) as intention-to-treat approaches for individual symptom items would have made analyses too complex and we were not aware of any specific procedures for individual symptom items. We hoped, however, that missing data at study endpoint (e.g. through dropout) was less of a problem for our comparison of two active treatments, which should have had relatively more similar levels of dropout as compared to, for instance, studies with wait-list control arm.
We investigated heterogeneity between studies using Cochrane’s Q and the I 2 statistic. Publication bias was tested for meta-analysis of the sum-score depression outcome by visual funnel plot inspection and using Egger’s test [ 56 ].
Step 2: Development and validation of the Symptom-Oriented Therapy (SOrT) metric
Computation of the sort metric.
If our meta-analyses of individual depressive symptoms demonstrated treatment differences between ADM and psychotherapy, this would have potential benefit for the development of individualised treatment. To that end, we wished to provide researchers with a meta-analysis-based tool to compute what we term Symptom-Oriented Therapy (SOrT) metric. This metric is based on the quantitative results from meta-analyses, which served as weightings for patients’ individual depressive symptoms (on either HAM-D or BDI). Specifically, computation of the SOrT metric followed the formula SOrT = ∑ i m i s i , where m equals the meta-analytic effect size (favouring ADM or psychotherapy) as (converted) SMD, s equals the symptom score on BDI or HAM-D, and i equals a specific symptom item. Of note, m is defined as converted SMD and not pOR because SMDs follow a linear scale centred at zero, which allows more feasible computation and interpretation. See Additional file 4 for a detailed rationale and discussion of the SOrT metric, potential extensions of the formula, and hypothetical computation examples (Additional file 4 : Table S5).
Validation in MARS study
We computed SOrT scores for patients who took part in the MARS study, a subset of which has data available on both the BDI and HAM-D measures and was targeted in this validation step ( n = 407). The MARS project was a naturalistic inpatient study of MDD patients conducted between years 2000 and 2015 at the Max Planck Institute of Psychiatry (Munich, Germany), the Bezirkskrankenhaus Augsburg (Germany), and the Klinikum Ingolstadt (Germany) [ 43 ]. Participating patients received various psychological assessments, and genetic and neuroendocrine measures were obtained with the goal of identifying (i) drug response predictors and (ii) subgroups of patients with similar biological pathophysiology.
We (i) provided descriptive statistics of patients’ SOrT scores for BDI and HAM-D based on the MARS psychiatric inpatient sample, (ii) compared these on important clinical and demographic characteristics, and, most importantly, (iii) cross-validated the SOrT metric for BDI and HAM-D. This cross-validation was performed by computing (i) correlations between SoRT scores of scales and (ii) differences of BDI and HAM-D SOrT scores on an individual person level, respectively. Based on this, we were able to determine potential scale dependency or independency of the SOrT metric, which, in turn, provided an indication of its value as a tool for further research.
Validation in PReDICT study
We computed SOrT scores for patients who took part and were randomised in the PReDICT study ( n = 344) and performed analyses on all patients who completed the RCT ( n = 234). PReDICT was an RCT comparing CBT versus escitalopram versus duloxetine for the treatment of depression [ 44 , 57 ]. For validation and in line with the setup of this investigation, ADM groups of escitalopram and duloxetine were pooled. SOrT score computation was done using meta-analytic effect estimates based on all retrieved studies except the PReDICT study, so that validation of the clinical utility of our metric was done independently from the meta-analytic “training” samples. Similar to the approach for the PAI used by DeRubeis et al. [ 25 ], we divided patients into those who received their optimal treatment (i.e. the SOrT score valence matches the treatment group) versus those who received their non-optimal treatment (i.e. the SOrT score valence does not match the treatment group). Doing this allowed us to compare outcome at end of treatment as suggested by HAM-D sum-scores of the optimal versus non-optimal groups and test for significant differences using a simple independent samples t test. In a second step, we followed the same procedure with a more restrictive subsample that was more extreme in their SOrT scores. Specifically, we used the two-thirds of patients with more extreme SOrT scores and compared optimal versus non-optimal treatment allocation groups. These procedures were applied both for SOrT scores created from BDI and for SOrT scores created from HAM-D. In sum, this allowed us to see whether the SOrT metric might pose a clinically significant benefit for treatment allocation.
Timeline (steps 1 and 2)
We describe the planned timeline for conductance of this registered report as well as timeline adherence in Additional file 5 .
Literature search
The literature search was conducted on 31 January 2019 and revealed 4567 reports in total. Following duplicate removal, screening, and full-text assessments, we included 38 studies in our qualitative synthesis [ 57 , 58 , 59 , 60 , 61 , 62 , 63 , 64 , 65 , 66 , 67 , 68 , 69 , 70 , 71 , 72 , 73 , 74 , 75 , 76 , 77 , 78 , 79 , 80 , 81 , 82 , 83 , 84 , 85 , 86 , 87 , 88 , 89 , 90 , 91 , 92 , 93 , 94 ]. For our quantitative synthesis on the sum-score level, this was reduced to 27 studies with information on the HAM-D and 19 studies with information on the BDI. After corresponding with original authors to obtain individual symptom-level data, we were able to use 9 and 4 studies with HAM-D and BDI information, respectively, for our symptom-level meta-analyses. Of note, studies that reported data on both HAM-D and BDI were included in sum-score and symptom-specific analyses of both scales. Figure 1 shows the PRISMA flow diagram, and Additional file 6 : Table S6 provides details of the included studies.
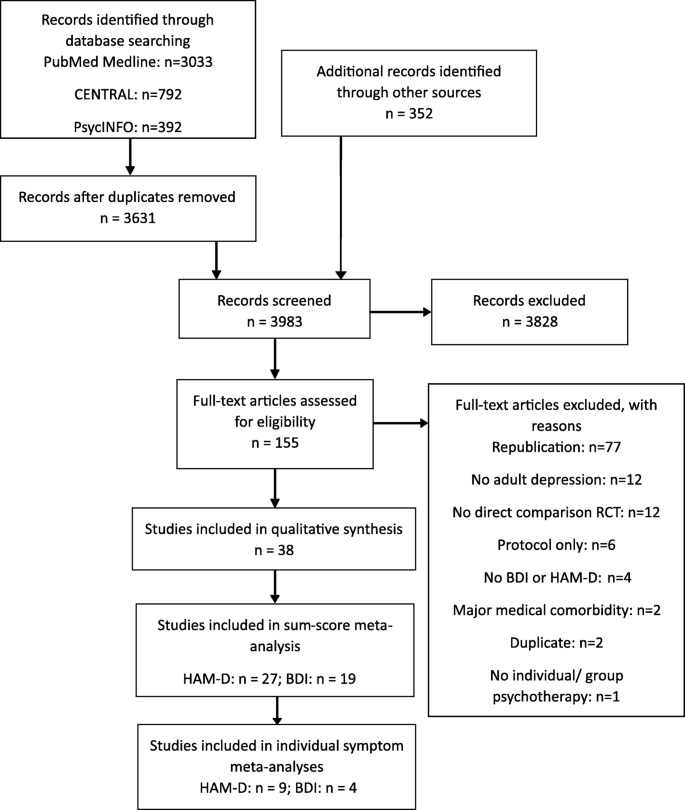
Adapted PRISMA flow diagram
Risk of bias
Results from Cochrane Risk of Bias tool ratings by NK and JKB are presented in Fig. 2 for individual risk of bias categories. Most studies have high overall risk of bias or reason for some concerns. Considering that the overall risk of bias takes forward ratings of individual bias categories, however, it is unsurprising that individual bias categories are more diverse. In particular, low risk of bias arises from deviations from intended interventions while selection of reported results and randomisation process ambiguities were more concerning.
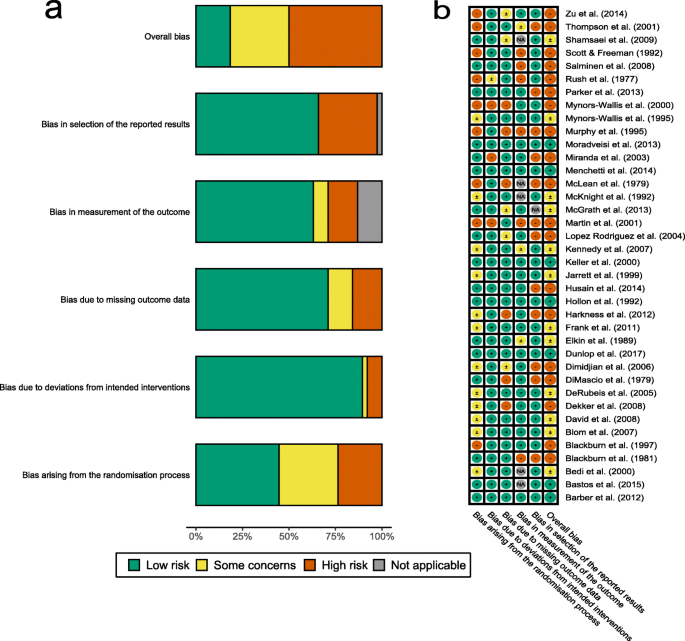
Risk of bias ratings a overall and b for specific studies
Sum-score meta-analyses
As previously done, we repeated sum-score meta-analyses for both HAM-D and BDI scales whenever outcome data were available in original studies. This allowed meta-analysis for 27 and 19 studies with 2433 and 1548 patients for HAM-D and BDI, respectively. Corresponding to completer only analyses on the individual symptom level, endpoint statistics for completers only were used in meta-analyses. We deviated from this procedure for 1 study, which only reported intention-to-treat statistics at endpoint [ 86 ]. Meta-analyses did not reveal significant differences in endpoint depressive symptom severity between psychotherapy and ADM for the HAM-D (SMD = 0.00, 95% CI − 0.09–0.10; Cochrane’s Q = 38.0, degrees of freedom [df] = 26, p = 0.060; I 2 = 18.4%; Fig. 3 ) and the BDI (SMD = − 0.05, 95% CI − 0.24–0.15; Cochrane’s Q = 61.1, df = 18, p < 0.001; I 2 = 69.2%; Fig. 4 ).
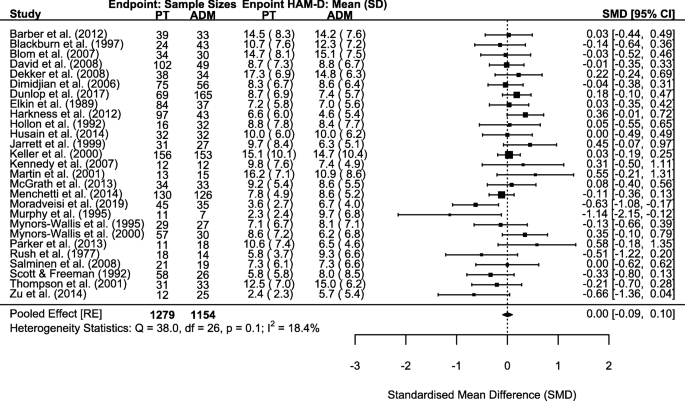
Forest plot of HAM-D sum-score meta-analysis
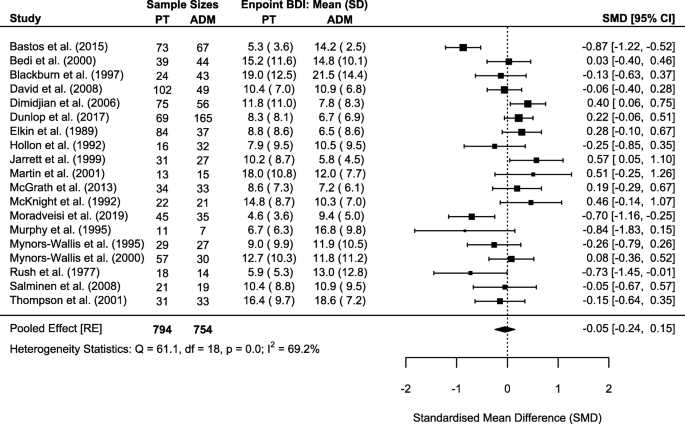
Forest plot of BDI sum-score meta-analysis
We assessed publication bias for HAM-D and BDI sum-score meta-analyses by visual funnel plot inspection (Additional file 7 : Fig. S1-S2) and Egger’s test; neither revealed indications of publication bias in favour of psychotherapy or ADM (HAM-D: z = − 0.81, p = 0.418; BDI: z = − 0.72, p = 0.474).
Beyond these pre-registered analyses, we further explored sum-score meta-analysis results by evaluating differential dropout between study arms. In particular, we performed meta-regression analyses including percentage of greater dropout in ADM compared to psychotherapy as moderator. These results revealed significant moderation of differential dropout for the HAM-D ( p = 0.018) but not for the BDI ( p = 0.124; Additional file 7 : Table S7). Importantly, RCTs with greater dropout in their ADM arm(s) were those with more favourable effects for psychotherapy and vice versa. Additional file 7 : Figs. S3-S4 visualise results for sum-score meta-analyses for HAM-D and BDI, respectively. We also explored differential dropout in a separate meta-analysis of 35 studies with information on baseline and endpoint sample sizes. Overall, 458 of 2163 (21.2%) and 557 of 2133 (26.1%) patients dropped out from psychotherapy and ADM arms, respectively. This difference was significant and indicated that psychotherapy was more acceptable to patients (OR = 0.74, 95% CI 0.59–0.92; Cochrane’s Q = 57.7, df = 34, p = 0.007; I 2 = 40.8%; see Additional file 7 : Fig. S5 for the forest plot).
Individual symptom meta-analyses
We conducted individual symptom meta-analyses for 9 and 4 studies providing individual symptom data for HAM-D and BDI, respectively. Importantly, the number of studies and, correspondingly, the number of patients included in meta-analyses varied across symptoms with ranges of 421–1166 (median = 1166) and 379–502 (median = 501) completer patients for HAM-D and BDI items, respectively. The reasons for differing sample sizes across symptoms varied for the following reasons: We had data from fewer studies assessing the BDI, questionnaire versions differed, and the lack of variance in a particular symptom of a particular study necessitated the removal of such a study from a meta-analysis. Regarding differing questionnaire versions, for instance, some studies used a HAM-D version with more than 17 items. For the BDI, the items differ in versions I and II. Aggregated pooled meta-analytic effect sizes can be seen in Fig. 5 . Additional file 8 : Tables S8-S9 include forest plots with sample sizes and heterogeneity statistics for each symptom of HAM-D and BDI, respectively. Additional file 8 : Fig. S6 shows effect size comparisons of HAM-D and BDI symptoms per symptom category (symptom categories as defined in Additional file 3 : Table S2). These comparisons indicated similar effect sizes between symptom categories of guilt, loss of energy, and suicidality while there was a strong divergence between symptom categories of work and interests and loss of libido.
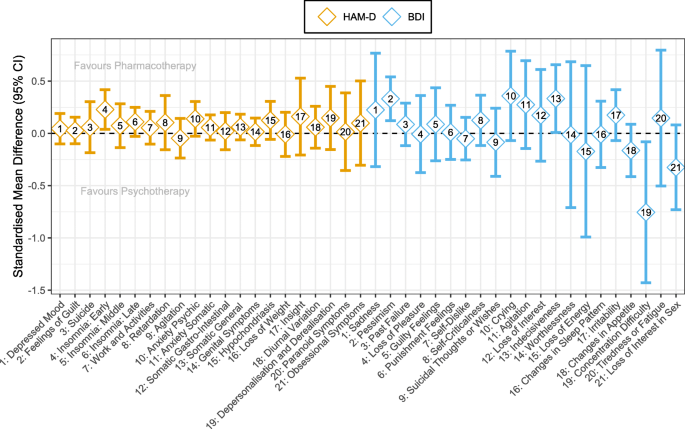
Pooled effect sizes (pORs converted to SMDs) on individual symptom level
As meta-analytic results indicate, few symptoms showed nominally significant differences between psychotherapy and ADM (favouring ADM: HAM-D item 4 [insomnia: early], BDI item 2 [pessimism] and BDI item 13 [indecisiveness]; favouring psychotherapy: BDI item 19 [concentration difficulty]). Importantly, after family-wise error correction (using the Benjamini-Hochberg method) [ 95 ], none of these differences remained significant (all p > 0.05).
Sensitivity analyses
To determine the influence of choice of effect size metric, we repeated individual symptom meta-analyses using “simple” ORs and SMDs in comparison to our metric of choice, the proportional OR (pOR). These sensitivity analyses provided a technical replication of our results indicating that choice of pOR as effect size metric was not driving our results (see Additional file 8 for details).
Exploratory comparison with Boschloo et al. [ 96 ]
Following pre-acceptance of this registered report, Boschloo et al. published a highly similar report to this study as RCTs comparing CBT (as one form of psychotherapy) versus ADM were meta-analysed on the individual symptom level [ 96 ]. The HAM-D was used as the sole outcome rating scale, but SMDs between treatments were calculated as differences in symptom scores from baseline to study endpoint.
As we deemed it important for the field, we performed an exploratory comparison of our results with theirs. Details of this exploratory comparison are provided in Additional file 8 . In sum, symptom-specific effect sizes from our meta-analyses were not associated with effect sizes from Boschloo et al. and only with small-to-moderate correlation when we restricted our meta-analysis to RCTs investigating CBT only.
We validated the SOrT metric using (i) a descriptive summary, (ii) assessment of clinical and demographic associations with our metric, and (iii) cross-validation between BDI- and HAM-D-based SOrT scores.
First, HAM-D and BDI SOrT metrics were approximately normally distributed in the sample (HAM-D: min = 0.20, median = 1.72, mean = 1.71, max = 3.18, SD = 0.55; BDI: min = − 1.98, median = 0.87, mean = 0.86, max = 3.72, SD = 1.04; Fig. 6 ). Contrary to our expectations, however, SOrT metric distributions were not centred around 0 and, in case of HAM-D SOrT scores, did not even include 0. A likely reason for the distributions not being centred around 0 is that most symptom-specific effect sizes were positive (i.e. favouring ADM) with 15/17 positive effect sizes for the HAM-D and 12/21 for the BDI. Second, we assessed clinical and sociodemographic correlates of respective SOrT scores. Importantly, we found high and small-to-moderate correlations between SOrT scores to their respective scale (HAM-D: Pearson’s r = 0.81, p < 0.001; BDI: Pearson’s r = 0.35, p < 0.001), which likely arises as an artefact of most effect sizes being positive. This also counters our initial goal of creating a treatment allocation metric (indicating psychotherapy versus ADM) as opposed to a mere measure of symptom severity. To highlight the distinction between a treatment allocation metric versus symptom severity associations, we report linear regression analyses, unadjusted and adjusted for baseline HAM-D and BDI sum-scores, of SOrT metric outcome on different sociodemographic and clinical predictor variables (Table 1 ). These analyses failed to reveal any consistent associations with our metric. Third, the small correlation of HAM-D and BDI SOrT scores (Pearson’s r = 0.12; cf. Fig. 6 ) was below the level considered meaningful for two treatment allocation metrics with identical goals (i.e. indicating favourable treatment by psychotherapy or ADM). In sum, our SOrT metric did not seem to be a valid measure with any clinical or sociodemographic correlates.
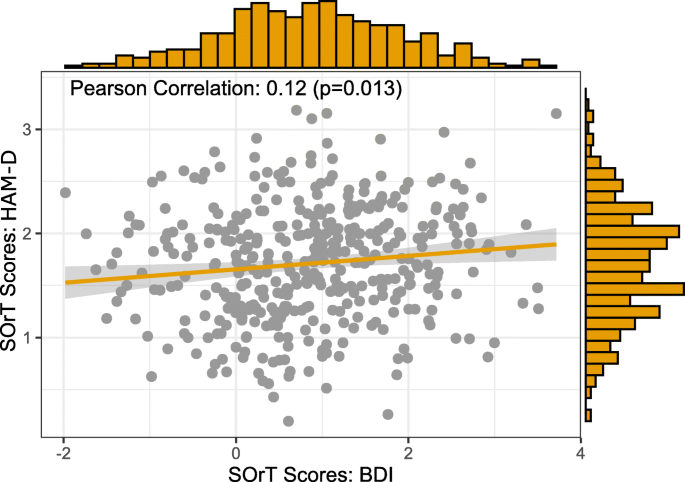
Association and distributions of HAM-D ( y -axis) and BDI ( x -axis) SOrT scores in the MARS sample
The SOrT metric was further evaluated in data from the PReDICT trial [ 44 , 57 ]. Again, SOrT scores were computed based on patients’ baseline symptom scores on HAM-D and BDI. Importantly, however, SOrT scores were based on effect sizes of individual symptom meta-analyses excluding PReDICT data to ascertain independence of our validation data (see comparison of effect sizes in Additional file 9 : Table S11). As with the MARS sample, distributions and correlations of HAM-D and BDI SOrT scores are visualised in Additional file 9 : Fig. S11. Contrary to our findings in MARS, we found a small, negative correlation between HAM-D and BDI SOrT scores (Pearson’s r = − 0.13, p = 0.054). Distributions were comparable for the HAM-D-based SOrT metric (min = 0.13, median = 1.15, mean = 1.15, max = 2.19, SD = 0.34) and more negative and variable for the BDI-based SOrT metric; this is consistent with a smaller number of trials included in BDI meta-analyses (min = − 5.27, median = − 0.98, mean = − 1.04, max = 2.09, SD = 1.39).
To compare optimal versus non-optimal SOrT-based treatment allocation, we initially pre-registered a sample split based on SOrT score valence (i.e. positive scores indicating optimal allocation to ADM and vice versa). As SOrT score distributions highlight, however, this approach was only possible for the BDI (23% of patients with positive SOrT scores) but not for the HAM-D (0% of patients with positive SOrT scores). Consequently, we decided to report an additional, exploratory classification of “optimal” versus “non-optimal” treatment allocation based on a SOrT score median split (i.e. patients above the median optimally treated by ADM and vice versa). Pre-registered and exploratory comparisons are reported in Table 2 . None of these analyses revealed any potential benefit of allocating patients to treatment based on their SOrT scores. We performed additional linear regression analyses of these comparisons, unadjusted and adjusted for the HAM-D at baseline, to delineate potential dependency of the SOrT metric on symptom severity (Additional file 9 : Tables S12-S14). These results align with pre-registered t tests.
Exploratory analyses
We conducted two further sets of exploratory analyses to compare our results with those by Boschloo et al. [ 96 ] and alterating computation of the SOrT metric to \( \mathrm{SOrT}=\frac{\sum_i{m}_i{s}_i}{\sum_i{s}_i} \) , so that there were no artifactual correlations with symptom severity (see Additional file 9 for details). Results suggested that symptom-based metrics from Boschloo et al. [ 96 ] and with altered SOrT score computation did not offer reliable advantages for allocation to psychotherapy versus ADM.
This registered report outlines a detailed investigation of the comparative effectiveness of psychotherapy and ADM for the treatment of individual depressive symptoms and whether symptom-specific effectiveness information can serve precision allocation. We did not find ADM or psychotherapy to be more effective than the respective other treatment at study endpoints in depressive symptom sum-scores on HAM-D and BDI scales. Similarly, there was no clear advantage of either treatment for individual depressive symptoms. Using individual symptom meta-analysis results, we evaluated the Symptom-Oriented Therapy (SOrT) metric, which combines meta-analytic effect size estimates with patients’ symptom profiles prior to treatment. Validation analyses in MARS and PReDICT studies did not indicate that the SOrT metric constituted a valid measure, nor that it should be used as a precision metric to indicate favourable allocation to psychotherapy versus ADM.
Our findings of comparable effectiveness of psychotherapy and ADM at the end of acute treatment closely align with older [ 40 , 41 , 42 ] and more recent [ 97 ] meta-analytic work. Cuijpers and colleagues only recently conducted an extensive network meta-analysis quantifying and ranking multiple different treatments for adult depression, including psychotherapy, ADM, and their combination [ 97 , 98 ]: Across multiple sets of sensitivity analyses (e.g. high versus low risk of bias, optimised psychotherapy/ADM, excluding placebo-controlled studies) and looking at groups of studies with moderate, severe, and chronic depression, psychotherapy and ADM consistently showed comparable effectiveness. Yet, psychotherapy was more acceptable (as defined in terms of lower dropout rates) compared to ADM. It is reassuring that our findings, albeit with smaller number of included studies, fully replicate this report. Our exploratory meta-regression of the HAM-D further demonstrated that effectiveness estimates of specific studies were moderated by differential dropout in study arms. Accordingly, if only ADM or psychotherapy is available to patients (rather than the combination, which is most effective [ 97 ]), we agree with clinical recommendations by Cuijpers and colleagues in that psychotherapy would be preferable to ADM based on its greater acceptability. This indication may be particularly relevant for patients who are likely not to complete a full course of treatment and prone to dropout (e.g. younger patients [ 99 ]).
Symptom-specific meta-analyses did not show significant differential treatment effects of psychotherapy or ADM for specific depressive symptoms following multiple comparison corrections. Although we identified nominally significant treatment differences for some symptoms, these do not align with prior research findings [ 36 , 38 , 96 ]. Hence, it remains unclear whether true symptom-specific treatment differences exist or whether reports from our study and previous literature reflect false positive findings (see Additional file 10 for further discussion).
The absence of clear meta-analytic symptom differences may also explain why our proposed SOrT metric did not seem to offer any benefit for treatment allocation. Assuming meta-analytic effect sizes were representative of noise rather than signal, this would mean SOrT scores were merely superimposing (meta-analytic) noise onto patients’ baseline symptom profiles. Our failure to find predictive utility of the precision sum-score from Boschloo and colleagues [ 96 ] and of a SOrT metric based on their reported effect sizes adds to this discouraging conclusion that symptom scores may not be of value in predicting psychotherapy versus ADM response.
It is important to emphasise, however, that the SOrT metric and other symptom-based allocation metrics (cf. [ 96 , 100 ]) are likely specific to the comparison being made, so are restricted to the comparison of psychotherapy versus ADM in this report. Khazanov and colleagues, for instance, recently showed that patients with greater distress and anhedonia prior to treatment responded more favourably to a combination of cognitive therapy and ADM rather than to ADM alone. This suggests individual symptom information may be valuable for indicating combination versus monotherapy in depression [ 100 ]. Similarly, there are reports that low-grade inflammation shows specificity for somatic symptoms of depression (e.g. sleep problems, low energy, or increased appetite) [ 101 , 102 , 103 , 104 ], so ongoing studies evaluating immunotherapy as a treatment for depression may benefit from symptom-based treatment allocation rules. We therefore encourage researchers to apply the SOrT metric to other treatment comparisons to evaluate its value as a precision medicine tool. It could be helpful, therefore, to adjust SOrT scores for symptom severity as we highlighted in our exploratory analyses (cf. Additional file 9 ). Moreover, future research could evaluate a combination of symptom-weighted approaches (such as the SOrT metric) with significance thresholds. Specifically, symptom-based metrics might benefit from only including meta-analytic weights (and corresponding symptoms) that pass a specific meta-analytic significance threshold for the comparison in question, thus optimising the signal-to-noise ratio. It is interesting to note that this approach would be similar to how polygenic risk scores are created (e.g. using PRSice software [ 105 ]), which weight effect alleles by effect sizes, if a specific significance threshold has been reached for this allele. In sum, we hope symptom-based precision medicine tools receive further attention in future research despite the limited value for decision-making on allocation to psychotherapy versus ADM in depression.
Limitations
The present investigation has five major limitations. First, we were not able to include all studies identified from the literature in meta-analyses. This low coverage was due to varied reasons such as general data unavailability [ 59 , 62 , 63 , 86 , 89 , 90 ], insufficient reporting of sample sizes and summary statistics for inclusion in sum-score meta-analysis [ 68 , 75 , 85 , 94 ], and/or failure of authors to respond to inquiries (cf. Additional file 11 : Table S19). Based on this, we encourage more thorough reporting of summary statistics in original trials (ideally including completer and intention-to-treat samples) and also advocate publication of summary statistics in meta-analyses rather than effect sizes alone; this would enhance reproducibility. Despite limited data availability, it is reassuring that conclusions from sum-score meta-analyses match the most recent meta-analysis with the largest power [ 97 ]. Sample sizes for individual symptom meta-analyses were also relatively large and exceeded those of similar work by Boschloo et al. [ 96 ], which can be attributed to our broader focus on RCTs comparing ADM with psychotherapy (rather than to CBT only).
Second, we cannot conclude anything regarding symptom-specific differences between intervention comparisons other than psychotherapy versus ADM (as discussed before), and it is unclear if conclusions outside this study’s inclusion criteria would be meaningful. Our inclusion criteria, for instance, focussed on acute treatment of adult depression, so it is possible that symptom-specific differences following treatment with ADM versus psychotherapy exist in children, adolescents, or older patients. Similarly, symptom-specific differences may arise at follow-up in the form of residual symptoms (also not addressed in this report), which was recently shown in a RCT re-analysis [ 38 ].
Third, our analyses focussed on study completer data, though an intention-to-treat approach would be clinically more interesting. The data format used for present analyses did not allow for the identification of individual participants, which facilitated data sharing from original RCTs. Due to non-identifiability of individuals, however, we were not able to use imputation techniques for intention-to-treat analyses on the symptom level. Consequently, we could not verify whether our findings would have been different if all randomised participants had been included, rendering this an open question for future research.
Fourth, inferences from symptom-specific analyses are limited by the low reliability of individual symptoms. Depression measures, such as the HAM-D and BDI used in the present report, are not designed for reliable symptom-specific assessments [ 32 , 33 ]. Thus, our failure to find evidence for symptom-specific treatment effects and/or differences between present findings and Boschloo et al. [ 96 ] may be a consequence of low reliability of symptom assessments. Future research should ideally combine evidence from multiple indicators per assessed symptom to increase reliability.
Lastly, validation of the SOrT metric was only performed in data from the PReDICT study. Because the PReDICT study enrolled only treatment-naïve patients [ 44 , 57 ], it may not have provided the optimal sample for validating the SOrT metric, which was derived from more mixed samples. Future research should thus evaluate the utility of symptom-based metrics in other samples, which would require individual patient data. Ideally, cross-validation procedures could be used, which would average over unique characteristics of multiple validation samples.
In conclusion, we report the largest symptom-specific meta-analysis of direct comparisons of psychotherapy and ADM for depression. We did not find robust indications for symptom-specific effectiveness differences between treatments and this also extended to the sum-score level. We introduced the Symptom-Oriented Therapy (SOrT) metric as a precision treatment allocation tool, but failed to demonstrate its usefulness for improved allocation to psychotherapy or ADM. Though future symptom-specific work looking at other treatment comparisons could be valuable, our findings suggest that symptom information does not inform on whether any individual patient should receive psychotherapy or ADM.
Availability of data and materials
For maximum transparency and reproducibility, we attempt to provide as much data and materials as possible. In Additional file 11 : Table S19, we outline to what capacity we can share data from original RCTs identified in the systematic review. In Additional file 11 : Table S20, we provide a list of additional data and analysis scripts as available on the open science framework (OSF) platform under https://osf.io/qzjc9/ . We are not able to publicly share original data from the MARS study. Original data from PReDICT, however, is available as required by NIH data sharing policy and can be requested by contacting original authors.
Abbreviations
- Antidepressant medication
Beck Depression Inventory
Cognitive behavioural therapy
Cochrane Central Register of Controlled Trials
Confidence interval
Hamilton Anxiety Rating Scale
Hamilton Rating Scale for Depression
Individual patient data
Munich Antidepressant Response Signature
Major Depressive Disorder
Personalised Advantage Index
Emory Predictors of Remission in Depression to Individual and Combined Treatments
(Proportional) Odds ratio
Randomised controlled trial
Standard deviation
Standard error
Standardised mean difference
Symptom-Oriented Therapy
Otte C, Gold SM, Penninx BWJH, Pariante CM, Etkin A, Fava M, et al. Major depressive disorder. Nat Rev Dis Prim. 2016;2:16065. https://doi.org/10.1038/nrdp.2016.65 .
Article PubMed Google Scholar
McClintock SM, Husain MM, Wisniewski SR, Nierenberg AA, Stewart JW, Trivedi MH, et al. Residual symptoms in depressed outpatients who respond by 50% but do not remit to depressant medication. J Clin Psychopharmacol. 2011;31:180–6. https://doi.org/10.1097/JCP.0b013e31820ebd2c .
Article PubMed PubMed Central Google Scholar
Treadway MT, Waskom ML, Dillon DG, Holmes AJ, Park MTM, Chakravarty MM, et al. Illness progression, recent stress and morphometry of hippocampal subfields and medial prefrontal cortex in major depression. Biol Psychiatry. 2015;77:285–94. https://doi.org/10.1016/j.biopsych.2014.06.018 .
Chang CH, Chen MC, Hong Qiu M, Lu J. Ventromedial prefrontal cortex regulates depressive-like behavior and rapid eye movement sleep in the rat. Neuropharmacology. 2014;86:125–32. https://doi.org/10.1016/j.neuropharm.2014.07.005 .
Article CAS PubMed PubMed Central Google Scholar
Lekman M, Laje G, Charney D, Rush AJ, Wilson AF, Sorant AJM, et al. The FKBP5-gene in depression and treatment response—an association study in the sequenced treatment alternatives to relieve depression (STAR*D) cohort. Biol Psychiatry. 2008;63:1103–10. https://doi.org/10.1016/j.biopsych.2007.10.026 .
Bromet E, Andrade LH, Hwang I, Sampson NA, Alonso J, de Girolamo G, et al. Cross-national epidemiology of DSM-IV major depressive episode. BMC Med. 2011;9:90. https://doi.org/10.1186/1741-7015-9-90 .
Kessler RC, Bromet EJ. The epidemiology of depression across cultures. Annu Rev Public Health. 2013;34:119–38. https://doi.org/10.1146/annurev-publhealth-031912-114409 .
Global Burden of Disease Study 2013 Collaborators. Global, regional, and national incidence, prevalence, and years lived with disability for 301 acute and chronic diseases and injuries in 188 countries, 1990–2013: a systematic analysis for the Global Burden of Disease Study 2013. Lancet. 2015;386:743–800. https://doi.org/10.1016/S0140-6736(15)60692-4 .
Article PubMed Central Google Scholar
Köhler O, Benros ME, Nordentoft M, Farkouh ME, Iyengar RL, Mors O, et al. Effect of anti-inflammatory treatment on depression, depressive symptoms, and adverse effects: a systematic review and meta-analysis of randomized clinical trials. JAMA Psychiatry. 2014;71:1381–91. https://doi.org/10.1001/jamapsychiatry.2014.1611 .
Kappelmann N, Lewis G, Dantzer R, Jones PB, Khandaker GM. Antidepressant activity of anti-cytokine treatment: a systematic review and meta-analysis of clinical trials of chronic inflammatory conditions. Mol Psychiatry. 2018;23:335–43. https://doi.org/10.1038/mp.2016.167 .
Article CAS PubMed Google Scholar
Singh JB, Fedgchin M, Daly E, Xi L, Melman C, De Bruecker G, et al. Intravenous esketamine in adult treatment-resistant depression: a double-blind, double-randomization. Placebo-Controlled Study Biol Psychiatry. 2016;80:424–31. https://doi.org/10.1016/j.biopsych.2015.10.018 .
Cipriani A, Furukawa TA, Salanti G, Chaimani A, Atkinson LZ, Ogawa Y, et al. Comparative efficacy and acceptability of 21 antidepressant drugs for the acute treatment of adults with major depressive disorder: a systematic review and network meta-analysis. Lancet. 2018. https://doi.org/10.1016/S0140-6736(17)32802-7 .
Barth J, Munder T, Gerger H, Nüesch E, Trelle S, Znoj H, et al. Comparative efficacy of seven psychotherapeutic interventions for patients with depression: a network meta-analysis. PLoS Med. 2013;10:e1001454. https://doi.org/10.1371/journal.pmed.1001454 .
Cuijpers P, Cristea IA, Karyotaki E, Reijnders M, Huibers MJH. How effective are cognitive behavior therapies for major depression and anxiety disorders? A meta-analytic update of the evidence. World Psychiatry. 2016;15:245–58. https://doi.org/10.1002/wps.20346 .
Adli M, Wiethoff K, Baghai TC, Fisher R, Seemüller F, Laakmann G, et al. How effective is algorithm-guided treatment for depressed inpatients? Results from the randomized controlled multicenter German algorithm project 3 trial. Int J Neuropsychopharmacol. 2017;20:721–30. https://doi.org/10.1093/ijnp/pyx043 .
Gaynes B, Warden D, Trivedi M, Wisniewski S, Fava M, Rush AJ. What did STAR*D teach us? Results from a large-scale, practical, clinical trial for patients with depression. Psychiatr Serv. 2009;60:1439–45. https://doi.org/10.1176/appi.ps.60.11.1439 .
Rush AJ, Trivedi MH, Wisniewski SR, Nierenberg AA, Stewart JW, Warden D, et al. Acute and longer-term outcomes in depressed outpatients requiring one or several treatment steps: a STAR*D report. Am J Psychiatry. 2006;163:1905–17. https://doi.org/10.1176/appi.ajp.163.11.1905 .
Paul GL. Strategy of outcome research in psychotherapy. J Consult Psychol. 1967;31:109–18. https://doi.org/10.1037/h0024436 .
Iniesta R, Stahl D, McGuffin P. Machine learning, statistical learning and the future of biological research in psychiatry. Psychol Med. 2016;46:2455–65. https://doi.org/10.1017/S0033291716001367 .
Kessler RC, van Loo HM, Wardenaar KJ, Bossarte RM, Brenner LA, Cai T, et al. Testing a machine-learning algorithm to predict the persistence and severity of major depressive disorder from baseline self-reports. Mol Psychiatry. 2016;21:1366–71. https://doi.org/10.1038/mp.2015.198 .
Cuijpers P. Personalized treatment for functional outcome in depression. Medicographia. 2014;36:476–81.
Google Scholar
Dunlop BW, Rajendra JK, Craighead WE, Kelley ME, McGrath CL, Choi KS, et al. Functional connectivity of the subcallosal cingulate cortex and differential outcomes to treatment with cognitive-behavioral therapy or antidepressant medication for major depressive disorder. Am J Psychiatry. 2017;174:533–45. https://doi.org/10.1176/appi.ajp.2016.16050518 .
Niles AN, Loerinc AG, Krull JL, Roy-Byrne P, Sullivan G, Sherbourne CD, et al. Advancing personalized medicine: application of a novel statistical method to identify treatment moderators in the coordinated anxiety learning and management study. Behav Ther. 2017;48:490–500. https://doi.org/10.1016/j.beth.2017.02.001 .
Kraemer HC. Discovering, comparing, and combining moderators of treatment on outcome after randomized clinical trials: a parametric approach. Stat Med. 2013;32:1964–73. https://doi.org/10.1002/sim.5734 .
DeRubeis RJ, Cohen ZD, Forand NR, Fournier JC, Gelfand LA, Lorenzo-Luaces L. The personalized advantage index: translating research on prediction into individualized treatment recommendations. A Demonstration PLoS One. 2014;9:e83875. https://doi.org/10.1371/journal.pone.0083875 .
Webb CA, Trivedi MH, Cohen ZD, Dillon DG, Fournier JC, Goer F, et al. Personalized prediction of antidepressant v. placebo response: evidence from the EMBARC study. Psychol Med. 2018;:1–10. doi: https://doi.org/10.1017/S0033291718001708 .
Huibers MJH, Cohen ZD, Lemmens LHJM, Arntz A, Peeters FPML, Cuijpers P, et al. Predicting optimal outcomes in cognitive therapy or interpersonal psychotherapy for depressed individuals using the personalized advantage index approach. PLoS One. 2015;10:e0140771. https://doi.org/10.1371/journal.pone.0140771 .
Cohen ZD, Kim TT, Van HL, Dekker JJM, Driessen E. A demonstration of a multi-method variable selection approach for treatment selection: recommending cognitive–behavioral versus psychodynamic therapy for mild to moderate adult depression. Psychother Res. 2020;30:137–50. https://doi.org/10.1080/10503307.2018.1563312 .
Vittengl JR, Anna Clark L, Thase ME, Jarrett RB. Initial steps to inform selection of continuation cognitive therapy or fluoxetine for higher risk responders to cognitive therapy for recurrent major depressive disorder. Psychiatry Res. September 2016;2017(253):174–81.
Weitz E, Kleiboer A, van Straten A, Hollon SD, Cuijpers P. Individual patient data meta-analysis of combined treatments versus psychotherapy (with or without pill placebo), pharmacotherapy or pill placebo for adult depression: a protocol. BMJ Open. 2017;7:e013478. https://doi.org/10.1136/bmjopen-2016-013478 .
Fried EI, Nesse RM. The impact of individual depressive symptoms on impairment of psychosocial functioning. PLoS One. 2014;9.
Fried EI, Nesse RM. Depression sum-scores don’t add up: why analyzing specific depression symptoms is essential. BMC Med. 2015;13:72. https://doi.org/10.1186/s12916-015-0325-4 .
Fried EI. The 52 symptoms of major depression: lack of content overlap among seven common depression scales. J Affect Disord. 2017;208:191–7. https://doi.org/10.1016/j.jad.2016.10.019 .
American Psychiatric Association. Diagnostic and statistical manual of mental disorders (DSM-5®). Washington, DC: American Psychiatric Pub; 2013.
Book Google Scholar
Fried EI, Nesse RM. Depression is not a consistent syndrome: an investigation of unique symptom patterns in the STAR*D study. J Affect Disord. 2015;172:96–102.
Fournier JC, DeRubeis RJ, Hollon SD, Gallop R, Shelton RC, Amsterdam JD. Differential change in specific depressive symptoms during antidepressant medication or cognitive therapy. Behav Res Ther. 2013;51:392–8. https://doi.org/10.1016/j.brat.2013.03.010 .
Fried EI, van Borkulo CD, Epskamp S, Schoevers RA, Tuerlinckx F, Borsboom D. Measuring depression over time... Or not? Lack of unidimensionality and longitudinal measurement invariance in four common rating scales of depression. Psychol Assess. 2016;28:1354–67.
Dunlop BW, Polychroniou PE, Rakofsky JJ, Nemeroff CB, Craighead WE, Mayberg HS. Suicidal ideation and other persisting symptoms after CBT or antidepressant medication treatment for major depressive disorder. Psychol Med. 2018;:1–10. doi: https://doi.org/10.1017/S0033291718002568 .
Hieronymus F, Emilsson JF, Nilsson S, Eriksson E. Consistent superiority of selective serotonin reuptake inhibitors over placebo in reducing depressed mood in patients with major depression. Mol Psychiatry. 2015;21:523–30. https://doi.org/10.1038/mp.2015.53 .
Thoma NC, McKay D, Gerber AJ, Milrod BL, Edwards AR, Kocsis JH. A quality-based review of randomized controlled trials of cognitive-behavioral therapy for depression: an assessment and metaregression. Am J Psychiatry. 2012;169:22–30. https://doi.org/10.1176/appi.ajp.2011.11030433 .
Huhn M, Tardy M, Maria Spineli L, Kissling W, Förstl H, Pitschel-Walz G, et al. Efficacy of pharmacotherapy and psychotherapy for adult psychiatric disorders a systematic overview of meta-analyses. JAMA Psychiatry. 2014;71:706–15. https://doi.org/10.1001/jamapsychiatry.2014.112 .
Cuijpers P, Sijbrandij M, Koole SL, Andersson G, Beekman AT, Reynolds CF. The efficacy of psychotherapy and pharmacotherapy in treating depressive and anxiety disorders: a meta-analysis of direct comparisons. World Psychiatry. 2013;12:137–48. https://doi.org/10.1002/wps.20038 .
Hennings JM, Owashi T, Binder EB, Horstmann S, Menke A, Kloiber S, et al. Clinical characteristics and treatment outcome in a representative sample of depressed inpatients – findings from the Munich Antidepressant Response Signature (MARS) project. J Psychiatr Res. 2009;43:215–29. https://doi.org/10.1016/j.jpsychires.2008.05.002 .
Dunlop BW, Binder EB, Cubells JF, Goodman MM, Kelley ME, Kinkead B, et al. Predictors of remission in depression to individual and combined treatments (PReDICT): study protocol for a randomized controlled trial. Trials. 2012;13:1–18. https://doi.org/10.1186/1745-6215-13-106 .
Article CAS Google Scholar
Booth A, Clarke M, Dooley G, Ghersi D, Moher D, Petticrew M, et al. The nuts and bolts of PROSPERO: an international prospective register of systematic reviews. Syst Rev. 2012;1:1–8. https://doi.org/10.1186/2046-4053-1-2 .
Article Google Scholar
Cuijpers P, van Straten A, Warmerdam L, Andersson G. Psychological treatment of depression: a meta-analytic database of randomized studies. BMC Psychiatry. 2008;8:36. https://doi.org/10.1186/1471-244X-8-36 .
Higgins JPT, Altman DG, Gøtzsche PC, Jüni P, Moher D, Oxman AD, et al. The Cochrane Collaboration’s tool for assessing risk of bias in randomised trials. BMJ. 2011;343:d5928. https://doi.org/10.1136/bmj.d5928 .
Munder T, Barth J. Cochrane’s risk of bias tool in the context of psychotherapy outcome research. Psychother Res. 2017:1–9. doi: https://doi.org/10.1080/10503307.2017.1411628 .
Higgins JPT, Savović J, Page MJ, Sterne JAC. A revised tool to assess risk of bias in randomized trials (RoB 2.0). 2016. https://sites.google.com/site/riskofbiastool//welcome/rob-2-0-tool . Accessed 28 Feb 2018.
Jelicic Kadic A, Vucic K, Dosenovic S, Sapunar D, Puljak L. Extracting data from figures with software was faster, with higher interrater reliability than manual extraction. J Clin Epidemiol. 2016;74:119–23. https://doi.org/10.1016/j.jclinepi.2016.01.002 .
Brant R. Assessing proportionality in the proportional odds model for ordinal logistic regression. Biometrics. 1990;46:1171–8. https://doi.org/10.2307/2532457 .
Agresti A, Kateri M. Categorical data analysis. In: Lovric M, editor. International Encyclopedia of Statistical Science. Berlin: Springer Berlin Heidelberg; 2011. p. 206–8. https://doi.org/10.1007/978-3-642-04898-2 .
Chapter Google Scholar
Chinn S. A simple method for converting an odds ratio to effect size for use in meta-analysis. Stat Med. 2000;19:3127–31.
Viechtbauer W. Conducting meta-analyses in R with the metafor package. J Stat Softw. 2010;36:1–48.
R Core Team. R: a language and environment for statistical computing. 2017. https://www.r-project.org/ . Accessed 19 Sept 2017.
Egger M, Smith GD, Schneider M, Minder C. Bias in meta-analysis detected by a simple, graphical test. BMJ. 1997;315:629–34.
Dunlop BW, Kelley ME, Aponte-Rivera V, Mletzko-Crowe T, Kinkead B, Ritchie JC, et al. Effects of patient preferences on outcomes in the predictors of remission in depression to individual and combined treatments (PReDICT) study. Am J Psychiatry. 2017;174:546–56. https://doi.org/10.1176/appi.ajp.2016.16050517 .
Barber JP, Barrett MS, Gallop R, Rynn MA, Rickels K. Short-term dynamic psychotherapy versus pharmacotherapy for major depressive disorder. J Clin Psychiatry. 2012;73:66–73. https://doi.org/10.4088/JCP.11m06831 .
DiMascio A, Weissman MM, Prusoff BA, Neu C, Zwilling M, Klerman GL. Differential symptom reduction by drugs and psychotherapy in acute depression. Arch Gen Psychiatry. 1979;36:1450–6. https://doi.org/10.1001/archpsyc.1979.01780130068008 .
Dimidjian S, Hollon SD, Dobson KS, Schmaling KB, Kohlenberg RJ, Addis ME, et al. Randomized trial of behavioral activation, cognitive therapy, and antidepressant medication in the acute treatment of adults with major depression. J Consult Clin Psychol. 2006;74:658–70.
Elkin I, Shea T, Watkins JT, Imber SD, Sotsky SM, Collins JF, et al. National Institute of Mental Health treatment of depression collaborative research program. Arch Gen Psychiatry. 1989;46:971–82. https://doi.org/10.1001/archpsyc.1989.01810110013002 .
Frank E, Cassano GB, Rucci P, Thompson WK, Kraemer HC, Fagiolini A, et al. Predictors and moderators of time to remission of major depression with interpersonal psychotherapy and SSRI pharmacotherapy. Psychol Med. 2011;41:151–62. https://doi.org/10.1017/S0033291710000553 .
Harkness KL, Bagby RM, Kennedy SH. Childhood maltreatment and differential treatment response and recurrence in adult major depressive disorder. J Consult Clin Psychol. 2012;80:342–53. https://doi.org/10.1037/a0027665 .
Husain N, Chaudhry N, Fatima B, Husain M, Amin R, Chaudhry IB, et al. Antidepressant and group psychosocial treatment for depression: a rater blind exploratory RCT from a low income country. Behav Cogn Psychother. 2014;42:693–705. https://doi.org/10.1017/S1352465813000441 .
Jarrett RB, Schaffer M, McIntire D, Witt-Browder A, Kraft D, Risser RC. Treatment of atypical depression with cognitive therapy or phenelzine. Arch Gen Psychiatry. 1999;56:431. https://doi.org/10.1001/archpsyc.56.5.431 .
Keller MB, McCullough JP, Klein DN, Arnow B, Dunner DL, Gelenberg AJ, et al. A comparison of nefazodone, the cognitive behavioral-analysis system of psychotherapy, and their combination for the treatment of chronic depression. N Engl J Med. 2000;342:1462–70. https://doi.org/10.1056/NEJM200005183422001 .
Kennedy SH, Konarski JZ, Segal ZV, Lau MA, Bieling PJ, McIntyre RS, et al. Differences in brain glucose metabolism between responders to CBT and venlafaxine in a 16-week randomized controlled trial. Am J Psychiatry. 2007;164:778–88. https://doi.org/10.1176/ajp.2007.164.5.778 .
López Rodríguez J, López Butrón MA, Vargas Terrez BE, Villamil SV. Estudio doble ciego con antidepresivo, psicoterapia breve y placebo en pacientes con depresión leve a moderada. Salud Ment. 2004;27:53–61.
Bastos AG, Guimaraes LSP, Trentini CM. The efficacy of long-term psychodynamic psychotherapy, fluoxetine and their combination in the outpatient treatment of depression. Psychother Res. 2015;25:612–24. https://doi.org/10.1080/10503307.2014.935519 .
Martin SD, Martin E, Rai SS, Richardson MA, Royall R. Brain blood flow changes in depressed patients treated with interpersonal psychotherapy or venlafaxine hydrochloride. Arch Gen Psychiatry. 2001;58:641. https://doi.org/10.1001/archpsyc.58.7.641 .
McGrath CL, Kelley ME, Holtzheimer PE, Dunlop BW, Craighead WE, Franco AR, et al. Toward a neuroimaging treatment selection biomarker for major depressive disorder. JAMA Psychiatry. 2013;70:821–9. https://doi.org/10.1001/jamapsychiatry.2013.143 .
McKnight DL, Nelson-Gray RO, Barnhill J. Dexamethasone suppression test and response to cognitive therapy and antidepressant medication. Behav Ther. 1992;23:99–111. https://doi.org/10.1016/S0005-7894(05)80311-9 .
McLean PD, Hakstian AR. Clinical depression: comparative efficacy of outpatient treatments. J Consult Clin Psychol. 1979;47:818–36. https://doi.org/10.1037/0022-006X.47.5.818 .
Menchetti M, Rucci P, Bortolotti B, Bombi A, Scocco P, Kraemer HC, et al. Moderators of remission with interpersonal counselling or drug treatment in primary care patients with depression: randomised controlled trial. Br J Psychiatry. 2014;204:144–50. https://doi.org/10.1192/bjp.bp.112.122663 .
Miranda J, Chung JY, Green BL, Krupnick J, Siddique J, Revicki DA, et al. Treating depression in predominantly low-income young minority women. JAMA. 2003;290:57–65. https://doi.org/10.1001/jama.290.1.57 .
Moradveisi L, Huibers MJH, Renner F, Arasteh M, Arntz A. Behavioural activation v. antidepressant medication for treating depression in Iran: randomised trial. Br J Psychiatry. 2013;202:204–11. https://doi.org/10.1192/bjp.bp.112.113696 .
Murphy GE, Carney RM, Knesevich MA, Wetzel RD, Whitworth P. Cognitive behavior therapy, relaxation training, and tricyclic antidepressant medication in the treatment of depression. Psychol Rep. 1995;77:403–20. https://doi.org/10.2466/pr0.1995.77.2.403 .
Mynors-Wallis LM, Gath DH, Lloyd-Thomas AR, Tomlinson D. Randomised controlled trial comparing problem solving treatment with amitriptyline and placebo for major depression in primary care. BMJ. 1995;310:441–5. https://doi.org/10.1136/bmj.310.6977.441 .
Mynors-Wallis LM, Gath DH, Day A, Baker F. Randomised controlled trial of problem solving treatment, antidepressant medication, and combined treatment for major depression in primary care. BMJ. 2000;320:26–30. https://doi.org/10.1136/bmj.320.7226.26 .
Bedi N, Chilvers C, Churchill R, Dewey M, Duggan C, Fielding K, et al. Assessing effectiveness of treatment of depression in primary care. Partially randomised preference trial. Br J Psychiatry. 2000;177 d:312–8. internal-pdf://189.2.208.174/Bedi-2000-Assessing effectiveness of treatment.pdf LB - 01/8/30%5Cnhttp://bjp.rcpsych.org/content/bjprcpsych/177/4/312.full.pdf.
Parker G, Blanch B, Paterson A, Hadzi-Pavlovic D, Sheppard E, Manicavasagar V, et al. The superiority of antidepressant medication to cognitive behavior therapy in melancholic depressed patients: a 12-week single-blind randomized study. Acta Psychiatr Scand. 2013;128:271–81. https://doi.org/10.1111/acps.12049 .
Rush AJ, Beck AT, Kovacs M, Hollon S. Comparative efficacy of cognitive therapy and pharmacotherapy in the treatment of depressed outpatients. Cognit Ther Res. 1977;1:17–37. https://doi.org/10.1007/BF01173502 .
Salminen JK, Karlsson H, Hietala J, Kajander J, Aalto S, Markkula J, et al. Short-term psychodynamic psychotherapy and fluoxetine in major depressive disorder: a randomized comparative study. Psychother Psychosom. 2008;77:351–7. https://doi.org/10.1159/000151388 .
Scott AI, Freeman CP. Edinburgh primary care depression study: treatment outcome, patient satisfaction, and cost after 16 weeks. BMJ. 1992;304:883–7. https://doi.org/10.1136/bmj.304.6831.883 .
Shamsaei F, Rahimi A, Zarabian M, Sedehi M. Efficacy of pharmacotherapy and cognitive therapy, alone and in combination in major depressive disorder. Hong Kong J Psychiatry. 2008;18:76–80.
Thompson LW, Coon DW, Gallagher-Thompson D, Sommer BR, Koin D. Comparison of desipramine and cognitive/behavioral therapy in the treatment of elderly outpatients with mild-to-moderate depression. Am J Geriatr Psychiatry. 2001;9:225–40. https://doi.org/10.1097/00019442-200108000-00006 .
Zu S, Xiang Y-T, Liu J, Zhang L, Wang G, Ma X, et al. A comparison of cognitive-behavioral therapy, antidepressants, their combination and standard treatment for Chinese patients with moderate–severe major depressive disorders. J Affect Disord. 2014;152–154:262–7. https://doi.org/10.1016/j.jad.2013.09.022 .
Hollon SD, DeRubeis RJ, Evans MD, Wiemer MJ, Garvey MJ, Grove WM, et al. Cognitive therapy and pharmacotherapy for depression. Arch Gen Psychiatry. 1992;49:774. https://doi.org/10.1001/archpsyc.1992.01820100018004 .
Blackburn IM, Bishop S, Glen AI, Whalley LJ, Christie JE. The efficacy of cognitive therapy in depression: a treatment trial using cognitive therapy and pharmacotherapy, each alone and in combination. Br J Psychiatry. 1981;139:181–9 http://www.ncbi.nlm.nih.gov/pubmed/3140 .
Blackburn I-M, Moore RG. Controlled acute and follow-up trial of cognitive therapy and pharmacotherapy in out-patients with recurrent depression. Br J Psychiatry. 1997;171:328–34. https://doi.org/10.1192/bjp.171.4.328 .
Blom MBJ, Jonker K, Dusseldorp E, Spinhoven P, Hoencamp E, Haffmans J, et al. Combination treatment for acute depression is superior only when psychotherapy is added to medication. Psychother Psychosom. 2007;76:289–97. https://doi.org/10.1159/000104705 .
David D, Szentagotai A, Lupu V, Cosman D. Rational emotive behavior therapy, cognitive therapy, and medication in the treatment of major depressive disorder: a randomized clinical trial, posttreatment outcomes, and six-month follow-up. J Clin Psychol. 2008;64:728–46. https://doi.org/10.1002/jclp.20487 .
Dekker JJM, Koelen JA, Van HL, Schoevers RA, Peen J, Hendriksen M, et al. Speed of action: the relative efficacy of short psychodynamic supportive psychotherapy and pharmacotherapy in the first 8 weeks of a treatment algorithm for depression. J Affect Disord. 2008;109:183–8. https://doi.org/10.1016/j.jad.2007.10.015 .
DeRubeis RJ, Hollon SD, Amsterdam JD, Shelton RC, Young PR, Salomon RM, et al. Cognitive therapy vs medications in the treatment of moderate to severe depression. Arch Gen Psychiatry. 2005;62:409. https://doi.org/10.1001/archpsyc.62.4.409 .
Benjamini Y, Hochberg Y. Controlling the false discovery rate: a practical and powerful approach to multiple testing. J R Stat Soc Ser B (Statistical Methodol). 1995;57:289–300.
Boschloo L, Bekhuis E, Erica S, Reijnders M, Derubeis RJ, Dimidjian S, et al. The symptom-specific efficacy of antidepressant medication vs. cognitive behavioral therapy in the treatment of depression : results from an individual patient data meta-analysis. World Psychiatry. 2019;18:183–91.
Cuijpers P, Noma H, Karyotaki E, Vinkers CH, Cipriani A, Furukawa TA. A network meta-analysis of the effects of psychotherapies, pharmacotherapies and their combination in the treatment of adult depression. World Psychiatry. 2020;19:92–107. https://doi.org/10.1002/wps.20701 .
Craighead WE, Dunlop BW. Combination psychotherapy and antidepressant medication treatment for depression: for whom, when, and how. Annu Rev Psychol. 2014;65:267–300. https://doi.org/10.1146/annurev.psych.121208.131653 .
Zilcha-Mano S, Keefe JR, Chui H, Rubin A, Barrett MS, Barber JP. Reducing dropout in treatment for depression. J Clin Psychiatry. 2016;77:e1584–90. https://doi.org/10.4088/JCP.15m10081 .
Khazanov GK, Xu C, Dunn BD, Cohen ZD, DeRubeis RJ, Hollon SD. Distress and anhedonia as predictors of depression treatment outcome: a secondary analysis of a randomized clinical trial. Behav Res Ther. 2020;125:103507. https://doi.org/10.1016/j.brat.2019.103507 .
Fried EI, von Stockert S, Haslbeck JMB, Lamers F, Schoevers RA, Penninx BWJH. Using network analysis to examine links between individual depressive symptoms, inflammatory markers, and covariates. Psychol Med. 2019;:1–9. doi: https://doi.org/10.1017/S0033291719002770 .
Jokela M, Virtanen M, Batty G, Kivimäki M. Inflammation and specific symptoms of depression. JAMA Psychiatry. 2016;73:87–8. https://doi.org/10.1001/jamapsychiatry.2015.1977 .
Chu AL, Stochl J, Lewis G, Zammit S, Jones PB, Khandaker GM. Longitudinal association between inflammatory markers and specific symptoms of depression in a prospective birth cohort. Brain Behav Immun. 2019;76:74–81. https://doi.org/10.1016/j.bbi.2018.11.007 .
Lamers F, Milaneschi Y, de Jonge P, Giltay EJ, Penninx BWJH. Metabolic and inflammatory markers: associations with individual depressive symptoms. Psychol Med. 2018;48:1102–10. https://doi.org/10.1017/S0033291717002483 .
Choi SW, O’Reilly PF. PRSice-2: polygenic risk score software for biobank-scale data. Gigascience. 2019;8:1–6. https://doi.org/10.1093/gigascience/giz082 .
Download references
Acknowledgements
We are grateful to all original authors, research groups, funders, and participants of original RCTs comparing psychotherapy and ADM in depression that constituted the foundation for this investigation. We specifically thank the National Institute of Mental Health (NIMH) as sponsor of the Barber et al. (MH 061410 [JP Barber]), Jarrett et al. (MH-45043 & MH-38238 [RB Jarrett]), and McGrath et al. (R01 MH073719 [HS Mayberg], T32 GM08695 [CL McGrath], K23 MH086690 [BW Dunlop], & K23 MH077869 [PE Holtzheimer]) studies, as well as studies funded by the Australian National Health and Medical Research Council (#1037196 and #GNT1176689 [G Parker]).
We also thank Josefine Moultrie for her support in developing and piloting the search strategy and Britta Dumser for helping with the comparison of BDI and HAM-D symptoms and support on the Cochrane Risk of Bias tool. Lastly, we thank Marc Volkert for sharing his thoughts on the choice of effect size metric for meta-analysis and formulation of the SOrT metric.
This project is funded by the Max Planck Institute of Psychiatry. The MARS project was supported by grants of the German Federal Ministry of Education and Research (BMBF), project no. 01ES0811 (Molecular Diagnostics) and 01EE1401D (German Research Network for Mental Disorders). PReDICT study was supported by the following National Institutes of Health grants: P50 MH077083; R01 MH080880; UL1 RR025008; M01 RR0039; and the Fuqua family foundations. Forest Labs and Elli Lilly Inc. donated the study medications, escitalopram and duloxetine, respectively, and were otherwise uninvolved in the study design, data collection, data analysis, or interpretation of findings.
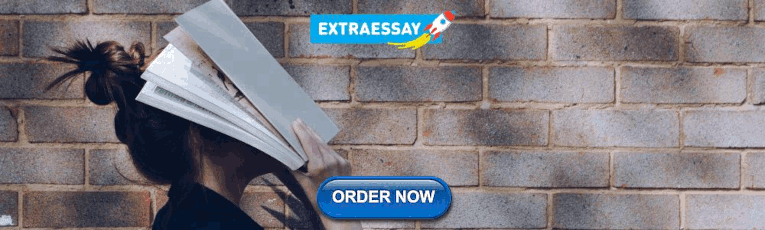
Author information
Authors and affiliations.
Max Planck Institute of Psychiatry, Kraepelinstraße 2-10, 80804, Munich, Germany
Nils Kappelmann, Martin Rein, Julia Fietz, Martin E. Keck & Johannes Kopf-Beck
International Max Planck Research School for Translational Psychiatry (IMPRS-TP), Munich, Germany
Nils Kappelmann & Julia Fietz
Department of Neurology and Neurosurgery, Icahn School of Medicine at Mount Sinai, New York, NY, USA
Helen S. Mayberg
Department of Psychiatry and Behavioral Sciences, Emory University School of Medicine, Atlanta, GA, USA
Helen S. Mayberg, W. Edward Craighead & Boadie W. Dunlop
Department of Psychology, Emory University, Atlanta, GA, USA
W. Edward Craighead
Institute for Early Life Adversity Research, University of Texas Dell Medical School in Austin, Austin, TX, USA
Charles B. Nemeroff
Department of Psychiatry and Human Behavior, Brown University School of Medicine, Providence, RI, USA
Martin Keller
Department of Psychology, Stony Brook University, Stony Brook, NY, USA
Daniel N. Klein
Department of Psychiatry and Behavioral Sciences, Stanford University, Stanford, CA, 94304, USA
Bruce A. Arnow
Division of Psychology and Mental Health, The University of Manchester, Manchester, UK
Nusrat Husain
Department of Psychiatry, The University of Texas Southwestern Medical Center, Dallas, TX, USA
Robin B. Jarrett
Department of Psychology, Truman State University, Kirksville, MO, USA
Jeffrey R. Vittengl
Department of Biomedical and Neuromotor Sciences, University of Bologna, Bologna, Italy
Marco Menchetti
School of Psychiatry, University of New South Wales, Sydney, NSW, Australia
Gordon Parker
Gordon F. Derner School of Psychology, Adelphi University, Garden City, New York, USA
Jacques P. Barber
Contemporary Institute of Psychoanalysis and Transdisciplinarity of Porto Alegre, Porto Alegre, Brazil
Andre G. Bastos
Department of Research, Arkin Mental Health Care, Amsterdam, Netherlands
Jack Dekker & Jaap Peen
You can also search for this author in PubMed Google Scholar
Contributions
NK had the initial idea for the project and its conceptualisation. NK drafted the manuscript with supervision and support from JKB. NK and JKB both performed the literature search, data extraction, and risk of bias ratings. NK was responsible for all statistical analyses and figures. NK and MR were responsible for the search strategy and timeline. JF was responsible for IPD and data management sections. HSM, WEC, BWD, CBN, MK, DNK, BAA, NH, RBJ, JRV, MM, GP, JPB, AGB, JD, and JP were responsible for providing and sharing expertise on their RCT data and related analyses. NK and JKB assume responsibility for the accuracy and integrity of this work. All authors critically reviewed the manuscript and have given approval to the final version of the manuscript.
Corresponding author
Correspondence to Nils Kappelmann .
Ethics declarations
Ethics approval and consent to participate.
For the systematic review part of this study, ethics approval was not applicable as it is necessary inclusion criteria for the to-be-evaluated RCTs.
Validation of the SOrT metric was conducted using the Munich Antidepressant Response Signature (MARS) study and Emory Predictors of Remission in Depression to Individual and Combined Treatments (PReDICT) study. MARS has received approval from the Ethics Committee of the Ludwig Maximilians University in Munich, Germany, and PReDICT from the Emory Institutional Review Board and the Grady Hospital Research Oversight Committee. Both studies were conducted in concordance with the Declaration of Helsinki [ 43 , 44 ]. Participants’ written informed consent was ascertained in MARS and PReDICT studies [ 43 , 44 ].
Consent for publication
Consent for publication was not generally applicable for the systematic review as the data from studies included in meta-analyses were in an individual symptom format (see explanation in data extraction and acquisition section), which is fully anonymous. However, we still checked whether authors of RCTs described that written informed consent was obtained, also because we allowed authors to send us individual patient data in case this made sharing of their data more convenient.
Competing interests
NK, MR, JF, BAA, AGB, MM, GP, AGB, JD, JP, and JKB do not have any competing financial or other interests relating to the content of this study. HSM holds intellectual property in the field of deep brain stimulation for depression and is a consultant to Abbott Labs who has licenced the IP. WEC is a board member of Hugarheill ehf, an Icelandic company dedicated to the prevention of depression, and he receives book royalties from John Wiley & Sons. His research is supported by the NIH, the Mary and John Brock Foundation, and the Fuqua family foundations. He is a consultant to the George West Mental Health Foundation and is a member of the Scientific Advisory Boards of the ADAA and AIM for Mental Health. BWD has received research support from Acadia, Assurex Health, Axsome, Intra-Cellular Therapies, Janssen, National Institute of Mental Health, and Takeda. He has served as a consultant to Assurex Health and Aptinyx. CBN has received funding from the National Institutes of Health and the Stanley Medical Research Institute. In the last 3 years, he has served as a consultant to Xhale, Takeda, Taisho Pharmaceutical Inc., Signant Health, Sunovion Pharmaceuticals Inc., Janssen Research & Development LLC, Magstim, Inc., Navitor Pharmaceuticals, Inc., TC MSO, Inc., Intra-Cellular Therapies, Inc., EMA Wellness, Gerson Lehrman Group (GLG), and Acadia Pharmaceuticals, and served on the Board of Directors for the Gratitude America, Anxiety Disorders Association of America (ADAA), and Xhale Smart, Inc. CBN is a stockholder in Xhale, Celgene, Seattle Genetics, Abbvie, OPKO Health, Inc., Antares, BI Gen Holdings, Inc., Corcept Therapeutics Pharmaceuticals Company, TC MSO, Inc., Trends in Pharma Development, LLC, and EMA Wellness, and serves on the Scientific Advisory Boards of the American Foundation for Suicide Prevention (AFSP), Brain and Behavior Research Foundation (BBRF), Xhale, ADAA, Skyland Trail, Signant Health, Laureate Institute for Brain Research (LIBR), Inc. CBN reports income sources or equity of $10,000 or more from American Psychiatric Publishing, Xhale, Signant Health, CME Outfitters, Intra-Cellular Therapies, Inc., Magstim, and EMA Wellness, and has patents on the method and devices for transdermal delivery of lithium (US 6,375,990B1), the method of assessing antidepressant drug therapy via transport inhibition of monoamine neurotransmitters by ex vivo assay (US 7,148,027B2), and Compounds, Compositions, Methods of Synthesis, and Methods of Treatment (CRF Receptor Binding Ligand) (US 8,551, 996 B2). MK is supported by the NIH and The John J. McDonnell and Margaret T.O. O’Brien Foundation. DNK receives grant funding from the National Institute of Mental Health (NIMH). NH is the chair of the board of trustees of Manchester Global Foundation (MGF) which was founded in 2015 as a Charitable Incorporated Organisation (CIO) registered in England and Wales. NH is the past Trustee of Pakistan Institute of Living & Learning (PILL), Abaseen Foundation and Lancashire Mind. NH has attended educational events sponsored by pharmaceutical industry. RBJ’s medical center collects the payments from the cognitive therapy she provides to patients. RBJ is a paid consultant to the National Institute of Mental Health and is a paid reviewer for UpToDate. She owns stock equity in Amgen, Johnson and Johnson, and Procter and Gamble. JRV is a paid reviewer for UpToDate. JPB received medication and placebo from Pfizer and NIMH funding for the data he contributed to the present study. MEK reports the following potential conflicts of interest: speakers bureau honoraria and other continuing medical education activity: AstraZeneca (Switzerland), Eli Lilly (Switzerland), Lundbeck (Switzerland), Vifor (Switzerland), and Zeller (Switzerland), as well as advisory panel payment from Lundbeck Switzerland.
Additional information
Publisher’s note.
Springer Nature remains neutral with regard to jurisdictional claims in published maps and institutional affiliations.
Supplementary information
Additional file 1: table s1..
Search Strategy for Identification of RCTs of Psychotherapy versus Pharmacotherapy for Depression.
Additional file 2.
Standardised author contacting and quality evaluation procedure.
Additional file 3 Tables S2-S4.
Content overlap assessment of Beck Depression Inventory (BDI) and Hamilton Rating Scale for Depression (HAM-D). Table S2- Content Overlap of HAM-D and BDI-II Items Sorted by Item Numbering. Table S3- Content Overlap of HAM-D and BDI-II Items Sorted by Equivalent Items. Table S4- Content Overlap of BDI-I and BDI-II Items Sorted by Item Numbering.
Additional file 4: Table S5.
Development and considerations of a Symptom-Oriented Therapy (SOrT) metric. Table S5- Hypothetical SOrT Metric Computation for Three Patients.
Additional file 5.
Timeline and timeline adherence of the registered report.
Additional file 6: Table S6.
Study overview of RCTs included in qualitative synthesis.
Additional file 7: Table S7, Figs. S1-S5.
Sum-score meta-analysis results. Table S7- Meta-regression of HAM-D and BDI sum-score meta-analyses on differential dropout. Fig. S1- Funnel plot of HAM-D sum-score meta-analysis. Fig. S2- Funnel plot of BDI sum-score meta-analysis. Fig. S3- Meta-Regression of HAM-D sum-score meta-analysis on differential dropout. Fig. S4- Meta-Regression of BDI sum-score meta-analysis on differential dropout. Fig. S5- Funnel plot of HAM-D sum-score meta-analysis. Fig. S6- Forest plot of dropout meta-analysis.
Additional file 8: Tables S8-S10, Figs. S6-S10.
Individual symptom meta-analysis results and related sensitivity and exploratory analyses. Table S8- Individual symptom forest plots for the HAM-D. Table S9- Individual symptom forest plots for the BDI. Table S10- Correlations between meta-analytic effect size metrics. Fig. S6- Effect size comparison of HAM-D and BDI per symptom type. Fig. S7- Individual symptom effect sizes per effect size metric used in meta-analyses. Fig. S8- Associations between meta-analytic effect size metrics. Fig. S9- Effect size association to Boschloo et al. Fig. S10- Effect size association to Boschloo et al. for RCTs with CBT only.
Additional file 9: Tables S11-S18, Fig. S11.
Validation analyses of SOrT metric in MARS and PReDICT samples and related exploratory analyses. Table S11- Effect sizes of individual symptom meta-analyses with versus without Dunlop et al. Table S12- Linear regression analysis of 12-week HAM-D sum-scores on SOrT-based BDI treatment allocation match following valence split. Table S13- Linear regression analysis of 12-week HAM-D sum-scores on SOrT-based treatment allocation match following median split. Table S14- Linear regression analysis of 12-week HAM-D sum-scores on SOrT-based treatment allocation match following 2/3 extreme group split. Table S15- Treatment allocation match prediction using Boschloo et al.-based SOrT and sum-scores. Table S16- Regression-based treatment allocation match prediction using Boschloo et al.-based SOrT and sum-scores. Table S17- Association of symptom severity with updated SOrT scores. Table S18- Treatment allocation match prediction using updated SOrT metric. Fig. S11- Association and distributions of HAM-D and BDI SOrT scores in PReDICT.
Additional file 10.
Discussion on nominally significant treatment differences of psychotherapy and ADM for specific depressive symptoms.
Additional file 11: Tables S19-S20.
Overview of available data and materials from original studies and presented work. Table S19 Availability of Original Study Data. Table S20. Online File Overview as Available on https://osf.io/qzjc9/ .
Rights and permissions
Open Access This article is licensed under a Creative Commons Attribution 4.0 International License, which permits use, sharing, adaptation, distribution and reproduction in any medium or format, as long as you give appropriate credit to the original author(s) and the source, provide a link to the Creative Commons licence, and indicate if changes were made. The images or other third party material in this article are included in the article's Creative Commons licence, unless indicated otherwise in a credit line to the material. If material is not included in the article's Creative Commons licence and your intended use is not permitted by statutory regulation or exceeds the permitted use, you will need to obtain permission directly from the copyright holder. To view a copy of this licence, visit http://creativecommons.org/licenses/by/4.0/ . The Creative Commons Public Domain Dedication waiver ( http://creativecommons.org/publicdomain/zero/1.0/ ) applies to the data made available in this article, unless otherwise stated in a credit line to the data.
Reprints and permissions
About this article
Cite this article.
Kappelmann, N., Rein, M., Fietz, J. et al. Psychotherapy or medication for depression? Using individual symptom meta-analyses to derive a Symptom-Oriented Therapy (SOrT) metric for a personalised psychiatry. BMC Med 18 , 170 (2020). https://doi.org/10.1186/s12916-020-01623-9
Download citation
Received : 12 April 2018
Accepted : 07 May 2020
Published : 05 June 2020
DOI : https://doi.org/10.1186/s12916-020-01623-9
Share this article
Anyone you share the following link with will be able to read this content:
Sorry, a shareable link is not currently available for this article.
Provided by the Springer Nature SharedIt content-sharing initiative
- Major depressive disorder
- Depression symptoms
- Psychotherapy
- Systematic review
- Meta-analysis
- Precision psychiatry
- Symptom-oriented therapy metric
BMC Medicine
ISSN: 1741-7015
- Submission enquiries: [email protected]
- General enquiries: [email protected]
Change Password
Your password must have 6 characters or more:.
- a lower case character,
- an upper case character,
- a special character
Password Changed Successfully
Your password has been changed
Create your account
Forget yout password.
Enter your email address below and we will send you the reset instructions
If the address matches an existing account you will receive an email with instructions to reset your password
Forgot your Username?
Enter your email address below and we will send you your username
If the address matches an existing account you will receive an email with instructions to retrieve your username

- April 01, 2024 | VOL. 181, NO. 4 CURRENT ISSUE pp.255-346
- March 01, 2024 | VOL. 181, NO. 3 pp.171-254
- February 01, 2024 | VOL. 181, NO. 2 pp.83-170
- January 01, 2024 | VOL. 181, NO. 1 pp.1-82
The American Psychiatric Association (APA) has updated its Privacy Policy and Terms of Use , including with new information specifically addressed to individuals in the European Economic Area. As described in the Privacy Policy and Terms of Use, this website utilizes cookies, including for the purpose of offering an optimal online experience and services tailored to your preferences.
Please read the entire Privacy Policy and Terms of Use. By closing this message, browsing this website, continuing the navigation, or otherwise continuing to use the APA's websites, you confirm that you understand and accept the terms of the Privacy Policy and Terms of Use, including the utilization of cookies.
The Critical Relationship Between Anxiety and Depression
- Ned H. Kalin , M.D.
Search for more papers by this author
Anxiety and depressive disorders are among the most common psychiatric illnesses; they are highly comorbid with each other, and together they are considered to belong to the broader category of internalizing disorders. Based on statistics from the Substance Abuse and Mental Health Services Administration, the 12-month prevalence of major depressive disorder in 2017 was estimated to be 7.1% for adults and 13.3% for adolescents ( 1 ). Data for anxiety disorders are less current, but in 2001–2003, their 12-month prevalence was estimated to be 19.1% in adults, and 2001–2004 data estimated that the lifetime prevalence in adolescents was 31.9% ( 2 , 3 ). Both anxiety and depressive disorders are more prevalent in women, with an approximate 2:1 ratio in women compared with men during women’s reproductive years ( 1 , 2 ).
Across all psychiatric disorders, comorbidity is the rule ( 4 ), which is definitely the case for anxiety and depressive disorders, as well as their symptoms. With respect to major depression, a worldwide survey reported that 45.7% of individuals with lifetime major depressive disorder had a lifetime history of one or more anxiety disorder ( 5 ). These disorders also commonly coexist during the same time frame, as 41.6% of individuals with 12-month major depression also had one or more anxiety disorder over the same 12-month period. From the perspective of anxiety disorders, the lifetime comorbidity with depression is estimated to range from 20% to 70% for patients with social anxiety disorder ( 6 ), 50% for patients with panic disorder ( 6 ), 48% for patients with posttraumatic stress disorder (PTSD) ( 7 ), and 43% for patients with generalized anxiety disorder ( 8 ). Data from the well-known Sequenced Treatment Alternatives to Relieve Depression (STAR*D) study demonstrate comorbidity at the symptom level, as 53% of the patients with major depression had significant anxiety and were considered to have an anxious depression ( 9 ).
Anxiety and depressive disorders are moderately heritable (approximately 40%), and evidence suggests shared genetic risk across the internalizing disorders ( 10 ). Among internalizing disorders, the highest level of shared genetic risk appears to be between major depressive disorder and generalized anxiety disorder. Neuroticism is a personality trait or temperamental characteristic that is associated with the development of both anxiety and depression, and the genetic risk for developing neuroticism also appears to be shared with that of the internalizing disorders ( 11 ). Common nongenetic risk factors associated with the development of anxiety and depression include earlier life adversity, such as trauma or neglect, as well as parenting style and current stress exposure. At the level of neural circuits, alterations in prefrontal-limbic pathways that mediate emotion regulatory processes are common to anxiety and depressive disorders ( 12 , 13 ). These findings are consistent with meta-analyses that reveal shared structural and functional brain alterations across various psychiatric illnesses, including anxiety and major depression, in circuits involving emotion regulation ( 13 ), executive function ( 14 ), and cognitive control ( 15 ).
Anxiety disorders and major depression occur during development, with anxiety disorders commonly beginning during preadolescence and early adolescence and major depression tending to emerge during adolescence and early to mid-adulthood ( 16 – 18 ). In relation to the evolution of their comorbidity, studies demonstrate that anxiety disorders generally precede the presentation of major depressive disorder ( 17 ). A European community-based study revealed, beginning at age 15, the developmental relation between comorbid anxiety and major depression by specifically focusing on social phobia (based on DSM-IV criteria) and then asking the question regarding concurrent major depressive disorder ( 18 ). The findings revealed a 19% concurrent comorbidity between these disorders, and in 65% of the cases, social phobia preceded major depressive disorder by at least 2 years. In addition, initial presentation with social phobia was associated with a 5.7-fold increased risk of developing major depressive disorder. These associations between anxiety and depression can be traced back even earlier in life. For example, childhood behavioral inhibition in response to novelty or strangers, or an extreme anxious temperament, is associated with a three- to fourfold increase in the likelihood of developing social anxiety disorder, which in turn is associated with an increased risk to develop major depressive disorder and substance abuse ( 19 ).
It is important to emphasize that the presence of comor‐bid anxiety symptoms and disorders matters in relation to treatment. Across psychiatric disorders, the presence of significant anxiety symptoms generally predicts worse outcomes, and this has been well demonstrated for depression. In the STAR*D study, patients with anxious major depressive disorder were more likely to be severely depressed and to have more suicidal ideation ( 9 ). This is consistent with the study by Kessler and colleagues ( 5 ), in which patients with anxious major depressive disorder, compared with patients with nonanxious major depressive disorder, were found to have more severe role impairment and more suicidal ideation. Data from level 1 of the STAR*D study (citalopram treatment) nicely illustrate the impact of comorbid anxiety symptoms on treatment. Compared with patients with nonanxious major depressive disorder, those 53% of patients with an anxious depression were less likely to remit and also had a greater side effect burden ( 20 ). Other data examining patients with major depressive disorder and comorbid anxiety disorders support the greater difficulty and challenge in treating patients with these comorbidities ( 21 ).
This issue of the Journal presents new findings relevant to the issues discussed above in relation to understanding and treating anxiety and depressive disorders. Drs. Conor Liston and Timothy Spellman, from Weill Cornell Medicine, provide an overview for this issue ( 22 ) that is focused on understanding mechanisms at the neural circuit level that underlie the pathophysiology of depression. Their piece nicely integrates human neuroimaging studies with complementary data from animal models that allow for the manipulation of selective circuits to test hypotheses generated from the human data. Also included in this issue is a review of the data addressing the reemergence of the use of psychedelic drugs in psychiatry, particularly for the treatment of depression, anxiety, and PTSD ( 23 ). This timely piece, authored by Dr. Collin Reiff along with a subgroup from the APA Council of Research, provides the current state of evidence supporting the further exploration of these interventions. Dr. Alan Schatzberg, from Stanford University, contributes an editorial in which he comments on where the field is in relation to clinical trials with psychedelics and to some of the difficulties, such as adequate blinding, in reliably studying the efficacy of these drugs ( 24 ).
In an article by McTeague et al. ( 25 ), the authors use meta-analytic strategies to understand the neural alterations that are related to aberrant emotion processing that are shared across psychiatric disorders. Findings support alterations in the salience, reward, and lateral orbital nonreward networks as common across disorders, including anxiety and depressive disorders. These findings add to the growing body of work that supports the concept that there are common underlying factors across all types of psychopathology that include internalizing, externalizing, and thought disorder dimensions ( 26 ). Dr. Deanna Barch, from Washington University in St. Louis, writes an editorial commenting on these findings and, importantly, discusses criteria that should be met when we consider whether the findings are actually transdiagnostic ( 27 ).
Another article, from Gray and colleagues ( 28 ), addresses whether there is a convergence of findings, specifically in major depression, when examining data from different structural and functional neuroimaging modalities. The authors report that, consistent with what we know about regions involved in emotion processing, the subgenual anterior cingulate cortex, hippocampus, and amygdala were among the regions that showed convergence across multimodal imaging modalities.
In relation to treatment and building on our understanding of neural circuit alterations, Siddiqi et al. ( 29 ) present data suggesting that transcranial magnetic stimulation (TMS) targeting can be linked to symptom-specific treatments. Their findings identify different TMS targets in the left dorsolateral prefrontal cortex that modulate different downstream networks. The modulation of these different networks appears to be associated with a reduction in different types of symptoms. In an editorial, Drs. Sean Nestor and Daniel Blumberger, from the University of Toronto ( 30 ), comment on the novel approach used in this study to link the TMS-related engagement of circuits with symptom improvement. They also provide a perspective on how we can view these and other circuit-based findings in relation to conceptualizing personalized treatment approaches.
Kendler et al. ( 31 ), in this issue, contribute an article that demonstrates the important role of the rearing environment in the risk to develop major depression. Using a unique design from a Swedish sample, the analytic strategy involves comparing outcomes from high-risk full sibships and high-risk half sibships where at least one of the siblings was home reared and one was adopted out of the home. The findings support the importance of the quality of the rearing environment as well as the presence of parental depression in mitigating or enhancing the likelihood of developing major depression. In an accompanying editorial ( 32 ), Dr. Myrna Weissman, from Columbia University, reviews the methods and findings of the Kendler et al. article and also emphasizes the critical significance of the early nurturing environment in relation to general health.
This issue concludes with an intriguing article on anxiety disorders, by Gold and colleagues ( 33 ), that demonstrates neural alterations during extinction recall that differ in children relative to adults. With increasing age, and in relation to fear and safety cues, nonanxious adults demonstrated greater connectivity between the amygdala and the ventromedial prefrontal cortex compared with anxious adults, as the cues were being perceived as safer. In contrast, neural differences between anxious and nonanxious youths were more robust when rating the memory of faces that were associated with threat. Specifically, these differences were observed in the activation of the inferior temporal cortex. In their editorial ( 34 ), Dr. Dylan Gee and Sahana Kribakaran, from Yale University, emphasize the importance of developmental work in relation to understanding anxiety disorders, place these findings into the context of other work, and suggest the possibility that these and other data point to neuroscientifically informed age-specific interventions.
Taken together, the papers in this issue of the Journal present new findings that shed light onto alterations in neural function that underlie major depressive disorder and anxiety disorders. It is important to remember that these disorders are highly comorbid and that their symptoms are frequently not separable. The papers in this issue also provide a developmental perspective emphasizing the importance of early rearing in the risk to develop depression and age-related findings important for understanding threat processing in patients with anxiety disorders. From a treatment perspective, the papers introduce data supporting more selective prefrontal cortical TMS targeting in relation to different symptoms, address the potential and drawbacks for considering the future use of psychedelics in our treatments, and present new ideas supporting age-specific interventions for youths and adults with anxiety disorders.
Disclosures of Editors’ financial relationships appear in the April 2020 issue of the Journal .
1 Substance Abuse and Mental Health Services Administration (SAMHSA): Key substance use and mental health indicators in the United States: results from the 2017 National Survey on Drug Use and Health (HHS Publication No. SMA 18-5068, NSDUH Series H-53). Rockville, Md, Center for Behavioral Health Statistics and Quality, SAMHSA, 2018. https://www.samhsa.gov/data/sites/default/files/cbhsq-reports/NSDUHFFR2017/NSDUHFFR2017.htm Google Scholar
2 Kessler RC, Chiu WT, Demler O, et al. : Prevalence, severity, and comorbidity of 12-month DSM-IV disorders in the National Comorbidity Survey Replication . Arch Gen Psychiatry 2005 ; 62:617–627, correction, 62:709 Crossref , Medline , Google Scholar
3 Merikangas KR, He JP, Burstein M, et al. : Lifetime prevalence of mental disorders in U.S. adolescents: results from the National Comorbidity Survey Replication–Adolescent Supplement (NCS-A) . J Am Acad Child Adolesc Psychiatry 2010 ; 49:980–989 Crossref , Medline , Google Scholar
4 Kessler RC, McGonagle KA, Zhao S, et al. : Lifetime and 12-month prevalence of DSM-III-R psychiatric disorders in the United States: results from the National Comorbidity Survey . Arch Gen Psychiatry 1994 ; 51:8–19 Crossref , Medline , Google Scholar
5 Kessler RC, Sampson NA, Berglund P, et al. : Anxious and non-anxious major depressive disorder in the World Health Organization World Mental Health Surveys . Epidemiol Psychiatr Sci 2015 ; 24:210–226 Crossref , Medline , Google Scholar
6 Dunner DL : Management of anxiety disorders: the added challenge of comorbidity . Depress Anxiety 2001 ; 13:57–71 Crossref , Medline , Google Scholar
7 Kessler RC, Sonnega A, Bromet E, et al. : Posttraumatic stress disorder in the National Comorbidity Survey . Arch Gen Psychiatry 1995 ; 52:1048–1060 Crossref , Medline , Google Scholar
8 Brawman-Mintzer O, Lydiard RB, Emmanuel N, et al. : Psychiatric comorbidity in patients with generalized anxiety disorder . Am J Psychiatry 1993 ; 150:1216–1218 Link , Google Scholar
9 Fava M, Alpert JE, Carmin CN, et al. : Clinical correlates and symptom patterns of anxious depression among patients with major depressive disorder in STAR*D . Psychol Med 2004 ; 34:1299–1308 Crossref , Medline , Google Scholar
10 Hettema JM : What is the genetic relationship between anxiety and depression? Am J Med Genet C Semin Med Genet 2008 ; 148C:140–146 Crossref , Medline , Google Scholar
11 Hettema JM, Neale MC, Myers JM, et al. : A population-based twin study of the relationship between neuroticism and internalizing disorders . Am J Psychiatry 2006 ; 163:857–864 Link , Google Scholar
12 Kovner R, Oler JA, Kalin NH : Cortico-limbic interactions mediate adaptive and maladaptive responses relevant to psychopathology . Am J Psychiatry 2019 ; 176:987–999 Link , Google Scholar
13 Etkin A, Schatzberg AF : Common abnormalities and disorder-specific compensation during implicit regulation of emotional processing in generalized anxiety and major depressive disorders . Am J Psychiatry 2011 ; 168:968–978 Link , Google Scholar
14 Goodkind M, Eickhoff SB, Oathes DJ, et al. : Identification of a common neurobiological substrate for mental illness . JAMA Psychiatry 2015 ; 72:305–315 Crossref , Medline , Google Scholar
15 McTeague LM, Huemer J, Carreon DM, et al. : Identification of common neural circuit disruptions in cognitive control across psychiatric disorders . Am J Psychiatry 2017 ; 174:676–685 Link , Google Scholar
16 Beesdo K, Knappe S, Pine DS : Anxiety and anxiety disorders in children and adolescents: developmental issues and implications for DSM-V . Psychiatr Clin North Am 2009 ; 32:483–524 Crossref , Medline , Google Scholar
17 Kessler RC, Wang PS : The descriptive epidemiology of commonly occurring mental disorders in the United States . Annu Rev Public Health 2008 ; 29:115–129 Crossref , Medline , Google Scholar
18 Ohayon MM, Schatzberg AF : Social phobia and depression: prevalence and comorbidity . J Psychosom Res 2010 ; 68:235–243 Crossref , Medline , Google Scholar
19 Clauss JA, Blackford JU : Behavioral inhibition and risk for developing social anxiety disorder: a meta-analytic study . J Am Acad Child Adolesc Psychiatry 2012 ; 51:1066–1075 Crossref , Medline , Google Scholar
20 Fava M, Rush AJ, Alpert JE, et al. : Difference in treatment outcome in outpatients with anxious versus nonanxious depression: a STAR*D report . Am J Psychiatry 2008 ; 165:342–351 Link , Google Scholar
21 Dold M, Bartova L, Souery D, et al. : Clinical characteristics and treatment outcomes of patients with major depressive disorder and comorbid anxiety disorders: results from a European multicenter study . J Psychiatr Res 2017 ; 91:1–13 Crossref , Medline , Google Scholar
22 Spellman T, Liston C : Toward circuit mechanisms of pathophysiology in depression . Am J Psychiatry 2020 ; 177:381–390 Link , Google Scholar
23 Reiff CM, Richman EE, Nemeroff CB, et al. : Psychedelics and psychedelic-assisted psychotherapy . Am J Psychiatry 2020 ; 177:391–410 Link , Google Scholar
24 Schatzberg AF : Some comments on psychedelic research (editorial). Am J Psychiatry 2020 ; 177:368–369 Link , Google Scholar
25 McTeague LM, Rosenberg BM, Lopez JW, et al. : Identification of common neural circuit disruptions in emotional processing across psychiatric disorders . Am J Psychiatry 2020 ; 177:411–421 Link , Google Scholar
26 Caspi A, Moffitt TE : All for one and one for all: mental disorders in one dimension . Am J Psychiatry 2018 ; 175:831–844 Link , Google Scholar
27 Barch DM : What does it mean to be transdiagnostic and how would we know? (editorial). Am J Psychiatry 2020 ; 177:370–372 Abstract , Google Scholar
28 Gray JP, Müller VI, Eickhoff SB, et al. : Multimodal abnormalities of brain structure and function in major depressive disorder: a meta-analysis of neuroimaging studies . Am J Psychiatry 2020 ; 177:422–434 Link , Google Scholar
29 Siddiqi SH, Taylor SF, Cooke D, et al. : Distinct symptom-specific treatment targets for circuit-based neuromodulation . Am J Psychiatry 2020 ; 177:435–446 Link , Google Scholar
30 Nestor SM, Blumberger DM : Mapping symptom clusters to circuits: toward personalizing TMS targets to improve treatment outcomes in depression (editorial). Am J Psychiatry 2020 ; 177:373–375 Abstract , Google Scholar
31 Kendler KS, Ohlsson H, Sundquist J, et al. : The rearing environment and risk for major depression: a Swedish national high-risk home-reared and adopted-away co-sibling control study . Am J Psychiatry 2020 ; 177:447–453 Abstract , Google Scholar
32 Weissman MM : Is depression nature or nurture? Yes (editorial). Am J Psychiatry 2020 ; 177:376–377 Abstract , Google Scholar
33 Gold AL, Abend R, Britton JC, et al. : Age differences in the neural correlates of anxiety disorders: an fMRI study of response to learned threat . Am J Psychiatry 2020 ; 177:454–463 Link , Google Scholar
34 Gee DG, Kribakaran S : Developmental differences in neural responding to threat and safety: implications for treating youths with anxiety (editorial). Am J Psychiatry 2020 ; 177:378–380 Abstract , Google Scholar
- Cited by None
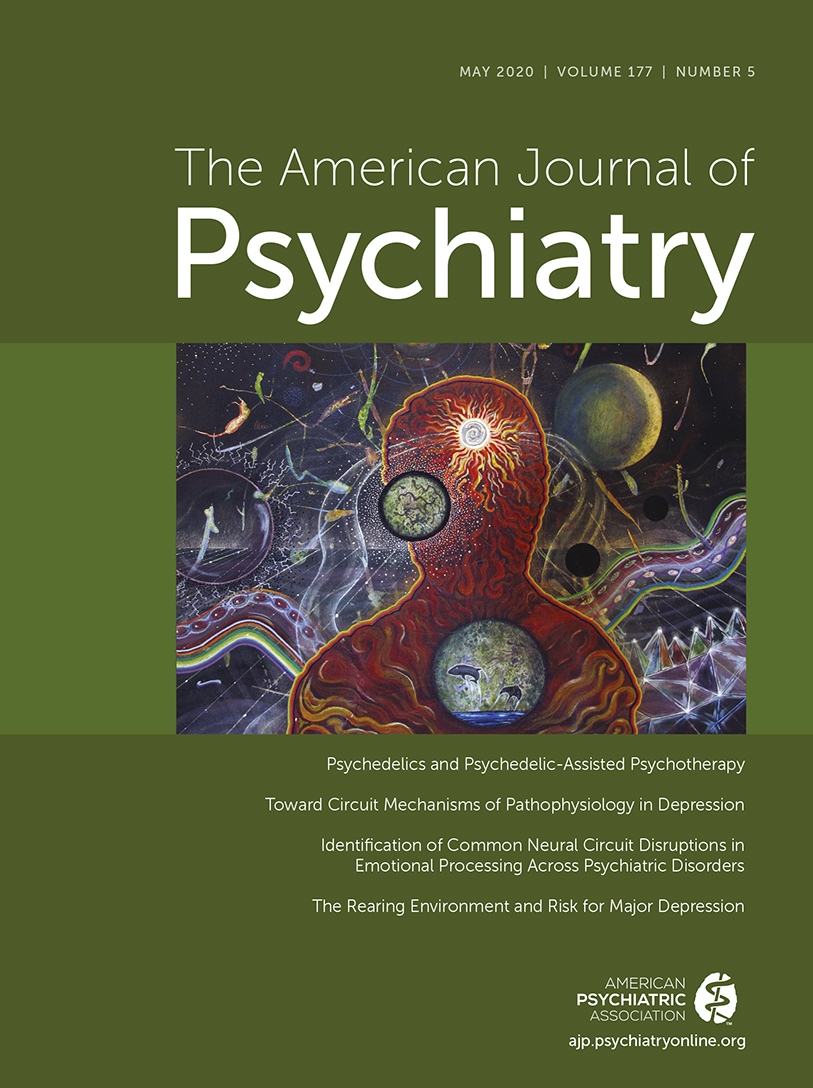
- Neuroanatomy
- Neurochemistry
- Neuroendocrinology
- Other Research Areas
- Bipolar Disorder
- Therapy Center
- When To See a Therapist
- Types of Therapy
- Best Online Therapy
- Best Couples Therapy
- Best Family Therapy
- Managing Stress
- Sleep and Dreaming
- Understanding Emotions
- Self-Improvement
- Healthy Relationships
- Student Resources
- Personality Types
- Guided Meditations
- Verywell Mind Insights
- 2023 Verywell Mind 25
- Mental Health in the Classroom
- Editorial Process
- Meet Our Review Board
- Crisis Support
7 Depression Research Paper Topic Ideas
Nancy Schimelpfening, MS is the administrator for the non-profit depression support group Depression Sanctuary. Nancy has a lifetime of experience with depression, experiencing firsthand how devastating this illness can be.
Cara Lustik is a fact-checker and copywriter.
:max_bytes(150000):strip_icc():format(webp)/Cara-Lustik-1000-77abe13cf6c14a34a58c2a0ffb7297da.jpg)
In psychology classes, it's common for students to write a depression research paper. Researching depression may be beneficial if you have a personal interest in this topic and want to learn more, or if you're simply passionate about this mental health issue. However, since depression is a very complex subject, it offers many possible topics to focus on, which may leave you wondering where to begin.
If this is how you feel, here are a few research titles about depression to help inspire your topic choice. You can use these suggestions as actual research titles about depression, or you can use them to lead you to other more in-depth topics that you can look into further for your depression research paper.
What Is Depression?
Everyone experiences times when they feel a little bit blue or sad. This is a normal part of being human. Depression, however, is a medical condition that is quite different from everyday moodiness.
Your depression research paper may explore the basics, or it might delve deeper into the definition of clinical depression or the difference between clinical depression and sadness .
What Research Says About the Psychology of Depression
Studies suggest that there are biological, psychological, and social aspects to depression, giving you many different areas to consider for your research title about depression.
Types of Depression
There are several different types of depression that are dependent on how an individual's depression symptoms manifest themselves. Depression symptoms may vary in severity or in what is causing them. For instance, major depressive disorder (MDD) may have no identifiable cause, while postpartum depression is typically linked to pregnancy and childbirth.
Depressive symptoms may also be part of an illness called bipolar disorder. This includes fluctuations between depressive episodes and a state of extreme elation called mania. Bipolar disorder is a topic that offers many research opportunities, from its definition and its causes to associated risks, symptoms, and treatment.
Causes of Depression
The possible causes of depression are many and not yet well understood. However, it most likely results from an interplay of genetic vulnerability and environmental factors. Your depression research paper could explore one or more of these causes and reference the latest research on the topic.
For instance, how does an imbalance in brain chemistry or poor nutrition relate to depression? Is there a relationship between the stressful, busier lives of today's society and the rise of depression? How can grief or a major medical condition lead to overwhelming sadness and depression?
Who Is at Risk for Depression?
This is a good research question about depression as certain risk factors may make a person more prone to developing this mental health condition, such as a family history of depression, adverse childhood experiences, stress , illness, and gender . This is not a complete list of all risk factors, however, it's a good place to start.
The growing rate of depression in children, teenagers, and young adults is an interesting subtopic you can focus on as well. Whether you dive into the reasons behind the increase in rates of depression or discuss the treatment options that are safe for young people, there is a lot of research available in this area and many unanswered questions to consider.
Depression Signs and Symptoms
The signs of depression are those outward manifestations of the illness that a doctor can observe when they examine a patient. For example, a lack of emotional responsiveness is a visible sign. On the other hand, symptoms are subjective things about the illness that only the patient can observe, such as feelings of guilt or sadness.
An illness such as depression is often invisible to the outside observer. That is why it is very important for patients to make an accurate accounting of all of their symptoms so their doctor can diagnose them properly. In your depression research paper, you may explore these "invisible" symptoms of depression in adults or explore how depression symptoms can be different in children .
How Is Depression Diagnosed?
This is another good depression research topic because, in some ways, the diagnosis of depression is more of an art than a science. Doctors must generally rely upon the patient's set of symptoms and what they can observe about them during their examination to make a diagnosis.
While there are certain laboratory tests that can be performed to rule out other medical illnesses as a cause of depression, there is not yet a definitive test for depression itself.
If you'd like to pursue this topic, you may want to start with the Diagnostic and Statistical Manual of Mental Disorders (DSM). The fifth edition, known as DSM-5, offers a very detailed explanation that guides doctors to a diagnosis. You can also compare the current model of diagnosing depression to historical methods of diagnosis—how have these updates improved the way depression is treated?
Treatment Options for Depression
The first choice for depression treatment is generally an antidepressant medication. Selective serotonin reuptake inhibitors (SSRIs) are the most popular choice because they can be quite effective and tend to have fewer side effects than other types of antidepressants.
Psychotherapy, or talk therapy, is another effective and common choice. It is especially efficacious when combined with antidepressant therapy. Certain other treatments, such as electroconvulsive therapy (ECT) or vagus nerve stimulation (VNS), are most commonly used for patients who do not respond to more common forms of treatment.
Focusing on one of these treatments is an option for your depression research paper. Comparing and contrasting several different types of treatment can also make a good research title about depression.
A Word From Verywell
The topic of depression really can take you down many different roads. When making your final decision on which to pursue in your depression research paper, it's often helpful to start by listing a few areas that pique your interest.
From there, consider doing a little preliminary research. You may come across something that grabs your attention like a new study, a controversial topic you didn't know about, or something that hits a personal note. This will help you narrow your focus, giving you your final research title about depression.
Remes O, Mendes JF, Templeton P. Biological, psychological, and social determinants of depression: A review of recent literature . Brain Sci . 2021;11(12):1633. doi:10.3390/brainsci11121633
National Institute of Mental Health. Depression .
American Psychiatric Association. Diagnostic and Statistical Manual of Mental Disorders, Fifth Edition . American Psychiatric Association.
National Institute of Mental Health. Mental health medications .
Ferri, F. F. (2019). Ferri's Clinical Advisor 2020 E-Book: 5 Books in 1 . Netherlands: Elsevier Health Sciences.
By Nancy Schimelpfening Nancy Schimelpfening, MS is the administrator for the non-profit depression support group Depression Sanctuary. Nancy has a lifetime of experience with depression, experiencing firsthand how devastating this illness can be.
This paper is in the following e-collection/theme issue:
Published on 9.4.2024 in Vol 8 (2024)
Nudges and Prompts Increase Engagement in Self-Guided Digital Health Treatment for Depression and Anxiety: Results From a 3-Arm Randomized Controlled Trial
Authors of this article:

Original Paper
- Trevor van Mierlo 1 * , MBA, MScCH, MScBMR, GEMBA, DBA ;
- Renante Rondina 2 * , PhD ;
- Rachel Fournier 1 * , MSc
1 Evolution Health, Toronto, ON, Canada
2 Rotman School of Managment, University of Toronto, Toronto, ON, Canada
*all authors contributed equally
Corresponding Author:
Trevor van Mierlo, MBA, MScCH, MScBMR, GEMBA, DBA
Evolution Health
206-90 Eglinton Avenue East
Toronto, ON, M4P 2Y3
Phone: 1 4166448476
Email: [email protected]
Background: Accessible and effective approaches to mental health treatment are important because of common barriers such as cost, stigma, and provider shortage. The effectiveness of self-guided treatment is well established, and its use has intensified because of the COVID-19 pandemic. Engagement remains important as dose-response relationships have been observed. Platforms such as Facebook (Meta Platform, Inc), LinkedIn (Microsoft Corp), and X Corp (formerly known as Twitter, Inc) use principles of behavioral economics to increase engagement. We hypothesized that similar concepts would increase engagement in self-guided digital health.
Objective: This 3-arm randomized controlled trial aimed to test whether members of 2 digital self-health courses for anxiety and depression would engage with behavioral nudges and prompts. Our primary hypothesis was that members would click on 2 features: tips and a to-do checklist. Our secondary hypothesis was that members would prefer to engage with directive tips in arm 2 versus social proof and present bias tips in arm 3. Our tertiary hypothesis was that rotating tips and a to-do checklist would increase completion rates. The results of this study will form a baseline for future artificial intelligence–directed research.
Methods: Overall, 13,224 new members registered between November 2021 and May 2022 for Evolution Health’s self-guided treatment courses for anxiety and depression. The control arm featured a member home page without nudges or prompts. Arm 2 featured a home page with a tip-of-the-day section. Arm 3 featured a home page with a tip-of-the-day section and a to-do checklist. The research protocol for this study was published in JMIR Research Protocols on August 15, 2022.
Results: Arm 3 had significantly younger members ( F 2,4564 =40.97; P <.001) and significantly more female members ( χ 2 4 =92.2; P <.001) than the other 2 arms. Control arm members (1788/13,224, 13.52%) completed an average of 1.5 course components. Arm 2 members (865/13,224, 6.54%) clicked on 5% of tips and completed an average of 1.8 course components. Arm 3 members (1914/13,224, 14.47%) clicked on 5% of tips, completed 2.7 of 8 to-do checklist items, and completed an average of 2.11 course components. Completion rates in arm 2 were greater than those in arm 1 ( z score=3.37; P <.001), and completion rates in arm 3 were greater than those in arm 1 ( z score=12.23; P <.001). Engagement in all 8 components in arm 3 was higher than that in arm 2 ( z score=1.31; P <.001).
Conclusions: Members engaged with behavioral nudges and prompts. The results of this study may be important because efficacy is related to increased engagement. Due to its novel approach, the outcomes of this study should be interpreted with caution and used as a guideline for future research in this nascent field.
International Registered Report Identifier (IRRID): RR2-10.2196/37231
Introduction
Since its earliest use in the mid-1990s, digital health promised personalized treatments that patients could access from home. It was anticipated that treatment would have a broad reach, resulting in improved health outcomes and decreased costs [ 1 - 3 ]. Over the past 2 decades, and with increasing consistency, research examining the efficacy of self-guided digital health interventions show evidence of efficacy, especially for those with mental health concerns [ 4 - 7 ].
Although digital health interventions appear to be effective, poor adherence and lack of compliance have remained consistent patterns in research [ 8 - 10 ]. This pattern was first recognized in 2005 and coined The Law of Attrition [ 11 ].
As early as 2009, systematic reviews identified poor adherence and lack of compliance as engagement issues that required attention [ 12 ]. These issues persist, as demonstrated by a recent meta-analytic review on digital interventions for depression, which showed efficacy but highlighted compliance as a major challenge [ 13 ].
Adherence and compliance are complex and rooted in several systemic and individual factors [ 14 - 19 ]. However, it is an important topic as evidence indicates a dose-response relationship, and higher levels of engagement are associated with improved health outcomes [ 20 , 21 ].
Moreover, digital health interventions are becoming increasingly common and accessible. Patients’ use of, and trust, in these interventions has been intensified by the COVID-19 pandemic. The use of self-guided digital health interventions for mental health concerns is growing [ 22 , 23 ] because of access barriers such as high cost, stigma, and lack of access due to a shortage of professionals who can meet this growing demand [ 24 , 25 ].
How do we increase engagement in digital health programs to maximize their efficacy?
Behavioral Economics
Behavioral economics leverages psychological experimentation to develop theories about human decision-making. The field has identified a range of unconscious biases around how people think and feel [ 26 , 27 ].
The utility of behavioral economics is vast. Digital health has leveraged the discipline to investigate how people use digital health programs and to gain insights into the characteristics of people who use them. Several digital health studies have investigated the use of these strategies, including cooperative games and incentives [ 28 ], gamification [ 29 , 30 ], serious games [ 31 , 32 ], and positive behavioral support [ 33 , 34 ].
Our Use of Behavioral Economics
In our study, we examined the effectiveness of the nudge theory and behavioral prompts in 2 ad libitum self-guided digital behavior change courses.
Nudge Theory
Nudge theory, popularized in the 2008 book Nudge: Improving Decisions About Health, Wealth, and Happiness [ 27 ] , leverages indirect, positive suggestions to influence decision-making and behavior.
There is a lack of quality research analyzing the use of nudges in digital health. A 2019 scoping review examined the use of nudges in both web-based and real-world settings in physical activity interventions [ 35 ]. Of the 35 publications reviewed, 8 were web-based studies. The authors concluded that although nudging may be an effective approach to promote physical activity, there are large gaps in research, and further studies that are explicitly based on nudge insights are needed.
A 2020 editorial in Personalized Medicine addressed the meaningful adoption of nudges in digital health [ 36 ]. The authors acknowledged that using nudges in digital health interventions is rare and advocated for the use of nudges to promote positive behavior change.
Behavioral Prompts
In applied behavioral analysis, behavioral prompts are cues specifically designed to encourage a specific task [ 34 ]. In this study, we used 2 types of behavioral prompts anchored in the nudge theory: daily tips and a to-do checklist ( Table 1 ).
Directive, Social Proof, and Present Bias Tips
Directive content, as the name suggests, offers concrete suggestions to members [ 37 ]. These types of tips are brief and instructional.
Social proof is derived from the behavioral economics concept where we tend to copy the actions of those around us. These tips speak of our tendency to be swayed by other people’s choices, which we attempt to mirror [ 38 , 39 ].
Present bias is the inclination to prefer a smaller present reward now over a larger reward later. These tips encourage users to perform a task that provides an immediate benefit [ 40 , 41 ].
Our Use of Behavioral Prompts and Technical Functionality of Tips and to-Do Checklist Items
An example of a course tool in both Overcoming Anxiety and Overcoming Depression courses is goal setting. Goal setting is an important component of cognitive behavioral therapy (CBT). However, in a self-guided environment, many members are unsure of how to set personal goals. Evolution Health encourages members to review goals set by others as examples.
Figure 1 shows an example of a tip of the day that encourages members to review the goals of other members.
Figure 2 shows an example of the completed to-do checklist item, set goals . In this figure, the item is marked complete by a check box. This signifies that a member has clicked on the item and visited the page.
By clicking on either the tip ( Figure 2 ) or the to-do checklist item ( Figure 3 ), the member is brought to member goals, a course component that allows members to browse various goals set by other members ( Figure 3 ).
Engagement experiments in popular non–health care digital platforms are common. Although they are scientific in nature, they are not typically published, as they are conducted within private companies.
For example, social network sites such as Facebook (Meta Platforms, Inc), LinkedIn (Microsoft Corp), and X Corp. (formerly known as Twitter) generate revenue based on ad revenue derived from page views and the time members spend on their site. In a 2015 presentation, it was revealed that LinkedIn had >400 controlled experiments being conducted per day [ 42 ]. Similar studies with an ad libitum population are required for digital health, and this study is an attempt to fill this gap.
We have not observed sufficient evidence in the literature to determine whether nudges and prompts can be strategically applied to increase engagement with and decrease attrition in the courses for depression and anxiety [ 43 ]. Furthermore, because of the nascent state of behavioral economics within digital health, we did not find any quantitative benchmarks that would help us determine whether our use of nudges and prompts was successful.

Our primary hypothesis (H1) was that members would engage with the tips and to-do checklist. Engagement of the members was determined by the percentage of tips that were clicked on compared to the number shown. Because there is no prior literature, we first needed to establish baseline data. Data were reported as observational and served as a benchmark for future studies.
Our secondary hypothesis was that members would prefer to engage with directive tips in arm 2 versus social proof or present bias tips in arm 3. To assess the preference for engagement, we compared the number of tips clicked in arm 2 versus those clicked in arm 3.
Our tertiary hypothesis was that the addition of tips and a to-do checklist would increase completion rates with course tools. Increased completion rates were determined by comparing completion rates in arms 2 and 3 with those in arm 1 (control group).
In each hypothesis, we assessed whether engagement was influenced by gender or age.
The digital health platform used in this study was managed by Evolution Health. Evolution Health is an evidence-based, self-guided digital health platform that features courses and brief interventions based on behavior change techniques including CBT, stages of change, structured relapse prevention, normative feedback, and harm reduction.
The platform offers interactive courses and quizzes for people with mental health, addiction, and obesity issues. The platform contains a moderated community based on social cognitive theory.
Memberships are available to individuals who register through the organization’s free-to-consumer program and white-label instances that are licensed by employers, insurance companies, employee assistance programs, educational institutions, nonprofit organizations, for-profit health care organizations, and individual therapists.
The research protocol for this study was published in JMIR Research Protocols on August 15, 2022 [ 44 ]. The International Registered Report Identifier is DERR1-10.2196/37231.
The Interventions
The 2 interventions in this study contain self-guided interactive behavior change treatment courses based on best practices, and both have been examined extensively in the literature [ 9 , 45 - 53 ].
The 2 interventions have undergone several iterations. For example, Overcoming Anxiety was the first intervention noted in The Law of Attrition (previously known as The Panic Center) paper by Eysenbach [ 11 ]. In that iteration, the course contained a tunnel design with 12 successive sessions. The course now has a gamified free-form matrix design, among other technical and usability enhancements.
Table 2 outlines each course’s current theoretical construct and evidence base. Table 3 outlines the main course components.
a CBT: cognitive behavioral therapy.
Ethical Considerations
At registration, all members endorsed a checkbox to confirm that they consented to have their nonidentifiable data used for research purposes and approved the platform’s privacy policy. The participants did not receive compensation for their involvement. The platform is available in several languages, and the English language privacy policy and terms of use are presented in Multimedia Appendix 1 . All data collection policies and procedures adhered to the international privacy guidelines [ 54 - 56 ] and the Helsinki Declaration of 1975 [ 57 ].
This study was conducted on self-guided treatment for depression and anxiety, but it did not measure clinical outcomes. Although the study participants were randomly assigned to a control or intervention arm, the study was a randomized controlled trial and not a randomized clinical trial [ 58 ]. This study did not assess whether the study participants’ engagement with course tools decreased the depressive symptoms, severity of panic attacks, or frequency of panic attacks.
As described earlier, evidence indicates that higher levels of engagement are associated with improved health outcomes, and the literature observes dose-response relationships. However, any clinical outcomes related to the engagement strategies used in this study will need to be tested in future research.
As the study was based on unidentifiable data and no clinical measures were tested, it was deemed exempt from further review by the Evolution Health Institutional Review Board (IRB#000014034, FWA00033737).
Power and Sample Size
The study was designed to have a power of 0.95, indicating a 95% probability of correctly detecting a statistically significant difference in engagement with tips between the 2 treatment groups (arm 2 vs arm 3). On the basis of a 2-tailed t test with a conventional α level (.05) for statistical significance, we required a sample of 1302 members with 651 in each group to detect a small effect size of Cohen d =0.2. Refer to Multimedia Appendix 2 for the CONSORT-EHEALTH (Consolidated Standards of Reporting Trials of Electronic and Mobile Health Applications and Online Telehealth) checklist [ 59 ].
Randomization
During the registration process, new members were assigned to 1 of the 3 arms using a random number generator ( Figure 4 ). Randomization was conducted using simple randomization.

Intervention Groups
Members randomized to arm 1 were presented with a dashboard that did not contain behavioral nudges. Figure 5 presents a screenshot of the arm 1 dashboard for a member who chose to engage with the depression course.

Members randomized to arm 2 were presented with a dashboard that contained a tip-of-the-day section containing directive content. The randomization strategy for the 31 directive tips was randomization without replacement.
Figure 6 presents a screenshot of the arm 2 dashboard for a member who chose to engage with the depression course.

Members randomized to arm 3 were presented with 2 sections that contained nudges. The first was a tip-of-the-day section containing social proof and present bias cues. At each log-in, members saw a new tip. The randomization strategy for the tips was randomization without replacement. There were 15 social proof tips and 15 present bias tips.
In addition to the tip of the day, arm 3 featured a to-do checklist that listed 8 course components. When a member clicked on a component, they were brought to the exercise. As mentioned previously, if a member clicks on a to-do checklist item, it is marked complete with a check mark.
Figure 7 presents a screenshot of the arm 2 dashboard for a member who chose to engage with the depression course.

Data Collection
A custom data collection interface and reporting mechanism were developed by Evolution Health. Age and gender data were collected at registration or through a secure sign on with various white-label instances. Data were collected for each member who was randomized into the experiment. The course components promoted by tips and to-do checklist items are listed in Textbox 1 . Members who participated in both courses were not counted twice.
The following behaviors were tracked in the custom database for each tip and to-do checklist item that was randomly presented to a member:
- If the nudge was shown
- If the nudge was clicked on
- If a member completed the course component described in the tip or to-do checklist item.
It was possible for a single member to participate in both courses. However, the study design was to test the behavioral prompts, not the courses. The participants were randomized to an intervention arm, in which behavioral nudges and prompts were consistent across courses.
Action code and course component
- 1 (item in the to-do checklist): Uploading a personal image (avatar) to their profile
- 2 (item in the to-do checklist): Completing cognitive behavioral therapy session 1
- 3 (item in the to-do checklist): Use of the program diary (mood tracker or symptom tracker)
- 4 (item in the to-do checklist): Read a community post
- 5 (item in the to-do checklist): Review a worksheet
- 6 (item in the to-do checklist): Set personal goals
- 7 (item in the to-do checklist): Complete the depression and anxiety test
- 8 (item in the to-do checklist): Watch the getting started video
- 9: Review another member’s profile
- 10: Post in the community
- 11: Read other member’s goals
- 12: Give a community member a “thumbs up”
- 13: Encourage a community member by clicking their “show support” icon
- 14: Private message a community moderator
Participants
As there were no barriers to registration and many new members registered with the platform for purposes other than treatment, we removed those who registered but did not return to the intervention (nonparticipants) from the analysis.
Data Analyses
All data were analyzed using mixed effect logistic regression with members as a random variable. Mixed effect logistic regression was conducted using the glmer() function from the lme4 package in R software (R Foundation for Statistical Computing), with the default optimizer Bound Optimization BY Quadratic Approximation, a derivative-free optimization algorithm used for problems with bound constraints.
For the tip-of-the-day section, the outcome variable was whether a user clicked on a tip presented to them (no or yes), and the predictors were arm (2 or 3), gender (female or male), and age group (18 to 30 years to >60 years).
For the to-do checklist items, the outcome variable was whether a user clicked on a checklist item (no or yes), and the predictors were gender and age group.
For the course components, the outcome variable was whether a user completed a component (no or yes), and the predictors were arm (1, 2, or 3), gender, and age group.
Between November 2021 and May 2022, data were collected from new members who self-registered for Evolution Health’s self-guided treatment program for anxiety and depression. All members were randomized into 1 of the 3 arms.
First, members with test accounts and unauthenticated accounts were removed from the data set, resulting in a population of 13,224 members. Then, members whose accounts were missing demographic data (376/13,224, 2.84%) were removed from the data set, followed by members aged <18 (129/13,224, 0.97%), resulting in a population of 12,719 members.
Finally, of the 13,224 members, 8567 (65.46%) with nonparticipant accounts were removed, resulting in a study population of 4567 (34.53%). Of the 4567 members, 1788 (39.15%) were randomized into arm 1, 865 (18.94%) into arm 2, and 1914 (41.9%) into arm 3 ( Figure 8 ).

Demographic Characteristics
The average age of the members in arms 1, 2, and 3 was 44.4 (SD 10.69), 45.4 (SD 10.62), and 41.8 (SD 11.17), respectively ( Table 4 ). The 51 to 60 years age group was the most populous age category in each of the arms (arm 1: 777/1788, 43.45%; arm 2: 420/865, 48.6%; and arm 3: 586/1914, 30.61%). The members of arm 3 were somewhat younger ( F 2,4564 =40.97; P <.001) than the other 2 arms.
Regarding gender ( Table 5 ), most members identified as women (arm 1: 1466/1788, 81.99%; arm 2: 751/865, 86.8%; and arm 3: 1770/1914, 92.48%). Few members identified as transgender individuals, nonbinary or third gender individuals, or preferred not to say (arm 1: 5/1788, 0.28%; arm 2: 1/865, 1.2%; and arm 3: 2/1914, 0.1%). Arm 3 had more female members ( χ 2 4 =92.21; P <.001) than the other 2 arms.
During the study period, there were 13,510 total log-ins, with initial registration considered as 1 log-in ( Table 6 ). The average number of log-ins for male members was 3.89 and female members was 2.83. The average visit duration (AVD) for members who engaged with depression and anxiety course tools ranged from 6 minutes and 45 seconds, with 7.65 pages viewed, to 24 minutes and 21 seconds, with 12.56 pages viewed.
Tip of the Day
In arm 2, there were 31 revolving tips, and in arm 3, there were 30 revolving tips. Therefore, the probability of a member assigned to the arm 2 seeing a specific tip at least once is .088, and the probability of a member assigned to the arm 3 seeing a specific tip at least once is .091.
A total of 11,431 tips were displayed, of which 564 (4.93%) were clicked on ( Table 7 ). In arm 2, 3622 tips were shown, of which 190 (5.24%) were clicked on. In arm 3, 7809 tips were shown, of which 374 (4.79%) were clicked on. Mixed effect logistic regression revealed no statistically significant differences between the 2 arms regarding the number of tips clicked on ( P =.25).
In arm 2, female members clicked on 171 (5.82%) tips out of the 2937 tips shown to them and male members clicked on 19 (2.8%) tips out of the 682 tips shown to them, whereas in arm 3, females members clicked on 350 (4.94%) out of the 7081 tips shown to them and male members clicked on 24 (3.3%) out of the 722 tips shown to them ( Table 8 ).
There were no statistically significant differences between number of tips clicked on between age groups ( Table 9 ).
Mixed effect logistic regression ( Table 10 ) revealed that female members clicked on more tips than male members ( z score=2.58; P =.01). Although the role of gender and engagement should be more thoroughly examined in future studies, this finding is consistent with other research on platform components [ 60 ].
There were no substantial differences between age groups.
a OR: odds ratio.
To-Do Checklist
As outlined in Textbox 1 and illustrated in Figure 5 , the to-do checklist contained 8 items. The members of arm 3 completed an average of 2.7 (34%) out of 8 course components.
The checklist item with the highest engagement was complete the depression and anxiety test , which was completed by 51.4% (55/107 of the members; Table 11 ). The second most popular item was watch the getting started video , with 48.2% (923/1914) of the members engaging with the behavioral cue. The checklist item with lowest engagement was upload my image with nearly one-fifth (360/1914, 18.8%) of the members engaging with the behavioral cue.
It should be noted that 2 items read a community post and complete the depression and anxiety test were hidden for some members. This is due to these elements being feature flags and some Evolution Health clients and research partners choosing to hide these features from their membership base.
Although this resulted in a lower number of members seeing these cues and having access to the course components, the percentage of members who viewed the cues and engaged with the components was noteworthy.
Table 12 lists the engagement results for checklist use and gender. Mixed effect logistic regression found that female members clicked on more checklist items than male members ( z score=2.07; P =.04). Although the role of gender and engagement should be more thoroughly examined in future studies, this finding is consistent with other research on platform components [ 60 ].
Tables 13 and 14 list the engagement with checklist use and age. Members aged 41 to 50 years and 51 to 60 years were significantly less likely to click on a to-do checklist item ( z score=2.1; P <.04) than members aged 18 to 30 years ( z score=4.35; P <.001). No other differences were found between the age groups.
Comparison of Course Components Completed by Arm
Tables 15 and 16 outline course component completion rates by arm. Using the set goals example, 17% (304/1788) of the members in the control arm, 19.8% (171/865) in arm 2, and 31.35% (600/1914) in arm 3 completed the goals exercise.
a RC: randomized control.
b PB: present bias.
c SP: social proof.
d TD: to-do checklist.
e CBT: cognitive behavioral therapy.
f N/A: not applicable.
The course component with the highest completion rate was complete the depression and anxiety test, with 51.4% (60/117) in arm 3, 47% (17/36) in arm 2, and 24.1% (47/195) in arm 1. The course component with the lowest completion rate was upload my image, with 18.65% (357/1914) in arm 3, 1.9% (16/865) in arm 2, and 0.73% (13/1788) in arm 1.
As mentioned previously, the 2 items read a community post and complete the depression and anxiety test were not seen by all members. Some Evolution Health clients do not offer these 2 tools to their membership base. Consequently, there are high engagement percentages but low member use.
For technical reasons, the completion of 2 components review a worksheet and watch the getting started video were not captured in arm 1. However, about one-third of the members in arm (606/1914, 31.66%) reviewed a worksheet compared to about 2% of those (21/865, 2.4%) in arm 2, and almost half (923/1914, 48.22%) of the members in arm 3 watched the getting started video compared to about 3% of those (24/865, 2.8%) in arm 2.
Engagement with 6 of the 8 measurable components was higher in arm 2 than in arm 1 ( z score=3.59; P <.001) and higher in arm 3 than in arm 1 ( z score=10.91; P <.001). Engagement in 8 of the 8 components was higher in arm 3 than in arm 2 ( z score=4.93; P <.001).
Overall, the use of the tips and to-do checklist items resulted in increased engagement. Members in the control arm completed an average of 1.52 course components versus 1.8 course components in arm 2 and 2.11 course components in arm 3.
CBT Course Completion Rates
The purpose of CBT is to help individuals deal with overwhelming problems; this is achieved by teaching people how to deal with negative thoughts and beliefs [ 61 ]. In traditional in-person therapy, the duration of CBT treatment depends on a variety of factors, including health disorders (eg, depression, anxiety, posttraumatic stress disorder, and sleep disorders), symptom severity, duration of therapy, practitioner availability, cost, willingness of the patient to do homework, convenience, culture, treatment settings, and the therapeutic alliance [ 62 ].
The recommended duration of CBT varies. Harvard Medical School recommends 30- to 60-minute sessions over 12 to 20 weeks [ 63 ], the United Kingdom’s National Health Services recommends 30- to 60-minute sessions once a week or every 2 weeks for 6 to 20 sessions [ 64 ], and the Mayo Clinic states that therapeutic encounters can range from 5 to 20 sessions [ 65 ].
According to the US National Institutes of Health’s National Library of Medicine, some people undergoing CBT feel much better after a few sessions, whereas others need treatment for several months [ 66 ]. In Canada, the Centre for Addiction and Mental Health notes that some people improve in 4 to 6 sessions, whereas others may need >20 sessions [ 67 ].
Structured CBT sessions are 1 of the 8 course components in the Evolution Health platform, and like the 7 other course components examined in this study, members’ engagement is optional. The courses Overcoming Depression and Overcoming Anxiety both comprised 9 sessions. On average, 41% (1851/4567) of the members chose to engage with and complete session 1 ( Table 15 ).
If members completed a course, they received a course completion certificate ( Figure 9 ).
Unlike the platform’s addiction courses, where some members are incentivized by customer sponsors to complete a course (eg, some human resource clients reward their employees for completing the quit smoking behavior change course), to the best of our knowledge, participants in the Overcoming Depression and Overcoming Anxiety courses were not rewarded for course completion or receiving a certificate.
Although the goal of CBT is to decrease symptoms and the duration of treatment varies widely per individual, course completion may be a poor indicator of wellness. However, as engagement is the primary measure of this study and the literature indicates that higher levels of engagement are associated with improved health outcomes, it may be beneficial to form a baseline observation for course completion rates.
Regarding course completion, 31 members in the Overcoming Depression course and 88 members in the Overcoming Anxiety course received course completion certificates. There were no statistically significant differences in the course completion rates between the 3 arms.
In addition, 256 of 4567 members of the N members received a certificate for completing a supplementary 1-session more help course, which was less intense and dealt with specific subjects such as overcoming grief, problems in relationships, or role transitions. A greater proportion of members in arm 2 received more certificates for the more help course than did the members in arm 1 ( P =.02). There were no differences of interaction between gender or age groups.

This 3-arm randomized controlled trial tested whether behavioral nudges and prompts could be successfully applied within 2 self-guided treatment digital health programs for anxiety and depression.
The average age of the members was 43.8 years, and 87.3% (3987/4567) identified as female members. The average number of log-ins for female members was 2.83 versus 3.89 for male members. The average number of log-ins should be interpreted with caution, as in other studies on the Evolution Health population; engagement appears to follow the properties of power laws [ 53 ].
H1 Summary: Engagement With Behavioral Nudges and Prompts
Our H1 was to analyze whether the members would engage with the tips and to-do checklist.
Regarding gender, mixed effect logistic regression found that female members clicked on more tips than male members ( z score=2.58; P =.01).
Members in arm 3 completed an average of 2.7 (34%) out of the 8 course components featured on the to-do checklist. Engagement ranged from 18.81% (360/1914; upload my image ) to 51.4% (55/107; complete the depression and anxiety test ).
Mixed effect logistic regression found that female members clicked on more checklist items than male members ( z score=2.07; P =.04). Members aged 41 to 50 years and 51 to 60 years were significantly less likely to click on a to-do checklist item ( z score=2.1; P =.04) than members aged 18 to 30 years ( z score=4.35; P <.001). No other differences were found between the age groups.
Secondary Hypothesis Summary: Members’ Preference of Directive Tips in Arm 2 or Social Proof or Present Bias Tips in Arm 3
There were no statistically significant differences in the number of tips clicked on between directive tips in arm 2 and social proof and present bias tips in arm 3 ( P =.25).
Tertiary Hypothesis Summary: Tips and to-Do Checklist
Completion rates of 6 out of the 8 course components increased in both experimental arms from baseline use in arm 1. Completion rates in arm 2 were greater than those in arm 1 ( z score=3.59; P <.001), and completion rates in arm 3 were greater than those in arm 1 ( z score=10.91; P <.001).
A total of 2 out of the 8 course tools ( review a worksheet and watch the getting started video ) did not have arm 1 baseline statistics. However, completion rates for review a worksheet in arm 2 versus arm 3 increased from 2.4% to 31.66%. The completion rate for watch the getting started video increased from 2.8% to 48.22%.
Completion rates for the course component complete CBT session 1 were slightly higher in arm 2 (376/865, 43.5%) than in arm 3 (806/1914, 42.11%). Completion rates of arm 2 were greater than those in arm 1 ( z score=2.99; P =.003), and completion rates in arm 3 were greater than those in arm 1 ( z score=2.91; P =.004). Completion rates of the both arms 2 and 3 were significantly higher than those in arm 1 (669/1788, 37.42%).
The highest increase in course component use was for the upload my image component, with 0.73% (13/1788) of the members uploading an image in arm 1, 1.9% (16/865) in arm 2, and 18.65% (357/1914) in arm 3. This is interesting as personalization in digital applications is now common; however, the Evolution Health platform is designed to be anonymous, and before uploading, members were advised to only upload nonidentifying images.
However, high z scores require further investigation. For example, high scores may be due to arm 3 members being more likely to complete components than arm 1 members.
In the literature, efficacy studies analyzing general CBT completion rates are rare, and this is due to complexities surrounding the delivery of CBT for different indications, symptom severity, duration of therapy, practitioner availability, cost, willingness of the patient to do homework, convenience, culture, treatment settings, and therapeutic alliance.
Owing to the nature of the medium, measuring course completion rates in digital health courses is relatively simple. However, digital health courses should not be held to different standards than traditional in-person therapy, where treatment success is often measured by the therapist by observing decreases in symptoms.
In 2005, Eysenbach [ 11 ] published The Law of Attrition , which recognized that a substantial portion of users drop out of eHealth (digital health) trials before completion and that the high dropout rate makes the efficacy of digital health programs less believable. The paper noted that researchers often compare digital health dropout rates with those of clinical drug trials.
Similar to traditional CBT, where treatment can run from a few weekly sessions to >20 sessions, the digital health literature has shown dose-response effects. Users of self-guided digital health courses interact with devices, not trained therapists. Until digital health platforms can unobtrusively and accurately detect symptom severity, measuring course completion rates will continue to be an inaccurate measure of efficacy.
As higher levels of engagement are associated with improved health outcomes [ 20 , 21 ] and due to the complex methodological issues associated with establishing efficacy rates in population-based digital health CBT programs with high reach, research should continue to focus on strategies that increase adherence and engagement [ 8 - 10 , 12 ].
Practical Implications
The arm 3 home page is presently the default setting for the home pages for the Overcoming Anxiety and Overcoming Depression courses. We expect to see increases in course engagement based on the use of behavioral nudges and prompts. Ongoing data collection from all members will contribute to future research.
Strengths and Limitations
A strength of this study is that it was conducted in an ad libitum environment. Unlike many digital health studies, a large study population in the thousands was leveraged rather than smaller groups. As participants were not aware of participating in the experiment, this limited participant bias and the Hawthorne effect.
A limitation is that, especially due to the anonymity of members, we have no way of identifying participants or validating their demographic information. Although we have no way of knowing whether the registrants are people with depression or anxiety who are seeking help, the removal of nonparticipants should mitigate the effects on the overall results.
Some Evolution Health clients promote certain course tools, or health care professionals may direct their clients to use certain platform attributes. Therefore, the tips or to-do checklist items may not be a factor in their engagement. Second, many members may simply ignore the behavioral cues and complete certain course tools based on their own preference.
The behavioral cues related to read a community post and complete the depression and anxiety test were not seen by all members. This is due to these elements being feature flags and some Evolution Health clients choosing not to offer these tools to their membership base. Although this resulted in fewer members seeing these cues and having access to the course components, the percentage of members who viewed the cues and engaged with the components was noteworthy.
As this study was designed to form a baseline for future research, there are some methodological issues that can be explored further. For example, unlike the tips presented in arm 2, the use of tips in arm 3 may or may not have been influenced by adding the to-do checklist. Alternatively, using directive tips in arm 3, rather than social proof and present bias tips, may increase the use of the to-do checklist.
Notably, our evidence indicates that nudges and prompts increase engagement in self-guided treatment programs for depression and anxiety. On the basis of these encouraging yet preliminary results, we can proceed with more sophisticated studies that will examine more enhanced strategies designed to increase platform engagement.
Future Directions
As mentioned earlier, there has been scant published research regarding the implementation of behavioral economic strategies designed to increase engagement in digital health programs. At minimum, this randomized controlled trial was successful in confirming member engagement through the use of these strategies.
As recommended by Aschbacher et al [ 68 ] in a recent study involving digital mental health and dose responses, machine learning models can help enable precision by analyzing engagement patterns over time. Combined with another recent paper by Forbes et al [ 24 ] analyzing digital interventions for depression, which noted that it is important to develop standardized ways of reporting adherence and engagement so that effective comparisons across different interventions could be measured, baseline outcomes need to be established.
The baseline outcomes of this study can serve as guideposts for future studies. With machine learning models in place and with the goal of improving member outcomes and platform efficacy, Evolution Health considers the following research questions:
- Will members of addiction-focused courses (eg, managing drinking and quitting smoking) follow similar engagement patterns if nudges and prompts are made available to them? Which nudges and prompts can be used universally, and which work best for specific mental health or addiction indications?
- The average member logged into the platform 2.38 times. Can specific tips be introduced at onset to promote log-ins?
- There were no statistically significant differences in the number of tips clicked on between directive tips in arm 2 and social proof and present bias tips in arm 3 ( P =.25). However, arm 2 featured 31 rotating directive tips and arm 3 featured 15 social proof tips and 15 present bias tips. Which tips were most engaging (eg, directive, social proof, or present bias tips)? Which content areas were most engaging (eg, goal setting, community themed, or specific exercises such as the depression and anxiety tests)? Which tips were the most engaging genders or age groups?
- The average arm 3 member clicked on 2.7 out of 8 to-do checklist items. Which items were these members most likely to click on first? Were there specific patterns of engagement that may influence which tips should be shown to specific members?
- The course components differ in the effort required to complete them. For example, completing a CBT session is more intensive than the few minutes required to complete the depression and anxiety test. Furthermore, one member may take several minutes to contemplate their goals, whereas others may have concrete goals already established. Future research should analyze duration in relation to each course component, as these data may be leveraged to create tailored tips or to-do content for specific user engagement patterns.
- The AVD for members who engaged with depression and anxiety course tools ranged from 6 minutes and 45 seconds, with 7.65 pages viewed, to 24 minutes and 21 seconds, with 12.56 pages viewed. In the future, AVD may be used as a benchmark, as overall engagement may be an important metric to calculate when observing dose-response relationships.
- Members arrived at the platform through the free-to-consumer program and white-label instances licensed by clients that range from employers, insurance companies, employee assistance programs, educational institutions, nonprofit organizations, for-profit health care organizations, and individual therapists. Measuring AVD from these referral sources and patterns of course tool use may assist in creating targeted engagement recommendations.
- The depression and anxiety test has been validated in a separate study, and the algorithm reports whether members qualify for 30 separate mood and anxiety disorders [ 48 ]. Symptoms related to these disorders were also collected and reported to the members. Future research should analyze these data to investigate the possible relationships between symptomology, symptom severity, and engagement patterns.
- A recent paper by Forbes et al [ 24 ] analyzing patient adherence with digital interventions for depression noted that it is important to standardize reporting adherence and engagement. Our future work will focus on establishing baseline metrics. For example, an acceptable click rate for a tip is x% or an acceptable click rate for a to-do checklist item is y%.
- The platform contains courses for addictive behaviors (eg, smoking cessation and problem drinking), in which members who complete these courses receive a certificate of completion. We are aware that some workforce members received incentives from their human resources department for completing courses and receiving certificates. It may be worthwhile to compare the course completion rates between incentivized addictive behavior courses and nonincentivized mood disorder courses.
- This experiment examined the use of behavioral prompts. Future studies may examine overcoming behavioral barriers such as bounded rationality or choice architecture.
It cannot be assumed that the outcomes observed on the Evolution Health platform will be replicated in other environments. Further research in the combined fields of digital health and behavioral economics is required.
Conclusions
Members of the Evolution Health platform’s self-guided digital health courses engaged with behavioral nudges and prompts. From this preliminary analysis, it appears that both the tips and to-do checklists increased engagement in course components.
To the best of our knowledge, this is the first randomized controlled trial designed to test the implementation of behavioral nudges and prompts in web-based self-guided courses for mood disorders. The results of this study may be important because efficacy is related to increased engagement.
Owing to its novel approach, the outcomes of this study should be interpreted with caution but may be used as a guideline for future research.
Data Availability
The data sets generated during and analyzed during this study are not publicly available due to Evolution Health’s data privacy policy, terms of use, and user agreement but are available from the corresponding author on reasonable request.
Research emerging from this and ongoing data collection will be leveraged to train artificial intelligence models to better understand how to increase and encourage healthy behavior changes. Making these data publicly available may have the opposite effect and enable the development of models that can detect and target susceptible populations.
The rich data set from this study, ongoing engagement data that are continually collected, or other platform data sets can be made available to researchers interested in conducting studies for noncommercial purposes. Interested researchers are encouraged to contact Evolution Health.
Conflicts of Interest
TVM is the chief executive officer and founder of Evolution Health, the owner of the Evolution Health digital health care platform. RF is an Evolution Health board member. No funds were received for conducting this study.
Privacy policy and terms of use.
CONSORT-eHEALTH checklist (V 1.6.1).
- DeChant HK, Tohme WG, Mun SK, Hayes WS, Schulman KA. Health systems evaluation of telemedicine: a staged approach. Telemed J. 1996;2(4):303-312. [ CrossRef ] [ Medline ]
- Picot J, Cradduck T. The telehealth industry in Canada: industry profile and capability analysis. The Keston Group and Infotelmed Communications. Mar 2000. URL: https://citeseerx.ist.psu.edu/document?repid=rep1&type=pdf&doi=c7f26186673239a22d731d13857780ba0a5c7918 [accessed 2024-02-26]
- Schneider SJ, Walter R, O'Donnell R. Computerized communication as a medium for behavioral smoking cessation treatment: controlled evaluation. Comput Hum Behav. 1990;6(2):141-151. [ CrossRef ]
- Barak A, Hen L, Boniel-Nissim M, Shapira N. A comprehensive review and a meta-analysis of the effectiveness of internet-based psychotherapeutic interventions. J Technol Hum Serv. 2008;26(2-4):109-160. [ CrossRef ]
- Krämer R, Köhne-Volland L, Schumacher A, Köhler S. Efficacy of a web-based intervention for depressive disorders: three-arm randomized controlled trial comparing guided and unguided self-help with waitlist control. JMIR Form Res. Apr 04, 2022;6(4):e34330. [ FREE Full text ] [ CrossRef ] [ Medline ]
- Mohr DC, Kwasny MJ, Meyerhoff J, Graham AK, Lattie EG. The effect of depression and anxiety symptom severity on clinical outcomes and app use in digital mental health treatments: meta-regression of three trials. Behav Res Ther. Dec 2021;147:103972. [ FREE Full text ] [ CrossRef ] [ Medline ]
- Mamukashvili-Delau M, Koburger N, Dietrich S, Rummel-Kluge C. Long-term efficacy of internet-based cognitive behavioral therapy self-help programs for adults with depression: systematic review and meta-analysis of randomized controlled trials. JMIR Ment Health. Aug 22, 2023;10:e46925. [ FREE Full text ] [ CrossRef ] [ Medline ]
- Christensen H, Griffiths KM, Korten AE, Brittliffe K, Groves C. A comparison of changes in anxiety and depression symptoms of spontaneous users and trial participants of a cognitive behavior therapy website. J Med Internet Res. Dec 22, 2004;6(4):e46. [ FREE Full text ] [ CrossRef ] [ Medline ]
- Farvolden P, Denisoff E, Selby P, Bagby RM, Rudy L. Usage and longitudinal effectiveness of a web-based self-help cognitive behavioral therapy program for panic disorder. J Med Internet Res. Mar 26, 2005;7(1):e7. [ FREE Full text ] [ CrossRef ] [ Medline ]
- Wu RC, Delgado D, Costigan J, Maciver J, Ross H. Pilot study of an internet patient-physician communication tool for heart failure disease management. J Med Internet Res. Mar 26, 2005;7(1):e8. [ FREE Full text ] [ CrossRef ] [ Medline ]
- Eysenbach G. The law of attrition. J Med Internet Res. Mar 31, 2005;7(1):e11. [ FREE Full text ] [ CrossRef ] [ Medline ]
- Christensen H, Griffiths KM, Farrer L. Adherence in internet interventions for anxiety and depression. J Med Internet Res. Apr 24, 2009;11(2):e13. [ FREE Full text ] [ CrossRef ] [ Medline ]
- Moshe I, Terhorst Y, Philippi P, Domhardt M, Cuijpers P, Cristea I, et al. Digital interventions for the treatment of depression: a meta-analytic review. Psychol Bull. Aug 2021;147(8):749-786. [ CrossRef ] [ Medline ]
- Claxton AJ, Cramer J, Pierce C. A systematic review of the associations between dose regimens and medication compliance. Clin Ther. Aug 2001;23(8):1296-1310. [ CrossRef ] [ Medline ]
- DiMatteo MR. Variations in patients' adherence to medical recommendations: a quantitative review of 50 years of research. Med Care. Mar 2004;42(3):200-209. [ CrossRef ] [ Medline ]
- Krueger KP, Berger BA, Felkey B. Medication adherence and persistence: a comprehensive review. Adv Ther. Jul 2005;22:313-356. [ CrossRef ]
- van Mierlo T, Fournier R, Ingham M. Targeting medication non-adherence behavior in selected autoimmune diseases: a systematic approach to digital health program development. PLoS One. Jun 24, 2015;10(6):e0129364. [ FREE Full text ] [ CrossRef ] [ Medline ]
- Viswanathan M, Golin CE, Jones CD, Ashok M, Blalock SJ, Wines RC, et al. Interventions to improve adherence to self-administered medications for chronic diseases in the United States: a systematic review. Ann Intern Med. Dec 04, 2012;157(11):785-795. [ FREE Full text ] [ CrossRef ] [ Medline ]
- Zivin K, Kales HC. Adherence to depression treatment in older adults: a narrative review. Drugs Aging. 2008;25(7):559-571. [ CrossRef ] [ Medline ]
- Enrique A, Palacios JE, Ryan H, Richards D. Exploring the relationship between usage and outcomes of an internet-based intervention for individuals with depressive symptoms: secondary analysis of data from a randomized controlled trial. J Med Internet Res. Aug 01, 2019;21(8):e12775. [ FREE Full text ] [ CrossRef ] [ Medline ]
- McVay MA, Bennett GG, Steinberg D, Voils CI. Dose-response research in digital health interventions: concepts, considerations, and challenges. Health Psychol. Dec 2019;38(12):1168-1174. [ FREE Full text ] [ CrossRef ] [ Medline ]
- Alharbi F. The use of digital healthcare platforms during the COVID-19 pandemic: the consumer perspective. Acta Inform Med. Mar 2021;29(1):51-58. [ FREE Full text ] [ CrossRef ] [ Medline ]
- Twenge JM, Joiner TE. Mental distress among U.S. adults during the COVID-19 pandemic. J Clin Psychol. Dec 2020;76(12):2170-2182. [ FREE Full text ] [ CrossRef ] [ Medline ]
- Forbes A, Keleher MR, Venditto M, DiBiasi F. Assessing patient adherence to and engagement with digital interventions for depression in clinical trials: systematic literature review. J Med Internet Res. Aug 11, 2023;25:e43727. [ FREE Full text ] [ CrossRef ] [ Medline ]
- Bond RR, Mulvenna MD, Potts C, O’Neill S, Ennis E, Torous J. Digital transformation of mental health services. Npj Ment Health Res. Aug 22, 2023;2:13. [ CrossRef ]
- Kahneman D. Thinking, Fast & Slow. London, UK. Penguin Books; 2015.
- Thaler R. In: Thaler RH, Sunstein CR, editors. Nudge : Improving Decisions About Health. London, UK. Penguin Books; 2009.
- van Mierlo T, Hyatt D, Ching AT, Fournier R, Dembo RS. Behavioral economics, wearable devices, and cooperative games: results from a population-based intervention to increase physical activity. JMIR Serious Games. Jan 28, 2016;4(1):e1. [ FREE Full text ] [ CrossRef ] [ Medline ]
- Six SG, Byrne KA, Tibbett TP, Pericot-Valverde I. Examining the effectiveness of gamification in mental health apps for depression: systematic review and meta-analysis. JMIR Ment Health. Nov 29, 2021;8(11):e32199. [ FREE Full text ] [ CrossRef ] [ Medline ]
- Zhang M, Ying J, Ho RC. Gamified cognitive bias interventions for psychiatric disorders: protocol of a systematic review. JMIR Res Protoc. Oct 16, 2018;7(10):e10154. [ FREE Full text ] [ CrossRef ] [ Medline ]
- Susi T, Johannesson M, Backlund P. Serious games: an overview. School of Humanities and Informatics, University of Skövde. Feb 5, 2007. URL: https://www.diva-portal.org/smash/get/diva2:2416/fulltext01.pdf [accessed 2024-02-26]
- Tan JW, Zary N. Diagnostic markers of user experience, play, and learning for digital serious games: a conceptual framework study. JMIR Serious Games. Jul 16, 2019;7(3):e14620. [ FREE Full text ] [ CrossRef ] [ Medline ]
- Black M, Brunet J. A wearable activity tracker intervention with and without weekly behavioral support emails to promote physical activity among women who are overweight or obese: randomized controlled trial. JMIR Mhealth Uhealth. Dec 16, 2021;9(12):e28128. [ FREE Full text ] [ CrossRef ] [ Medline ]
- What is positive behavior support? The Association for Positive Behavior Support. URL: https://www.apbs.org/pbs [accessed 2024-02-26]
- Forberger S, Reisch L, Kampfmann T, Zeeb H. Nudging to move: a scoping review of the use of choice architecture interventions to promote physical activity in the general population. Int J Behav Nutr Phys Act. Sep 03, 2019;16(1):77. [ FREE Full text ] [ CrossRef ] [ Medline ]
- Shah N, Adusumalli S. Nudges and the meaningful adoption of digital health. Per Med. Nov 2020;17(6):429-433. [ CrossRef ] [ Medline ]
- Milkman K, Patel MS, Gandhi L, Graci HN, Gromet DM, Ho H, et al. A megastudy of text-based nudges encouraging patients to get vaccinated at an upcoming doctor's appointment. Proc Natl Acad Sci U S A. May 18, 2021;118(20):e2101165118. [ FREE Full text ] [ CrossRef ] [ Medline ]
- Laibson D. Life-cycle consumption and hyperbolic discount functions. Eur Econ Rev. May 31, 1998;42(3-5):861-871. [ CrossRef ]
- O'Donoghue T, Rabin M. Present bias: lessons learned and to be learned. Am Econ Rev. May 2015;105(5):273-279. [ CrossRef ]
- Burnkrant RE, Cousineau A. Informational and normative social influence in buyer behavior. J Consum Res. Dec 01, 1975;2(3):206-215. [ CrossRef ]
- van Teunenbroek C, Bekkers R, Beersma B. Look to others before you leap: a systematic literature review of social information effects on donation amounts. Nonprofit Volunt Sect Q. 2020;49(1):53-73. [ CrossRef ]
- Xu Y, Chen N, Fernandez A, Sinno O, Bhasin A. From infrastructure to culture: A/B testing challenges in large scale social networks. In: Proceedings of the 21th ACM SIGKDD International Conference on Knowledge Discovery and Data Mining. 2015. Presented at: KDD '15; August 10-13, 2015; Sydney, Australia. [ CrossRef ]
- O'Brien HL, Toms EG. What is user engagement? A conceptual framework for defining user engagement with technology. J Am Soc Inf Sci Technol. Feb 28, 2008;59(6):938-955. [ CrossRef ]
- Rondina R, van Mierlo T, Fournier R. Testing behavioral nudges and prompts in digital courses for the self-guided treatment of depression and anxiety: protocol for a 3-arm randomized controlled trial. JMIR Res Protoc. Aug 15, 2022;11(8):e37231. [ FREE Full text ] [ CrossRef ] [ Medline ]
- Cunningham JA, van Mierlo T, Fournier R. An online support group for problem drinkers: AlcoholHelpCenter.net. Patient Educ Couns. Feb 2008;70(2):193-198. [ CrossRef ] [ Medline ]
- Davis J. The Panic Center. Child Adolesc Ment Health. Feb 2007;12(1):49-50. [ CrossRef ] [ Medline ]
- Farvolden P, Cunningham J, Selby P. Using e-health programs to overcome barriers to the effective treatment of mental health and addiction problems. J Technol Hum Serv. 2009;27(1):5-22. [ CrossRef ]
- Farvolden P, McBride C, Bagby RM, Ravitz P. A web-based screening instrument for depression and anxiety disorders in primary care. J Med Internet Res. 2003;5(3):e23. [ FREE Full text ] [ CrossRef ] [ Medline ]
- Mulvaney-Day N, Marshall T, Downey Piscopo K, Korsen N, Lynch S, Karnell LH, et al. Screening for behavioral health conditions in primary care settings: a systematic review of the literature. J Gen Intern Med. Mar 2018;33(3):335-346. [ FREE Full text ] [ CrossRef ] [ Medline ]
- Selby P, Farvolden P, Van Mierlo T. Demographic characteristics, cessation history and stages of change status among users of a web-based smoking cessation program. In: Proceedings of the National Conference on Tobacco or Health. 2002. Presented at: NCTOH 2002; November 19-21, 2002; San Francisco, CA.
- Selby P, van Mierlo T, Voci SC, Parent D, Cunningham JA. Online social and professional support for smokers trying to quit: an exploration of first time posts from 2562 members. J Med Internet Res. Aug 18, 2010;12(3):e34. [ FREE Full text ] [ CrossRef ] [ Medline ]
- Urbanoski K, van Mierlo T, Cunningham J. Investigating patterns of participation in an online support group for problem drinking: a social network analysis. Int J Behav Med. Oct 2017;24(5):703-712. [ CrossRef ] [ Medline ]
- van Mierlo T, Hyatt D, Ching AT. Mapping power law distributions in digital health social networks: methods, interpretations, and practical implications. J Med Internet Res. Jun 25, 2015;17(6):e160. [ FREE Full text ] [ CrossRef ] [ Medline ]
- Directive 95/46/EC of the European parliament and of the council on the protection of individuals with regard to the processing of personal data and on the free movement of such data. European Union. 1995. URL: https://www.refworld.org/legal/reglegislation/eu/1995/en/13712 [accessed 2024-02-26]
- Personal Information Protection and Electronic Documents Act (S.C. 2000, c. 5). Government of Canada. 2000. URL: https://laws-lois.justice.gc.ca/eng/acts/p-8.6/ [accessed 2024-02-26]
- Summary of the HIPAA privacy rule. U.S. Department of Health and Human Services. URL: https://www.hhs.gov/hipaa/for-professionals/privacy/laws-regulations/index.html [accessed 2024-02-26]
- WMA declaration of Helsinki – ethical principles for medical research involving human subjects. World Medical Association. URL: https://www.wma.net/policies-post/wma-declaration-of-helsinki-ethical-principles-for-medical-research-involving-human-subjects/ [accessed 2024-02-26]
- The "C" in your RCT - and what it means for the current state of Digital Health. Evolution Health. Nov 30, 2023. URL: https://www.linkedin.com/pulse/c-your-rct-what-means-current-state-digital-health-jvsfc?trk=public_post_feed-article-content [accessed 2024-02-26]
- Eysenbach G, CONSORT-EHEALTH Group. CONSORT-EHEALTH: improving and standardizing evaluation reports of web-based and mobile health interventions. J Med Internet Res. Dec 31, 2011;13(4):e126. [ FREE Full text ] [ CrossRef ] [ Medline ]
- van Mierlo T, Li X, Hyatt D, Ching AT. Demographic and indication-specific characteristics have limited association with social network engagement: evidence from 24,954 members of four health care support groups. J Med Internet Res. Feb 17, 2017;19(2):e40. [ FREE Full text ] [ CrossRef ] [ Medline ]
- Serafini G, Costanza A, Aguglia A, Amerio A, Placenti V, Magnani L, et al. Overall goal of cognitive-behavioral therapy in major psychiatric disorders and suicidality: a narrative review. Med Clin North Am. Jan 2023;107(1):143-167. [ CrossRef ] [ Medline ]
- Fernandez E, Salem D, Swift JK, Ramtahal N. Meta-analysis of dropout from cognitive behavioral therapy: magnitude, timing, and moderators. J Consult Clin Psychol. Dec 2015;83(6):1108-1122. [ CrossRef ] [ Medline ]
- Youn SJ, Marques L. Intensive CBT: how fast can I get better? Harvard Health Publishing. Oct 23, 2018. URL: https://tinyurl.com/ckmb56ur [accessed 2024-02-26]
- Overview - Cognitive behavioural therapy (CBT). National Health Service. URL: https://tinyurl.com/msbfzt8h [accessed 2023-01-04]
- Cognitive behavioral therapy. Mayo Clinic. URL: https://www.mayoclinic.org/tests-procedures/cognitive-behavioral-therapy/about/pac-20384610 [accessed 2023-01-04]
- Cognitive behavioral therapy. National Institutes of Health National Library of Medicine. URL: https://www.ncbi.nlm.nih.gov/books/NBK279297/ [accessed 2024-02-26]
- Cognitive behavioual therapy. Centre for Addiction and Mental Health. URL: https://www.camh.ca/en/health-info/mental-illness-and-addiction-index/cognitive-behavioural-therapy [accessed 2024-01-04]
- Aschbacher K, Rivera LM, Hornstein S, Nelson BW, Forman-Hoffman VL, Peiper NC. Longitudinal patterns of engagement and clinical outcomes: results from a therapist-supported digital mental health intervention. Psychosom Med. Sep 01, 2023;85(7):651-658. [ CrossRef ] [ Medline ]
Abbreviations
Edited by A Mavragani; submitted 07.09.23; peer-reviewed by JC Buckey, ES Silverman, L Callender; comments to author 21.12.23; revised version received 04.01.24; accepted 13.02.24; published 09.04.24.
©Trevor van Mierlo, Renante Rondina, Rachel Fournier. Originally published in JMIR Formative Research (https://formative.jmir.org), 09.04.2024.
This is an open-access article distributed under the terms of the Creative Commons Attribution License (https://creativecommons.org/licenses/by/4.0/), which permits unrestricted use, distribution, and reproduction in any medium, provided the original work, first published in JMIR Formative Research, is properly cited. The complete bibliographic information, a link to the original publication on https://formative.jmir.org, as well as this copyright and license information must be included.

An official website of the United States government
The .gov means it’s official. Federal government websites often end in .gov or .mil. Before sharing sensitive information, make sure you’re on a federal government site.
The site is secure. The https:// ensures that you are connecting to the official website and that any information you provide is encrypted and transmitted securely.
- Publications
- Account settings
Preview improvements coming to the PMC website in October 2024. Learn More or Try it out now .
- Advanced Search
- Journal List
- Indian J Psychiatry
- v.52(Suppl1); 2010 Jan
An overview of Indian research in depression
Sandeep grover.
Department of Psychiatry, Postgraduate Institute of Medical Education and Research, Chandigarh, India
Alakananda Dutt
Ajit avasthi.
Depression as a disorder has always been a focus of attention of researchers in India. Over the last 50-60 years, large number of studies has been published from India addressing various aspects of this commonly prevalent disorder. The various aspects studied included epidemiology, demographic and psychosocial risk factor, neurobiology, symptomatology, comorbidity, assessment and diagnosis, impact of depression, treatment related issues and prevention of depression in addition to the efficacy and tolerability of various antidepressants. Here, we review data on various aspects of depression, originating from India.
INTRODUCTION
Depression is a disorder of major public health importance, in terms of its prevalence and the suffering, dysfunction, morbidity, and economic burden. Depression is more common in women than men. The report on Global Burden of Disease estimates the point prevalence of unipolar depressive episodes to be 1.9% for men and 3.2% for women, and the one-year prevalence has been estimated to be 5.8% for men and 9.5% for women. It is estimated that by the year 2020 if current trends for demographic and epidemiological transition continue, the burden of depression will increase to 5.7% of the total burden of disease and it would be the second leading cause of disability-adjusted life years (DALYs), second only to ischemic heart disease.[ 1 ] In view of the morbidity, depression as a disorder has always been a focus of attention of researchers in India. Various authors have tried to study its prevalence, nosological issues, psychosocial risk factors including life events, sympto matology in the cultural context, comorbidity, psychoneurobiology, treatment, outcome, prevention, disability and burden. Some of the studies have also tried to address various issues in children and elderly.
This review focuses on research done on various depressive disorders in India. For this, a thorough internet search was done using key words like depression, life events, prevalence, classification, cultural issues, outcome, prevention, disability and burden etc in various combinations. The various search engines like Pubmed, Google Scholar, Sciencedirect, Search Medica, Scopus, And Medknow etc were used. In addition thorough search of all the issues of Indian Journal of Psychiatry available online was done. Hand search of some of the missing issues was also attempted and this yielded a few more articles. Review articles which were felt to be not reflecting the Indian scenario to a large extent or not covering the available Indian data were excluded. Treatment issues (antidepressants) are reviewed separately by us in this compilation of annotations to be published. Data from animal studies and originating in the form of case reports and small case series, until felt necessary haven’t been included. The available data has been organized under the headings of epidemiology, demographic and psychosocial risk factors, neurobiology, symptomatology, comorbidity, assessment and diagnosis, impact of depression, treatment related issues and prevention of depression.
Epidemiology
Many studies have estimated the prevalence of depression in community samples and the prevalence rates have varied from 1.7 to 74 per thousand population.[ 2 , 3 ] Reddy and Chandrasekhar[ 2 ] carried out a metanalysis, which included 13 studies on epidemiology of psychiatric disorders which include 33572 subjects from the community and reported prevalence of depression to be 7.9 to 8.9 per thousand population and the prevalence rates were nearly twice in the urban areas.[ 2 ] The findings with regard to prevalence in urban population are in line with the findings of a survey done on the entire adult population of an industrial township, which showed that the prevalence rate for depression to be 19.4 per thousand.[ 4 ]
A recent large population-based study from South India, which screened more than 24,000 subjects in Chennai using Patient Health Questionnaire (PHQ)-12 reported overall prevalence of depression to be 15.1% after adjusting for age using the 2001 census data.[ 5 ] In another recent study, Nandi et al .[ 3 ] compared the prevalence of depression in the same catchment area after a period of 20 years (first in 1972 and then in 1992) and reported that the prevalence of depression increased from 49.93 cases per 1000 population to 73.97 cases per 1000 population.[ 3 ] Studies done in primary care clinics/center have estimated a prevalence rate of 21-40.45%.[ 6 – 9 ] Studies done in hospitals have shown that 5 to 26.7% of cases attending the psychiatric outpatient clinics have depression.[ 10 – 13 ]
Studies on the elderly population, either in the community, inpatient, outpatient and old age homes have shown that depression is the commonest mental illness in elderly subjects.[ 14 – 19 ] Nandi et al .[ 14 ] studied psychiatric morbidity of the elderly population of a rural community in West Bengal. In a sample of 183 subjects (male 85, female 98) they found 60% of the population to be mentally ill with higher morbidity in women compared to men (77.6% and 42.4% respectively). There was significantly more morbidity in population in the age group 70-74 and 80+ as compared to normal population. The total mental morbidity rate was as high as 612/1000 population. Depression was the commonest illness of old age in this sample, the rate being 522/1000 population (101 cases out of 112 were diagnosed as cases of depression). Women had a higher rate of depression-704/1000 population. Another significant finding was the high rate of morbidity amongst the widowed persons.
An epidemiological study from rural Uttar Pradesh showed that psychiatric morbidity in the geriatric group (43.32%) was higher than in the nongeriatric group (4.66%) and most common psychiatric morbidity was neurotic depression, followed by manic-depressive psychosis depression, and anxiety state. Psychiatric morbidity was more prevalent in those who were socially, economically, and educationally disadvantaged.[ 15 ] Recent community-based studies have reported a prevalence rate of 21.7% to 45.9%.[ 20 , 21 ]
Chhabra and Kar[ 16 ] studied the profile of psychiatric disorders in elderly psychiatric inpatients and reported that mood disorders were the most common diagnosis (46.5%). Older studies from Gero-psychiatric clinics reported a prevalence of depression ranging from 13 to 22.2%.[ 22 , 23 ] A recent outpatient study, which evaluated psychiatric morbidity in 100 randomly selected elderly subjects attending geriatric clinic, found that 29% patients suffered from psychiatric illness of which depressive disorders were the most common.[ 17 ] Another study also reported depression to be the most common psychiatric diagnosis among the 1586 elderly subjects (age ≥60 years), who attended the Geriatric Clinic of the All India Institute of Medical Sciences, New Delhi.[ 24 ] In a study of old age home population, Guha and Valdiya[ 18 ] reported that major depressive disorder (13.4%) was the most common psychiatric diagnosis in this population.
With regard to epidemiology of depression in children and adolescents in a community sample from south India, Srinath et al .[ 25 ] reported a prevalence of 0.1% in the 4-16 year age group and no child in the age group 0-3 was diagnosed to have depression. Another study from north India reported an annual incidence rate of 1.61/1000 children in a community based study on school children.[ 26 ] Clinic-based studies have reported a prevalence rate of 1.2 to 9.2% for the affective dis orders, amongst which unipolar depression was the commonest category in most of the studies.[ 27 – 32 ] However, in a recent study evaluating the trend of various diagnoses in clinic population, Malhotra et al .[ 33 ] reported increase in prevalence of affective disorders from 2% to 13.49% in children (0-14 years) attending the psychiatric outpatient clinics. Studies done in women during and after pregnancy have reported incidence of post-natal depression to be 11%.[ 34 ]
Demographic and psychosocial risk factors for depression
In terms of sociodemographic variables studies have shown that depression is more common in women,[ 4 , 5 , 35 – 37 ] younger subjects,[ 13 ] in subjects from poor economic background[ 5 , 36 , 38 ] and subjects with poor nutritional status,[ 38 ] Muslims,[ 35 ] those who are divorced or widowed,[ 5 ] those residing in nuclear families[ 39 ] and urban areas.[ 2 ] Studies which have evaluated the subjects with late onset or old age depression (first episode of depression at or after the age of 50) have also shown that depression is more common in low social class, widowed state,[ 14 , 37 , 40 ] unemployed condition, low educational level, in subjects living in nuclear family or in those living alone.[ 15 , 21 , 37 , 40 ] With regard to gender most of the studies have reported that it is more common in elderly females,[ 14 , 37 , 40 ] however, some clinic-based studies suggest that it is more common in elderly males.[ 41 ] It is also seen that prevalence of depression increases with increasing age in elderly.[ 20 ]
Studies have shown that compared to healthy controls and subjects with schizophrenia, depressed patients have significantly greater number of life events prior (6-12 months) to the onset of their illness.[ 42 – 46 ] In terms of type of life events, it is seen that depressed patients experience significantly higher proportion of life events related to death of a family member, personal health related events, bereavement, interpersonal and social events[ 34 , 42 , 44 , 47 ] and lower number of life events in the form of illness of family members compared to patients with schizophrenia.[ 44 ]
It is also seen that compared to patients with mild depression patients with moderate and severe depression tend to use avoidance as a coping strategies more frequently for the stressful life events, suggesting that it may be a maladaptive way to cope with the situation, which is responsible for development of depression.[ 43 ] Studies have also reported that parental loss before the age of 18 years, parental disharmony and eldest birth order tend to be more common in subjects with depression.[ 48 ]
Studies in elderly also suggest that life events, especially financial problems and death in the family are as important a precipitating event for depression as they are in young adult.[ 20 , 40 , 49 ] It is also seen that stressful life events were specifically more in the elderly females and those with lower per capita income.[ 50 ]
With respect to life events in children and adolescents, Patel et al . found that depressed adolescent girls report life events in the form of death of a family member, change in residence, failure in examination, end of a relationship and serious illness.[ 51 ] Other risk factors identified to be associated with depression in children include stress at school and family as well as family history of mental illness.[ 52 ] However, one of the older studies failed to find a link between childhood bereavement and depression.[ 53 ]
Women as a group have also received considerable attention with regard to risk factors for development of depressive disorders. In an incidence study of common mental disorders, Patel et al .[ 54 ] reported that poverty (low income and having difficulty in making ends meet), being married as compared with being single, use of tobacco, experiencing abnormal vaginal discharge and reporting a chronic physical illness were associated with risk of developing a common mental disorder.[ 54 ] Studies have also reported that economic and interpersonal relationship difficulties, partner violence, sexual coercion by the partner as the common causal factors related to development of depression in general and depression during antenatal and postnatal period.[ 34 , 55 – 58 ] It has been shown that gender of the newborn child is an important determinant of postnatal depression.[ 34 , 56 , 57 ]
Among the psychological factors, attribution style was proposed to predispose individuals to depression and maintain depressive symptoms once they develop. A study using the Attribution Style Questionnaire[ 59 ] showed that depressed patients have a specific attribution style for their failures and successes in comparison to patients with schizophrenia and medical disorders. According to this study, patients with depression made more internal, stable and global attributions for bad events when compared to other disorders.[ 60 ] A study evaluating the cognitive model of depression as given by Beck failed to find support for the causal role of cognitive errors in relapse of depressed subjects as a significant proportion of patients were free from cognitive distortions following remission. However, it was also observed that those who had persistent cognitive distortions during remission ran the risk of early relapse.[ 61 ] It has also been seen that patients with neurosis, including depression, have poor social interactions and reports of more interactions of unpleasant type and less of pleasant type of social interactions as compared with healthy controls.[ 62 ]
With regard to personality factors, a study showed that higher scores on the hardiness, a personality trait, correlates with lower scores on the depression scale suggesting that presence of hardiness doesn’t allow depressive feelings to become more severe.[ 63 ]
Studies on Neurobiology
Compared to schizophrenia, there is relatively less research on the neurobiology of depression from India.
Neurochemicals
Studies have evaluated the levels of catecholamine metabolites in cerebrospinal fluid, urine and blood. Studies have showed that urinary 5-hydroxyindoleacetic acid (5HIAA), a metabolic end product of serotonin was significantly higher in depressed patients as compared to controls and these values decreased following successful treatment.[ 64 , 65 ] However, one study didn’t find any difference in the CSF 5HIAA levels between subjects with depression and healthy controls.[ 66 ] Studies have also shown significant positive correlation between the severity of depression and the patient’s urinary 5HIAA values[ 64 , 65 ] and negative correlation between the suicidal ideations and the patient’s urinary and cerebrospinal fluid (CSF) 5HIAA and homovanillic acid (HVA) values.[ 65 ] It has also been found that compared to controls, platelet serotonin (5HT) uptake is significantly lower in patients with depression, which improves temporarily with Electroconvulsive Therapy (ECT).[ 67 ] Another study by the same authors showed that treatment with Imipramine resulted in significant decrease in platelet 5HT uptake while ECT led to a significant increase in uptake.[ 68 ] With regard to dopamine metabolites, it has been seen that HVA levels are low in depressed subjects compared to controls and this correlates with platelet monoamine oxidase (MAO) activity, erythrocyte adenosine deaminase activity and 5-HIAA levels.
Further it was seen that compared to healthy controls HVA/5-HIAA ratio was also lower in subjects with depression.[ 66 ] Studies have also shown that both ECT and imipramine cause significant reduction in platelet MAO activity which normalizes during the post-treatment phase.[ 69 ] It has been demonstrated that there is significantly low serum dopamine-β-hydroxylase activity in patients with psychotic major depressive disorder compared to healthy controls. However, dopamine-β-hydroxylase activity did not differ between healthy controls, acute schizophrenia patients, subjects with non-psychotic depression and mania.[ 70 ] Srinivasan et al .[ 71 ] also showed that during the acute phase of depression there is low urinary 5-vinyl mandelic acid (VMA), which decrease further during treatment with imipramine. The authors also observed similar finding with respect to effect of imipramine in normal subjects. The levels also normalize in post treatment phase in depressed subjects and after stoppage of treatment in normal subjects.
Electrophysiology
Many studies have evaluated the pretreatment P300 amplitude and latency in depressed subjects and compared the same with healthy controls. All studies have consistently shown that P300 amplitude is smaller in depressed subjects and this normalizes with recovery[ 72 – 74 ] suggesting that it may be a state marker of depression. However, the findings with regard to pretreatment P300 latency are inconsistent.[ 72 – 74 ] Studies have also shown that P300 latency has a significant positive correlation with age of the patient and severity of depression while P300 amplitude have a significant negative correlation with age[ 73 ] and severity of depression.[ 72 ] A study showed that P300 amplitude is lower in depressed subjects, but there is no difference between subjects with depression and dysthymia,[ 74 ] however, the pretreatment P300 amplitude does not predict the treatment response.[ 75 ]
Studies have also shown that there is no difference between subjects of melancholic depression and control group in the latency of the middle components of evoked potential.[ 76 ] Studies done using Bereitschafts potential (BP) a measure of frontal lobe functions have also shown that the amplitude and frequency are lower in subjects with melancholic depression compared to the healthy controls.[ 76 ]
Endocrinology
As a marker for melancholia, studies have shown that dexamethasone suppression test (DST) has low sensitivity but high specificity.[ 77 – 79 ] Studies have also shown that, compared to DST suppressors, DST non-suppressors are significantly more depressed, attempt suicide more frequently, have higher rates of past and family history of depression, more frequently require electroconvulsive therapy and show better response to treatment.[ 79 ] A similar finding with respect to response to treatment has also been reported in another study.[ 80 ] Another study on subjects with post-stroke depression showed that DST response parallels the clinical course and response to treatment.[ 81 ]
A study of absolute eosinophil counts as an indirect measure of adrenocortical function showed that initial pretreatment level of eosinophils was higher than normal in depressed subjects, which showed a variable response to treatment indicating towards a varying degree of diminished activity of adrenal cortex.[ 82 ] Studies have also shown that plasma cortisol levels are higher in depressed subjects compared to healthy subjects and the increase in levels do not correlate with severity of depression.[ 83 ] Studies have also demonstrated that abnormal plasma cortisol level can differentiate patients with depression from patients with schizophrenia and healthy controls with a confidence level of 60%-93.3%. Urinary free cortisol after dexamethasone suppression test was considered as the best indicator of differentiating depressed patients from healthy subjects and patients with schizophrenia.[ 84 ]
Adrenocorticotrophic Hormone (ACTH) measurements, both at baseline and post-DST in patients of major depression, have been found to be significantly high at baseline and post DST as compared to healthy controls.[ 85 ] A study evaluated the role of dexamethasone in treatment based on the hypothesis that dexamethasone would hasten recovery, but did not find any advantage in favor of dexamethasone compared to placebo.[ 86 ]
Two studies have found that significantly higher number of patients with unipolar depression have subnormal T3 and T4 levels and a corresponding increase in thyroid stimulating hormone (TSH) levels compared with healthy controls.[ 87 , 88 ] In the second study, mildly depressed patients had significantly lower and severely depressed patients had significantly higher levels of TSH suggesting the direct relationship of severity of depression and TSH levels.[ 88 ] Another study found that 20.5% subjects of major depressive disorders have hypothyroidism.[ 89 ] When drug naive first episode depression patients were evaluated, they showed significantly higher T4 levels, but there was no significant difference in the level of T3 and TSH between depressed and healthy control subjects. However, when depressed subjects with and without psychotic features were compared, it was seen that subjects with psychotic symptoms had significantly higher levels of TSH.[ 90 ]
It has been shown that there is significant increase in total CSF protein levels in depressed subjects compared to subjects with neurological and surgical illnesses. It was seen that levels of immunoglobulin (Ig) M, IgG and IgA are higher in depressed and neurologically ill subjects compared to subjects with surgical illnesses. Further, when the levels of immunoglobulins were compared between depressed and normal subjects, IgG and IgA were significantly higher in depressed subjects. However, the study did not find evidence of viral markers for rubella and cytomegalovirus in CSF of depressed subjects.[ 91 ]
Extracellular fluid
Verma and Wig[ 92 ] showed that the extracellular fluid (ECF) volume of patients with depression tends to be lower than normal controls and normalization of the same correlates with clinical improvement.
Studies have shown that urinary melatonin levels can help in distinguishing subjects of endogenous depression from those with neurotic depression. Nocturnal as well as 24 hours urinary melatonin levels are low in subjects with endogenous depression whereas subjects with neurotic depression have higher than normal levels. It was also seen that melatonin levels were related to suicide attempts, diurnal variation and psychomotor retardation.[ 93 ]
Neurocognitive functioning
Studies from India suggest that definite cognitive impairments are present in the domains of intelligence and memory (Bhatia’s Battery test or the Weschler Adult Performance Intelligence Scale and PGI memory scale) in the depressed state but these don’t persist following recovery.[ 94 – 96 ] It is also reported that subjects with depression perform poorly on the Wisconsin Card Sorting Test (WCST) as compared to controls suggesting cognitive inflexibility and prefrontal dysfunction. Further, more severe illness is associated with greater impairment in the executive functioning on WCST.[ 97 ] Other studies which have evaluated various cognitive domains have shown that when patients with depression are asked to discriminate the emotional tone in terms of intensity of facial expression while presented in pairs, it is seen that they are highly evaluative of sadness and less evaluative of happiness, in comparison to the normal.[ 98 ]
A recent study, showed the importance of genetic factors in treatment response. Significantly better response to escitalopram was seen in patients homozygous for long allele of the serotonin transporter gene compared to the patients who were homozygous for short allele or heterozygous for short and long allele.[ 99 ] Another study showed that short variants of D7S1875 marker in LEP gene may be a risk factor for depression.[ 100 ]
A recent study evaluated the serum total cholesterol level in depressed subjects and showed that there is significant elevation of serum total cholesterol in depressed patients compared with normal controls and this persists even after controlling for the confounders.[ 101 ] Another study suggested that measurement of serum cholesterol levels may actually indicate towards hypothyroidism in depressed subjects.[ 89 ]
Symptomatology
Many studies have reported the symptom profile of subjects with depressive disorders.[ 5 , 13 , 36 , 39 , 52 , 102 – 118 ] The findings of symptomatology in general can be understood with respect to somatic symptoms, guilt and other depressive ideations, suicidal behavior, phenomenology of delusions and sleep architecture. Studies have also compared the symptomatology across different regions of the country. Studies have also attempted to distinguish the phenomenology in depression from negative symptoms of schizophrenia and the phenomenology in dysthymia.
One common theme with regard to symptomatology of depression, which has been reported by most of the researchers is high prevalence of somatic symptoms and some studies report that somatic symptoms are the most common manifestation of depression in India.[ 5 , 36 , 104 – 106 , 110 , 111 , 119 , 113 , 114 , 122 ] Erna Hoch[ 120 ] also reported that many Indian subjects with depression have hypochondriacal ideas considering body and its functioning. Studies have also shown pain as a depressive equivalent symptom.[ 42 , 1 ] Studies which have compared Indian subjects with depressed subjects from the West have also reported that somatic symptoms are more common in Indian subjects.[ 104 ] On the other hand studies on prevalence of functional somatic complaints in patients attending the psychiatric outpatient have also reported that most of these cases are diagnosed as depression.[ 122 , 124 ] It has also been shown that depressed subjects have greater difficulty in identifying bodily sensations and feelings as well as in expressing feelings.[ 125 ]
However, some of the studies evaluating depressive symptoms using the standardized instrument have reported that other symptoms are also present quite frequently in depressed subjects. One study which assessed 100 subjects with depression on HDRS reported that depressed mood and difficulties in work are present in all cases. Other symptoms reported in more than 50% of subjects included late insomnia, somatic anxiety, initial insomnia, psychic anxiety, suicidal ideations, retardation, loss of insight, middle insomnia, genital symptoms, hypochondriasis, gastrointestinal symptoms, agitation somatic symptoms in general and diurnal variation. Depersonalization, paranoid and obsessional symptoms were reported very infrequently. Guilt was present in about half of the subjects.[ 106 ] Gutpa et al .[ 103 ] also studied the symptomatology of depression from north India and compared it with findings from south India. Significantly higher number of subjects from north India reported joylessness, disruption in social functioning, lack of self confidence, early morning awakening, lack of appetite, feeling of pressure, other psychological symptoms, psychomotor restlessness, mood worsening in the morning, subjective experience of memory loss, retardation and guilt feeling; significantly higher number of subjects from south India reported hypochondriasis. There was no difference in other symptoms.
Another study from north India evaluated the symptomatology of depression and reported sadness, lack of interest, disturbed sleep, hypochondriasis, poor concentration, agitation, suicidal thoughts, and appetite change as the commonly occurring symptoms. Guilt was reported in about 40% of cases. The authors also compared their findings with studies from other parts of the country and the West and reported that lack of interest was more common in subjects from north India while reduced self-confidence, delusions and suicidal thoughts were seen more often in the south Indian sample.[ 47 ] Studies from Mumbai less frequently reported hypochondriasis, guilt, weight change, reduced interest and more frequently reported constipation.[ 47 ]
Earlier a few dynamically oriented psychiatrists envisaged guilt as the core symptom of depression. It is also suggested that guilt is less commonly seen in eastern population compared to the west. Studies done in India suggest that it is present in 5.3-67.5% of subjects.[ 36 , 47 , 106 , 126 – 129 ] Bhattacharyya and Vyas[ 130 ] also reported lesser frequency of guilt feeling in Indian subjects compared to Australian subjects. Venkoba Rao[ 127 ] on the basis of karma theory hypothesized that guilt may not be integral part of depression in Indian subjects and is actually a consequence of depression.[ 127 ] Sethi et al .[ 131 ] also reported that there is no relationship of guilt with severity of depression[ 131 ] and Trivedi et al .[ 132 ] reported higher level of guilt in depressed subjects as compared to neurotic subjects.[ 132 ]
Studies have also evaluated suicidal thinking in depressed subjects. Venkoba Rao and Nammalvar[ 107 ] reported that about two-third of depressed subjects have suicidal behavior and on the basis of the content they classified suicidal ideation into four broad categories, viz., ideas to kill oneself, a mere wish to die, a wish to be killed and a fourth unclassifiable category. In a recent study on the relationship between anger and suicidality, depressed patients with anger attacks exhibited more suicide-related phenomena in comparison to depressed patients without anger attacks.[ 133 ] Studies which have evaluated depressed subjects with suicidal ideation have shown that 16.6% of these subjects make suicidal attempt and a higher risk of suicidal attempt is found in individuals less than 30 years of age, single men, married women and students and higher education.
Attempters scored significantly higher in severity of suicidal ideation, agitation and paranoid symptoms whereas among non-attempters, hypochondriasis and general somatic symptoms were more common.[ 111 ] Studies have also shown that depressed subjects who attempt suicide are at higher risk of indulging in further suicidal behavior, compared to those who do not attempt.[ 134 ] However, it has also been shown that presence of suicidal behavior does not predict overall poor clinical outcome.[ 135 ]
Studies have reported that amongst the delusions in subjects with depression, delusions of persecution occurs most frequently (67.5%) (with persecution involving either the patients themselves or people close to them) followed by delusions of reference. Hypochondriacal, guilt and nihilistic delusions, which are considered classical in depression, are relatively uncommon in Indian subjects.[ 109 ]
In terms of sleep architecture, it is reported that subjects with depression have lesser total sleep time, longer sleep latency, frequent awakenings, greater wake-after-sleep onset and offset times, lesser sleep efficiency and tendency to wake up earlier than controls. Subjects with severe depression differ from patients with mild and moderate depression with regards to total sleep time, night-time sleep and sleep efficiency.[ 136 ]
Studies which have tried to distinguish depression from negative symptoms have shown that depressed patients score significantly higher on subjective complaints, total score, global ratings on Scale for the Assessment of Negative Symptoms (SANS), while schizophrenia is associated with significantly higher scores on global rating of alogia, poor eye contact, inappropriate affect, and blocking.[ 115 ] Another study showed that anhedonia-asociality are seen commonly in both patients with depression and schizophrenia while the global ratings on affective flattening, alogia, avolition and inattention are significantly higher in subjects with schizophrenia.[ 114 ]
A study which tried to distinguish the symptomatology of chronic major depression and dysthymia showed that symptomatically dysthymia and chronic major depression are indistinguishable.[ 137 ]
Studies have evaluated the symptomatology of depression in elderly depressed subjects too and have reported that the common symptoms in order of frequency were sadness, depressed mood, somatic symptoms and signs, suicidal ideas, lack of energy, anxiety or tension, inability to fall asleep, early awakening, hopelessness, irritability and inability to enjoy.[ 41 ] Another study from community sample reported that disturbed sleep pattern is the most common symptom in depressed elderly subjects.[ 21 ]
One study which evaluated the symptomatology of depression in children and adolescents reported multiple somatic complaints as the most common presenting complaint in children with depression.[ 117 ] Another study, which compared the symptomatology of children and adults, showed that more children than the adults presented with the somatic symptoms and the predominant mood symptom in children was irritability in contrast to sadness in adults.[ 118 ] Other commonly reported symptoms of depression across studies include low mood, diminished interest in play and activities, excessive tiredness, low self-esteem, problems with concentration, behavior symptoms like anger and aggression, decreased interest in school and recent deterioration in school performance, death wish and suicidal behavior.[ 52 , 117 ]
In terms of symptomatology in postpartum depression, infanticidal ideas have been reported in depressed mothers.[ 34 ] Studies evaluating subjects with seasonal affective disorder found that atypical vegetative features are not prominent part of the symptomatology in India.[ 138 , 139 ]
Comorbidity
Many studies done in subjects with depression have shown high level of comorbidity of both psychiatric and physical illnesses, especially in elderly individuals with depression.[ 140 , 141 ] On the other hand studies on the prevalence of psychiatric morbidity in physical disorders have shown that depression is quite prevalent in these conditions.
The commonly reported physical illnesses in subjects with depression include those involving the musculoskeletal, cardiovascular and ophthalmological systems and the commonly reported diagnosis in order of frequency were osteoarthritis, hypertension and cataract in one study.[ 141 ] The commonly and consistently reported comorbid conditions in children with depression include anxiety and conversion/dissociative disorder.[ 51 , 116 , 117 ] Other comorbid conditions include, dysthymia, adjustment disorder, conduct disorder and attention deficit hyperactivity disorder.[ 52 , 117 , 118 ]
Studies done in subjects with intentional self harm,[ 142 ] subjects with serious suicide attempts,[ 143 ] divorce seeking couples,[ 144 ] earth quake victims,[ 145 ] women after childbirth,[ 56 ] obsessive compulsive disorder,[ 146 ] alcohol dependent subjects,[ 147 ] dementia,[ 148 ] Dhat syndrome,[ 149 , 150 ] medical in-patients,[ 151 – 153 ] epilepsy,[ 154 ] neurological disorders,[ 155 ] end stage renal disease,[ 156 , 157 ] cancer,[ 158 ] chronic and disfiguring skin disorders,[ 159 ] age related macular degeneration,[ 160 ] HIV-infected heterosexuals,[ 161 ] industrial population[ 162 ] have shown that depression is the most common or one of the most common psychiatric diagnosis in such patient groups and prevalence rate as high as 86.7% has been reported.
Assessment and Diagnostic issues
In clinical and research work, apart from uniform diagnostic criteria, some means to objectively quantify the presence of particular symptoms and level of their severity is required. For this purpose, a number of rating scales have been devised worldwide, of which clinician rated Hamilton Depression Rating Scale (HDRS),[ 163 ] self reporting Beck Depressive Inventory,[ 164 ] Montgomery-Asberg Depression rating scale (MADRS) are the most popular ones. Indian researchers have adapted/modified these scales for the Indian population.[ 165 – 167 ] Additionally scales like Amritsar Depressive Inventory (ADI) a self reporting scale has been developed on the basis of symptoms and signs of depression as manifested by Indian patients.[ 168 ] Avasthi et al .[ 169 ] translated the PRIME-MD questionnaire in Hindi and showed that it is useful for screening various psychiatric disorders. Brief Patient Health Questionnaire (PHQ) has also been translated in 11 languages and validated for Indian population.[ 170 ] Many studies have also evaluated the psychometric properties of various scales in India.[ 171 – 173 ]
Impact of Depression
Studies have shown that depressive disorders lead to significant dysfunction,[ 174 ] disability[ 175 ] and poor quality of life in sufferers[ 176 ] and pose a significant burden on the caregivers.[ 177 ] The pattern of burden experienced by relatives of patients with affective disorders and schizophrenia have been shown to be similar, being principally felt in the areas of family routine, leisure, interaction and finances. However, the caregivers of subjects with depression experience lesser degree of burden compared to caregivers of schizophrenia and bipolar disorders.[ 177 , 178 ] Another study showed that the burden of dysthymia is similar to neurotic disorders like obsessive compulsive disorder and generalized anxiety disorder.[ 179 ] It has also been seen that patients with dysthymia have significant impairment on measures of quality of life, disability, social support and marital adjustment compared to normal/medically ill controls. The study also showed that duration of illness and severity of depression are the most important correlates of impaired quality of life and disability.[ 180 ] A study assessing the relationship of stigma to both depression and somatization in psychiatric patients of south India showed that although both depressive and somatic symptoms were distressing, perceived stigma was more for depressive symptoms. Depressive symptoms were perceived as socially disadvantageous as compared to somatization symptoms.[ 167 ]
Course and Outcome
There are very few studies which have evaluated the course and outcome of depression. Venkoba Rao and Nammalvar[ 181 ] followed up 109 out of 122 cases of endogenous depression 3-13 years after their index diagnosis. No recurrence occurred in 28 cases. Forty-two cases turned out to be bipolar and 21 remained unipolar. Manic episodes outnumbered the depressive ones. The change of polarity from depression to mania occurred within three years after the initial depression, though in others the shift occurred between 3 to 12 years. The number of episodes of depression before the onset of mania varied from one to three. While the onset of depression before the age of 40 years predisposed to recurrences, there was risk of chronicity in those patients who developed the illness after 40 years. Gada[ 182 ] studied 92 out of 100 cases of major depressive disorder five to 10 years after the index diagnosis and showed that 36.6% cases had no recurrence of episodes and 63.4% had recurrence. Of the total subjects, 37.8% were diagnosed as bipolar disorders and 25.6% were diagnosed as recurrent depressive disorder.
The change of diagnosis from major depressive disorder to bipolar disorder occurred within three years after the initial depression in 77% of cases. In another study Brown et al .[ 183 ] reported that in their cohort all subjects experienced full recovery within the one-year period. At one-year follow-up, 71% of depressive patients demonstrated no symptoms or social impairment.[ 183 ] The mean duration of depressive episode was 14.2 weeks and the rate of relapse was 18%. The authors concluded that overall outcome was considerably more favorable than in comparable studies of affective disorders in developed settings. All these findings suggest that depressive disorders have high chance of recurrence and many of the subjects initially diagnosed as cases of unipolar depression are later diagnosed as bipolar disorder. In another prospective study of subjects with seasonal affective disorder (SAD), Avasthi et al .[ 184 ] reported that after the initial diagnosis of SAD the subjects did not display any variation in mood, behavior, sleep pattern and weight fluctuation over a period of five to seven years.
Study done in elderly patients with depression have reported that complete recovery occurs in 58% of cases and 24% of cases have partial recovery with 18% registering relapses.[ 41 ] Sachdev et al .[ 185 ] studied various factors related to prognosis of depression and found that sleep-disturbances, agitation, depth of depression, age at the time of present onset, age at first onset, history over one year with no symptom-free period, sudden onset of illness, adequate premorbid personality and adequate psychogenesis are of prognostic significance.
Treatment related issues
One of the important patient characteristic which has been reported to influence treatment adherence is their attitudes and beliefs towards medication. In a recent study, Chakraborty et al .[ 186 ] reported that most of the patients value the doctor-patient relationship and their partners are also supportive regarding diagnosis and treatment of depression. However, most patients have erroneous beliefs regarding antidepressants per se which in turn influence the drug compliance.
Sethi et al .[ 187 ] proposed a prevention model for depression focusing on improving social network and educational programs, designed to educate the public with regard to the risks inherent to change of jobs, residence, pattern of living as well as how to protect against them and removal of malnutrition and infections. A recent review suggests that religiosity can be protective against depression.[ 188 ]
Conclusion and future directions
Depression is the most common psychiatric disorder reported in most of the community based studies. It is also reported as one of the most common psychiatric disorder in outpatient clinic population and in subjects seen in various medical and surgical setting. It is also reported to be the most common psychiatric disorder in elderly subjects across various settings. Studies from India have also shown that life events during the period preceding the onset of depression play a major role in depression. Studies on women have also shown the importance of identifying risk factors like interpersonal conflicts, marital disharmony and sexual coercion.
There is need for further study of factors like cost, attitude towards treatment, adherence, compliance and neurobiological correlates. There is also a need to study the course of depressive disorders in India so as to determine the need and duration of continuation treatment. Studies should also evaluate the cost-effective models of treatment which can be easily used in the primary care setting to effectively treat depression.
Source of Support: Nil
Conflict of Interest: None declared.
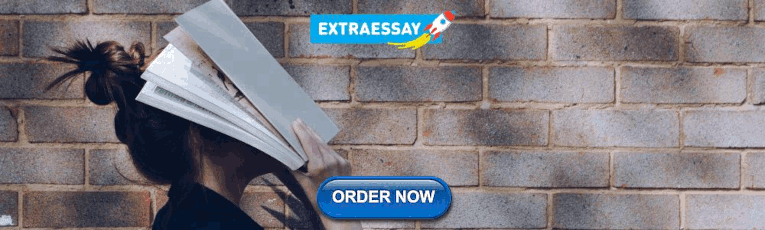
IMAGES
VIDEO
COMMENTS
Our lack of knowledge cannot be put down to a scarcity of research in existing treatments. In the past decades, more than 500 randomised trials have examined the effects of antidepressant medications, and more than 600 trials have examined the effects of psychotherapies for depression (although comparatively few are conducted for early-onset depression).
Depression is a prevalent psychiatric disorder that often leads to poor quality of life and impaired functioning. Treatment during the acute phase of a major depressive episode aims to help the patient reach a remission state and eventually return to their baseline level of functioning. Pharmacotherapy, especially selective serotonin reuptake ...
The paper gives a complete overview of what is known about therapies for depression. • Several different types of therapy are effective. • Therapy can be effectively be delivered in different formats. • Therapy is as effective as drugs at the short, but more effective at the long term. • Combined treatment is better than drugs or ...
Abstract. Treatment outcomes for major depressive disorder (MDD) need to be improved. Presently, no clinically relevant tools have been established for stratifying subgroups or predicting outcomes ...
Treatment for depression exists, such as pharmacotherapy, cognitive behavioural therapy, and other modalities. ... This review may be used as an evidence base by those in public health, clinical practice, and research. This paper discusses key areas in depression research; however, an exhaustive discussion of all the risk factors and ...
Depression affects over 300 million individuals worldwide and is responsible for most of the 800,000 annual suicides. Clinical practice guidelines (CPGs) for treatment of depression, founded on scientific evidence, are essential to improve patient care. However, economic and sociocultural factors may influence CPG elaboration, potentially leading to divergences in their recommendations.
This issue of the Journal is broadly focused on mood disorders, with an emphasis on understanding how treatments for major depressive disorder may work and how the efficacy of current neuromodulation and antidepressant medication treatment strategies can be enhanced. The issue begins with an overview by Drs. Manish Jha and Sanjay Matthew on treatment-resistant depression (); they focus on ...
Using a community-based participatory research (CBPR) design, the study team developed a model of depression care for this population. The study involved 45 people in the model development and 65 people in the model testing, who were patients, family members, village health volunteers (VHVs), community and religious leaders, healthcare ...
The idea that depression is the result of abnormalities in brain chemicals, particularly serotonin (5-hydroxytryptamine or 5-HT), has been influential for decades, and provides an important ...
Molecular Psychiatry - Advances in depression research: second special issue, 2020, with highlights on biological mechanisms, clinical features, co-morbidity, genetics, imaging, and treatment
Identification of clinical practice guidelines. We recently reported CPGs for the pharmacological treatment of noncommunicable diseases that could be considered "high-quality" [].In that study, we conducted individual systematic reviews for each included disease; and using the second version of the Appraisal of Guidelines for Research and Evaluation (AGREE II), evaluated 421 CPGs to ...
2. The effects of treatment of depression on quality of life and other outcomes. Although too little research has focused on what patients consider to be the most important goals of treatment and the effects of treatments on these goals, there is a considerable literature showing that treatment of depression not only affects depression, but many other outcomes.
Depression treatment should strive for full remission. The essential treatment option of antidepressants (ADs) is endorsed by numerous national depression care guidelines (DCGs), e.g. [1,2,3,4], particularly in moderate to severe depression, as robust evidence indicates their effectiveness and safety [].AD monotherapy is an evidence-based treatment option for acute depression and relapse ...
In line with the Research Domain Criteria Project launched by the National Institute of Mental Health (Insel, 2014; Insel et al., 2010), a distinguished aim in developing an integrated neuroscientific model of depression therefore has to be the separation of distinct aetiological and pathophysiological trajectories which, although eventually ...
Background Antidepressant medication (ADM) and psychotherapy are effective treatments for major depressive disorder (MDD). It is unclear, however, if treatments differ in their effectiveness at the symptom level and whether symptom information can be utilised to inform treatment allocation. The present study synthesises comparative effectiveness information from randomised controlled trials ...
This issue of the Journal also presents original research articles that address topics related to the treatment of bipolar disorder, the use of a new transcranial magnetic stimulation (TMS) strategy for treatment of refractory depression, and the effects of gender-affirming interventions on the treatment of mood and anxiety disorders in ...
Also included in this issue is a review of the data addressing the reemergence of the use of psychedelic drugs in psychiatry, particularly for the treatment of depression, anxiety, and PTSD . This timely piece, authored by Dr. Collin Reiff along with a subgroup from the APA Council of Research, provides the current state of evidence supporting ...
Analysis of published papers around the world from 2009 to 2019 in depressive disorder. A The total number of papers [from a search of the Web of Science database (search strategy: TI = (depression$) or ts = ("major depressive disorder$")) and py = (2009-2019), Articles)].B The top 10 countries publishing on the topic.C Comparison of papers in China and the USA.
PDF | Major depression is a mood disorder characterized by a sense of inadequacy, despondency, decreased activity, pessimism, anhedonia and sadness... | Find, read and cite all the research you ...
The possible causes of depression are many and not yet well understood. However, it most likely results from an interplay of genetic vulnerability and environmental factors. Your depression research paper could explore one or more of these causes and reference the latest research on the topic. For instance, how does an imbalance in brain ...
Schematically, one may categorize the treatment of depression into three phases: acute, continuation, and maintenance. 3, 4 As summarized in Table I, each phase is defined by specific aims and strategies. Some aspects remain under discussion, especially those concerning the appropriate duration of long-term treatment.
The results of this study will form a baseline for future artificial intelligence-directed research. Methods: Overall, 13,224 new members registered between November 2021 and May 2022 for Evolution Health's self-guided treatment courses for anxiety and depression. The control arm featured a member home page without nudges or prompts.
Yi and Xiaoli used a bibliometric method to analyze the characteristics of the literature on the treatment of depression by Chinese medicine in the last 10 years. Zhou and Yan ... performed a bibliometric analysis of domestic and international research papers on depression-related genes from 2003 to 2007. A previous review of depression-related ...
Epidemiology. Many studies have estimated the prevalence of depression in community samples and the prevalence rates have varied from 1.7 to 74 per thousand population.[2,3] Reddy and Chandrasekhar[] carried out a metanalysis, which included 13 studies on epidemiology of psychiatric disorders which include 33572 subjects from the community and reported prevalence of depression to be 7.9 to 8.9 ...