Thank you for visiting nature.com. You are using a browser version with limited support for CSS. To obtain the best experience, we recommend you use a more up to date browser (or turn off compatibility mode in Internet Explorer). In the meantime, to ensure continued support, we are displaying the site without styles and JavaScript.
- View all journals
- My Account Login
- Explore content
- About the journal
- Publish with us
- Sign up for alerts
- Data Descriptor
- Open access
- Published: 16 August 2022
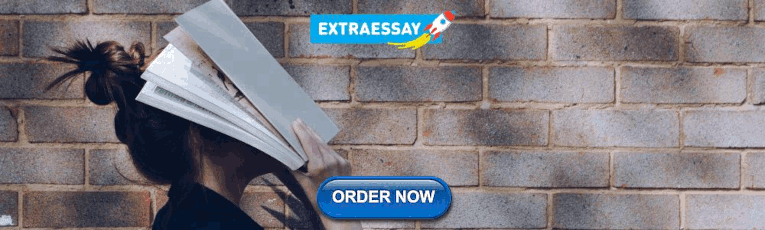
An Artificial Intelligence Dataset for Solar Energy Locations in India
- Anthony Ortiz 1 ,
- Dhaval Negandhi 2 ,
- Sagar R. Mysorekar 3 ,
- Shivaprakash K. Nagaraju 3 ,
- Joseph Kiesecker 3 ,
- Caleb Robinson 1 ,
- Priyal Bhatia 3 ,
- Aditi Khurana 3 ,
- Jane Wang 1 ,
- Felipe Oviedo 1 &
- Juan Lavista Ferres 1
Scientific Data volume 9 , Article number: 497 ( 2022 ) Cite this article
11k Accesses
7 Citations
39 Altmetric
Metrics details
- Energy conservation
- Environmental impact
Rapid development of renewable energy sources, particularly solar photovoltaics (PV), is critical to mitigate climate change. As a result, India has set ambitious goals to install 500 gigawatts of solar energy capacity by 2030. Given the large footprint projected to meet renewables energy targets, the potential for land use conflicts over environmental values is high. To expedite development of solar energy, land use planners will need access to up-to-date and accurate geo-spatial information of PV infrastructure. In this work, we developed a spatially explicit machine learning model to map utility-scale solar projects across India using freely available satellite imagery with a mean accuracy of 92%. Our model predictions were validated by human experts to obtain a dataset of 1363 solar PV farms. Using this dataset, we measure the solar footprint across India and quantified the degree of landcover modification associated with the development of PV infrastructure. Our analysis indicates that over 74% of solar development In India was built on landcover types that have natural ecosystem preservation, or agricultural value.
Similar content being viewed by others
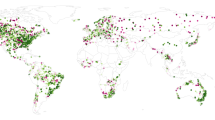
Global prediction of extreme floods in ungauged watersheds
Grey Nearing, Deborah Cohen, … Yossi Matias
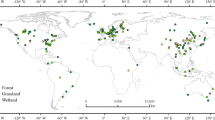
Meta-analysis shows the impacts of ecological restoration on greenhouse gas emissions
Tiehu He, Weixin Ding, … Quanfa Zhang
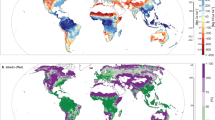
Accounting for albedo change to identify climate-positive tree cover restoration
Natalia Hasler, Christopher A. Williams, … Susan C. Cook-Patton
Background & Summary
India is rapidly expanding its deployment of clean energy 1 . The dual benefits of climate mitigation potential, and lower cost of production, make renewable energy cost-competitive compared to coal and other conventional energy sources. Therefore, to achieve the nationally determined contribution (NDC) targets such as: 40% share of non-fossil fuel cumulative power generation capacity, and to halt greenhouse gasses (GHGs) emission from fossil fuels, India has committed to 500 gigawatts (GW) of installed renewable energy capacity by 2030 2 . India intends to reach 225 GW of renewable power capacity by 2022 exceeding the target of 175 GW pledged during the Paris Agreement. As of 2018 India ranks fifth in installed renewable energy capacity with the fourth most attractive renewable energy market in the world.
Solar energy is expected to play an increasingly larger role in India’s clean energy transition. Of the 2030 (500 GW) target by 2030, solar energy is expected to contribute 300 GW 3 . Over the last five years, the installed capacity for solar energy has increased more than five-folds 4 . Of the total renewable energy (RE) capacity added during this period, more than two-thirds has come from utility-scale solar photovoltaic. Solar energy companies in India project the same trend to continue over the next five years with utility-scale solar energy expected to add 39 GW of the 60 GW of installed RE capacity 5 .
Despite the policy commitments in India, many studies have questioned the land-based targets for solar energy deployment and have highlighted the difficulties related to disputes over land use 6 , 7 . Renewable energy requires a large amount of space 8 . If these energy installations aren’t sited carefully, they can cause significant damage to wildlife, natural habitats and critical ecosystem services and even generate greenhouse gas emissions that reduce their climate benefits 9 . Despite the recognition of these challenges policy makers and governments have struggled to maintain robust geospatial information on the rapid expansion of renewable energy technologies. Access to these data will be critical to assess past impacts and planning to avoid future conflicts.
At present, there is limited information that is compiled and publicly available on the location of utility-scale solar photovoltaic projects across the country. Most location information for a project is typically limited to its associated jurisdictional boundary. The lack of more specific information, such as project boundaries, makes it difficult to identify factors that may be driving land suitability for such projects, and thus deprive policy-makers of the relevant information to expedite development. In addition, without such information, it is difficult to understand the nature of land-use changes driven by solar energy in India. This is particularly significant as some land-use changes from solar development (e.g., from biodiversity-rich habitats, or those places that are important for agriculture or pasture lands for local grazing-dependent communities) may lead to socio-ecological land conflicts and ultimately slow the transition to renewable energy. While some of the facility-level location information is collected by government agencies during project-level planning and construction phases, this information is not typically made publicly available. Other datasets that are publicly available, i.e. OpenStreetMap, usually do not capture the full range of development given sampling biases of these crowd sourcing approaches. Fortunately, freely available high-resolution remotely sensed imagery and new artificial intelligence techniques make it possible to now map utility-scale projects 10 , 11 , 12 , 13 .
We present the first country-wide database of solar photovoltaic farms for the country of India and show that it is feasible to also detect when the solar farms were created – allowing for further land use and sustainable development analysis. Our contributions are twofold:
A novel methodology for creating datasets of remotely sensed objects using satellite imagery when labeled data available is limited. This new method consists of a semantic segmentation model trained in stages using human-machine interaction and hard negative mining (HNM).
The quantification of land cover change associated with solar energy development in India. This analysis can inform policy makers to develop policies ensuring renewable energy is developed in low conflict areas.
Materials and Methods
Datasets are often created using human experts of crowdsourced labelers. However, there are use cases, like detecting small objects on the surface of the earth, where this task is costly, time consuming, and unscalable. When sufficient labeled data is available, machine learning models tend to be helpful reducing the time required to accomplish this task. Here we present a methodology for creating datasets of remotely sensed objects using satellite imagery when labeled data available is limited. To develop our map of utility-scale solar arrays across India first we assembled point labels of known solar PV farms and used human-machine interaction for a user to finetune an unsupervised model to create weak segmentation labels, labels obtained through weakly supervised learning 14 , of the solar farms. Then we paired these weak pixel-wise segmentation labels with geo-located Sentinel 2 imagery to train a supervised segmentation neural network and further improved in multiple stages of Hard Negative Mining (HNM). Finally, we estimated when solar PV installations were constructed and assessed the land use prior to construction for each array. Finally human experts validated the output of the AI model and individual solar arrays were clustered into solar farms using distance-based clustering. Figure 1 describes the proposed methodology.
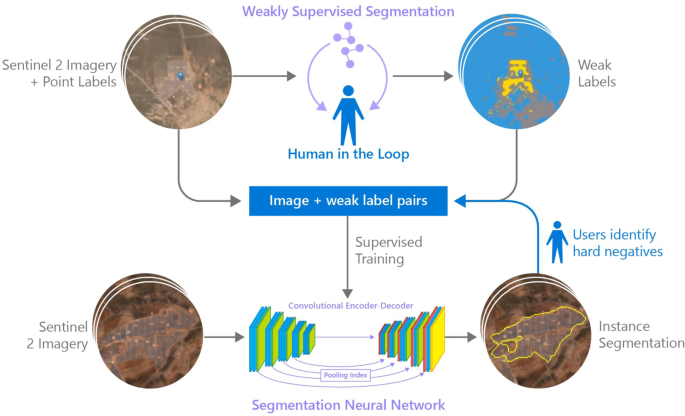
Proposed solar PV mapping pipeline. Given a small set of point labels and its corresponding Sentinel 2 imagery, pixels are clustered into multiple clusters (64 for our experiments). These clusters are merge into a user defined smaller set of classes (three in this example) using a linear classifier. Cluster merge results are shown in a web tool where a human user provides feedback on which pixels belong to the solar farms class or to the other background classes and the linear classifier if finetuned based on the feedback from the user. This weakly supervised segmentation process is represented at the top of this figure and is interactively performed to obtain weak semantic labels like the example shown at the top right of the figure. These labels paired with the corresponding geo-located Sentinel 2 image are used to create a semantic segmentation dataset suitable for supervised training of a solar farm semantic segmentation model. The obtained segmentation neural network can be used to perform inference for solar farms in novel scenes as shown at the bottom of the figure. False positive predictions are considered hard negatives and are used to augment the training dataset and finetune the supervised segmentation neural network improving its false positive rate. This process of performing inference in novel scenes, adding hard negative to the training set and finetuning the supervised model further can be repeated multiple times until the performance of the results is good enough for large scale inference.
Solar farms point labels dataset
We used a set of 117 geo-referenced point labels corresponding to the center point of different solar installations for the states of Madhya Pradesh (45-point labels) and Maharashtra (72-point labels) in India to train our initial solar mapping model. We also obtained 191 noisy solar installations point labels for four other Indian states including Kerala (15), Telangana (28), Karnataka (73), Andhra Pradesh (75). The noisy points labels did not accurately match the exact solar installation location. These labels were obtained using previously mapped solar farms through OSM and other Nature of Conservancy (TNC) partners.
Sentinel 2 (S2) satellite imagery
The Sentinel-2 program developed by the European Space Agency (ESA) provides global imagery in thirteen spectral bands at 10 m–60 m spatial resolution and a revisit time of approximately five days free of cost. In this work, we use 12 of the available spectral bands while excluding S2 Band 10 which is used mostly to mask out clouds since cloudy scenes were filtered out as the input to the solar mapping model.
Copernicus Global Land Cover
The Dynamic Land Cover map at 100 m resolution (CGLS-LC100) from Copernicus provides global land cover map at 100 m spatial resolution for the period 2015–2019 over the entire Globe, derived from the PROBA-V 100 m time-series. The product also includes all basic land cover classes including shrubs, herbaceous vegetation, cultivated and managed vegetation/agriculture, urban/built up, bare/sparse vegetation, snow and ice, permanent water bodies, and more.
NRSC Land Use Land Cover
Land Use Land Cover (LULC) maps for the country of India generated by the National Remote Sensing Centre (NRSC) at the Indian Space Research Organization 15 . Annual land use/land cover mapping is carried out at 1:250k scale and is made available at approximately 60 m/px resolution. Figure 6 shows a snapshot of the Land Use Land Cover data for the year 2017 at a 50 m/px resolution along a legend for the classes covered. This data along the Copernicus Global Land Cover is used for the land cover change analysis.
Semi-supervised label generation: from point labels to semantic annotations
Finding solar installations from satellite imagery can be formulated as a semantic segmentation computer vision task. The goal of semantic image segmentation is to label each pixel of an image with a corresponding class of what is being represented 16 . However, pixel-wise labels are required for semantic segmentation 17 . Manually creating segmentation labels is costly and time consuming. This problem exacerbates while working with noisy point labels with non-systematic displacements errors. To overcome this limitation and generate semantic labels at scale we first pre-trained a convolutional neural network to cluster pixels from Sentinel 2 satellite imagery by color in an unsupervised manner. We used an interactive web application similar to the one proposed by Robinson et al . 18 to quickly fine-tune the network to cluster pixels corresponding to solar installations into a single solar installation class as shown in Fig. 2 . This fine-tuned model is then used to obtain noisy semantic labels for all available point labels as shown in Fig. 2 parts D and E. The pixel-wise labels obtained make it possible to create a small semantic segmentation dataset suitable to train supervised semantic segmentation models.

Human-Machine interaction for unsupervised semantic label generation pipeline. ( A ) Use point labels to find features, ( B ) Initial unsupervised model will segment imagery at pixel level by color, ( C ) Fine-tuning to segment solar farms (yellow) vs other (blue, grey), ( D ) Apply the fine-tuned model to generate weak pixel-wise labels ( E ) Download labels generated in D as GeoTIFFs to incorporate into a solar installation semantic segmentation dataset of noisy semantic labels.
Weak labels solar PV installations segmentation dataset
Following the described semi-supervised semantic label generation approach applied to the solar farms point labels dataset for all states but Maharashtra, we generated an initial segmentation dataset consisting of 234 Sentinel 2 image patches of size 256 × 256 containing solar PV installations and corresponding pixel-wise labels for the classes “background” (0) and “solar PV installation” (1) and 50 pairs of randomly sampled images patches without solar installations with the corresponding pixel-wise labels. The dataset was split into training (80%), validation (10%), and test (10%) disjoint sets.
Pristine labels solar PV farms test set
The 72 locations with known solar farms from the point label dataset from Maharashtra, we manually labeled the outlines of the solar farms. These polygons along with corresponding Sentinel 2 imagery constitute what we call the pristine labels solar PV farms and were reserved for testing the models.
Supervised semantic segmentation of solar farms
Now we formalize our solar farms mapping approach. Let ( x n ) N represent a set of training Sentinel 2 satellite image patches. Each image patch x n is associated with a corresponding pixel-wise semantic segmentation mask. For each pixel ( i, j ) in the image patch x n we aim to assign a label l n = 1 when the pixel belongs to a solar installation and l n = 0 otherwise. For the segmentation of solar installations, we trained several U-Net models 19 with different depths and number of input filters on the solar PV installations segmentation training set. We used the Adam optimizer 20 with a batch size of 32 to train all our models. All neural network models were trained from randomly initialized weights using a learning rate (LR) of 0.001 (The LR hyperparameter controls how much the model weights change in response to the estimated error each time the model weights are updated) for 50 epochs (i.e., we showed the neural network all training samples 50 times). We decay the learning rate by 10% after 5 epochs of no performance improvement in the validation set. Weighted binary cross-entropy was used as the loss function. The model architecture with best performance in the validation set was selected for the rest of the experiments.
Hard Negative Mining (HNM)
The previously described dataset contains “easy” background examples obtained from a random sampling procedure. Models trained on the created dataset will see far more “easy” negative samples from background regions than difficult negative samples from areas similar in appearance, shape, or spectral signature solar PV installations. It has been shown that some form of hard negative mining is useful to improve the performance of object detectors 21 , 22 . In this work, we adopt a bootstrapping 23 approach where we train an initial model and test it by doing inference across different new sentinel image tiles. Inference results were visually inspected for false positive predictions. These false positive predictions represent “hard negative samples” and were added to the train set of the solar PV segmentation dataset. The segmentation model can now be re-trained using the new training set for better performance. The HNM procedure can be repeated multiple times.
Predictions post-processing
We incorporated OpenStreetMap 24 data to remove false positive predictions over road areas. We also used the Normalized Difference Snow Index (NDSI) 25 and the Normalized Difference Water Index (NDWI) 26 to remove false positive predictions around snow and water bodies, respectively.
Solar farms initial development
We use Microsoft’s Planetary Computer to query all available Sentinel 2 cloud-free imagery between 2015 and December of 2020 matching the outline of each of the predicted solar PV farms. We apply Temporal Cluster Matching (TCM), an algorithm for detecting changes in time series of remotely sensed imagery when footprint labels are only available for a single point in time 27 , to the Sentinel 2 i magery time series obtain from the planetary computer to identify when the detected solar farms from 2020 were first built using Sentinel 2 temporal. Figure 10 shows the KL divergence for all scenes in the S2 imagery time series used as input for the solar farm shown in Fig. 9 . The black horizontal line represents the median of the KL divergence values. The median KL divergence is used as threshold to determine the scene of initial development. TCM successfully predicts scene 41 as the scene in which the initial development of the solar farm is first observed. TCM was used to estimate the year of development for each solar farm in the released dataset. Scene 41 along with scenes pre and post development are shown on Fig. 9 along with the dates the scene was collected and the TCM computed KL divergence values. The estimated year of development is included for each solar farm in the released dataset. Note: Microsoft’s Planetary Computer is freely available at https://planetarycomputer.microsoft.com/ .
Land cover change analysis
The year of initial development obtained using TCM, along with the Copernicus annual Global Land Cover from 2015 to 2019 and the NRSC Land Use Land Cover data from 2017 previously described, facilitates the study on environmental and socio-economic implications of solar photovoltaic energy development by analyzing which landcover classes are being impacted by solar farms. Figure 7 shows Sentinel 2 imagery before the detected solar installation was built (2016) and after it was built (2020) along with the corresponding landcover from Copernicus annual Global Land Cover to illustrate how it can be informative of the type of landcover being impacted by the installation of the solar PV farms.
Data Records
Our solar farms dataset is stored in vector data form for the use of the community. The final dataset includes 1363 validated and grouped solar PV installations. We provide the vector data in the form of polygons or multi-polygons outlining the solar farms and center points with the geo-coordinates for the center of each installation in a file named “ https://github.com/microsoft/solar-farms-mapping/blob/main/data/solar_farms_india_2021.geojson ”.
The dataset includes the following variables:
fid: Unique identifier for a solar farm. It is shared by polygons belonging to the same farm.
Area: The area of the site in squared meters ( m 2 ).
Latitude: Latitude corresponding to the center point of the solar installation.
Longitude: Longitude corresponding to the center point of the solar installation.
State: Indian State where the solar PV installation is located at.
The raw data format can also be found at Zenodo at: https://zenodo.org/record/5842519#.Yn7UT_iZND9 under Creative Commons Attribution 4.0 International license 28 .
Technical Validation
Table 1 shows the performance of our model using the test set from our Solar PV installations segmentation dataset described in the previous section. We can observe how pixel-wise intersection over the union (IoU), mean pixel accuracy, and pixel-wise precision (rate of correct pixel predictions among all positive pixel predictions) improve by retraining the model with hard negative samples while pixel-wise recall (rate of correct pixel predictions among all pixels corresponding to a solar PV installation) decreases slightly as hard negative mining forces the model to be more conservative. Object-wise metrics like farm-wise recall (rate of correct solar-farm detections among all solar PV farms) better describe the performance of the model for this task since missing certain solar farm pixels have no practical effect in being able to detect the solar installation. Pixel-wise metrics are also more susceptible to being affected by the noisy nature of the dataset.
Table 2 shows the performance of our model in the small pristine solar PV test set of manually labeled solar farms. Our best model shows 80.7% intersection over the union and mean pixel accuracy of 95.6%, a pixel-wise precision of 91%, a pixel-wise recall of 86.6% and a farm-wise Recall of 94.4% before post-processing. This indicates that lower pixel-wise performance in the weak labels solar PV installations dataset might be due to the noise in the ground truth.
For qualitative results, Fig. 3 shows sample predictions from our model for a diverse set of image patches at different scales and under different background conditions. It shows robust segmentation performance across different locations. Figure 4 shows predictions over time for a single solar farm. It shows consistent performance in different imaging conditions.
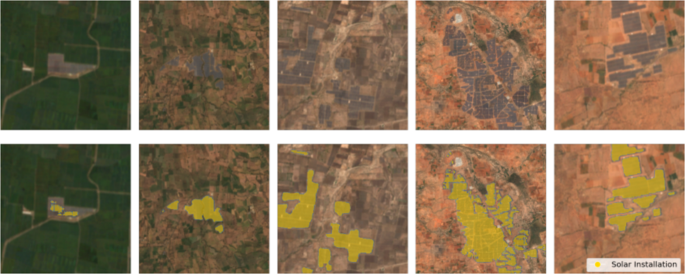
Example predictions. Examples of correct solar farm predictions from our model for different areas in India. Our model accurately outlines solar farms across different areas and backgrounds.
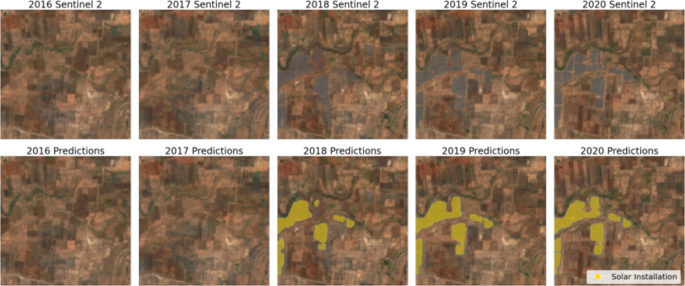
Predictions over time. Solar farms predictions across time for sentinel imagery median composites for the years 2016, 2017, 2018, 2019, 2020. These predictions allow the Pearson correlation analysis between Karnataka solar install capacity and model predictions.
Pearson correlation analysis of historical solar install capacity and temporal model predictions
The state of Karnataka in India provides information about the installed solar photovoltaic installed capacity since 2016 as shown in Table 3 . We obtain annual Sentinel 2 median composites using all available scenes with under 3% cloud coverage obtained between January and May for the years 2016–2020 covering the entire state of Karnataka. For the years 2016, 2017, and 2018 surface reflectance Sentinel products were not available. To alleviate this covariate shift, we perform tile-wise histogram matching 29 from Top of Atmosphere (ToA) Sentinel 2 median composites to the 2020 tiles surface reflectance Sentinel 2 median composites of the same area.
To further study the performance of our model, we conducted a Pearson correlation coefficient analysis between installed solar capacity and the predicted total solar installation area for the state of Karnataka in India. To do this, we run inference for the different median composite Sentinel 2 imagery after histogram matching 29 . Model predictions were polygonised and used to estimate the area of individual predictions. The total solar farm area predicted by our model is used to make a correlation analysis with the solar install capacity presented in Table 3 .
Figure 5 shows the Pearson correlation between total area of solar farms across time predicted by our model for the state of Karnataka and the total installed solar capacity in Thousand BTU’s per Hour (MB). The Pearson correlation coefficient is 0.957 indicating a very strong relationship between our model predictions and the solar install capacity released by the Indian state of Karnataka. The coefficient of determination ( R 2 ) or proportion of the variance of solar install capacity explained by our model predictions is 91.57% .
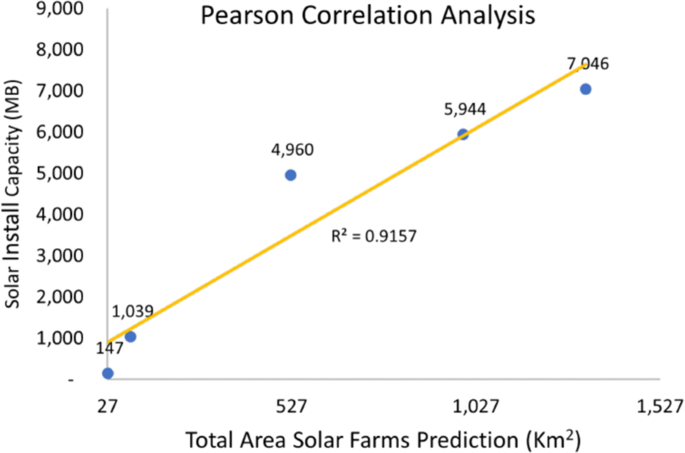
Pearson correlation between total area solar farms across time predicted by our model for the state of Karnataka and the solar install capacity in Thousand BTU’s per hour units (MB).
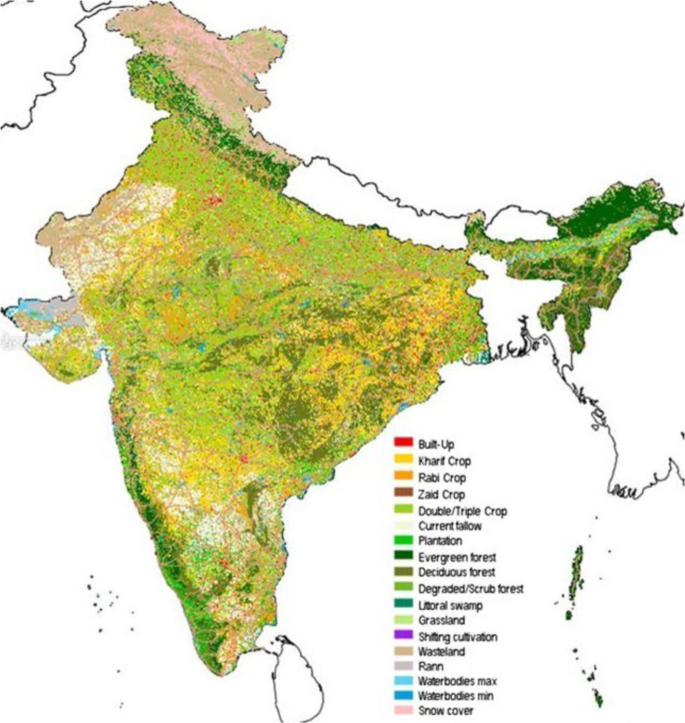
Landcover data at 50 m/px spatial resolution for the country of India for the year 2017. Note: The boundaries of India shown here are neither authenticated nor verified and are not to scale. They are only meant for graphical representation. All efforts have been made to make them accurate, however, neither Microsoft nor TNC own any responsibility for the correctness or authenticity of the same.
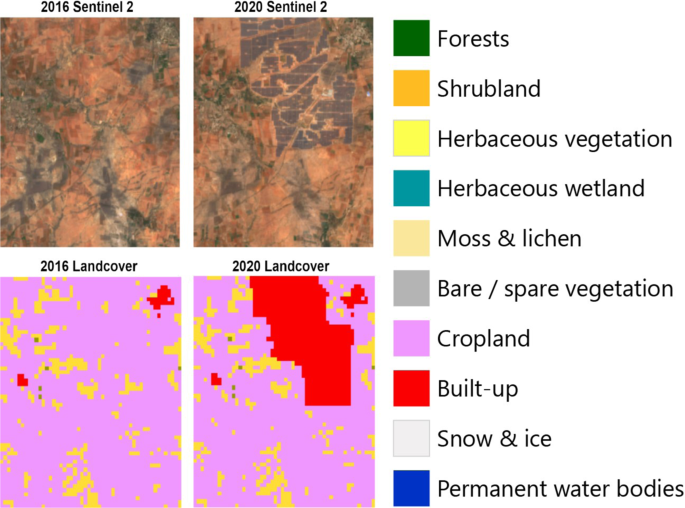
Sentinel 2 imagery before the detected solar installation was built (2016) and after it was built (2020) along with the corresponding landcover low resolution information. This information can be used to estimate how landcover changed to support the building of solar installations at scale. Note that solar PV installation is not a land cover type covered on this dataset.
Land cover land use change analysis results
Table 4 shows the percentage of each land cover class converted by solar PV installations across India. Over 74% of the solar farms installations in India were built on land cover types that could create potential biodiversity and food security conflicts - 67.6% of agricultural land and 6.99% of natural habitat - of which 38.6% of agricultural land may have potential to cultivate seasonal crops including Kharif (Kharif crops, or monsoon crops are domesticated plants that are cultivated and harvested during the Indian subcontinent’s monsoon season), Rabi (Rabi crops are agricultural crops that are sown in winter and harvested in the spring), and Zaid (Zaid crops are summer season crops), and 28.95% of land with plantation crop/orchards. The natural land cover types included sensitive ecosystems such as evergreen, deciduous, and littoral swamp forest with potential biodiversity value. However, our results are sensitive to data limitation. Because, as we strictly restricted our model threshold to reduce false positive solar areas, we were only able to map ~20% of currently installed utility-scale solar projects across India. Therefore, our results and interpretation of land use of impact of PV installations can change as and when future studies are able to map entire utility scale solar projects across India.
Manual data validation
To check the accuracy of our model predictions we performed a manual validation process. We overlaid our final model predictions after post-processing for the entire country of India on several base map layers inside QGIS and Google Earth software applications. We added Esri and Google Maps Satellite imagery in QGIS as a base map. We zoomed to each solar farm record and visually evaluated the overlay to tag the record either as a valid farm or invalid prediction or roof top (our model often mapped roof top solar farms as well). Figure 3 show multiple examples of valid solar farms predictions. Figure 8 show examples of invalid (A, C) and roof top solar predictions (B, D, E). We also cross-checked this by using historical high-resolution imagery available from Google Earth Pro’s “Show historical imagery” feature. In some cases where the use of satellite imagery was not conclusive enough, we also conducted Internet searches to identify reports or news about the presence of solar farms in each area. For example, public reports were used to validate the solar farm footprint for Rewa Solar power plant in Madhya Pradesh since part of the solar footprint was not current in the base maps used for reference.
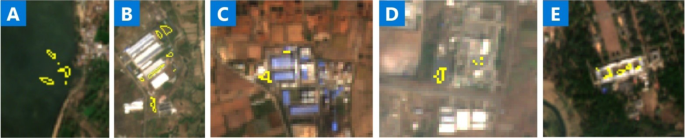
Examples of false positive predictions. The most common false positive predictions from our solar mapping model include electric plants (middle), construction sites (right), and seldom parking lots.
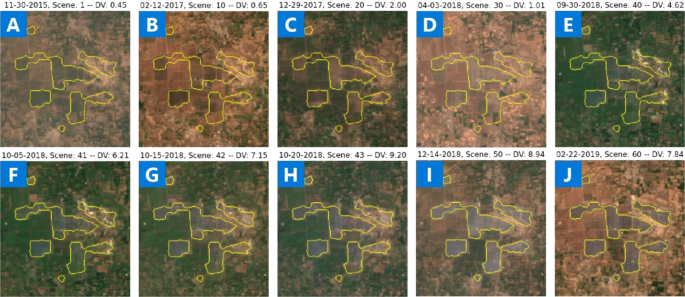
Set of sample scenes of cloud free Sentinel 2 imagery from time series for a predicted solar farm used as input to the Temporal Cluster Matching algorithm.
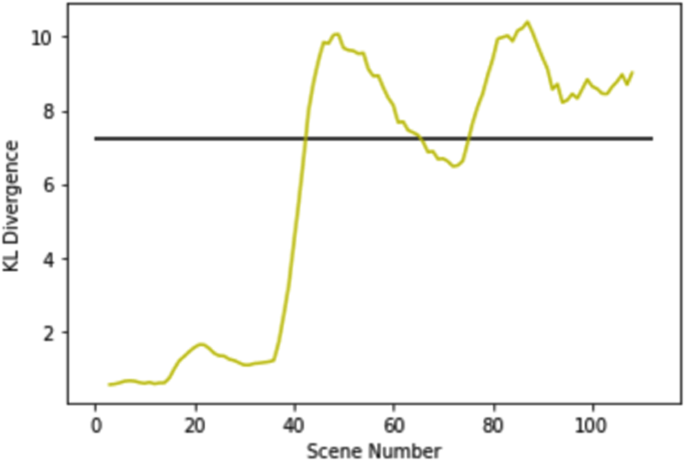
KL Divergence TCM corresponding to the time series imagery used on Fig. 9 . TCM successfully predicted scene 41 as the scene in which the initial development of the solar farm is first observed.
Cross India solar photovoltaics farms database generation
Most solar PV farms include tens of thousands of solar panels arranged in a non-contiguous way. Hence, our model would predict multiple independent polygons. The 4421 manually validated valid predictions from the previous analysis were spatially clustered based on a distance metric into multi-polygons to obtain 1363 individual solar PV farms. Table 5 shows the manual validation results. 92.54% of model predictions correspond to valid solar farms (85.27%) or roof top solar (7.27%) with only 7.46% of the predictions corresponding to invalid farms. Figure 11 shows center point locations for all predicted and validated solar farms in India across all states.
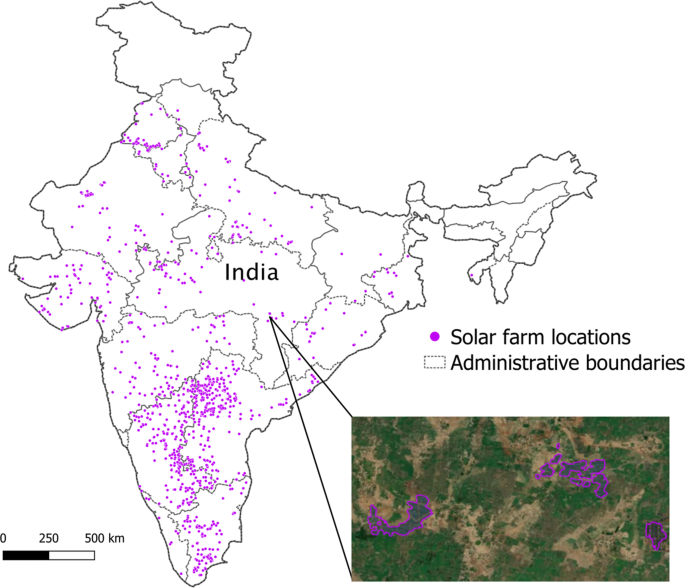
Solar PV Installations Mapping Results. Map showing outlines of solar farms detected by our model. Note: The boundaries of India shown here are neither authenticated nor verified and are not to scale. They are only meant for graphical representation. All efforts have been made to make them accurate, however, neither Microsoft nor TNC own any responsibility for the correctness or authenticity of the same.
Usage Notes
Solar energy is projected to be the major contributor to the renewable energy capacity addition in India and across the globe in the next couple of decades. Rapid deployment of renewable energy is critical to avoid the disastrous impacts of climate change. Using the power of artificial intelligence, we have developed a spatially explicit semantic segmentation model using noisy pixel-wise labels and hard negative mining to map utility-scale solar projects across India with a mean identification accuracy of 92%. Application of this model across the globe can help identify factors driving land suitability for solar projects and help public agencies plan better to facilitate solar energy development apart from helping track progress on solar energy developed. In addition, by mapping spatial patterns of solar development we can better understand land-use changes that may be driven by utility-scale projects. Empowering stakeholders which such information will catalyze rapid development of renewable energy while ensuring limited impacts to local communities and natural ecosystems in the process.
Different approaches have been previously proposed for automatic detection of PV arrays from very high-resolution satellite imagery using machine learning 10 , 11 , 12 . These approaches often rely on high resolution aerial imagery that is only freely available in the United States (with 1 m/px spatial resolution) and dense labels that are expensive to collect. This work shows that solar farm detection is feasible with lower resolution (10 m) imagery that is freely available worldwide and low-cost point. Other studies use geospatial variables including population demographics, housing characteristics to determine the variables that are predictive of photovoltaic (PV) energy adoption 10 . Concurrently to our work, Dunnett et al . published a global dataset for windmill and solar farm locations 30 relying on the solar farms/windmills being previously present in OpenStreetMap (OSM). Unfortunately, the methods that rely on surveys, OSM, and surrogate predictive variables are limited in completeness and scale. For example, Dunnett et al . includes 328 valid solar PV installations across India, our approach, on the other hand, is able to detect 1363 solar farms including 1035 never mapped before on OSM. Also, concurrently to our work, Kruitwagen et al . published a global inventory of solar installations using satellite imagery predictions 13 . This study included 372 solar PV installations across India, while missing many installations.
Six years ago, the international community finalized the Paris Agreement—an historic international climate change agreement—that included new commitments from all countries and outlined a set of rules for the global system over the coming years. The agreement sets out a system to track the progress of countries towards their targets—including principles defining “transparency and accountability” provisions. The dataset we have developed for India if expanded to other countries could be a simple and transparent mechanism to track progress on the deployment of solar energy that can help hold countries accountable to deliver on their climate targets.
Land-use and land-cover change is a pervasive, accelerating, and impactful process. Land-use and land-cover change is driven by human actions, and, in many cases, it also drives changes that impact humans. Understanding these patterns is critical for formulating effective environmental policies and management strategies. Because our dataset allows for both the identification of the spatial location of new solar development as well as the timing of that development, the dataset can be used in conjunction with land-use change models to better understand patterns of future change. Given the large land footprint associated with solar and onshore wind energy development there is potential for renewable energy expansion to involve the clearing of natural lands or fragmenting wildlife habitat and converting fertile agriculture land 8 . Our analysis of past land use change driven by solar development in India indicates almost 7% of development occurred within habitats important both for biodiversity and carbon storage i.e. evergreen, deciduous, and littoral swamp forest. In the face of climate change, which is likely to interact strongly with other stressors, biodiversity conservation and agriculture food security requires proactive adaptation strategies 31 . Renewable energy’s potential benefits to biodiversity from climate change mitigation will only be realized if development can prevent impacts to remaining natural habitat. Maintaining intact natural habitats and maintaining or improving the connectivity of land for the movement of both individuals and ecological processes, may provide the best opportunity for species and ecological systems to adapt to changing climate 32 .
On the other hand, the increasing demand for implementing renewable energy projects could put arable agriculture land under pressure 7 . Globally fertile arable land suitable for agriculture is limited owing to natural conditions and nature protection, and is threatened by processes like urbanization, demographic shift, and climate change 33 . Further, overtime the demand for land to implement renewable energy projects expected to impact productive agriculture lands. Therefore, the loss of productive agriculture land may lead to new dimensions of land use conflicts and provoke economic, ecological, political, and social conflict disruptions, and may encourage food-versus-energy controversy. Given that we observed that nearly two thirds of solar development was located in agricultural areas, avoiding conversion to productive agricultural land will be an important strategy for renewable energy deployment. Thus, guiding renewable energy development toward areas with lower conflict will be important. Understanding the factors associated with renewable energy development and predicting future expansion patterns will allow to proactively identify potential conflicts between renewable energy and other important land uses. The first step in this process is having access to data on the locations of solar installations that can be regularly updated.
Code availability
The dataset will be made publicly available for researchers, conservationist, policy makers, and solar developers to further explore conservation and solar energy development relationships, help inform policy decisions and minimize solar development effects in ecosystems at: https://researchlabwuopendata.blob.core.windows.net/solar-farms/solar_farms_india_2021.geojson .
Source code with our model architecture implementation, trained models accompany with instructions on how to use it is available on GitHub at: https://github.com/microsoft/solar-farms-mapping for anyone to use under MIT open-source license.
BloombergNEF, Emerging markets outlook 2020 https://global-climatescope.org/assets/data/reports/climatescope-2020-report-en.pdf (2020).
Central Electricity Authority (CEA), All India installed capacity (in MW) of power stations, March 2021 https://cea.nic.in/wp-content/uploads/installed/2021/03/installed_capacity.pdf (2021).
Central Electricity Authority (CEA), Report on optimal generation capacity mix for 2029-30 https://cea.nic.in/old/reports/others/planning/irp/Optimal_mix_report_2029-30_FINAL.pdf (2020).
Central Electricity Authority (CEA), All India installed capacity (in MW) of power stations March 2020 https://cea.nic.in/wp-content/uploads/2020/02/installed_capacity-03-1.pdf (2020).
India Renewable Power CEO Survey 2020 https://bridgetoindia.com/report/india-renewable-power-ceo-survey-2020 (2020).
Mohan, A. Whose land is it anyway? energy futures & land use in India. Energy Policy 110 , 257–262 (2017).
Article Google Scholar
Kiesecker, J. et al . Renewable energy and land use in India: A vision to facilitate sustainable development. Sustainability 12 , 281 (2020).
Kiesecker, J. M. & Naugle, D. E. Energy sprawl solutions: Balancing global development and conservation. Island Press (2017).
Kiesecker, J. et al . Hitting the target but missing the mark: unintended environmental consequences of the Paris climate agreement. Front. Environ. Sci. 7 , 151 (2019).
Google Scholar
Davidson, C., Drury, E., Lopez, A., Elmore, R. & Margolis, R. Modeling photovoltaic diffusion: an analysis of geospatial datasets. Environ. Res. Lett. 9 , 074009 (2014).
Article ADS Google Scholar
Yu, J., Wang, Z., Majumdar, A. & Rajagopal, R. Deepsolar: A machine learning framework to efficiently construct a solar deployment database in the United States. Joule 2 , 2605–2617 (2018).
Malof, J. M., Bradbury, K., Collins, L. M. & Newell, R. G. Automatic detection of solar photovoltaic arrays in high resolution aerial imagery. Appl. energy 183 , 229–240 (2016).
Kruitwagen, L. et al . A global inventory of photovoltaic solar energy generating units. Nature 598 , 604–610 (2021).
Article ADS CAS Google Scholar
Zhou, Z.-H. A brief introduction to weakly supervised learning. Natl. science review 5 , 44–53 (2018).
National Remote Rensing Centre, ISRO, Land use land cover(250k), government of India, Hyderabad, India https://bhuvan-app1.nrsc.gov.in/thematic/thematic/index.php (2017).
Long, J., Shelhamer, E. & Darrell, T. Fully convolutional networks for semantic segmentation. In Proceedings of the IEEE Conference on Computer Vision and Pattern Recognition , 3431–3440 (2015).
Ortiz, A. et al . Integrated learning and feature selection for deep neural networks in multispectral images. In Proceedings of the IEEE Conference on Computer Vision and Pattern Recognition Workshops , 1196–1205 (2018).
Robinson, C. et al . Human-machine collaboration for fast land cover mapping. In Proceedings of the AAAI Conference on Artificial Intelligence , vol. 34, 2509–2517 (2020).
Ronneberger, O., Fischer, P. & Brox, T. U-Net: Convolutional networks for biomedical image segmentation. In International Conference on Medical image computing and computer-assisted intervention , 234–241 (2015).
Kingma, D. P. & Ba, J. Adam: A method for stochastic optimization. Preprint at https://arxiv.org/abs/1412.6980 (2014).
Jin, S. et al . Unsupervised hard example mining from videos for improved object detection. In Proceedings of the European Conference on Computer Vision (ECCV) , 307–324 (2018).
Zhao, Z.-Q., Zheng, P., Xu, S.-T. & Wu, X. Object detection with deep learning: A review. IEEE transactions on neural networks learning systems 30 , 3212–3232 (2019).
Wan, S., Chen, Z., Zhang, T., Zhang, B. & Wong, K.-K. Bootstrapping face detection with hard negative examples. Preprint at https://arxiv.org/abs/1608.02236 (2016).
OpenStreetMap contributors. Planet dump retrieved from https://planet.osm.org . https://www.openstreetmap.org (2017).
Hall, D. K. & Riggs, G. A. Normalized-Difference Snow Index (NDSI). Springer Netherlands, 779–780 (2011).
Gao, B.-C. NDWI—a normalized difference water index for remote sensing of vegetation liquid water from space. Remote Sensing of Environment 58 , 257–266 (1996).
Robinson, C., Ortiz, A., Ferres, J. M. L., Anderson, B. & Ho, D. E. Temporal cluster matching for change detection of structures from satellite imagery. In Proceedings of the 4th ACM SIGCAS Conference on Computing and Sustainable Societies (2021).
Ortiz, A. et al . An Artificial Intelligence Dataset for Solar Energy Locations in India. Zenodo https://doi.org/10.5281/zenodo.5842519 (2022).
Shapira, D., Avidan, S. & Hel-Or, Y. Multiple histogram matching. In 2013 IEEE International Conference on Image Processing , 2269–2273 (2013).
Dunnett, S., Sorichetta, A., Taylor, G. & Eigenbrod, F. Harmonised global datasets of wind and solar farm locations and power. Sci. data 7 , 1–12 (2020).
Mawdsley, J. R., O’malley, R. & Ojima, D. S. A review of climate-change adaptation strategies for wildlife management and biodiversity conservation. Conserv. Biol. 23 , 1080–1089 (2009).
Anderson, M. G. & Ferree, C. E. Conserving the stage: climate change and the geophysical underpinnings of species diversity. PloS one 5 , e11554 (2010).
Ketzer, D. Land Use Conflicts between Agriculture and Energy Production: Systems Approaches to Allocate Potentials for Bioenergy and Agrophotovoltaics. Ph.D. thesis, Department of Physical Geography, Stockholm University (2020).
Download references
Acknowledgements
We thank MacArthur Foundation of India, the Microsoft AI for Good initiative, and The Cynthia and George Mitchell Foundation for Funding Support.
Author information
Authors and affiliations.
Microsoft AI for Good Research Lab, Redmond, WA, USA
Anthony Ortiz, Caleb Robinson, Jane Wang, Felipe Oviedo & Juan Lavista Ferres
Forum for the Future, New Delhi, India
Dhaval Negandhi
The Nature of Conservancy (TNC), New Delhi, India
Sagar R. Mysorekar, Shivaprakash K. Nagaraju, Joseph Kiesecker, Priyal Bhatia & Aditi Khurana
You can also search for this author in PubMed Google Scholar
Contributions
A.O., D.N., S.M., J.K. and J.L.F. conceived the experiment(s), A.O., S.M. and C.R. conducted the experiment(s), A.O., D.N., J.K. and J.L.F. analyzed the results. P.B. and S.M. performed the manual technical validation of results. All authors reviewed the manuscript.
Corresponding authors
Correspondence to Anthony Ortiz or Juan Lavista Ferres .
Ethics declarations
Competing interests.
The authors declare no competing interests.
Additional information
Publisher’s note Springer Nature remains neutral with regard to jurisdictional claims in published maps and institutional affiliations.
Rights and permissions
Open Access This article is licensed under a Creative Commons Attribution 4.0 International License, which permits use, sharing, adaptation, distribution and reproduction in any medium or format, as long as you give appropriate credit to the original author(s) and the source, provide a link to the Creative Commons license, and indicate if changes were made. The images or other third party material in this article are included in the article’s Creative Commons license, unless indicated otherwise in a credit line to the material. If material is not included in the article’s Creative Commons license and your intended use is not permitted by statutory regulation or exceeds the permitted use, you will need to obtain permission directly from the copyright holder. To view a copy of this license, visit http://creativecommons.org/licenses/by/4.0/ .
Reprints and permissions
About this article
Cite this article.
Ortiz, A., Negandhi, D., Mysorekar, S.R. et al. An Artificial Intelligence Dataset for Solar Energy Locations in India. Sci Data 9 , 497 (2022). https://doi.org/10.1038/s41597-022-01499-9
Download citation
Received : 18 January 2022
Accepted : 23 June 2022
Published : 16 August 2022
DOI : https://doi.org/10.1038/s41597-022-01499-9
Share this article
Anyone you share the following link with will be able to read this content:
Sorry, a shareable link is not currently available for this article.
Provided by the Springer Nature SharedIt content-sharing initiative
Quick links
- Explore articles by subject
- Guide to authors
- Editorial policies
Sign up for the Nature Briefing newsletter — what matters in science, free to your inbox daily.

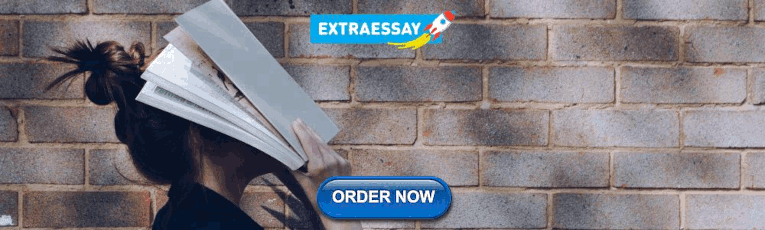
To read this content please select one of the options below:
Please note you do not have access to teaching notes, adoption of solar energy in india: a study through interpretive structural modelling.
World Journal of Science, Technology and Sustainable Development
ISSN : 2042-5945
Article publication date: 13 July 2021
Issue publication date: 15 November 2021
Adoption of solar energy plays an important role in the growth of a country. There are many factors which influence the adoption of solar energy in India. The study is designed to identify factors that determine the acceptance or rejection of solar energy systems in India.
Design/methodology/approach
Relationship among identified variables is established through interpretive structural modelling (ISM) and thus a conceptually validated model is evolved. Further, MICMAC analysis is conducted to understand the driving power and dependence of these variables.
It is revealed that experience and habit, awareness and social influence are the intermediary variables. MICMAC Analysis shows that no variable is disconnected from the system and all the variables influence the adoption of solar energy in India.
Practical implications
The present study is expected to be useful to decision makers, end users and research organisations related to solar energy adoption.
Originality/value
Various intentional factors influencing solar energy systems adoption have been acknowledged in the present study, thus making it useful for formulation of action plans and enhance the usage of solar energy systems to improve environment quality.
- Solar energy
Acknowledgements
The authors of the study would like to give their sincere thanks to Impactful Policy Research in Social Science (IMPRESS) and Indian Council of Social Science Research (ICSSR) for funding the research and allowing us to know about the adoption level of solar energy systems in India.
Sahu, G.P. , Singh, P. and Dwivedi, P. (2021), "Adoption of solar energy in India: a study through interpretive structural modelling", World Journal of Science, Technology and Sustainable Development , Vol. 18 No. 4, pp. 457-473. https://doi.org/10.1108/WJSTSD-04-2021-0043
Emerald Publishing Limited
Copyright © 2021, Emerald Publishing Limited
Related articles
We’re listening — tell us what you think, something didn’t work….
Report bugs here
All feedback is valuable
Please share your general feedback
Join us on our journey
Platform update page.
Visit emeraldpublishing.com/platformupdate to discover the latest news and updates
Questions & More Information
Answers to the most commonly asked questions here
Solar energy policy of India: An overview
Ieee account.
- Change Username/Password
- Update Address
Purchase Details
- Payment Options
- Order History
- View Purchased Documents
Profile Information
- Communications Preferences
- Profession and Education
- Technical Interests
- US & Canada: +1 800 678 4333
- Worldwide: +1 732 981 0060
- Contact & Support
- About IEEE Xplore
- Accessibility
- Terms of Use
- Nondiscrimination Policy
- Privacy & Opting Out of Cookies
A not-for-profit organization, IEEE is the world's largest technical professional organization dedicated to advancing technology for the benefit of humanity. © Copyright 2024 IEEE - All rights reserved. Use of this web site signifies your agreement to the terms and conditions.

Home » Magazine Exclusive » COVER STORY: India Gears Up For Solar Waste Recycling Challenge
COVER STORY: India Gears Up For Solar Waste Recycling Challenge

Highlights :
- As Solar Capacity Ramps Up In India, Recycling Emerges On The Horizon As a Critical Need.
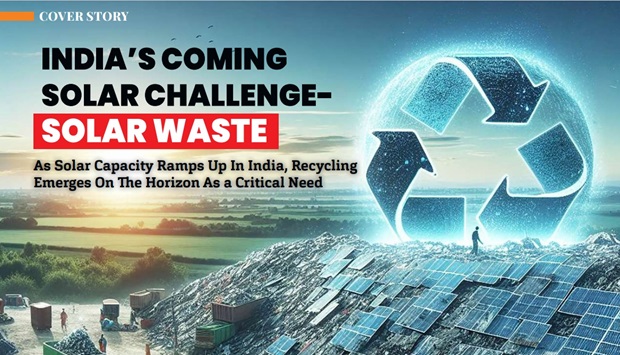
The latest report from the Central Electricity Authority (CEA) states that India’s total solar energy capacity crossed 75 Gigawatts (GW) by the end of February this year. According to its own estimates, the country will need 292 GW of total solar capacity by 2030.
With the steady additions of solar power to the Indian grid, the South Asian country is also adding something that it would not like to cherish– a million tonnes of anticipated solar waste. Linked directly to the total projected lifetime of most solar installations at 25 years, the waste issue is likely to be accelerated by the premature retirement of many modules to be replaced with higher efficiency modules in time. The global solar recycling market, estimated at just around $250 million this year, is expected to grow to be a $2 billion plus market in very quick time, perhaps as early as 2032, depending on price movements in critical minerals used.
The heightened pace of solar deployments worldwide and in India certainly point to a market that will be vital to burnishing solar’s green credentials in the next few years.
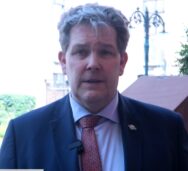
Jan Clyncke
This volume of waste set to be generated at the end of their lives will be further augmented due to damage owing to extreme weather events, transportation, manufacturing, or other sources. Jan Clyncke , Chief Executive Officer (CEO) of PV Cycle, a European not-for-profit venture involved in solar module recycling globally, told Saur Energy even the 73 GW of solar capacity alone could lead to the generation of a massive 4.5 million tonnes of solar waste. This quantum of solar PV is likely to be a reality at the end of their lives.
New Delhi–based think tank, the Council on Energy, Environment and Water (CEEW), recently released a report on solar waste on March 20 this year. The report said that India’s installed 66.7 GW capacity, as of FY23, generated about 100 kilotonnes (kt) of waste, which will increase to 340 kt by 2030. It also said that India’s cumulative solar waste will increase 32 times between 2030 and 2050, hinting at the scale of waste created by the renewable energy source.
The report also said that around 67 percent of this waste is expected to be generated in five renewable-rich states of India–Rajasthan, Gujarat, Karnataka, Andhra Pradesh, and Tamil Nadu. Before CEEW, IRENA (International Renewable Energy Agency), NSEFI (National Solar Energy Federation of India) and CSTEP also came up with their own projections of the likely generation of solar waste in India under different circumstances.
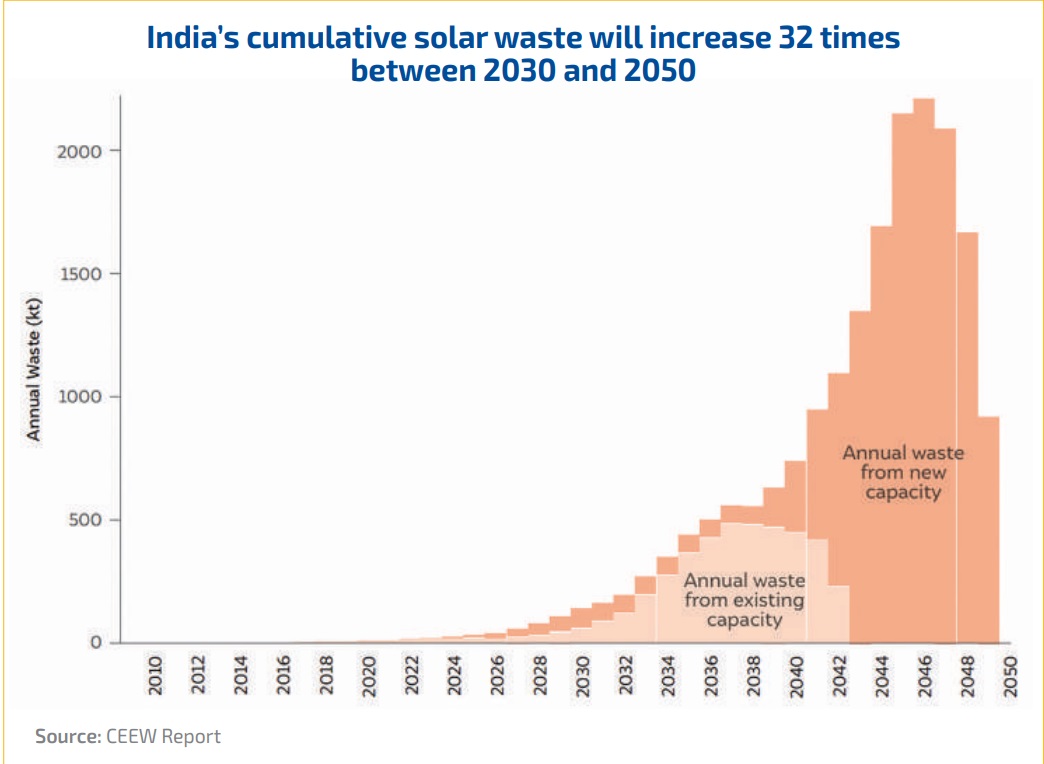
Solar Waste & Challenges
Unlike other sources of waste with little economic value attached, new age green materials like discarded solar panels or solar waste possess several items of economic value, including critical minerals like Silicon, Copper, and others, as well as other components used to make solar panels. In this, they join another key component of the green transition, Lithium Ion batteries. But where Lithium batteries are already looking at recycling levels of over 95%, solar modules have some way to go.A solar PV module (crystalline silicon) primarily comprises Glass (around 74%). Other items include Aluminum, Silicon, Copper, Tin, Silver, Zinc, Lead, Tedlar (back sheet), and Encapsulant. As per the Critical Mineral List of the Indian Ministry of Mines, components like Silicon, Copper, Tellurium, and Cadmium are considered critical minerals.
The sector is currently witnessing several challenges, ranging from the large involvement of the informal sector to technological and infrastructural hurdles, funding, awareness, and much more. This is in addition to the lack of authentic data, monitoring, and regulation of the whole sector in the absence of stringent rules and regulations for the sector.
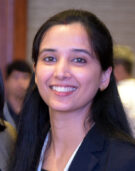
Akanksha Tyagi
Akanksha Tyagi , Co-author of the latest CEEW report on solar waste and Programme Lead at the organisation, told Saur Energy that limited efficient recycling technologies that are able to recover all module components at the highest purity grades are a challenge, which can be addressed by expediting technological innovations.
She said incentives for recycling when the economics are unfavourable would help recyclers initiate solar module recycling. “Additionally, there is a need to address the low economy of scale for solar waste recycling by demand aggregation from various sources. Aggregating the waste modules shall help to achieve the critical mass. This will improve the economics for pilot demonstrations and thereby generate India-specific data on waste management parameters. Here, the manufacturers can combine efforts to collect waste and channel it to recyclers to achieve manageable volumes,” Tyagi told Saur Energy.
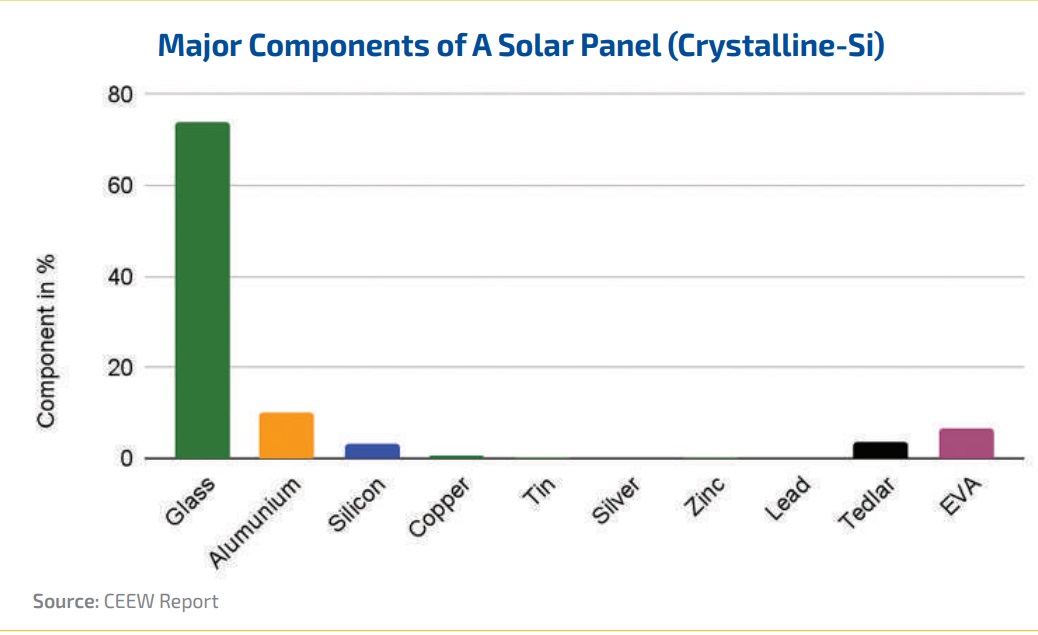
India’s Policy Preparedness
Globally, the European Union (EU) seems to be one of the pioneers in taking concrete steps towards planning to focus on recycling solar waste. In 2012, the EU developed a legislation– Waste Electrical and Electronic Equipment (WEEE) . The law mandates collecting and treating end-of-life solar modules and their recycling. It also puts the onus on solar module manufacturers, importers, and rebranders to comply with these rules with its Extended Producer Responsibility (EPR) provisions.
The Indian government has also started taking steps in this direction. In 2022, the Ministry of New and Renewable Energy (MNRE) submitted its detailed report on the circular economy in solar panels to the NITI Aayog. The same year, the Ministry of Environment, Forest and Climate Change (MoEFCC) released its E-Waste Management Rules 2022 , which included solar PV modules and cells under its ambit. These rules came into effect on April 1, 2023.
The rules mandate that solar module manufacturers and recyclers register with a central portal of the Central Pollution Control Board (CPCB) and file their annual returns. The module makers were also asked to store solar module or cell waste generated upto 2034-35 These rules also asked the module makers to put the details of the inventory of their solar modules or cells on the portal.
As per MoEFCC, India will get its first-ever detailed report from the solar module makers on their total module sales and replacement by the end of June this year. This is likely to bridge the data gap for the sector at the national and granular levels.
“Soon, the Central Pollution Control Board’s portal for Electronic waste (EPR) will provide data on annual module sales and modules replaced. It remains to be seen if this data will be available for the public or only for registered entities. However, this data will be presented at an aggregated level (for a solar cell/module producer), so we need more granular data like the data on how many modules are being replaced and for which activity under each project (commissioned/under construction),” CEEW’s Tyagi added.
These e-waste rules of 2022, however, have put the onus on the solar module manufacturers for the scientific disposal and management of the solar waste they created. However, little detail and a lack of a clear pathway for solar waste recycling remain absent. A senior official from the MoEFCC said that until now, the ministry has not put the liability of EPR targets for solar module manufacturers under the e-waste rules as there is not enough solar waste available for recycling and it will take some time to make the solar recycling business a viable business model.
Bangalore-based think tank Center for Study of Science, Technology and Policy (CSTEP) published a white paper last year on solar recycling. The research paper, which studied several norms, standards, and laws globally, said that more provisions were needed in the policy to boost the circular economy in the solar industry in India.
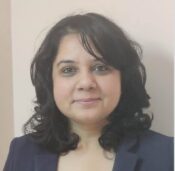
Anjali Taneja
Anjali Taneja , Senior Policy Specialist leading the Sustainability vertical at CSTEP and the author of the white paper, told Saur Energy, “While the handling of photovoltaic (PV) waste has been brought under the e-waste guidelines, what could perhaps be considered more effective is to have a special provision for PV waste under the e-waste guidelines to segregate PV waste management from the overall e-waste handling.”
She also added, “Our research, which also included a series of stakeholder consultations, found that having certain mechanisms such as recycling benchmarks, regulation of a waste disposal fee, a waste monitoring and tracking system, and continuous engagements between the government, industry, and civil society could prove to be beneficial for a comprehensive solar waste recycling policy.”
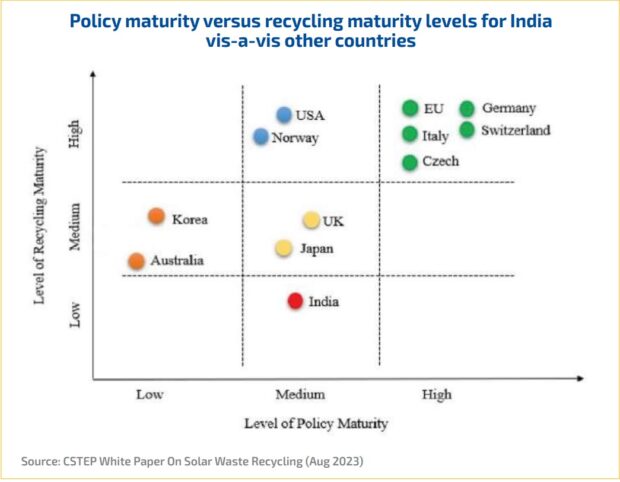
Industry preparedness
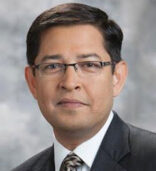
Sujoy Ghosh
The solar industry is also slowly preparing to pave the way for more scientific recycling of solar panels so that they can either be reused as solar panels again or the extracted components of economic importance could be used in some industries.
For example, global solar module manufacturer First Solar sends the extracted polyolefins from the modules to be used to produce footwear. It also re-uses the CdTeSe semiconductor material extracted from EOL (end of life) modules in manufacturing new modules.
Sujoy Ghosh , Country Managing Director (India) of First Solar, said that to make the recycling business more viable, the industry needs to diversify the end use of the extracted items from the solar panels because reusing glass for new solar panels would be challenging and a costlier affair. He also said that refurbishing older solar panels would not be attractive for the solar market in the future. “I do not believe refurbishing solar panels for the future would be a good idea from a technology point of view because, with time, technologies are becoming more efficient, thereby lowering the cost/watt produced for new modules So, refurbished modules with low efficiency polycrystalline may not be competitive against new modules,” Ghosh said.
Ghosh also talked about the costs involved in solar cycling globally. He said the current regulated solar panel recycling fee is around 1.63 Euros per module in France, excluding cost of logistics. He said that in the overall recycling value chain logistics account for 50-60%
Additional cost dilemma
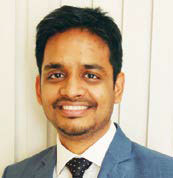
Vineet Mittal
Navitas Solar is a Surat-based solar module manufacturer. It has collaborated with Green World Sourcing Solutions for waste disposal. This local e-waste aggregator takes solar waste from the firm and supplies it to another recycling firm.
Vineet Mittal , Co-Founder at Navitas Solar, told Saur Energy that there are a lot of issues concerned with the additional cost burden for solar module manufacturers when it comes to solar module recycling. He said that the new segment puts the onus on the module makers. Mittal said that financing solar recycling needed a well-thought-out strategy that could allay the fears of Indian solar makers over the additional burden beyond manufacturing modules.
“Several solar cells and modules are still imported from countries like China to India. I believe if more policies towards these are made, special focus should be given to allow a level playing field for Indian solar module makers. The total responsibility for its recycling to Indian module makers may put an extra financial burden on them. This could be negated with some government-initiated reforms like imposing additional tax or cess in imports of modules in India to take care of the extra financial burden for recycling,” Mittal told Saur Energy.
Mirunalini Chellapan , Director of Swelect Energy Systems, another Indian solar module maker, suggests that the additional finances for solar recycling could also be taken care of by imposing a cess or tax in ‘frontloading’ when the modules are sold to the developers. She said the tax component imposed at this trading level can negate the additional financial burden.
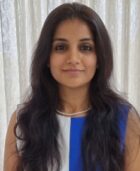
Mirunalini Chellapan
She also suggested other ways to boost the circularity of solar modules in India. She told Saur Energy, “First of all, solar waste should be treated differently from e-waste, and a separate category of renewable waste should be created. Second, dumping solar modules in landfills should be strictly penalised. Thirdly, the government can also count on the recycling cost and mention it in their tenders to take care of the segment from the initial phase itself in large government projects.”
Prashanth Mathur , CEO of Saatvik Solar told Saur Energy that all solar panels do not become a waste product till the end of their lives, but some, either due to damage or low efficiency, get replaced even in below the period, leading to premature solar waste generation.
He also raised the issue of the need to focus on the financials for solar recycling: “Somebody has to pay for this. This could come either in the form of an incentive, tax, cess, or something else. Maybe we can make such an arrangement where the consumer can also pay if such arrangements are made in the trade system.”
Mathur said that his firm has already started working with European firm PV Cycle to ensure the recycling of its solar waste. “We started working with them for the last three years. Under the arrangement, they take the waste and manage it with their expertise at their end. We did it voluntarily to shift our focus towards circularity.”
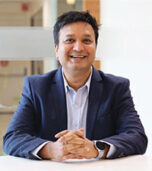
Prashant Mathur
Due to the anticipation of a lower scale of solar waste in India, recycling systems have mostly remained on a pilot scale. For example, the Maharashtra Institute of Technology – World Peace University, Pune experimented with using crushed solar panels as a replacement for sand for use with concrete in building construction as part of a pilot project. Experts claim that opportunities do exist in the recycling segment in India, too, but they demand a focus on research and development.
“Finance-related challenges in the recycling of solar panel waste could be sorted through better incentives and schemes for investment-building and research and development by leveraging public–private partnerships and perhaps by offering attractive pricing mechanisms for manufacturers and producers handling PV waste. Moreover, market-based schemes to encourage the start-up industry in PV waste management could prove to be beneficial. In other words, a conducive business environment and various support mechanisms can help alleviate financial barriers, particularly during the initial phases. When managing end-of-life (EoL) waste,” CSTEP’s Anjali Taneja added.
PV Cycle, a European firm, works as a Producer Responsibility Organization (PRO) involved in collection, monitoring and auditing. It has collaborated with several Indian firms, too.
Jan Clyncke, CEO of PY Cycle, told Saur Energy that there is no technical dearth for recycling of solar waste but the problem mainly lies currently on the scale of supply of solar waste, which can decide on the fate of new recycling firms and their business models.
When asked about the important parameters that could be part of any policy in India towards solar recycling, Jan said, “It should have standards for collection and targets which can bolster the confidence of new firms who want to join the recycling industry with some innovative ideas.”
A senior official from the MNRE told Saur Energy that MoEFCC is likely to develop a new policy framework for solar module recycling by 2024. MNRE is also holding talks with different stakeholders on the issue and may come up with some offerings anytime after 2024.
While the Indian government has taken steps to start framing policies, many questions remain unanswered for the Indian solar module makers. Some of these include clarity on the fate of solar panels if the solar module firm suffers insolvency before the end of their lives and the fate of modules used in projects that saw acquisitions or witnessed equity transfers.
Financing is another major concern for solar module makers, and they need clarity to plan their roadmap. On the other hand, many e-waste and other recyclers in the country have also explored opportunities in the sector. They are waiting for clear answers, technology and support to jump onto the bandwagon. A more clear-cut, target-based, tailor-made solar recycling for India is needed when India is emerging as one of the major solar market leaders globally. What is clear is that whatever be the policy, the economics of the business mean that multiple sites close to major installation clusters will be needed, along with incentives as well as a compliance framework that ensures that the country is ready for the first wave of discarded panels that will start building up from 2030 or before.
Recent NEWS
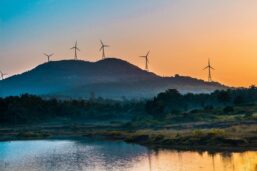
Recent IN Talks
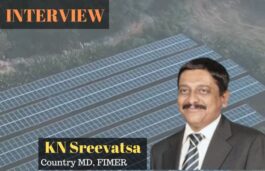
Interview
- FIMER’s Made In India Inverters Shine In ...
- Husk Power Plans To Commission More Mini-Grids In ...
- “CSH In India Is Best Suited For Heating ...
- “Our Nickel Recycling Plant Will Take ...
- “International Initiatives Such As CBAM And ...
- BatX’s Strong Network Aided In Boosting Battery ...
- ARENQ Working To Develop 3-Wheeler EVs With ...
- My Vote Goes To String PV Inverters Against ...
- “700+ Wp Modules Increase Generation By ...
- “The Cabling In Solar Systems Is Designed ...
- “We Are Targeting To Achieve Installation ...
- India Has An Opportunity In Solar Cell ...
Solar Products
- Microtek Expects 20%-40% Market Share Under PM ...
- Israeli Researchers Introduce AI-Designed Solar ...
- US Agri Startup Aigen Brings Solar Powered ...
- ChakraPaani: A ‘Solar Hand Pump’ Designed to ...
- Component Manufacturer BENY to Reveal Latest ...
- Best Power Equipments Unveils ESS Product- NRGX ...
- Solis Launches New Hybrid Inverter in South Africa
- India To Become World’s Second-Largest ...
- IIT Roorkee, GIC Re Collaborate to Develop Solar ...
- Solar Trackers Surge; Poised To Reach 37,196.86 ...
- Elves: Shinola Camping Accessory Set
- Seeon 180° backpack
April 2, 2024
Eclipse Psychology: When the Sun and Moon Align, So Do We
How a total solar eclipse creates connection, unity and caring among the people watching
By Katie Weeman
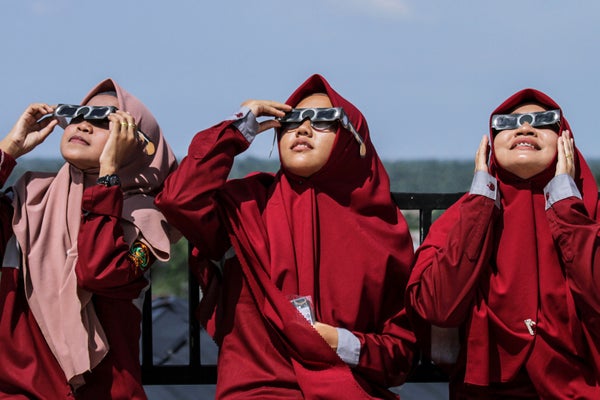
Students observing a partial solar eclipse on June 21, 2020, in Lhokseumawe, Aceh Province, Indonesia.
NurPhoto/Getty Images
This article is part of a special report on the total solar eclipse that will be visible from parts of the U.S., Mexico and Canada on April 8, 2024.
It was 11:45 A.M. on August 21, 2017. I was in a grassy field in Glendo, Wyo., where I was surrounded by strangers turned friends, more than I could count—and far more people than had ever flocked to this town, population 210 or so. Golden sunlight blanketed thousands of cars parked in haphazard rows all over the rolling hills. The shadows were quickly growing longer, the air was still, and all of our faces pointed to the sky. As the moon progressively covered the sun, the light melted away, the sky blackened, and the temperature dropped. At the moment of totality, when the moon completely covered the sun , some people around me suddenly gasped. Some cheered; some cried; others laughed in disbelief.
Exactly 53 minutes later, in a downtown park in Greenville, S.C., the person who edited this story and the many individuals around him reacted in exactly the same ways.
On supporting science journalism
If you're enjoying this article, consider supporting our award-winning journalism by subscribing . By purchasing a subscription you are helping to ensure the future of impactful stories about the discoveries and ideas shaping our world today.
When a total solar eclipse descends—as one will across Mexico, the U.S. and Canada on April 8—everyone and everything in the path of totality are engulfed by deep shadow. Unlike the New Year’s Eve countdown that lurches across the globe one blocky time zone after another, the shadow of totality is a dark spot on Earth that measures about 100 miles wide and cruises steadily along a path, covering several thousand miles in four to five hours. The human experiences along that path are not isolated events any more than individual dominoes are isolated pillars in a formation. Once that first domino is tipped, we are all linked into something bigger—and unstoppable. We all experience the momentum and the awe together.
When this phenomenon progresses from Mexico through Texas, the Great Lakes and Canada on April 8, many observers will describe the event as life-changing, well beyond expectations. “You feel a sense of wrongness in those moments before totality , when your surroundings change so rapidly,” says Kate Russo, an author, psychologist and eclipse chaser. “Our initial response is to ask ourselves, ‘Is this an opportunity or a threat?’ When the light changes and the temperature drops, that triggers primal fear. When we have that threat response, our whole body is tuned in to taking in as much information as possible.”
Russo, who has witnessed 13 total eclipses and counting, has interviewed eclipse viewers from around the world. She continues to notice the same emotions felt by all. They begin with that sense of wrongness and primal fear as totality approaches. When totality starts, we feel powerful awe and connection to the world around us. A sense of euphoria develops as we continue watching, and when it’s over, we have a strong desire to seek out the next eclipse.
“The awe we feel during a total eclipse makes us think outside our sense of self. It makes you more attuned to things outside of you,” says Sean Goldy, a postdoctoral fellow at the department of psychiatry and behavioral sciences at Johns Hopkins University.
Goldy and his team analyzed Twitter data from nearly 2.9 million people during the 2017 total solar eclipse. They found that people within the path of totality were more likely to use not only language that expressed awe but also language that conveyed being unified and affiliated with others. That meant using more “we” words (“us” instead of “me”) and more humble words (“maybe” instead of “always”).
“During an eclipse, people have a broader, more collective focus,” Goldy says. “We also found that the more people expressed awe, the more likely they were to use those ‘we’ words, indicating that people who experience this emotion feel more connected with others.”
This connectivity ties into a sociological concept known as “collective effervescence,” Russo and Goldy say. When groups of humans come together over a shared experience, the energy is greater than the sum of its parts. If you’ve ever been to a large concert or sporting event, you’ve felt the electricity generated by a hive of humans. It magnifies our emotions.
I felt exactly that unified feeling in the open field in Glendo, as if thousands of us were breathing as one. But that’s not the only way people can experience a total eclipse.
During the 2008 total eclipse in Mongolia “I was up on a peak,” Russo recounts. “I was with only my husband and a close friend. We had left the rest of our 25-person tour group at the bottom of the hill. From that vantage point, when the shadow came sweeping in, there was not one man-made thing I could see: no power lines, no buildings or structures. Nothing tethered me to time: It could have been thousands of years ago or long into the future. In that moment, it was as if time didn’t exist.”
Giving us the ability to unhitch ourselves from time—to stop dwelling on time is a unique superpower of a total eclipse. In Russo’s work as a clinical psychologist, she notices patterns in our modern-day mentality. “People with anxiety tend to spend a lot of time in the future. And people with depression spend a lot of time in the past,” she says. An eclipse, time and time again, has the ability to snap us back into the present, at least for a few minutes. “And when you’re less anxious and worried, it opens you up to be more attuned to other people, feel more connected, care for others and be more compassionate,” Goldy says.
Russo, who founded Being in the Shadow , an organization that provides information about total solar eclipses and organizes eclipse events around the world, has experienced this firsthand. Venue managers regularly tell her that eclipse crowds are among the most polite and humble: they follow the rules; they pick up their garbage—they care.
Eclipses remind us that we are part of something bigger, that we are connected with something vast. In the hours before and after totality you have to wear protective glasses to look at the sun, to prevent damage to your eyes. But during the brief time when the moon blocks the last of the sun’s rays, you can finally lower your glasses and look directly at the eclipse. It’s like making eye contact with the universe.
“In my practice, usually if someone says, ‘I feel insignificant,’ that’s a negative thing. But the meaning shifts during an eclipse,” Russo says. To feel insignificant in the moon’s shadow instead means that your sense of self shrinks, that your ego shrinks, she says.
The scale of our “big picture” often changes after witnessing the awe of totality, too. “When you zoom out—really zoom out—it blows away our differences,” Goldy says. When you sit in the shadow of a celestial rock blocking the light of a star 400 times its size that burns at 10,000 degrees Fahrenheit on its surface, suddenly that argument with your partner, that bill sitting on your counter or even the differences among people’s beliefs, origins or politics feel insignificant. When we shift our perspective, connection becomes boundless.
You don’t need to wait for the next eclipse to feel this way. As we travel through life, we lose our relationship with everyday awe. Remember what that feels like? It’s the way a dog looks at a treat or the way my toddler points to the “blue sky!” outside his car window in the middle of rush hour traffic. To find awe, we have to surrender our full attention to the beauty around us. During an eclipse, that comes easily. In everyday life, we may need to be more intentional.
“Totality kick-starts our ability to experience wonder,” Russo says. And with that kick start, maybe we can all use our wonderment faculties more—whether that means pausing for a moment during a morning walk, a hug or a random sunset on a Tuesday. In the continental U.S., we won’t experience another total eclipse until 2044. Let’s not wait until then to seek awe and connection.
How to protect your eyes during the 2024 solar eclipse
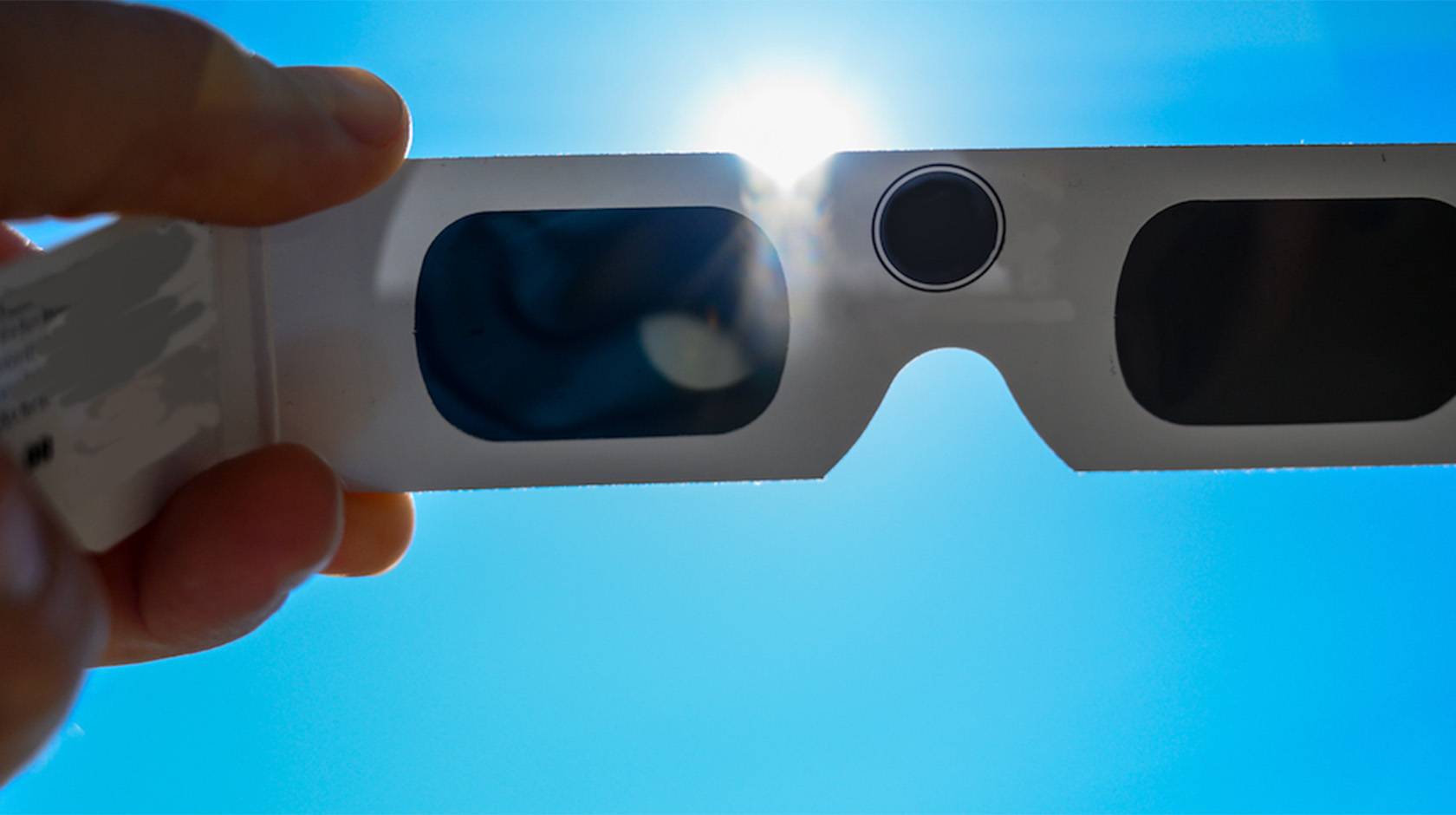
On April 8, millions of people across the United States will be tempted to stare at the sun as large areas of the country experience a total or partial solar eclipse . A solar eclipse is when the moon blocks, or partially blocks, the sun, casting a shadow on the earth.
But staring at the sun without eye protection — even if it’s partially blocked by the moon — can lead to vision loss.
The bad news is that there is no treatment for sun damage in the eye once it has occurred, according to Kareem Moussa , an ophthalmologist at the Ernest E. Tschannen Eye Institute at UC Davis Health.
“The damage can start to occur in less than a minute of staring at the sun, and it may not be noticeable until hours later,” Moussa said.
Moussa is an expert on the human retina. He explained that the eye focuses light onto the retina , the area at the back of the eye. The retina transmits signals from the eye to the brain through the optic nerve, allowing us to perceive images of the world.
“A functioning retina is required for good vision. Staring at the sun causes toxicity, or damage, to some of the cells in the retina. When these cells are damaged, the normal flow of information from the retina to the brain is interrupted,” Moussa explained.
Staring at the sun any time — not just during an eclipse — can lead to eye damage. Moussa has treated patients with sun-damaged retinas.
“Typically, the damage will become noticeable over the following 12 hours after staring at the sun and persists for three to four months. Usually, this leads to a blind spot in the middle of one’s vision or an area of distorted vision. The impact on vision can be severe,” Moussa said.
People can also experience objects looking crooked when they should be straight or things looking bigger or smaller than they should be. If someone is having any of these symptoms, they should seek an evaluation from an ophthalmologist.
Moussa notes that although anyone can develop severe damage from looking at the sun, kids are even more vulnerable. That’s because they have very clear lenses in their eyes, compared to adults, which let in more light. “As we get older, the lens inside the eye gradually becomes cloudier,” Moussa explained. “Younger people also tend to have more dilated pupils, which lets more light into the eye, and can lead to more damage from staring at the sun.”
For people who experience sun damage to their eyes, there is usually some recovery over the following six months. But for some, the damage may be permanent, and vision does not return to normal.
This is why preventing the damage in the first place is so important.
No sunglasses, and beware of fake eclipse glasses
The first thing to know is sunglasses will NOT protect your eyes from looking at the eclipse.
“Some people mistakenly think putting on very dark sunglasses will be sufficient. It will not!” Moussa said.
Protective glasses for viewing an eclipse are thousands of times darker than sunglasses.
“Unless the glasses have a solar filter that meets the ISO 12312-2 standard , which is the international safety standard for protecting your eyes from the sun, you will be at risk of developing significant eye damage if you look at the eclipse in sunglasses,” said Moussa.
Unfortunately, counterfeit and fake eclipse glasses that look exactly like authentic ones are hitting the market . Using counterfeit glasses could lead to eye damage.
The American Astronomical Society (AAS) cautions consumers against searching for eclipse glasses on Amazon, eBay, Temu or any other online marketplace and buying from whichever vendor offers the lowest price.
AAS has posted a list of North American manufacturers that meet the ISO 12312-2 international standards, for solar eclipse glasses and handheld solar viewers and a guide to watching the eclipse safely .
NASA also has a useful guide on different ways to safely view the eclipse.
With the right eye protection, viewing the eclipse or partial eclipse can be a great experience. The upcoming eclipse in the U.S. will be a once-in-a-generation event: a full solar eclipse won’t happen in the U.S. again until August 2044.
“The solar eclipse is an exciting event,” Moussa said. “Enjoy it safely.”
Tips in brief
- Ordinary sunglasses, even very dark sunglasses, are NOT safe for looking at the sun
- Make sure eclipse glasses are from a vendor verified as selling glasses that meet ISO 12312-2 standards
- Eye damage from looking at the sun can happen in less than a minute
- Kids’ eyes can be even more vulnerable to sun damage
- Beware of fake and counterfeit eclipse glasses
- NASA: Eclipse Eye Safety
- Suppliers of Safe Solar Viewers and Filters
- Solar eclipse eye safety
Keep reading
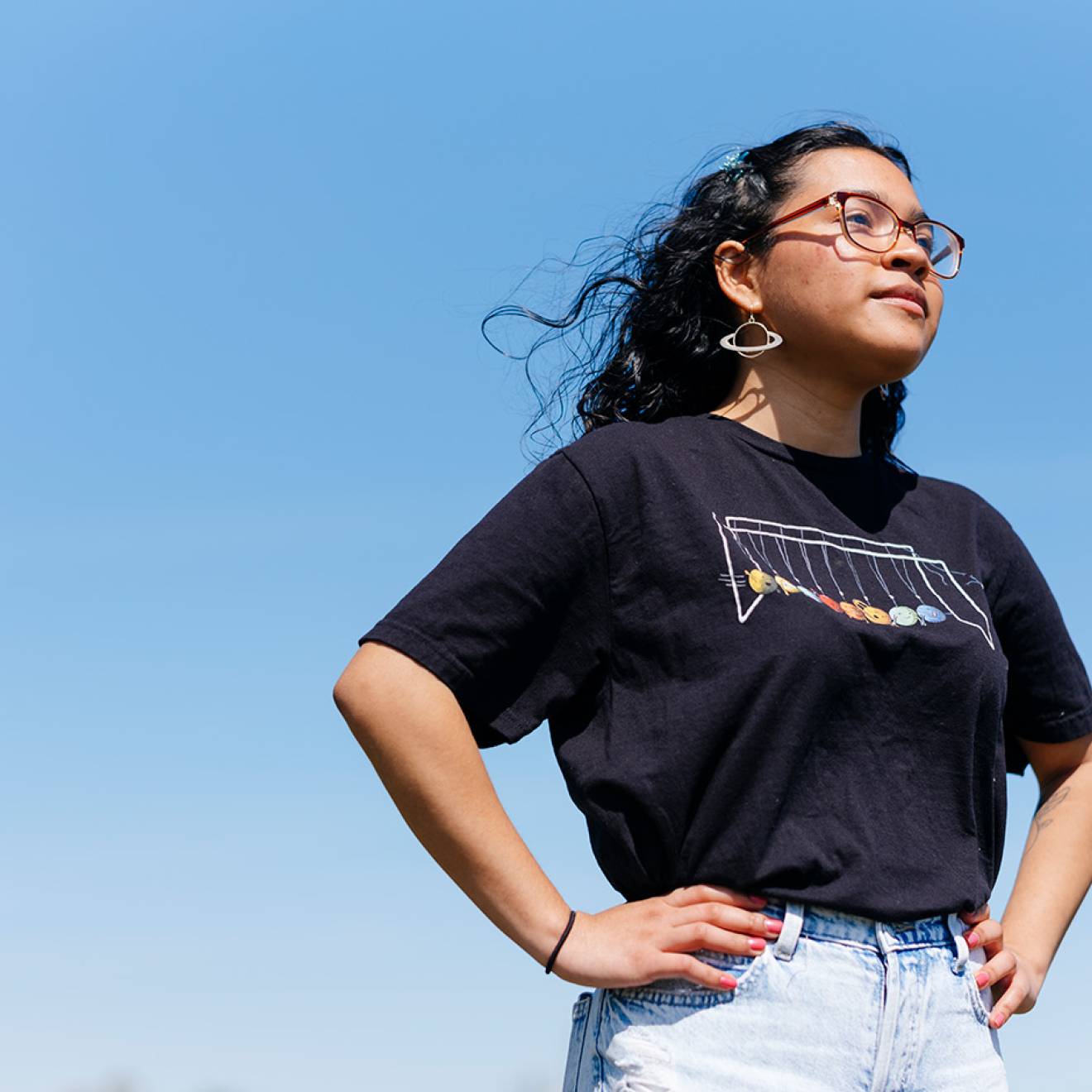
Coming of age in a warming world: How UC students are…
How do UC students think about their futures, knowing that the climate they were born into will probably look a whole lot different than the one they grow old in?
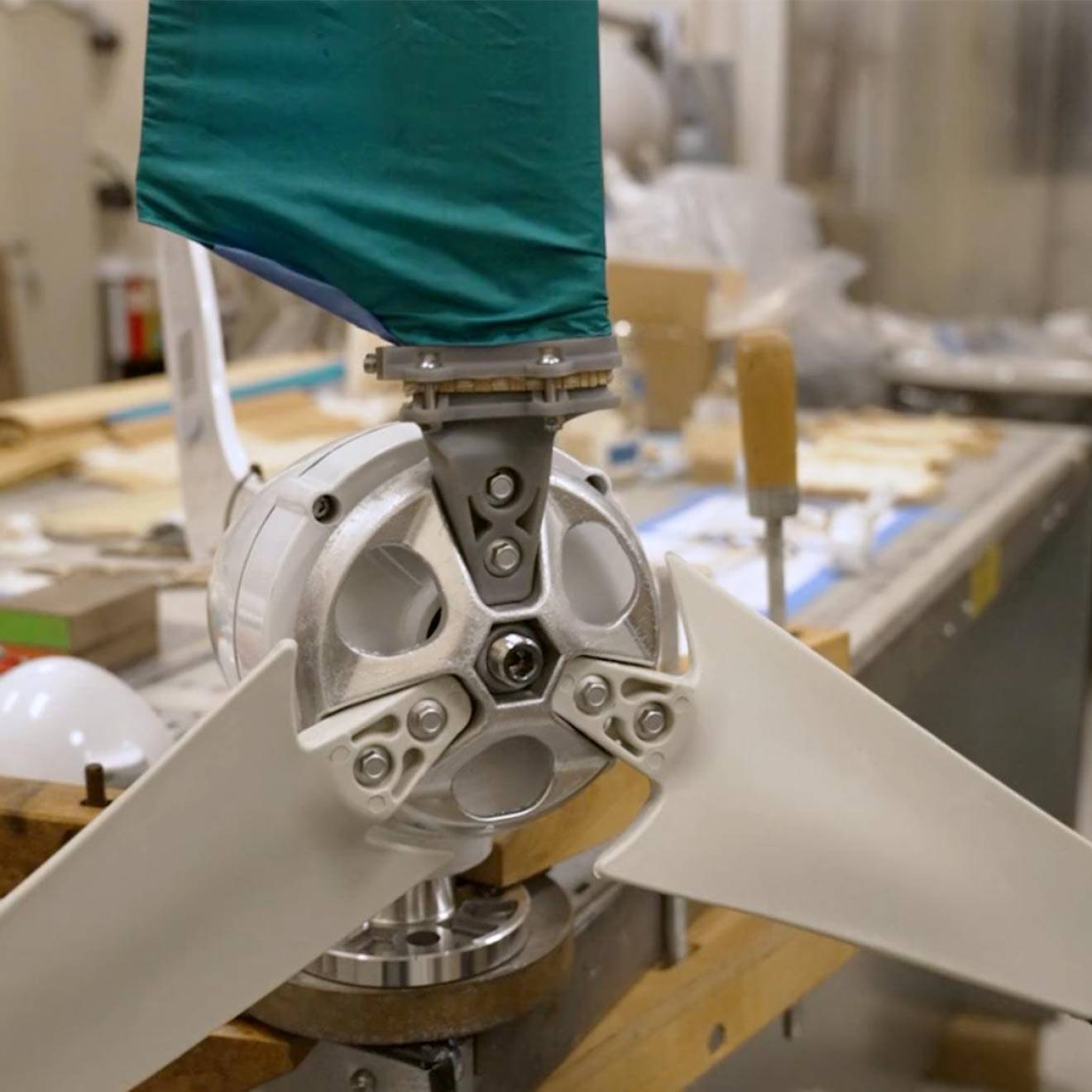
Making wind power even greener
Blades made from bamboo and mycelium could keep a growing number of wind blades out of landfills.
Current Status and Future Potential of Solar Energy Utilization in Rajasthan, India
- Conference paper
- First Online: 09 November 2022
- Cite this conference paper
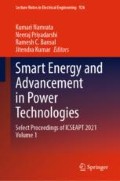
- Debajit Misra 41
Part of the book series: Lecture Notes in Electrical Engineering ((LNEE,volume 926))
422 Accesses
Rajasthan requires a huge amount of power due to its own demand and to supply its nearby areas. High solar radiation and plenty of unoccupied land make the state in a position to run a variety of solar power plants and equipment. This paper describes the main ways of generating solar electricity in the state, like using solar photovoltaic (PV), concentrated solar power (CSP). The paper also highlights different solar schemes, and large numbers of solar projects have already been installed at different locations. Currently, Rajasthan possesses the world’s largest solar park of 2245 MW capacity, named Bhadla Solar Park. Presently, total installed power capacity of Rajasthan is about 22.15 GW, while its total installed solar power capacity stands at 5.3 GW. At present, its share is about 15% of the total Indian solar installation. The state has already developed transmission and distribution network to supply power in the desert area. A strong power evacuation system is being developed, which could transmit 8000 MW of renewable energy to the state and national grid with the help of the Indian Solar Mission, the Asian Development Bank and the Clean Technology Fund. The state is involved in an aggregate solar power potential of 142.31 GW. Thus, in future, Rajasthan could be in a notable position towards solar energy deployment in India. The paper illustrates the current scenario, future potential and different routes of solar thermal energy utilization in Rajasthan.
- Solar energy
- Solar plant
This is a preview of subscription content, log in via an institution to check access.
Access this chapter
- Available as PDF
- Read on any device
- Instant download
- Own it forever
- Available as EPUB and PDF
- Compact, lightweight edition
- Dispatched in 3 to 5 business days
- Free shipping worldwide - see info
- Durable hardcover edition
Tax calculation will be finalised at checkout
Purchases are for personal use only
Institutional subscriptions
Misra D (2020) Floating photovoltaic plant in India: current status and future prospect. In: International conference on thermal engineering and management advances 2020, LNME. Springer, Singapore, pp 219–232
Google Scholar
Misra D (2019) Design of a stand-alone rooftop PV system for electrification of an academic building. Int J Eng Adv Technol 9(2):3955–3964
Article Google Scholar
Ganguly A, Misra D, Ghosh S (2010) Modeling and analysis of solar photovoltaic-electrolyzer-fuel cell hybrid power system integrated with a floriculture greenhouse. Energy Build 42(11):2036–2043
Misra D (2021) An experimental study on a portable SPV-integrated forced convective solar dryer. In: Advances in renewable energy and sustainable environment. Springer, Singapore, pp 233–244
Misra D, Ghosh S (2018) Evaporative cooling technologies for greenhouses: a comprehensive review. Agric Eng Int CIGR J 20(1):1–15
Misra D, Ghosh S (2019) Thermal modelling and performance assessment of a circular greenhouse with solar chimney assisted ventilation and fog Cooling. Agric Eng Int CIGR J 20(4):108–118
Singh H, Singh AK, Chaurasia PBL, Singh A (2005) Solar energy utilization: a key to employment generation in the Indian Thar Desert. Int J Sustain Energ 24(3):129–142
Pandey S, Singh VS, Gangwar NP, Vijayvergia MM, Prakash C, Pandey DN (2012) Determinants of success for promoting solar energy in Rajasthan, India. Renew Sustain Energy Rev 16(6):3593–3598
Meena RS, Sharma D, Rathore R (2014) The most promising solar hot spots in India development and policy: the Thar Desert of Rajasthan. Int J Eng Dev Res 3(1):74–79
Sukhatme SP, Nayak JK (1997) Solar energy in western Rajasthan. Curr Sci 62–68
Solar Energy, Ministry of New and Renewable Energy. Available at https://mnre.gov.in/solar/currentstatus/#:~:text=India%20is%20endowed%20with%20vast,providing%20huge%20scalability%20in%20India
Dawn S, Tiwari PK, Goswami AK, Mishra MK (2016) Recent developments of solar energy in India: perspectives, strategies and future goals. Renew Sustain Energy Rev 62:215–235
Report of the expert group on 175 GW RE by 2022. https://niti.gov.in/writereaddata/files/writereaddata/files/document_publication/report-175-GW-RE.pdf
Energy Statistics 2020. http://mospi.nic.in/sites/default/files/publication_reports/ES_2020_240420m.pdf
India becoming a global force in wind energy and solar power. https://www.evwind.es/2020/08/30/india-becoming-a-global-force-in-wind-energy-and-solar-power/76888
Central Electricity Authority of India. https://cea.nic.in/?lang=en
Rajasthan Rajya Vidyut Prasaran Nigam Ltd. https://energy.rajasthan.gov.in/content/dam/raj/energy/corporate-one-lines-vieer/pdf/Publications/T&C%20Manuals/Construction%20Manual%20For%20Lines1.pdf
Rajasthan Renewable Energy Transmission Investment Program. https://www.adb.org/sites/default/files/project-documents/45224/45224-002-dpta-en.pdf
Solar Energy Corporation of India Limited. https://www.seci.co.in/archives/data_archives
Rajasthan Renewables. https://www.renewablesindia.in/?region=29&selection_type=state
Rajasthan Renewable Energy Corporation Limited. https://energy.rajasthan.gov.in
Mercom India. https://mercomindia.com/world-largest-solar-park-bhadla/ .
NS Energy. https://www.nsenergybusiness.com/projects/bhadla-solar-park-rajasthan/
Solar Parks. https://mnre.gov.in/img/documents/uploads/bcf7e95e88ae4f8dbfa8bd25d21e5e12.pdf
Government of India Ministry of New and Renewable Energy Lok Sabha Unstarred Question no-1538. http://164.100.24.220/loksabhaquestions/annex/15/AU1538.pdf
Concentrating solar power projects in India. https://solarpaces.nrel.gov/by-country/IN
CSP Focus. http://www.cspfocus.cn/en/study/detail_84.htm
India One Solar Thermal Power Plant. https://india-one.net/
Rajasthan to set up 30,000 MW solar power plants by 2024–25. https://www.eqmagpro.com/rajasthan-to-set-up-30000-mw-solar-power-plants-by-2024-25/
NTPC Subsidiary Tenders for 3 MW Rooftop Solar Plant at IIT Jodhpur. https://www.saurenergy.com/solar-energy-news/ntpc-subsidiary-tenders-for-3-mw-rooftop-solar-plant-at-iit-jodhpur
Energy Statistics 2019. http://mospi.nic.in/publication/energy-statistics-2019
First farm-based solar power plant comes up in Rajasthan. https://www.thehindu.com/news/national/other-states/first-farm-based-solar-power-plant-comes-up-in-rajasthan/article34226558.ece
Mandatory use of Solar Water Heating Systems in Rajasthan. https://www.iea.org/policies/321-mandatory-use-of-solar-water-heating-systems-in-rajasthan
Rajasthan Energy Conservation Building Rules & Code- 2018. https://energy.rajasthan.gov.in/content/dam/raj/energy/rrecl/pdf/Activities/EC/ECBC_RULES_codes_2018_%20pdf.pdf
Brahma Kumaris. http://www.brahmakumaris.org/hope/renewable-energy/india-one-solar-thermal-power-plant
Sun Focus, UNDP–GEF CSH Project. https://www.in.undp.org
Rajasthan sets a goal of 30,000 MW solar capacity in 5 years. https://www.sourceadvisory.in/forum/general-discussions/rajasthan-sets-a-goal-of-30-000-mw-solar-capacity-in-5-years
PV Magazine. https://www.pv-magazine-india.com/
Saur Energy. https://www.saurenergy.com/solar-energy-news
Back to Growth: Union budget 2021–22 announcement—renewable Energy. https://www.investindia.gov.in/team-india-blogs/back-growth-union-budget-2021-22-announcement-renewable-energy
Rajasthan Solar Policy 2019. https://jalore.rajasthan.gov.in/content/dam/doitassets/jalore/pdffiles/Rajasthan%20Solar%20Energy%20Policy2019.pdf
Rajasthan Budget Analysis 2020–21. https://prsindia.org/budgets/states/rajasthan-budget-analysis-2020-21
Steps to enhance domestic manufacturing of solar PV cells and modules. https://pib.gov.in/PressReleaseIframePage.aspx?PRID=1742795
Desert Power India 2050. https://www.powergrid.in/sites/default/files/footer/smartgrid/desert_power_india.pdf
Rajasthan has attracted investment proposals of over 1.5 lakh crore in renewable energy. https://government.economictimes.indiatimes.com/news/psu/with-1-5-lakh-crore-renewable-energy-investment-proposal-in-hand-rajasthan-aims-to-increase-installed-capacity-by-30000-mw-rrecl-cmd-subhodh-agarwal/82014059
Download references
Author information
Authors and affiliations.
Department of Mechanical Engineering, Techno India Group, Kolkata, 700091, India
Debajit Misra
You can also search for this author in PubMed Google Scholar
Corresponding author
Correspondence to Debajit Misra .
Editor information
Editors and affiliations.
Department of Electrical Engineering, National Institute of Technology Jamshedpur, Jamshedpur, Jharkhand, India
Kumari Namrata
Department of Energy Technology, Aalborg University, Esbjerg, Denmark
Neeraj Priyadarshi
Department of Electrical Engineering, University of Sharjah, Sharjah, United Arab Emirates
Ramesh C. Bansal
Jitendra Kumar
Rights and permissions
Reprints and permissions
Copyright information
© 2023 The Author(s), under exclusive license to Springer Nature Singapore Pte Ltd.
About this paper
Cite this paper.
Misra, D. (2023). Current Status and Future Potential of Solar Energy Utilization in Rajasthan, India. In: Namrata, K., Priyadarshi, N., Bansal, R.C., Kumar, J. (eds) Smart Energy and Advancement in Power Technologies. Lecture Notes in Electrical Engineering, vol 926. Springer, Singapore. https://doi.org/10.1007/978-981-19-4971-5_51
Download citation
DOI : https://doi.org/10.1007/978-981-19-4971-5_51
Published : 09 November 2022
Publisher Name : Springer, Singapore
Print ISBN : 978-981-19-4970-8
Online ISBN : 978-981-19-4971-5
eBook Packages : Energy Energy (R0)
Share this paper
Anyone you share the following link with will be able to read this content:
Sorry, a shareable link is not currently available for this article.
Provided by the Springer Nature SharedIt content-sharing initiative
- Publish with us
Policies and ethics
- Find a journal
- Track your research
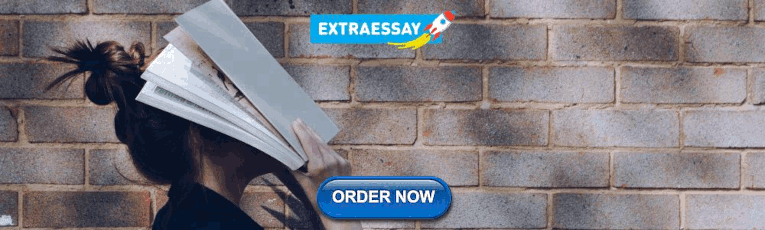
IMAGES
VIDEO
COMMENTS
The review was carried out of a large number of research papers, articles and reports published during the last ten years, both in India and abroad, after collecting scholarly research articles published mostly in Elsevier and ProQuest Direct databases. The dependent variable is the growth of solar energy usage in India.
Abstract. Solar photovoltaic rooftop has emerged as a potential green technology to address climate change issues by reducing reliance on conventional fossil fuel based energy. With a strong commitment to increase the renewable sources based energy capacity to 175 GW by 2022, India has a target to install 100 GW of solar energy capacity. Of ...
More than 5000 trillion kWh/year solar energy incidents over India are estimated, with most parts receiving 4-7 kWh/m2. Currently, energy consumption in India is about 1.13 trillion kWh/year ...
Conference Paper. Jun 2021. Le Hong Lam. Hoang Van Minh Ky. Trinh Trung Hieu. Nguyen Huu Hieu. View. June 2017 ·. PDF | On Mar 31, 2018, Abhishek Kumar and others published A Review Paper on ...
Hence, India needs to harness the vast potential (750 GWp) of solar energy to fulfil its energy need and to mitigate the problem of greenhouse gas emission. Thus, this review paper covered the status of the solar energy in India, state-wise irradiance data as well as potential of solar power generation.
able resources including biomass and wind energy. The novel solar energy innovations oer a remarkable chance to lessening of ozone-depleting substance discharge. Also, by subbing the conventional energy sources, it decreases an unnatural weather change. In this paper, rstly to develop an addressable approach about uncertainty in India's energy
The use of solar power spread exponentially in India during the last few years. There is an affluent amount of solar energy present in India. The average solar insolation received in India is approximately 200 MW/km-square with an average 250-300 sunny day in a year. The solar radiation varies geographically.
India make a global leader in solar energy and the mission envisages an installed solar generation capacity of 20,000 MW by 2020, 100,000 MW by 2030 and of 200,000 MW by 2050. The total expected funding from the government for the 30-year period will run to Rs. 85,000 crore to 105,000 crore [31]. 13.
This paper considers options for a future Indian power economy in which renewables, wind and solar, could meet 80% of anticipated 2040 power demand supplanting the country's current reliance on ...
Solar photovoltaic (PV) is a novel and eco-friendly power source. India's vast solar resources present tremendous solar energy use prospects. The solar PV growth in India has spanned over fifty years, with a significant increase during the past decade. To meet the requirements of the rapidly expanding PV power market in India, it is essential to define, scrutinize, and comprehend the ...
Rapid development of renewable energy sources, particularly solar photovoltaics (PV), is critical to mitigate climate change. As a result, India has set ambitious goals to install 500 gigawatts of ...
solar energy production per watt i nstalled, with an. insolation of 1,700 to 1,900 kilowatt hours per kilowatt. peak (kWh/KWp). 25 .1 MW was adde d in 2010 and 468.3. MW in 2011. Table.3 shows the ...
Keywords. Solar energy; ISM; MICMAC; India; Acknowledgements. The authors of the study would like to give their sincere thanks to Impactful Policy Research in Social Science (IMPRESS) and Indian Council of Social Science Research (ICSSR) for funding the research and allowing us to know about the adoption level of solar energy systems in India.
India's energy crisis can be resolved by using reliable sources of renewable resources like solar energy with minimum adverse ecological effects. Several photovoltaic projects have been sanctioned based on rooftops models and land-based solar parks to address energy security concerns. India's strategy focusing on increasing the installation of new solar plants, lead to the vast expansion of ...
This paper aims to study the solar energy scenario in India by looking into the potential, the usage across various sectors like rooftop solar, solar thermal applications and also the targets set by the government. The study also looks into the incentives and policies for implementing and achieving the national target.
2.1 Impact of renewable energy. India has huge reserves of coal, the fifth largest in the world after USA, Russia, China and Australia. According to the Ministry of Statistics and Programme Implementation, the estimated reserves of coal were 308.80 billion tonnes as on 31 March 2016 and estimated total reserves of lignite as on 31 March 2016 were 44.59 billion tonnes.
Many large projects have been proposed in India, some of them are: i).Thar Desert of India has best solar power projects, estimated to generate 700 to 2,100 GW, ii). The Jawaharlal Nehru National ...
The current available biomass energy in India was approximately 640 million metric tonnes per annum as discussed in Table 5. Biogas is an often overlooked and neglected aspect of renewable energy in India. While solar, wind and hydropower are measured discussion in the country, they are not the only options available.
India with a total of 300 sunny days has a lot of potential in solar energy. This research paper gives an overview of the current scenario and the future possibilities of solar power in India. The research paper also focuses on different aspects related to solar power and initiatives taken by the government of India to fulfill the countries ...
New Delhi-based think tank, the Council on Energy, Environment and Water (CEEW), recently released a report on solar waste on March 20 this year. The report said that India's installed 66.7 GW capacity, as of FY23, generated about 100 kilotonnes (kt) of waste, which will increase to 340 kt by 2030. It also said that India's cumulative ...
This article is part of a special report on the total solar eclipse that will be visible from parts of the U.S., Mexico and Canada on April 8, 2024. It was 11:45 A.M. on August 21, 2017. I was in ...
A Survey on Solar Power for Present and Future Perspective in Indian Market. April 2021. DOI: 10.1007/978-981-33-4080-0_12. In book: Renewable Power for Sustainable Growth, Proceedings of ...
With ambitious renewable energy capacity addition targets, there is an ongoing transformation in the Indian power system. This paper discusses the various applications of variable generation forecast, state-of-the-art solar PV generation forecasting methods, latest developments in generation forecasting regulations and infrastructure, and the new challenges introduced by VRE generation. Day ...
With the right eye protection, viewing the eclipse or partial eclipse can be a great experience. The upcoming eclipse in the U.S. will be a once-in-a-generation event: a full solar eclipse won't happen in the U.S. again until August 2044. "The solar eclipse is an exciting event," Moussa said. "Enjoy it safely." Tips in brief
1. Introduction. India has pledged ambitious goals to increase its clean energy capacity in its submitted Nationally Determined Contribution (NDC) to the Paris Agreement on climate change [1].This includes a commitment to install low carbon energy capacity up to 40% of the total installed electrical capacity by 2030 and to reduce the carbon intensity of its economy by 33-35% by 2030.
According to Energy Statistic Report 2019, India, total 48,175 numbers of off-grid solar pump, 6,852 numbers of street lighting systems, 187,968 numbers of home lighting systems, 225,851 numbers of solar lanterns have been installed in Rajasthan up to 31.03.2019 [ 31 ]. In that time off-grid solar plant was 30.34 MW.