Epilepsy-Definition, Classification, Pathophysiology, and Epidemiology
Affiliation.
- 1 Department of Neurology and Neurological Sciences, Stanford University School of Medicine, Palo Alto, California.
- PMID: 33155183
- DOI: 10.1055/s-0040-1718719
Seizures affect the lives of 10% of the global population and result in epilepsy in 1 to 2% of people around the world. Current knowledge about etiology, diagnosis, and treatments for epilepsy is constantly evolving. As more is learned, appropriate and updated definitions and classification systems for seizures and epilepsy are of the utmost importance. Without proper definitions and classification, many individuals will be improperly diagnosed and incorrectly treated. It is also essential for research purposes to have proper definitions, so that appropriate populations can be identified and studied. Imprecise definitions, failure to use accepted terminology, or inappropriate use of terminology hamper our ability to study and advance the field of epilepsy. This article begins by discussing the pathophysiology and epidemiology of epilepsy, and then covers the accepted contemporary definitions and classifications of seizures and epilepsies.
Thieme. All rights reserved.
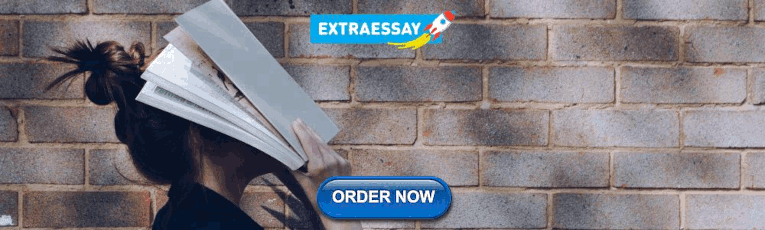
Publication types
- Epilepsy* / classification
- Epilepsy* / epidemiology
- Epilepsy* / etiology
- Epilepsy* / physiopathology
- Seizures* / classification
- Seizures* / epidemiology
- Seizures* / etiology
- Seizures* / physiopathology

- Open access
- Published: 17 September 2021
The pharmacological treatment of epilepsy: recent advances and future perspectives
- Emilio Perucca ORCID: orcid.org/0000-0001-8703-223X 1 , 2
Acta Epileptologica volume 3 , Article number: 22 ( 2021 ) Cite this article
33k Accesses
31 Citations
Metrics details
The pharmacological armamentarium against epilepsy has expanded considerably over the last three decades, and currently includes over 30 different antiseizure medications. Despite this large armamentarium, about one third of people with epilepsy fail to achieve sustained seizure freedom with currently available medications. This sobering fact, however, is mitigated by evidence that clinical outcomes for many people with epilepsy have improved over the years. In particular, physicians now have unprecedented opportunities to tailor treatment choices to the characteristics of the individual, in order to maximize efficacy and tolerability. The present article discusses advances in the drug treatment of epilepsy in the last 5 years, focusing in particular on comparative effectiveness trials of second-generation drugs, the introduction of new pharmaceutical formulations for emergency use, and the results achieved with the newest medications. The article also includes a discussion of potential future developments, including those derived from advances in information technology, the development of novel precision treatments, the introduction of disease modifying agents, and the discovery of biomarkers to facilitate conduction of clinical trials as well as routine clinical management.
The modern treatment of epilepsy started with the introduction of phenobarbital in 1912. The advent of phenytoin in the late thirties marked another milestone, because it was made possible by the introduction of animal models of antiseizure activity [ 1 ]. Similar models also played a key role in the subsequent development of many other antiseizure medications (ASMs). Today, the pharmacological armamentarium against epilepsy includes more than 30 drugs (Table 1 ). These drugs differ in their pharmacokinetics, efficacy, and adverse effect profile, thereby offering unprecedented opportunities to tailor treatment choices to individual needs [ 2 ]. Some of the ASMs introduced after 1985, usually referred to as second-generation drugs, have some safety advantages over older generation agents, but have not increased substantially the proportion of patients who achieve complete freedom from seizures [ 3 ]. For many of these patients, the feasibility of epilepsy surgery, or alternative therapies, should be given early consideration [ 4 , 5 ].
Despite the fact that pharmacoresistance has been little affected by the introduction of newer medications, the drug treatment of epilepsy has made major advances in the last 50 years. In particular, we learnt how to individualize drug selection based on specific patient characteristics such as age, gender, epilepsy syndrome, seizure type, comorbidities, comedications, and other factors affecting clinical response [ 6 ]. We also learnt how to optimize response by careful titration and adjustment of dosage, and use of serum drug levels whenever indicated [ 7 ]. We made major progress in understanding drug interactions, and recognizing the relative merits and indications of monotherapy and polytherapy [ 8 , 9 ]. Likewise, we improved our knowledge of the natural history of epilepsy syndromes, and characterized prognostic factors for seizure recurrence for patients in whom discontinuation of ASMs can be considered after an adequate period of freedom from seizures [ 10 ].
The purpose of the present article is to provide a concise overview of some advances in research on the drug treatment of epilepsy made in the last 5 years, and to discuss currently unmet needs as well as developments which are likely to occur in the foreseeable future.
Advances in characterizing the comparative effectiveness and safety of ASMs
A number of recent clinical trials and observational studies have provided valuable information which can assist physicians in making rational treatment selections. As a follow-up to the initial Standard and New Antiepileptic Drugs (SANAD) trials, which found lamotrigine to be superior to carbamazepine, oxcarbazepine, topiramate and gabapentin in time to treatment failure in patients with mostly focal epilepsy [ 11 ], and valproate to be superior to lamotrigine and topiramate in patients with mostly generalized and unclassified epilepsy [ 12 ], two more recent SANAD trials have been completed. In the first of these trials, 990 adults and children with newly diagnosed focal epilepsy were randomized to receive lamotrigine, levetiracetam or zonisamide, and followed-up for 2 years [ 13 ]. In the per-protocol analysis, lamotrigine was associated with a better 12-month remission from seizures compared with both levetiracetam and zonisamide. In the second SANAD-II trial, which used a similar protocol and enrolled 520 newly diagnosed adults and children, valproate was found to be more effective than levetiracetam in controlling seizures in a pooled cohort of patients with generalized or unclassified epilepsy [ 14 ]. These trials used a pragmatic design mimicking routine clinical practice, even though the possibility of assessment bias due to the open-label, unblinded design cannot be excluded. When considered together with other available lines of evidence, these findings confirm that lamotrigine should be regarded as one of the treatments of first choice for patients with focal seizures. Lamotrigine offers the advantage of being efficacious, generally devoid of adverse effects on mood and cognitive function, and with a low potential to cause adverse drug interactions, even though lamotrigine metabolisn can be affected by a variety of concurrently administered drugs [ 6 ]. One drawback of lamotrigine is the need for gradual titration in order to minimize the risk of serious skin rashes, and therefore it may not be the most appropriate drug for use in patients with frequent severe seizures requiring a prompt onset of antiseizure activity.
The findings from the SANAD studies that valproate is superior to lamotrigine, topiramate and levetiracetam in the treatment of patients with generalized epilepsy are consistent with other lines of evidence. In particular, a recent study from Denmark found that failure to achieve seizure freedom with valproate was the single most important predictor of pharmacoresistance in a cohort of 137 adults with idiopathic (genetic) generalized epilepsy [ 15 ]. The superior efficacy of valproate in controlling seizures associated with generalized epilepsy, however, creates a dilemma in the treatment of females of childbearing potential. In fact, valproate is regarded by the European Medicine Agency as contraindicated for use as first-line treatment in these women (unless the conditions of a rigorous pregnancy prevention programme are fulfilled), due to the higher risk of inducing teratogenic effects as well as impaired postnatal cognitive development in the offspring [ 16 , 17 ].
With respect to teratogenic potential of ASMs, prospective pregnancy registries have contributed greatly to characterize risks associated with individual medications. A particularly important advance was the 2018 publication of data from the international EURAP registry [ 18 ]. This study, based on analysis of 7355 prospective pregnancies exposed to 8 different ASM monotherapies provides risk estimates non only for specific ASMs, but also for different doses of the most commonly used drugs. Overall, the lowest prevalence of major congenital malformations (MCMs) in the offspring was associated with exposure to levetiracetam (2.8% prevalence), lamotrigine (2.9%) and oxcarbazepine (3.0%). Prevalence estimates were intermediate for topiramate (3.9%), carbamazepine (5.5%), phenytoin (6.4%) and phenobarbital (6.5%), and highest for valproate (10.3%). An increased risk with increasing dose was identified for lamotrigine and carbamazepine, and was most prominent for phenobarbital and valproate. In particular, the prevalence of MCMs (with 95% confidence intervals) associated with phenobarbital exposure was 2.7% (0.3–9.5%) at doses < 80 mg/day, 6.2% (3.0–11.1%) at doses > 80 to < 130 mg/day and 11.7% (4.8–22.6%) at doses > 130 mg/day. For valproate, the prevalence of MCMs was 6.3% (4.5–8.6%) at doses < 650 mg/day, 11.3% (9.0–13.9%) at doses > 650 to ≤ 1450 mg/day and 25.2% (17.6–34.2%) at doses > 1450 mg/day. These findings are important because they alert physicians about the need to consider teratogenic risks not only in relation to type of ASM prescribed, but also in relation to dose. A subsequent EURAP investigation documented a clear-cut decrease in the prevalence of MCMs over the period from 2000 to 2013 [ 19 ]. Specifically, there was a 27% decrease in prevalence of MCMs between pregnancies enrolled in the period 2010–2013 compared with those enrolled in the period 2000–2005 (Fig. 1 ). Further analysis of these data provided a strong indication that the improvement in pregnancy outcomes over time was related to changes in ASM prescription patterns, including a major decline in the proportion of pregnancies exposed to valproate. In fact, a reduction in teratogenic risk is one of the important advances associated with the introduction of second-generation ASMs [ 3 ].
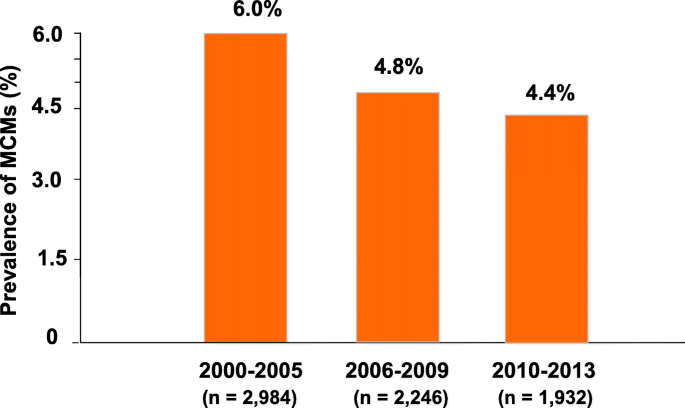
Prevalence of major congenital malformations (MCMs) following prenatal exposure to monotherapy with antiseizure medications (ASMs) among cases enrolled in the EURAP international registry during three different periods. Number of exposures during each period (listed in brackets) refer to the eight most common monotherapies, which accounted for 96.7 to 98.1% of all monotherapy exposures. Based on data from Tomson et al [ 19 ].
Introduction of novel ASMs
During the last 5 years, five novel ASMs (brivaracetam, cannabidiol, cenobamate, everolimus and fenfluramine) have been introduced into the market. Key features of each of these medications are summarized in Table 2 . The history of these drugs is illustrative of different strategies being used in developing novel ASMs.
The development of brivaracetam followed a paradigm which has been in place for a very long time, i.e. the structural modification of an already existing medication with the aim of improving its pharmacological profile. Examples of other ASMs developed with this strategy include methylphenobarbital and primidone (both structurally related to phenobarbital), the phenytoin derivative fosphenytoin, and oxcarbazepine and eslicarbazepine acetate, which represent successive modifications of the carbamazepine structure. In fact, levetiracetam itself was originally developed with the aim of improving the pharmacological profile of piracetam, and its antiseizure activity was discovered by chance. Brivaracetam was selected for development after extensive preclinical screening of a large numbers of levetiracetam derivatives. Compared with levetiracetam, brivaracetam has higher affinity for the synaptic vesicle 2A (SV2A), and a similar pharmacological profile [ 20 ]. Brivaracetam has been found to be superior to placebo in adjunctive-therapy trials in focal epilepsy, but its activity profile in other seizure types has not yet been defined in well designed controlled trials. One limitation of brivaracetam is its lack of efficacy when added-on to levetiracetam, presumably due to competition between the two drugs for the SV2A binding site. The still unanswered question about brivaracetam is whether, and to what extent, its efficacy and tolerability profile differs from that of levetiracetam. It has been suggested that brivaracetam is less likely to cause irritability and other behavioral disturbances compared with levetiracetam [ 20 ]. However, evidence on this remains inconclusive, because to date there have been no randomized head-to-head trials comparing these two drugs [ 21 ].
Another ASM recently approved for the treatment of focal seizures is cenobamate, a carbamate derivative which is also structurally related to previously developed drugs [ 22 ]. During clinical development, three confirmed cases of DRESS (Drug Reaction with Eosinophilia and Systemic Symptoms), including one fatality, were reported when cenobamate was titrated rapidly (weekly or faster titration). Consequently, a revised dosing scheme involving initiation at a small dose and slow titration at 2-week intervals has been implemented. In a safety study, no cases of DRESS were reported when 1339 patients were titrated using the slow titration scheme, and the drug has since been approved in the U.S. and Europe [ 23 ]. In the pivotal adjunctive-therapy randomized trial in patients with refractory focal seizures that led to regulatory approval, the most remarkable finding was the relatively high proportion of patients who achieved seizure freedom (21% in the 400 mg/day cenobamate group versus 1% in the placebo group) [ 24 ]. This contrasts with seizure freedom rates ranging from 0 to 6.5% in comparable trials with other second-generation ASMs [ 25 ]. Comparisons of outcome data across trials, however, should be interpreted cautiously, because of differences in clinical settings and characteristics of the patients. Moreover, seizure freedom data in randomized clinical trials refer to limited assessment periods (typically, a 12-week maintenance period) and can be inflated by the last-observation-carried-forward (LOCF) analysis, whereby patients who did not experience seizures but exited the trial prematurely are still counted as seizure-free in the final analysis. In the pivotal cenobamate trial, the proportion of randomized patients who were seizure-free during the entire 12-week maintenance period and did not exit the trial prematurely was 14%, which is still a relatively high proportion [ 24 ].
All three remaining ASMs introduced in the last 5 years share a common feature, i.e. they were approved for orphan indications. Specifically, cannabidiol was approved for the treatment of seizures associated with Dravet syndrome, Lennox-Gastaut syndrome and tuberous sclerosis complex, fenfluramine was approved for the treatment of seizures associated with Dravet syndrome, and everolimus was approved for the add-on treatment of focal seizures in patients with tuberous sclerosis complex (Table 2 ). Introduction of ASMs for orphan indications is a novel development, made possible by increased awareness of the unmet needs associated with many rare epilepsies and by regulatory incentives to develop drugs for these indications, particularly for children. In fact, only 2 of the 25 ASMs developed prior to 2015 were developed for orphan indications, compared with 3 out of 5 ASMs developed in the last 5 years.
Cannabidiol is one of the many active principles contained in the Cannabis plant, which has been used as an herbal remedy in China as early as 2000 BC. Unlike tetrahydrocannabidol (THC), cannabidiol lacks unwarranted psychoactive effects. Its antiseizure efficacy in its approved indications has been demonstrated in several adjunctive-therapy randomized placebo-controlled trials [ 26 ]. In these trials, many patients received concomitant treatment with clobazam, and the improvement in seizure control observed after adding cannabidiol could be ascribed at least in part to a drug interaction. In fact, cannabidiol is a powerful inhibitor of cytochrome CYP2C19 and by this mechanism increases more than three-fold the serum concentration of norclobazam, the active metabolite of clobazam. There is, however, also evidence that cannabidiol retains independent antiseizure activity, unrelated to its interaction with clobazam [ 27 ].
Another ASM recently introduced for the treatment of seizures associated with Dravet syndrome is fenfluramine, which was first marketed in the sixties and widely used in Europe and the U.S. for over 30 years as an appetite suppressant, either as racemic fenfluramine or as its d-enantiomer dexfenfluramine. In 1997, fenfluramine and dexfenfluramine were withdrawn from the market following the discovery of their association with cardiac valvulopathy and pulmonary hypertension. Prior to its withdrawal from the market, however, fenfluramine had been found to improve seizure control in a small cohort of patients with Dravet syndrome, who were allowed to continue treatment with the drug [ 28 ]. These observations led to recent conduction of randomized placebo-controlled adjunctive therapy trials, which demonstrated a robust seizure-suppressing effect in patients with Dravet syndrome, and subsequent regulatory approval for this indication both in Europe and the United States [ 29 ]. Recent data suggest that fenfluramine may also be useful for the treatment of seizures associated with Lennox-Gastaut syndrome [ 30 ]. To date, no evidence of cardiovascular toxicity has been found in patients with epilepsy treated with fenfluramine, possibly because the doses used for seizure protection are generally lower than those used originally for appetite suppression. Yet, there are still many unanswered questions concerning fenfluramine, including the serum levels of parent drug and its active de-ethylated metabolite norfenfluramine required for seizure suppression in children in comparison with those known to be associated with cardiovascular toxicity in adults [ 29 ]. The potential efficacy and safety advantages of developing individual enantiomers of fenfluramine and norfenfluramine should also be considered [ 29 ].
The last medication discussion in this section, everolimus, is an inhibitor of mTOR (mammalian Target Of Rapamycin). Its use in the treatment of focal seizures associated with tuberous sclerosis complex (TSC) has been prompted by evidence linking the pathogenesis of TSC to mTOR overactivation [ 31 ]. Accordingly, everolimus is an example of a novel strategy in drug development, i.e. a precision treatment targeting the etiology of the disease. Everolimus has been found to be effective in reducing tumor growth as well as drug-resistant seizures in TSC patients. Up to 40% of TSC patients show a significant improvement in seizure control when given adjunctive treatment with everolimus. In the pivotal trial that led to its regulatory approval for use as ASM, seizure frequency decreased progressively over time during treatment, suggesting a possible disease modifying effect [ 32 ]. However, a clinically relevant antiepileptogenic or disease-modifying effect has not yet been clearly demonstrated. The age at which treatment is initiated may be important for the final outcome, but controlled trials in children below 2 years of age have not yet been completed [ 31 ].
Cannabidiol, fenfluramine and everolimus are also examples of drugs approved initially for other indications. In fact, cannabidiol was first marketed in a fixed combination product with THC as a nasal spray for the treatment of spasticity associated with multiple sclerosis, fenfluramine was used initially as an appetite suppressant, and everolimus was first approved for the treatment of advanced kidney cancer, subependymal giant cell astrocytoma (SEGA) associated with TSC, pancreatic neuroendocrine tumors, and other tumors. The repurposing for use in epilepsy of drugs initially approved for other indications is one of the options being pursued in the effort to develop precision treatments (see below).
Introduction of novel formulations
Advances in epilepsy treatment can be achieved not only by developing novel drugs, but also by improving the pharmaceutical formulation of already available medications. One area where particularly significant advances have been made in the last 5 years is the development of novel formulations of ASMs for the treatment of seizure clusters and acute repetitive seizures in the out-of-hospital setting [ 33 ]. Until 2018, the only FDA-approved rescue ASM for out-of-hospital use was diazepam rectal gel. In 2019, the FDA approved two additional products for this indication, namely intranasal midazolam [ 34 ] and intranasal diazepam [ 35 ]. Use of these medications is associated with a rapid onset of antiseizure effect, thereby stopping seizures before they progress to established status epilepticus. The intranasal route is generally well accepted by patients and caregivers, as it avoids the social embarrassment associated with use of the rectal route.
Alternative non-rectal rescue formulations of benzodiazepines for out-of-hospital use have been available in other countries for a number of years. In particular, a rapidly absorbed buccal formulation of midazolam has been available in Europe since 2011 [ 36 ]. Innovative formulations of ASMs under development as potential rescue therapy for emergency use include a diazepam buccal film, and an inhaled formulation of alprazolam [ 33 ]. Oral formulations can also be used at times as a rescue treatment, but they can be associated with drawbacks in this setting, such as slower or suboptimal absorption, need for patient cooperation (which is not always feasible) and risk of aspiration [ 36 ].
Future perspectives
Extensive research is ongoing in many areas, and important advances leading to improved epilepsy outcomes are likely to occur in a not too distant future. A few relevant examples will be discussed below.
Increased application of technological tools to epilepsy management
In recent years, information technology (IT)-based applications have been increasingly utilized in epilepsy management, as shown by the widespread use of smartphones to record seizures in the out-hospital setting, and the expanding opportunities offered by Internet-based services in areas such as distant education and telemedicine [ 37 ]. Smartphone applications (apps) are also being increasingly used to assist people with epilepsy to manage and cope with their disease. Most of these apps focus on issues such as treatment management, medication adherence, health care communication, and seizure tracking [ 38 ]. Other apps are aimed at assisting healthcare professionals (HCPs), on example being tools to improve epilepsy diagnosis in non-specialist settings [ 39 , 40 ]. We recently designed a smartphone app to help HCPs in selecting ASM selection for patients with seizure onset at age 10 years or above, particularly in settings where no specialized expertise is available [ 41 , 42 ]. This app is freely available on the Internet ( www.epipick.org ). In a recent validation study, selection of ASMs recommended by the app based on individual patient characteristics was found to be associated with improved seizure outcomes and fewer adverse effects compared with use of ASMs not recommended by the app [ 43 ]. In the future, individualized ASM selection is likely to empowered by more sophisticated technology, including artificial intelligence (machine learning)-based approaches. In a recent study, a machine learning approach combining clinical, genetic and clinical trial data derived from individual patients permitted to construct a computerized model that predicted response to a specific ASM [ 44 ].
In addition to facilitating treatment selection, technology will increasingly assist patients and physicians in monitoring response to treatment through a variety of tools, including seizure detection devices [ 45 ]. Efforts are also ongoing into development of sophisticated technology, including artificial intelligence, for the prediction of epileptic seizures [ 46 ]. This could pave the way to innovative treatment strategies, such as the intermittent use of ASMs prior to the time at which a seizure is predicted to occur.
Precision therapies
In recent years, our understanding of the molecular mechanisms involved in the pathogenesis of epilepsies has improved considerably. One area where advances have been greatest is the genetics of the epilepsies, and in particular the discovery of gene mutations responsible for a large proportion of patients with developmental and epileptic encephalopathies (DEEs) [ 47 ]. The elucidation of an epileptogenic mutation permits to establish the functional abnormality responsible for the epilepsy in the affected individual, and to identify (or develop) precision-therapy medications that may be able to correct such abnormality. One example of a precision therapy is the utilization of the ketogenic diet to control seizures associated with Glucose Transporter Type 1 (GLUT1) deficiency syndrome. In this condition, GLUT1 deficiency results in impaired brain uptake of glucose and consequent neuronal dysfunction, which can be overcome by supplying the brain with an alternative source of energy [ 48 ]. As discussed in recent reviews [ 49 , 50 , 51 ], precision treatments targeting the mechanisms responsible for epilepsy in individuals with specific gene mutations may involve use of drugs previously approved for other indications, a process known as drug repurposing. One example of repurposed drug is the mTOR inhibitor everolimus for the treatment of seizures associated with TSC, as discussed above in this article. Approaches to identify repurposed drugs for specific monogenic epilepsies have been described [ 52 ]. In some cases, improved outcomes can be achieved not by administering additional drugs, but by removing medications that can paradoxically aggravate seizures in these patients [ 49 ]. Importantly, precision therapies are applicable not only to genetic epilepsies, but also to epilepsies due to other etiologies, such metabolic, inflammatory or immune-mediated causes [ 53 ].
At present, application of precision therapies in the management of epilepsy is still in its early days, and will likely expand as further knowledge accrues and newer and more effective targeted treatments are introduced. For genetic epilepsies, targeted (precision) treatments have been reported to improve outcomes in a considerable proportion patients with identified gene mutations [ 54 ], although a more recent survey gave a more sober assessment of the current impact of these treatments [ 55 ].
Biomarker-guided therapies
The search for biomarkers continues to be a hot topic in epilepsy research. Biomarkers can be based on a variety of measures such as genetic, molecular, cellular, imaging, and electrophysiological measures, other clinical or laboratory data, or a combination of these [ 56 , 57 ]. Biomarkers could potentially be used for different purposes, for example to improve diagnostic accuracy, to identify ongoing epileptogenesis and its mechanisms, to predict seizure response (or lack of response) to specific treatments, to assess the probability of seizure recurrence after treatment withdrawal, or to evaluate susceptibility to adverse drug effects. Some biomarkers, such as the HLA-B*15:02 antigen to identify individuals at high risk of carbamazepine-induced serious cutaneous adverse reactions among Han Chinese and other South Asian ethnic groups, are already in routine clinical use [ 58 ].
With respect to potential therapeutic advances, identification and validation of biomarkers could improve treatment outcomes in many ways [ 56 , 57 , 59 ]. First, biomarkers could be used to identify individuals at high risk of developing epilepsy after an epileptogenic insult, thereby permitting selection of these individuals for clinical trials of potential antiepileptogenic therapies. Second, identification of biomarkers predictive of seizure recurrence could facilitate decision on whether to start or withhold ASM therapy in patients who experienced a single seizure. Third, biomarkers predictive of a favorable response to a specific medication would be valuable to select patients to be enrolled in clinical trials of that medication, thereby increasing responder rate and sparing non-responders the burden of receiving placebo or an ineffective treatment. Fourth, biomarkers could theoretically be used to monitor response to treatment, by informing physicians at an early stage on whether the prescribed medication has the required efficacy and safety in a specific individual. Lastly, and most importantly, biomarkers could in the future inform physicians on which ASM is most likely to control seizures effectively and with fewest adverse effects. This may change radically treatment paradigms: for example, the value of a drug which is effective in achieving complete seizure control in 5% of patients with pharmacoresistant epilepsy would be greatly enhanced if we had a biomarker that can identify beforehand those patients who are responsive to that drug. In that scenario, we would use that drug only in responsive patients, thereby increasing the success rate to 100%.
In practice, in most situations it is unlikely that a single biomarker will provide optimal information for any intended purpose. More realistically, breakthroughs are likely to come from algorithms that utilize a combination of biomarkers and other clinical information. The development of artificial intelligence-based tools can facilitate greatly these approaches [ 44 ].
Novel drugs and the search for disease-modifying therapies
The modest impact of second-generation ASMs on seizure outcome in patients resistant to older agents justifies continuing efforts to develop newer and potentially more effective treatments (Table 3 ). Drug development is currently benefiting from many advances, including deeper knowledge of the mechanisms of epileptogenesis and seizure generation in relation to specific etiologies, improved understanding of mechanisms of pharmacoresistance, and availability of disease-specific models as well as models of pharmacoresistance [ 53 , 60 ]. These advances are changing the paradigms used to discover and develop new drugs.
An important paradigm change is a switch from a focus on medications aimed at suppressing seizures to a focus on treatments targeting the underlying disease, i.e. specific etiologies and the molecular mechanisms associated with such etiologies [ 51 , 61 ]. Future precision treatments emerging from this approach will include repurposed drugs [ 50 , 62 ], novel small molecules, and other treatments based on innovative technologies such as antisense oligonucleotides [ 63 , 64 ] and gene therapy [ 65 ]. Some of these therapies require invasive routes of administration, which are also being explored for innovative uses of already established medications [ 66 ].
A closely related paradigm change consists in targeting specifically epileptogenesis and other manifestations of the disease [ 60 , 62 , 67 ]. Such treatment could potentially be used to prevent epilepsy, to inhibit its progression (in those syndromes showing a progressive course), or to alter the appearance or progression of comorbidities such as intellectual disability and other disorders. A wide variety of compounds have been found to possess antiepileptogenic and/or neuroprotective activity in preclinical models through antiinflammatory, antioxidant and other mechanisms [ 68 , 69 , 70 , 71 ]. In addition to novel molecules, these compounds includes naturally occurring substances such as phytocannabinnoids, melatonin, erythropoietin, vitamins and other dietary constituents [ 68 , 70 , 72 , 73 , 74 ], as well as medications already approved for other indications, such as metformin [ 75 ], montelukast [ 76 ], atorvastatin, ceftriaxone, and losartan [ 62 ]. Whether these properties documented in animal models translate into benefit in the clinical setting remains to be demonstrated. Of note, a number of precision treatments directed at specific etiologies of epilepsy could exhibit disease-modifying effects, although it is possible that any medication acting on a single molecular pathway may not address all the complex comorbidities associated with aberrant neural networks [ 61 ].
As discussed above, clinical trials of investigational new drugs could be facilitated by development of biomarkers to detect the occurrence of epileptogenesis at an early stage, to identify drug responsive patients and to monitor response to treatment. Despite claims to the contrary [ 77 ], demonstrating that a chronically administered treatment started before seizure onset prevents the occurrence of seizures in patients still receiving that treatment does not prove epilepsy prevention, because any ASM having a purely symptomatic effect could also produce such an outcome. Likewise, some comorbidities, such as progression of cognitive disability, may be prevented solely as a result of seizure suppression. Truly innovative trial designs will be required to generate unequivocal evidence that a drug is effective in preventing epilepsy, or has a direct disease modifying effect [ 78 ].
Conclusions
Despite the fact that second-generation ASMs have not reduced substantially the burden of pharmacoresistance, advances in the drug treatment of epilepsy continue to be made. These advances result mostly from improved understanding of the comparative efficacy and safety of existing ASMs and from the introduction of newer medicines and innovative formulations. Further advances can be ascribed to technological tools for distant education, telemedicine, and patient empowerment made possible by self-management smartphone-based apps.
It likely that further important therapeutic advances will occur in the coming years. Thanks to ongoing multidisciplinary efforts, clinical outcome for people with epilepsy is likely to improve due to advances in IT technology, development of novel precision therapies, identification of biomarkers to guide drug development as well as routine clinical management, and, ultimately, introduction of truly innovative disease modifying therapies.
Availability of data and materials
Not applicable.
Abbreviations
Antiseizure medication
Developmental and epileptic encephalopathy
Cerebrospinal fluid
Drug Reaction with Eosinophilia and Systemic Symptoms
Dravet syndrome
Cytochrome P450
Electroencephalography
International Registry of Antiepileptic Drugs and Pregnancy
Food and Drug Administration (United States)
Healthcare professional
Gamma-aminobutyric acid
G protein-coupled receptor 55
Information technology
Lennox-Gastaut syndrome
Major congenital malformation
Last-observation-carried-forward
Mammalian Target Of Rapamycin
P-glycoprotein
Standard and New Antiepileptic Drugs
Subependymal giant cell astrocytoma
Synaptic vesicle 2A
Transient receptor potential vanilloid type 1
Tuberous sclerosis complex
Uridine 5′-diphospho-glucuronosyltransferase
Perucca E. Antiepileptic drugs: evolution of our knowledge and changes in drug trials. Epileptic Disord. 2019;21(4):319–29. https://doi.org/10.1684/epd.2019.1083 .
Article PubMed Google Scholar
Moshé SL, Perucca E, Ryvlin P, Tomson T. Epilepsy: new advances. Lancet. 2015;385(9971):884–98. https://doi.org/10.1016/S0140-6736(14)60456-6 .
Perucca E, Brodie MJ, Kwan P, Tomson T. 30 years of second-generation antiseizure medications: impact and future perspectives. Lancet Neurol. 2020;19(6):544–56. https://doi.org/10.1016/S1474-4422(20)30035-1 .
Article CAS PubMed Google Scholar
Dalic L, Cook MJ. Managing drug-resistant epilepsy: challenges and solutions. Neuropsychiatr Dis Treat. 2016;12:2605–16. https://doi.org/10.2147/NDT.S84852 .
Article CAS PubMed PubMed Central Google Scholar
Perucca P, Scheffer IE, Kiley M. The management of epilepsy in children and adults. Med J Aust. 2018;208(5):226–33. https://doi.org/10.5694/mja17.00951 .
Perucca E, Tomson T. The pharmacological treatment of epilepsy in adults. Lancet Neurol. 2011;10(5):446–56. https://doi.org/10.1016/S1474-4422(11)70047-3 .
Patsalos PN, Berry DJ, Bourgeois BF, Cloyd JC, Glauser TA, Johannessen SI, et al. Antiepileptic drugs--best practice guidelines for therapeutic drug monitoring: a position paper by the subcommission on therapeutic drug monitoring, ILAE Commission on Therapeutic Strategies. Epilepsia. 2008;49(7):1239–76. https://doi.org/10.1111/j.1528-1167.2008.01561.x .
Zaccara G, Perucca E. Interactions between antiepileptic drugs, and between antiepileptic drugs and other drugs. Epileptic Disord. 2014;16(4):409–31. https://doi.org/10.1684/epd.2014.0714 .
Schmidt D. Drug treatment strategies for epilepsy revisited: starting early or late? One drug or several drugs? Epileptic Disord. 2016;18(4):356–66. https://doi.org/10.1684/epd.2016.0882 .
Lamberink HJ, Otte WM, Geerts AT, Pavlovic M, Ramos-Lizana J, Marson AG, et al. Individualised prediction model of seizure recurrence and long-term outcomes after withdrawal of antiepileptic drugs in seizure-free patients: a systematic review and individual participant data meta-analysis. Lancet Neurol. 2017;16(7):523–31. https://doi.org/10.1016/S1474-4422(17)30114-X .
Marson AG, Al-Kharusi AM, Alwaidh M, Appleton R, Baker GA, Chadwick DW, et al. The SANAD study of effectiveness of carbamazepine, gabapentin, lamotrigine, oxcarbazepine, or topiramate for treatment of partial epilepsy: an unblinded randomised controlled trial. Lancet. 2007;369(9566):1000–15. https://doi.org/10.1016/S0140-6736(07)60460-7 .
Marson AG, Al-Kharusi AM, Alwaidh M, Appleton R, Baker GA, Chadwick DW, et al. The SANAD study of effectiveness of valproate, lamotrigine, or topiramate for generalised and unclassifiable epilepsy: an unblinded randomised controlled trial. Lancet. 2007b;369(9566):1016–26. https://doi.org/10.1016/S0140-6736(07)60461-9 .
Marson A, Burnside G, Appleton R, Smith D, Leach JP, Sills G, et al. The SANAD II study of the effectiveness and cost-effectiveness of levetiracetam, zonisamide, or lamotrigine for newly diagnosed focal epilepsy: an open-label, non-inferiority, multicentre, phase 4, randomised controlled trial. Lancet. 2021;397(10282):1363–74. https://doi.org/10.1016/S0140-6736(21)00247-6 .
Marson A, Burnside G, Appleton R, Smith D, Leach JP, Sills G, et al. The SANAD II study of the effectiveness and cost-effectiveness of valproate versus levetiracetam for newly diagnosed generalised and unclassifiable epilepsy: an open-label, non-inferiority, multicentre, phase 4, randomised controlled trial. Lancet. 2021;397(10282):1375–86. https://doi.org/10.1016/S0140-6736(21)00246-4 .
Gesche J, Khanevski M, Solberg C, Beier CP. Resistance to valproic acid as predictor of treatment resistance in genetic generalized epilepsies. Epilepsia. 2017;58(4):e64–9. https://doi.org/10.1111/epi.13702 .
Meador K. Teratogenicity and antiseizure medications. Epilepsy Curr. 2020;20(6_suppl):15S–7S.
Article Google Scholar
Tomson T, Battino D, Perucca E. Teratogenicity of antiepileptic drugs. Curr Opin Neurol. 2019;32(2):246–52. https://doi.org/10.1097/WCO.0000000000000659 .
Tomson T, Battino D, Bonizzoni E, Craig J, Lindhout D, Perucca E, et al. Comparative risk of major congenital malformations with eight different antiepileptic drugs: a prospective cohort study of the EURAP registry. Lancet Neurol. 2018;17(6):530–8. https://doi.org/10.1016/S1474-4422(18)30107-8 .
Tomson T, Battino D, Bonizzoni E, Craig J, Lindhout D, Perucca E, et al. Declining malformation rates with changed antiepileptic drug prescribing: an observational study. Neurology. 2019;93(9):e831–40. https://doi.org/10.1212/WNL.0000000000008001 .
Steinhoff BJ, Staack AM. Levetiracetam and brivaracetam: a review of evidence from clinical trials and clinical experience. Ther Adv Neurol Disord. 2019;12:1756286419873518.
Subramonian A, Farrah K. Brivaracetam versus levetiracetam for epilepsy: a review of comparative clinical safety: Canadian Agency for Drugs and Technologies in Health, Ottawa, Ontario, Canada; 2020. Available at: https://cadth.ca/sites/default/files/pdf/htis/2020/RC1322%20Comparing%202%20Antiepileptic%20Drugs%20Final.pdf (accessed June 8, 2021)
Löscher W, Sills GJ, White HS. The ups and downs of alkyl-carbamates in epilepsy therapy: how does cenobamate differ? Epilepsia. 2021;62(3):596–614. https://doi.org/10.1111/epi.16832 .
Roberti R, De Caro C, Iannone LF, Zaccara G, Lattanzi S, Russo E. Pharmacology of cenobamate: Mechanism of action, pharmacokinetics, drug-drug interactions and tolerability. CNS Drugs. 2021. https://doi.org/10.1007/s40263-021-00819-8 (online ahead of print).
Krauss GL, Klein P, Brandt C, Lee SK, Milanov I, Milovanovic M, et al. Safety and efficacy of adjunctive cenobamate (YKP3089) in patients with uncontrolled focal seizures: a multicentre, double-blind, randomised, placebo-controlled, dose-response trial. Lancet Neurol. 2020;19(1):38–48. https://doi.org/10.1016/S1474-4422(19)30399-0 .
Vossler DG. Remarkably high efficacy of cenobamate in adults with focal-onset seizures: a double-blind, randomized, placebo-controlled trial. Epilepsy Curr. 2020;20(2):85–7. https://doi.org/10.1177/1535759720903032 .
Article PubMed PubMed Central Google Scholar
Franco V, Bialer M, Perucca E. Cannabidiol in the treatment of epilepsy: current evidence and perspectives for further research. Neuropharmacology. 2021;185:108442. https://doi.org/10.1016/j.neuropharm.2020.108442 .
Bialer M, Perucca E. Does cannabidiol have antiseizure activity independent of its interactions with clobazam? An appraisal of the evidence from randomized controlled trials. Epilepsia. 2020;61(6):1082–9. https://doi.org/10.1111/epi.16542 .
Ceulemans B, Schoonjans AS, Marchau F, Paelinck BP, Lagae L. Five-year extended follow-up status of 10 patients with Dravet syndrome treated with fenfluramine. Epilepsia. 2016;57(7):e129–34. https://doi.org/10.1111/epi.13407 .
Odi R, Invernizzi RW, Gallily T, Bialer M, Perucca E. Fenfluramine repurposing from weight loss to epilepsy: what we do and do not know. Pharmacol Ther. 2021;226:107866. https://doi.org/10.1016/j.pharmthera.2021.107866 .
Bialer M, Johannessen SI, Koepp MJ, Levy RH, Perucca E, Perucca P, et al. Progress report on new antiepileptic drugs: a summary of the fifteenth Eilat conference on new antiepileptic drugs and devices (EILAT XV). II. Drugs in more advanced clinical development. Epilepsia. 2020;61(11):2365–85. https://doi.org/10.1111/epi.16726 .
Overwater IE, Rietman AB, van Eeghen AM, de Wit MCY. Everolimus for the treatment of refractory seizures associated with tuberous sclerosis complex (TSC): current perspectives. Ther Clin Risk Manag. 2019;15:951–5. https://doi.org/10.2147/TCRM.S145630 .
French JA, Lawson JA, Yapici Z, Ikeda H, Polster T, Nabbout R, et al. Adjunctive everolimus therapy for treatment-resistant focal-onset seizures associated with tuberous sclerosis (EXIST-3): a phase 3, randomised, double-blind, placebo-controlled study. Lancet. 2016;388(10056):2153–63. https://doi.org/10.1016/S0140-6736(16)31419-2 .
Fedak Romanowski EM, McNamara NA, Neil EE, Gottlieb-Smith R, Dang LT. Seizure rescue medications for out-of-hospital use in children. J Pediatr. 2021;229:19–25. https://doi.org/10.1016/j.jpeds.2020.10.041 .
Bouw MR, Chung SS, Gidal B, King A, Tomasovic J, Wheless JW, et al. Clinical pharmacokinetic and pharmacodynamic profile of midazolam nasal spray. Epilepsy Res. 2021;171:106567. https://doi.org/10.1016/j.eplepsyres.2021.106567 .
Boddu SHS, Kumari S. A short review on the intranasal delivery of diazepam for treating acute repetitive seizures. Pharmaceutics. 2020;12(12):1167. https://doi.org/10.3390/pharmaceutics12121167 .
Article CAS Google Scholar
Gidal B, Klein P, Hirsch LJ. Seizure clusters, rescue treatments, seizure action plans: unmet needs and emerging formulations. Epilepsy Behav. 2020;112:107391. https://doi.org/10.1016/j.yebeh.2020.107391 .
Fesler JR, Stanton S, Merner K, Ross L, McGinley MP, Bena J, et al. Bridging the gap in epilepsy care: a single-center experience of 3700 outpatient tele-epilepsy visits. Epilepsia. 2020;61(8):e95–e100. https://doi.org/10.1111/epi.16619 .
Alzamanan MZ, Lim KS, Akmar Ismail M, Abdul GN. Self-management apps for people with epilepsy: systematic analysis. JMIR Mhealth Uhealth. 2021;9(5):e22489. https://doi.org/10.2196/22489 .
Patterson V. The development of a smartphone application to help manage epilepsy in resource-limited settings. Seizure. 2020;79:69–74. https://doi.org/10.1016/j.seizure.2020.03.020 .
Giuliano L, Cicero CE, Trimarchi G, Todaro V, Colli C, Crespo Gómez EB, et al. Usefulness of a smartphone application for the diagnosis of epilepsy: validation study in high-income and rural low-income countries. Epilepsy Behav. 2021;115:107680. https://doi.org/10.1016/j.yebeh.2020.107680 .
Asadi-Pooya AA, Beniczky S, Rubboli G, Sperling MR, Rampp S, Perucca E. A pragmatic algorithm to select appropriate antiseizure medications in patients with epilepsy. Epilepsia. 2020;61(8):1668–77. https://doi.org/10.1111/epi.16610 .
Beniczky S, Rampp S, Asadi-Pooya AA, Rubboli G, Perucca E, Sperling MR. Optimal choice of antiseizure medication: agreement among experts and validation of a web-based decision support application. Epilepsia. 2021;62(1):220–7. https://doi.org/10.1111/epi.16763 .
Hadady L, Klivényi P, Asadi-Pooya AA, Rampp S, Fabó D, Bereczki C, et al. Web-based decision support system for patient-tailored selection of antiseizure treatment medication in adolescents and adults: An external validation study. 2021 (submitted).
Google Scholar
de Jong J, Cutcutache I, Page M, Elmoufti S, Dilley C, Fröhlich H, et al. Towards realizing the vision of precision medicine: AI based prediction of clinical drug response. Brain. 2021:awab108. https://doi.org/10.1093/brain/awab108 Online ahead of print.
Beniczky S, Wiebe S, Jeppesen J, Tatum WO, Brazdil M, Wang Y, et al. Automated seizure detection using wearable devices: a clinical practice guideline of the international league against epilepsy and the International Federation of Clinical Neurophysiology. Epilepsia. 2021;62(3):632–46. https://doi.org/10.1111/epi.16818 .
Rasheed K, Qayyum A, Qadir J, Sivathamboo S, Kwan P, Kuhlmann L, et al. Machine learning for predicting epileptic seizures using EEG signals: a review. IEEE Rev Biomed Eng. 2021;14:139–55. https://doi.org/10.1109/RBME.2020.3008792 .
Perucca P, Bahlo M, Berkovic SF. The genetics of epilepsy. Annu Rev Genomics Hum Genet. 2020;21(1):205–30. https://doi.org/10.1146/annurev-genom-120219-074937 .
Daci A, Bozalija A, Jashari F, Krasniqi S. Individualizing treatment approaches for epileptic patients with glucose transporter Type1 (GLUT-1) deficiency. Int J Mol Sci. 2018;19(1):122. https://doi.org/10.3390/ijms19010122 .
Article CAS PubMed Central Google Scholar
Perucca P, Perucca E. Identifying mutations in epilepsy genes: impact on treatment selection. Epilepsy Res. 2019;152:18–30. https://doi.org/10.1016/j.eplepsyres.2019.03.001 .
Specchio N, Pietrafusa N, Perucca E, Cross JH. New paradigms for the treatment of pediatric monogenic epilepsies: progressing toward precision medicine. Epilepsy Behav. 2021:107961. https://doi.org/10.1016/j.yebeh.2021.107961 Online ahead of print.
Byrne S, Enright N, Delanty N. Precision therapy in the genetic epilepsies of childhood. Dev Med Child Neurol. 2021. https://doi.org/10.1111/dmcn.14929 Online ahead of print.
Atkin TA, Maher CM, Gerlach AC, Gay BC, Antonio BM, Santos SC, et al. A comprehensive approach to identifying repurposed drugs to treat SCN8A epilepsy. Epilepsia. 2018;59(4):802–13. https://doi.org/10.1111/epi.14037 .
Löscher W, Potschka H, Sisodiya SM, Vezzani A. Drug resistance in epilepsy: clinical impact, potential mechanisms, and new innovative treatment options. Pharmacol Rev. 2020;72(3):606–38. https://doi.org/10.1124/pr.120.019539 .
Peng J, Pang N, Wang Y, Wang X-L, Chen J, Xiong J. Next-generation sequencing improves treatment efficacy and reduces hospitalization in children with drug-resistant epilepsy. CNS Neurosci Ther. 2019;25(1):14–20. https://doi.org/10.1111/cns.12869 .
Balestrini S, Chiarello D, Gogou M, Silvennoinen K, Puvirajasinghe C, Jones WD, et al. Real-life survey of pitfalls and successes of precision medicine in genetic epilepsies. J Neurol Neurosurg Psychiatry. 2021:jnnp-2020-325932. https://doi.org/10.1136/jnnp-2020-325932 Online ahead of print.
Kobylarek D, Iwanowski P, Lewandowska Z, Limphaibool N, Szafranek S, Labrzycka A, et al. Advances in the potential biomarkers of epilepsy. Front Neurol. 2019;10:685. https://doi.org/10.3389/fneur.2019.00685 .
Engel J Jr, Pitkänen A. Biomarkers for epileptogenesis and its treatment. Neuropharmacology. 2020;167:107735. https://doi.org/10.1016/j.neuropharm.2019.107735 .
Chang CJ, Chen CB, Hung SI, Ji C, Chung WH. Pharmacogenetic testing for prevention of severe cutaneous adverse drug reactions. Front Pharmacol. 2020;11:969. https://doi.org/10.3389/fphar.2020.00969 .
Simonato M, Agoston DV, Brooks-Kayal A, Dulla C, Fureman B, Henshall DC, et al. Identification of clinically relevant biomarkers of epileptogenesis - a strategic roadmap. Nat Rev Neurol. 2021;17(4):231–42. https://doi.org/10.1038/s41582-021-00461-4 .
Galanopoulou AS, Löscher W, Lubbers L, O'Brien TJ, Staley K, Vezzani A, et al. Antiepileptogenesis and disease modification: Progress, challenges, and the path forward-report of the preclinical working group of the 2018 NINDS-sponsored antiepileptogenesis and disease modification workshop. Epilepsia Open. 2021;6(2):276–96. https://doi.org/10.1002/epi4.12490 .
Kearney H, Byrne S, Cavalleri GL, Delanty N. Tackling epilepsy with high-definition precision medicine: a review. JAMA Neurol. 2019;76(9):1109–16. https://doi.org/10.1001/jamaneurol.2019.2384 .
Klein P, Friedman A, Hameed MQ, Kaminski RM, Bar-Klein G, Klitgaard H, et al. Repurposed molecules for antiepileptogenesis: missing an opportunity to prevent epilepsy? Epilepsia. 2020;61(3):359–86. https://doi.org/10.1111/epi.16450 .
Han Z, Chen C, Christiansen A, Ji S, Lin Q, Anumonwo C, et al. Antisense oligonucleotides increase Scn1a expression and reduce seizures and SUDEP incidence in a mouse model of Dravet syndrome. Sci Transl Med. 2020;12(558):eaaz6100.
Ahonen S, Nitschke S, Grossman TR, Kordasiewicz H, Wang P, Zhao X, et al. Gys1 antisense therapy rescues neuropathological bases of murine Lafora disease. Brain. 2021:awab194. https://doi.org/10.1093/brain/awab194 Online ahead of print.
Higurashi N, Broccoli V, Hirose S. Genetics and gene therapy in Dravet syndrome. Epilepsy Behav. 2021:108043. https://doi.org/10.1016/j.yebeh.2021.108043 Online ahead of print.
Cook M, Murphy M, Bulluss K, D'Souza W, Plummer C, Priest E, et al. Anti-seizure therapy with a long-term, implanted intra-cerebroventricular delivery system for drug-resistant epilepsy: a first-in-man study. EClinicalMedicine. 2020;22:100326. https://doi.org/10.1016/j.eclinm.2020.100326 .
Löscher W. The holy grail of epilepsy prevention: preclinical approaches to antiepileptogenic treatments. Neuropharmacology. 2020;167:107605. https://doi.org/10.1016/j.neuropharm.2019.04.011 .
Rahman MH, Akter R, Kamal MA. Prospective function of different antioxidant containing natural products in the treatment of neurodegenerative disease. CNS Neurol Disord drug targets 2020 Jul 22. Doi: https://doi.org/10.2174/1871527319666200722153611 . Online ahead of print.
Leavy A, Jimenez Mateos EM. Perinatal brain injury and inflammation: lessons from experimental murine models. Cells. 2020;9(12):2640. https://doi.org/10.3390/cells9122640 .
Yang N, Guan QW, Chen FH, Xia QX, Yin XX, Zhou HH, et al. Antioxidants targeting mitochondrial oxidative stress: promising neuroprotectants for epilepsy. Oxidative Med Cell Longev. 2020:6687185. https://doi.org/10.1155/2020/6687185 .
Zavala-Tecuapetla C, Cuellar-Herrera M, Luna-Munguia H. Insights into potential targets for therapeutic intervention in epilepsy. Int J Mol Sci. 2020;21(22):8573. https://doi.org/10.3390/ijms21228573 .
Frajewicki A, Laštůvka Z, Borbélyová V, Khan S, Jandová K, Janišová K, et al. Perinatal hypoxic-ischemic damage: review of the current treatment possibilities. Physiol Res. 2020;69(Suppl 3):S379–401. https://doi.org/10.33549/physiolres.934595 .
Murugan M, Boison D. Ketogenic diet, neuroprotection, and antiepileptogenesis. Epilepsy Res. 2020;167:106444. https://doi.org/10.1016/j.eplepsyres.2020.106444 .
Stone NL, Murphy AJ, England TJ, O'Sullivan SE. A systematic review of minor phytocannabinoids with promising neuroprotective potential. Br J Pharmacol. 2020;177(19):4330–52. https://doi.org/10.1111/bph.15185 .
Sanz P, Serratosa JM, Sánchez MP. Beneficial effects of metformin on the central nervous system, with a focus on epilepsy and Lafora disease. Int J Mol Sci. 2021;22(10):5351. https://doi.org/10.3390/ijms22105351 .
Tesfaye BA, Hailu HG, Zewdie KA, Ayza MA, Berhe DF. Montelukast: the new therapeutic option for the treatment of epilepsy. J Exp Pharmacol. 2021;13:23–31. https://doi.org/10.2147/JEP.S277720 .
Kotulska K, Kwiatkowski DJ, Curatolo P, Weschke B, Riney K, Jansen F, et al. Prevention of epilepsy in infants with tuberous sclerosis complex in the EPISTOP trial. Ann Neurol. 2021;89(2):304–14. https://doi.org/10.1002/ana.25956 .
Franco V, French JA, Perucca E. Challenges in the clinical development of new antiepileptic drugs. Pharmacol Res. 2016;103:95–104. https://doi.org/10.1016/j.phrs.2015.11.007 .
Download references
Acknowledgments
This article was not supported by any funding source.
Author information
Authors and affiliations.
Department of Internal Medicine and Therapeutics, Division of Clinical and Experimental Pharmacology, University of Pavia, Via Ferrata 9, 27100, Pavia, Italy
Emilio Perucca
Department of Neuroscience, Central Clinical School, Monash University, Melbourne, Victoria, 3004, Australia
You can also search for this author in PubMed Google Scholar
Contributions
The author was solely responsible for the conception and preparation of this article. The author read and approved the final manuscript.
Corresponding author
Correspondence to Emilio Perucca .
Ethics declarations
Ethics approval and consent to participate, consent for publication.
The author gave consent for publication of this article.
Competing interests
The author received speaker’s or consultancy fees from Angelini-Arvelle, Biogen, Eisai, GW Pharma, Sanofi, Sun Pharma, UCB Pharma, Xenon Pharma and Zogenix.
Additional information
This article is dedicated to the memory of Professor Alan Richens (1938–2021), my mentor and dear friend, in recognition of his outstanding contribution to advancing the pharmacological treatment of epilepsy.
Rights and permissions
Open Access This article is licensed under a Creative Commons Attribution 4.0 International License, which permits use, sharing, adaptation, distribution and reproduction in any medium or format, as long as you give appropriate credit to the original author(s) and the source, provide a link to the Creative Commons licence, and indicate if changes were made. The images or other third party material in this article are included in the article's Creative Commons licence, unless indicated otherwise in a credit line to the material. If material is not included in the article's Creative Commons licence and your intended use is not permitted by statutory regulation or exceeds the permitted use, you will need to obtain permission directly from the copyright holder. To view a copy of this licence, visit http://creativecommons.org/licenses/by/4.0/ .
Reprints and permissions
About this article
Cite this article.
Perucca, E. The pharmacological treatment of epilepsy: recent advances and future perspectives. Acta Epileptologica 3 , 22 (2021). https://doi.org/10.1186/s42494-021-00055-z
Download citation
Received : 28 June 2021
Accepted : 30 July 2021
Published : 17 September 2021
DOI : https://doi.org/10.1186/s42494-021-00055-z
Share this article
Anyone you share the following link with will be able to read this content:
Sorry, a shareable link is not currently available for this article.
Provided by the Springer Nature SharedIt content-sharing initiative
- Drug therapy
- Antiepileptic drugs
- Antiseizure medications
Acta Epileptologica
ISSN: 2524-4434
- Submission enquiries: Access here and click Contact Us
Thank you for visiting nature.com. You are using a browser version with limited support for CSS. To obtain the best experience, we recommend you use a more up to date browser (or turn off compatibility mode in Internet Explorer). In the meantime, to ensure continued support, we are displaying the site without styles and JavaScript.
- View all journals
- My Account Login
- Explore content
- About the journal
- Publish with us
- Sign up for alerts
- Open access
- Published: 11 May 2024
Performance investigation of epilepsy detection from noisy EEG signals using base-2-meta stacking classifier
- Torikul Islam 1 , 2 ,
- Redwanul Islam 2 ,
- Monisha Basak 2 ,
- Amit Dutta Roy 2 ,
- Md. Adil Arman 3 ,
- Samanta Paul 4 ,
- Oleksii Shandra 3 &
- Sk. Rahat Ali 2
Scientific Reports volume 14 , Article number: 10792 ( 2024 ) Cite this article
579 Accesses
Metrics details
- Biomedical engineering
Epilepsy is a chronic neurological disease, characterized by spontaneous, unprovoked, recurrent seizures that may lead to long-term disability and premature death. Despite significant efforts made to improve epilepsy detection clinically and pre-clinically, the pervasive presence of noise in EEG signals continues to pose substantial challenges to their effective application. In addition, discriminant features for epilepsy detection have not been investigated yet. The objective of this study is to develop a hybrid model for epilepsy detection from noisy and fragmented EEG signals. We hypothesized that a hybrid model could surpass existing single models in epilepsy detection. Our approach involves manual noise rejection and a novel statistical channel selection technique to detect epilepsy even from noisy EEG signals. Our proposed Base-2-Meta stacking classifier achieved notable accuracy (0.98 ± 0.05), precision (0.98 ± 0.07), recall (0.98 ± 0.05), and F1 score (0.98 ± 0.04) even with noisy 5-s segmented EEG signals. Application of our approach to the specific problem like detection of epilepsy from noisy and fragmented EEG data reveals a performance that is not only superior to others, but also is translationally relevant, highlighting its potential application in a clinic setting, where EEG signals are often noisy or scanty. Our proposed metric DF-A (Discriminant feature-accuracy), for the first time, identified the most discriminant feature with models that give A accuracy or above (A = 95 used in this study). This groundbreaking approach allows for detecting discriminant features and can be used as potential electrographic biomarkers in epilepsy detection research. Moreover, our study introduces innovative insights into the understanding of these features, epilepsy detection, and cross-validation, markedly improving epilepsy detection in ways previously unavailable.
Similar content being viewed by others
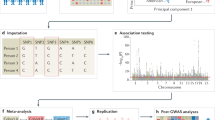
Genome-wide association studies
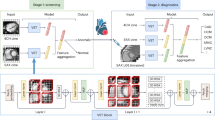
Screening and diagnosis of cardiovascular disease using artificial intelligence-enabled cardiac magnetic resonance imaging
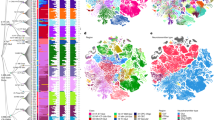
A high-resolution transcriptomic and spatial atlas of cell types in the whole mouse brain
Introduction.
Epilepsy is a prominent neurological disease that is denoted by repetitive and unprovoked seizures 1 , 2 . Seizures result from abnormal sudden electrical discharges in the brain, often typically presenting with loss of consciousness, convulsion, atypical movements, behavioral alterations or emotions 3 , 4 . Additionally, epilepsy is one of the most common neurological diseases since around 50 million people worldwide have it 5 .
The detection of epilepsy remains a significant challenge nowadays. It is a chronic neurological disease that can affect individuals of all ages. Moreover, aging can affect epilepsy detection significantly which makes epilepsy detection more challenging 6 , 7 . The etiology of epilepsy is heterogeneous, with variations observed among individuals. In certain instances, it is associated with underlying conditions such as traumatic brain injuries or developmental disorders 8 . Yet, the exact causes of epilepsy are still unknown and epilepsy detection presents a substantial challenge.
Recent advancements in diagnostic approaches allowed researchers and clinicians to shift from subjective assessments to more objective and quantifiable tests, recognized by improved accuracy since they provide more accurate results. Establishing a diagnosis of epilepsy most of the time involves a review of the exhaustive medical history and a physical examination, along with a series of tests. These tests include an electroencephalogram (EEG) to measure brain activity and imaging scans such as functional magnetic resonance imaging (fMRI) to identify any structural abnormalities in the brain. Epilepsy brings substantial changes to certain parts of the brain regions such as the hippocampus, amygdala, frontal cortex, temporal cortex, and olfactory cortex 9 , 10 , 11 . However, these structural changes occur in a rather slow progression.
EEG is a non-invasive test that measures and records the electrical activity of the brain using electrodes placed on the scalp 12 , 13 . During an EEG, the electrical signals generated by the brain are amplified and displayed as waveforms on a computer screen. The patterns observed in the EEG can provide valuable information including the presence of epilepsy, seizure classification, and seizure prediction 14 . Therefore, EEG plays a crucial role in the detection of epilepsy. Correspondingly, it will also facilitate the treatment of epilepsy which will be immensely helpful for human beings.
Literature review
In a study, a wavelet scalogram was utilized in the dataset described by Andrzejak et al. 15 with Alex net and achieved 100% accuracy 16 . Fourier-based synchro squeezing transform (SST) and convolutional neural network were applied in the publicly available CHB-MIT dataset and achieved 99.63% accuracy 17 . Multi-view feature learning was utilized with convolutional deep learning applied in the CHB-MIT dataset achieving 94.37% accuracy 18 . Till now different studies utilizing neural networks for epilepsy detection are discussed. Although, in various studies, researchers utilized neural networks but, it is having some dilemma too. Here, EEG signals are converted into images by different transform analysis which is an arduous and time-consuming task. Additionally, the convolutional neural network automatically extracts features from the image. Hence, researches are not aware of the features that play significant role in epilepsy detection. In a study, Discrete wavelet transform was used to decompose the data into sub-bands and then the wavelet energy distribution of each band was used as a feature set from the dataset described by Andrzejak et al. 15 utilized in artificial neural network (ANN) for classification achieved 95.0% accuracy 19 . However, ANN is very complex and it requires copious data for training. Thus, it is very time-consuming. Several machine learning models were used for epilepsy detection and they exhibited promising results. One study utilized wavelet transform on a dataset described by Andrzejak et al. 15 with K means clustering has achieved 96.67% accuracy 20 . Another study used the optimum allocation technique on the dataset described by Andrzejak et al. 15 with the logistic model tree achieved 96.67% accuracy 21 . Fuzzy distribution entropy (fDisEn) and wavelet packet decomposition were extracted from the dataset described by Andrzejak et al. 15 and were utilized in K-nearest neighbor. This method achieved 98.33% accuracy as per reference 22 . Symlet wavelet processing and grid search optimizer featured extracted from dataset described by Andrzejak et al. 15 and were used in gradient boosting machine. This method achieved 96.10% accuracy 1 . In some studies, researchers segmented the EEG signals. Thus, prolonged EEG signals were not required for classification. In a study, the 5 s epileptic segment was utilized with sample entropy and distributed entropy, using GA-SVM (genetic algorithm for feature selection and parameter optimization of support vector machine) on the dataset described by Andrzejak et al. 15 and achieved a maximum AUC of 96.67% 23 . In practical while data is recorded from humans there are so many noises and artifacts which makes epilepsy detection more challenging. Spectrogram images of EEG signals were utilized in a pre-trained convolutional neural network applied in the TUH dataset, which achieved the highest 88.30% accuracy as per as the reference 24 . A one-dimensional deep convolutional neural network was used for the automated identification of abnormal EEG signals without any feature extraction and applied in the TUH dataset, which achieved 79.34% accuracy 25 .
Research gap
A common practice in epilepsy research is often based on the use of smooth, noise-free datasets. As the data is mostly noise-free and smooth, researchers only utilized filters such as band pass, high pass, and low pass to put an end to noise from the signal. Therefore, researchers achieved significantly higher accuracy for epilepsy detection. In contrast, the real-world EEG signals are often contaminated by noise and various kinds of artifacts, which raises big questions regarding the practicality of the aforementioned methods. Therefore, the methodology proposed by the researchers will not work flawlessly.
Epilepsy detection from segmented EEG has supplementary utility. However, practical use of segmented EEG in detection still remains a limiting challenge for epilepsy diagnosis, since the accuracy rates drop much below certain conditions that remain to be fully understood. Therefore, although segmented has added value for epilepsy detection, researchers cannot take advantage of it.
Different researchers utilized non-identical features for their study 7 , 26 , 27 , 28 . Additionally, researchers claimed that the feature utilized in their study is the most prominent feature for epilepsy detection 26 . Thus, it is not transparent that actually which features play consequential role in epilepsy detection. Moreover, there appears to be no widely accepted significance order for epilepsy detection. Furthermore, researchers only have a few feature for their study 26 , 27 .
Researchers have not created any metric that can be utilized to exhibit the significance of the features for all the machine learning models. It is a notable issue since different features work differently with disparate machine learning models. Hence, it was not feasible for the researchers to construct a feature significance order for epilepsy detection that would remain accurate for all the models.
The field has thus far mostly focused on the use of either single machine learning or neural network-based models for epilepsy detection, leaving quite unexplored area of more promising hybrid models or the synergistic use of a few models. This narrow vision is missing the opportunity in which the fusion of different methodologies could make the system of epilepsy detection more accurate and robust. It points toward a very significant area for future research and development in this domain.
The main problems that we are striving to address in this paper: are (1) achieving the utmost accuracy in epilepsy detection utilizing noisy data (TUH dataset) which are more likely to practical EEG signals. (2) Finding out the reason behind accuracy mitigation while utilizing segmented EEG signals. (3) Finding out the discriminant features and evolving the feature order for epilepsy detection. (4) proposing a new metric that can be utilized to exhibit the significance of the features for all the machine learning models. (5) proposing a new hybrid model that will detect epilepsy more precisely than a conventional single model. To the best of our knowledge, existing research has not specifically addressed these challenges and hence, our work represents a significant advance, offering innovative solutions rather than mere incremental progress. This highlights the potential for translational application and highlights the innovation and impact of our research. Therefore, this research outcome will be substantial enough for epilepsy detection.
Methodology
Data preparation and processing.
In this study, we utilized the world’s largest publicly available EEG database which is called the TUH EEG corpus database 8 . The number of channels used for the recording of EEG signals is 32. Here, some channels are non EEG, such as (EEG-KKG, and EEG-RESP). We did not use these channels 29 . 10–20 electrode placement systems used in this dataset. This dataset carries annotation files by dint of it we can acquire knowledge about the event of each channel. Here, we used data from 10 disparate patients (5 epileptic, 5 normal). Delineate information regarding patients is provided in the supplementary information.
EEG may contain muscle activity, eye movement, power line interference, and interference from other devices. These are called noise of EEG signals. The primary purpose of EEG signals is to record the cerebral electrical activity of the brain. However, it may also record the electrical activity that is not generated from the cerebral region of the brain which is known as artifact of EEG signals. These noise and artifacts do not contain any significant information that may assist the analyze of EEG signals.
Noise and artifact evolve dilemma while analyzing EEG signals. Noise and artifact removal may increase the output of EEG signal analyze in a positive direction significantly. In the previous study, researchers utilized only filtering owing to remove the noise artifact. In this study, we have also utilized manual noise remove for better results.
Signal analysis and feature extraction
A bandpass filter is the most effective way to remove noise from the EEG signal. In our study, EEG signals were passed across a bandpass filter with a cut-off frequency between 0.1 to 44 Hz, resulting in high-frequency noise along with low-frequency superfluous signal being detached. MATLAB EEGLAB was utilized for the visualization as well as filtering of the signal. EEG signals before as well as after filtering are depicted in supplementary information.
After filtering the signal, noise, and artifacts were obviated from the signal manually by taking advantage of the annotation files. To discern the noise, we employed the image exhibited in the annotation files. EEG signals before as well as after removing the noise along with the artifact are depicted in supplementary information.
As the TUH EEG corpus dataset comprises a multi-channel EEG signal, it is necessary to select the appropriate channels. We used statistical features such as mean, median, and standard deviation for channel selection. Initially, we calculated statistical features for each channel of the EEG signal. Subsequently, we constructed co-relation as well as reckoned the p-value of the mean, median, and standard deviation for each channel of the EEG signal. Afterward, we selected two channels that have the upmost co-relation and p-value.
In this study, we segmented the Normal EEG signal and Epileptic EEG signal into 5-s fragments. Then, this 5-s EEG signal was used for feature extraction. Different signal fragments have been used in other research studies such as 0.1 s 30 , 2 s 31 , 32 , 4 s 33 , 5 s 23 , 34 , and 60 s 25 .
In the context of signal processing, features refer to specific characteristics of a signal. Additionally, features provide more relevant information about the signal context. To extract features various mathematical computations as well as algorithms are applied to the raw signals. For instance, in the case of EEG signals, there are copious features. Different techniques are utilized to extract these features.
Based on the idea of signal processing, a signal can have many features. However, the significance of the features is not alike. Disparate features have different noteworthiness. Feature ranking depicts the importance of the features of that particular signal.
Machine learning framework
A machine learning model is an algorithm that learns patterns from the given data to make prediction, and decisions without being explicitly programmed. These models initially process data, learn from data, and make decisions and predictions for unknown data.
Classification indices imply the performance of the machine learning models for classification tasks. These metrices indicate how well machine learning works, in making classification. Therefore, we can perceive that which model can be utilized for our stipulate classification task. In this study, we used four classification indices such as accuracy, precision, recall, f1 score.
Furthermore, to enhance the robustness of our findings, we used cross-validation. The basic idea of cross-validation is to divide the dataset into multiple subsets, train and test the model on different subsets, and then aggregate the results to get a more robust evaluation of the model's performance.
In this method, multiple base models are trained independently on the training data, each producing its predictions or decisions. The prime reason behind it is different models can capture different aspects of the data. All the models take the same feature value and make their predictions individually. Afterward, this prediction can be used for the classification or prediction that may outperform the single model.
A meta-classifier, is a higher-level model that combines the predictions or decisions of multiple base models to make a final prediction or classification. The purpose of the meta-classifier is to learn how to best combine the predictions of the base models to make a final prediction or classification. It takes into account the strengths and weaknesses of the base models and attempts to leverage the complementary information provided by them.
A key innovation in our methodology is the use of a stacking classifier which is the combination of base model and meta classifier. The idea behind stacking is to escalate predictive performance. In this study, we utilized two base model, and then the output of the base models were given to meta classifier.
Proposed metrics
In this study, we proposed a metric for depicting the significance of features. Initially, all the feature ranks are calculated for different models using permutation feature importance 35 , 36 , 37 , 38 . Different models gave disparate feature ranks. To solve this dilemma, we proposed new metric called DF-A (discriminant feature–accuracy). In this metric, accuracy implies the cut-off accuracy for the selected model. For example, DF-90 implies the models that gave 90 percent or above accuracy are taken into account. Afterward, the cumulative order for selected models is calculated for all the features. Cumulative feature order means the summation of feature order for any particular feature for the selected models. The most discriminant feature has the least feature order value. Then, features are arranged in ascending order of the cumulative feature order. In this way, we calculated the value of DF-A that exhibits the importance of features of a particular signal for any particular task.
Proposed model
In this study, we utilized two models for ensemble to mitigate the computational complexity. The output prediction of the model is utilized to evolve a new dataset. Afterwards, this meta-classifier is used with the new dataset. In this way, our proposed model exhibited in Fig. 1 outperformed all other models.
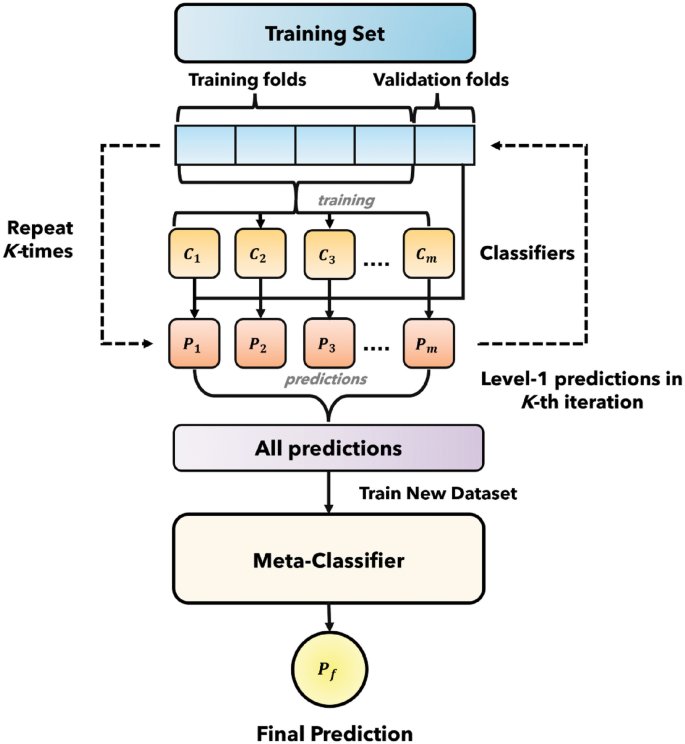
Stacking classifier (combining base model and meta classifier).
Proposed methodology
Here, Fig. 2 depicts the novel methodology used in this study for epilepsy detection along with feature ranking.
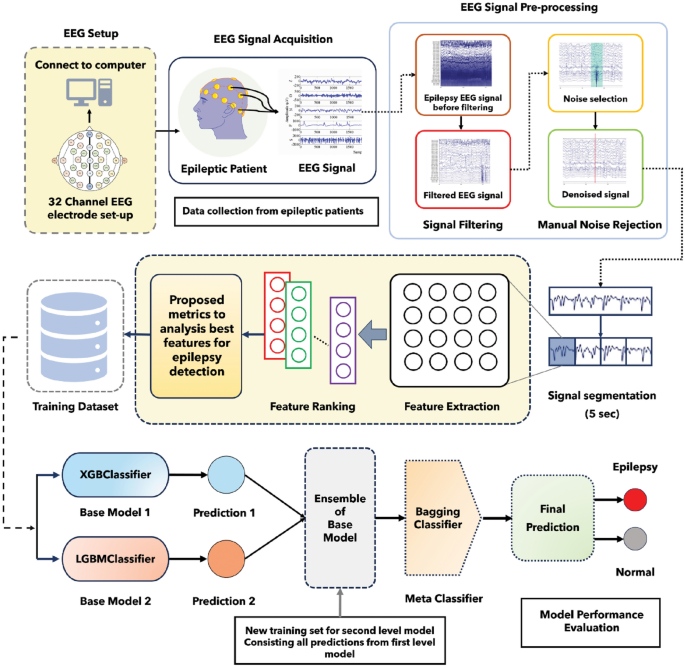
Graphical representation of the proposed methodology of this study.
Single base model
The results for the single model with 32 feature datasets is depicted in supplementary information. We divided the classifier model into two parts such as right and left. In the left side, classifier models that exhibited accuracy 95% or above. On the other hand, Classifier models that exhibited lower than 95% accuracy are on the right side.
DF-95 metrics
In order to exhibit the most significant feature DF-A metric was utilized. In this study, we selected 95% accuracy for DF-A metric as the accuracy parameter is variable and it can be altered. We used all the classifier models that exhibited 95% accuracy or above in single-model epilepsy detection task. Feature orders were calculated for all 32 features and seven classifier models that exhibited selected 95 accuracy or above. In Table 1 , first column shows the 32 features used in this study. From column 2 to column 8 shows the feature order of 32 features used in this study for selected seven classifiers. Here, feature order was calculated by permutation feature importance. Afterward, in column 9 shows cumulative ranking order which is calculated by simple arithmetic addition. Subsequently, DF-95 (discriminant features–accuracy) is calculated by observing the cumulative ranking order. The feature achieving the least cumulative ranking order is entitled as the most discriminant feature. In this way, all 32 features are ranked for DF-95. The results are depicted in Table 1 .
Base 2 meta stacking classifier
To reduce the computational complexity only 2 models were used as a Base model. XGB Classifier along with LGBM Classifier as a base classifier performed better than any other model. It may happened since they also performed significantly better as a single model for epilepsy detection with a 32-feature dataset. Therefore, results are depicted while they were utilized as base classifiers and others models as meta classifiers. We also investigated the impact of cross-validation in this study and utilized disparate CV values such as 10, 25, 50, 75, and 100.
Supplemental information depicts the results of models for cross-validation value 10. We can perceived that the highest accuracy, precision, recall, and F1 score were respectively 0.95 (+ /− 0.04), 0.96 (+ /− 0.06), 1.00 (+ /− 0.00), and 0.95 (+ /− 0.04). However, some models exhibited 1.00 (+ /− 0.00) recall, but accuracy, precision, and F1 score were low for them.
Supplemental information depicts the results of models for cross-validation value 25. We can perceived that the highest accuracy, precision, recall, and F1 score were respectively 0.95 (+ /− 0.04), 0.96 (+ /− 0.06), 1.00 (+ / − 0.00), and 0.96 (+ /− 0.06). However, some models exhibited 1.00 (+ /− 0.00) recall, but accuracy, precision, and F1 score were low for them.
Supplemental information depicts the results of models for cross-validation value 50. we can perceived that the highest accuracy, precision, recall, and F1 score were respectively 0.97 (+ / − 0.06) , 0.97 (+ / − 0.07) , 0.98 (+ / − 0.05), and 0.97 (+ / − 0.05) . Although some models exhibited 1.00 (+ /− 0.00) recall, but accuracy, precision, and F1 score were low for them.
Table 2 depicts the results of models for cross-validation value 75. We can perceived that the highest accuracy, precision, recall, and F1 score were respectively 0.98 (+ / − 0.05) , 0.98 (+ / − 0.07) , 0.98 (+ / − 0.05) and 0.98 (+ / − 0.04) . Although some models exhibited 1.00 (+ /− 0.00) recall, but accuracy, precision, and F1 score were low for them. Meta–Bagging Classifier provided the upmost results when it was used as a meta-classifier.
Supplemental information depicts results of models for cross-validation value 100. We can perceived that the highest accuracy, precision, recall, and F1 score were respectively 0.97 (+ /− 0.06), 0.97 (+ /− 0.07), 0.98 (+ /− 0.05), and 0.97 (+ /− 0.05). Although some models exhibited 1.00 (+ /− 0.00) recall, but accuracy, precision and F1 score were low for them.
Findings of the study
The most significant finding of this study are proposed combination of the Base-2-Meta model that exhibits higher accuracy than the usually used single model. Additionally, finding out the most discriminant feature for epilepsy detection. Moreover, we have proposed a metric DF-A for depicting the most discriminant feature that is persistent with most of the models that give A accuracy or above. Furthermore, we have also investigated the influence of cross-validation in this study. In addition, one of the most significant findings of this study is to work with a challenging dataset containing copious noise and artifacts and achieving higher accuracy by manual noise rejection and filtering. Besides, achieving upmost accuracy with 5-s fragments of EEG signals.
In our proposed model, we utilized XGB and LGBM classifiers as base classifiers. We used the Bagging Classifier as a meta-classifier. We observed from the results that our proposed model gave better accuracy the single base model. Accuracy, precision, recall and F1 score for this Base-2-Meta model are respectively 0.98 (+ /− 0.05), 0.98 (+ /− 0.07), 0.98 (+ /− 0.05) and 0.98 (+ /− 0.04). Additionally, it also renders better results than other Base-2-Meta models. Initially, base model used the dataset and made the initial prediction. The probability of prediction is used as a dataset for the meta-classifier.
We observed from the study that some models gave better accuracy when they were used as meta classifiers than utilized as a single classifier model. We realized from this escalation of results that the output result substantially depends on the Base classifier. While the classifier model works as a single classifier model they used the main feature dataset. Conversely, when they worked as a meta classifier they used the dataset evolved from the probability of prediction predicted by the Base classifier.
Our proposed metric, DF-95 rendered the rankings of the features for epilepsy detection. Previously, there was confusion about the most discriminant feature since different model implies different features as the most discriminant. Additionally, different researchers also claim different features as the most discriminant. However, our proposed metric resolves this dilemma.
We investigated the impact of cross-validation in this study. We observed that when we increased the cross-validation value model training time increased significantly. However, we also observed the escalation of results with the cross-validation. Thus, we have found a tradeoff between execution time and accuracy for cross-validation. Although a higher cross-validation value increases the complexity, it gives better results.
EEG signals contain various noise and artifacts and they lower the results of detection. However, researchers in their research work mostly utilized noise-free and smooth datasets. Thus, they were able to achieve the upmost accuracy with their proposed methodology. However, with the practical data their proposed models do not work up to the mark.
In this study, we utilized a manual noise rejection technique along with filtering the data. In addition, we utilized a novel statistical channel selection procedure to find out the most significant EEG channel. Afterward, features were extracted from these channels. Therefore, our proposed methodology achieved significant accuracy even with the dataset containing noise and artifacts.
We utilized fragments of EEG signals. Thus, a prolonged EEG signal is not required for epilepsy detection. In cases where only a small fragment of EEG signals is present, our proposed methodology can be utilized to accurately detect epilepsy with those small EEG signals.
We have found a tradeoff between signal-to-noise ratio (SNR) with the length of the EEG signals. When the length of the EEG signal is reduced, detection accuracy is also mitigated. Researchers utilized only filtering for noise eradication. However, filtering alone cannot eradicate the noise and artifact accurately. Therefore, when the length of the signals is reduced the signal part of the signal is reduced considerably but noise and artifacts do not reduce at that high level. Thus, a significant reduction of results is observed while the length of the signal is reduced.
Conversely, our methodology worked significantly even with the 5 s fragment of the EEG signals. In our proposed method, we utilized novel manual noise and artifact rejection techniques along with filtering. In this way, noise and artifacts can be removed significantly better than only filtering. Therefore, while the error rate with signal fragments for other studies is significantly high, in our study error rate with 5 s fragments of data is only 2%.
We have achieved up-to-the-mark results with our proposed methodology even though we utilized a dataset that contains copious noise and artifact-like practical data. We achieved this sublime result owing to our novel approaches. Therefore, our proposed methodology will be very useful for the practical application. Tables 3 and 4 exhibit the comparison of our study with previous studies conducted on the same dataset. From the comparison, it is evident that our study surpasses previous studies. This superiority is attributed to our innovative approach.
Limitations
In our study, although we achieved 98% accuracy, it can be further improved with additional novel techniques in the future. Although, we utilized 32 features, the number of features can be further improved which might raise the result. we rendered a ranking of features for epilepsy detection. However, this order of features was only calculated between the 32 features utilized in this study. In the future, if further features are augmented then the orders of the features can be altered. The result of our proposed metric also varied with the accuracy parameter added with it. We utilized 5 s fragments of EEG data. Therefore, while EEG data is less than 5 s, our proposed methodology cannot detect epilepsy as accurately as the result exhibited in Table 3 and Table 4 . Although our proposed methodology has a few drawbacks, it provides intriguing insights for epilepsy detection. However, in the future we are looking forward to providing more significant insights for epilepsy detection and resolving these drawbacks.
Although epilepsy detection is a challenging task, our proposed methodology can do it merely accurately. We utilized a novel manual noise rejection technique with filtering and extracted copious features for epilepsy detection. Afterward, a novel Base-2-Meta stacking model is utilized for the detection of epilepsy. Even though with the noisy data our proposed method can work up to the mark. We have also discovered the most discriminant features order in this study. In addition, our proposed metric DF-A used to exhibit the most discriminant feature unprecedentedly. We have also achieved significantly higher accuracy with 5 s fragments of EEG signals. The influence of cross-validation for the detection of epilepsy is investigated in this study. Our investigation provided significant insights for epilepsy detection. These insights can be considerably useful for the detection and treatment of epilepsy.
Data availability
The TUH EEG Epilepsy Corpus dataset was collected from the web link- https://isip.piconepress.com/projects/tuh_eeg/html/downloads.shtml .
Wang, X., Gong, G. & Li, N. Automated recognition of epileptic EEG states using a combination of symlet wavelet processing, gradient boosting machine, and grid search optimizer. Sensors 19 (2), 219. https://doi.org/10.3390/s19020219 (2019).
Article ADS MathSciNet PubMed PubMed Central Google Scholar
Islam, R. et al. Epileptic Seizure Detection from EEG Signal Using ANN-LSTM Model. In Proceedings of Trends in Electronics and Health Informatics: TEHI 2022 (eds Mahmud, M. et al. ) 129–141 (Springer, 2023). https://doi.org/10.1007/978-981-99-1916-1_10 .
Chapter Google Scholar
Lüders, H. O. et al. Proposal: Different types of alteration and loss of consciousness in epilepsy. Epilepsia https://doi.org/10.1111/epi.12595 (2014).
Article PubMed Google Scholar
Sharma, R., Sircar, P. & Pachori, R. B. Automated seizure classification using deep neural network based on autoencoder. in Handbook of Research on Advancements of Artificial Intelligence in Healthcare Engineering https://doi.org/10.4018/978-1-7998-2120-5.ch001 (2020).
Kumar, H., Debnath, S. & Sharma, A. Can epilepsy be cured? A review. Heal. Sci. Rev. https://doi.org/10.1016/j.hsr.2022.100062 (2022).
Article Google Scholar
Islam, T., Basak, M., Islam, R. & Roy, A. D. Investigating population-specific epilepsy detection from noisy EEG signals using deep-learning models. Heliyon https://doi.org/10.1016/j.heliyon.2023.e22208 (2023).
Article PubMed PubMed Central Google Scholar
Sharma, R., Pachori, R. B. & Sircar, P. Seizures classification based on higher order statistics and deep neural network. Biomed. Signal Process. Control https://doi.org/10.1016/j.bspc.2020.101921 (2020).
Walsh, S. et al. A systematic review of the risks factors associated with the onset and natural progression of epilepsy. Neurotoxicology https://doi.org/10.1016/j.neuro.2016.03.011 (2017).
Zhao, F. et al. Neuropsychological deficits in temporal lobe epilepsy: A comprehensive review. Ann. Indian Acad. Neurol. https://doi.org/10.4103/0972-2327.144003 (2014).
Chatzikonstantinou, A. Epilepsy and the hippocampus. In The Hippocampus in Clinical Neuroscience (eds Szabo, K. & Hennerici, M. G.) 121–142 (S. Karger AG, 2014). https://doi.org/10.1159/000356435 .
Sharma, R. Localization of epileptic surgical area using automated hybrid approach based on higher-order statistics with sensitivity analysis and residual wavelet transform. Biomed. Signal Process. Control https://doi.org/10.1016/j.bspc.2023.105192 (2023).
Kumar, Y., Dewal, M. L. & Anand, R. S. Epileptic seizure detection using DWT based fuzzy approximate entropy and support vector machine. Neurocomputing https://doi.org/10.1016/j.neucom.2013.11.009 (2014).
Sharma, R., Sircar, P. & Pachori, R. B. Automated focal EEG signal detection based on third order cumulant function. Biomed. Signal Process. Control https://doi.org/10.1016/j.bspc.2020.101856 (2020).
Sharma, R., Sircar, P. & Pachori, R. B. Computer-aided diagnosis of epilepsy using bispectrum of EEG signals. Appl. Biomed. Eng. Neurosci. https://doi.org/10.1007/978-981-13-7142-4_10 (2019).
Andrzejak, R. G. et al. Indications of nonlinear deterministic and finite-dimensional structures in time series of brain electrical activity: Dependence on recording region and brain state. Phys. Rev. E https://doi.org/10.1103/PhysRevE.64.061907 (2001).
Roy, A. D. & Islam, M. M. Detection of Epileptic Seizures from Wavelet Scalogram of EEG Signal Using Transfer Learning with AlexNet Convolutional Neural Network. https://doi.org/10.1109/ICCIT51783.2020.9392720 (2020).
Ozdemir, M. A., Cura, O. K. & Akan, A. Epileptic EEG classification by using time-frequency images for deep Learning. Int. J. Neural Syst. https://doi.org/10.1142/S012906572150026X (2021).
Yuan, Y., Xun, G., Jia, K. & Zhang, A. A multi-view deep learning framework for EEG seizure detection. IEEE J. Biomed. Heal. Informatics https://doi.org/10.1109/JBHI.2018.2871678 (2019).
Wani, S. M., Sabut, S. & Nalbalwar, S. L. Detection of epileptic seizure using wavelet transform and neural network classifier. In Computing, Communication and Signal Processing: Proceedings of ICCASP 2018 (eds Brijesh Iyer, S. L. et al. ) 739–747 (Springer, 2019). https://doi.org/10.1007/978-981-13-1513-8_75 .
Orhan, U., Hekim, M. & Ozer, M. EEG signals classification using the K-means clustering and a multilayer perceptron neural network model. Expert Syst. Appl. https://doi.org/10.1016/j.eswa.2011.04.149 (2011).
Kabir, E. & Siuly, Y. Z. Epileptic seizure detection from EEG signals using logistic model trees. Brain Inf. 3 (2), 93–100. https://doi.org/10.1007/s40708-015-0030-2 (2016).
Zhang, T., Chen, W. & Li, M. Fuzzy distribution entropy and its application in automated seizure detection technique. Biomed. Signal Process. Control 39 , 360–377. https://doi.org/10.1016/j.bspc.2017.08.013 (2018).
Li, P., Karmakar, C., Yan, C., Palaniswami, M. & Liu, C. Classification of 5-S epileptic EEG recordings using distribution entropy and sample entropy. Front. Physiol. https://doi.org/10.3389/fphys.2016.00136 (2016).
Thomas, J., Comoretto, L., Jin, J., Dauwels, J., Cash, S. S. & Westover, M. B. EEG CLassification Via Convolutional Neural Network-Based Interictal Epileptiform Event Detection. in Proceedings of the Annual International Conference of the IEEE Engineering in Medicine and Biology Society, EMBS , 2018, vol. 2018-July. doi: https://doi.org/10.1109/EMBC.2018.8512930 .
Yıldırım, Ö., Baloglu, U. B. & Rajendra Acharya, U. A deep convolutional neural network model for automated identification of abnormal EEG signals. Neural Comput. Appl. 32 (20), 15857–15868. https://doi.org/10.1007/s00521-018-3889-z (2020).
Boonyakitanont, P., Lek-uthai, A., Chomtho, K. & Songsiri, J. A review of feature extraction and performance evaluation in epileptic seizure detection using EEG. Biomed. Signal Process. Control 57 , 101702. https://doi.org/10.1016/j.bspc.2019.101702 (2020).
Rajendra Acharya, U., Vinitha Sree, S., Swapna, G., Martis, R. J. & Suri, J. S. Automated EEG analysis of epilepsy: A review. Knowl.-Based Syst. 45 , 147–165. https://doi.org/10.1016/j.knosys.2013.02.014 (2013).
Sharma, R., Sircar, P. & Pachori, R. B. A new technique for classification of focal and nonfocal EEG signals using higher-order spectra. J. Mech. Med. Biol. 19 (01), 1940010. https://doi.org/10.1142/S0219519419400104 (2019).
Teplan, M. Fundamentals of EEG measurement. Measurement science review. Meas. Sci. Rev. 2(2), (2002).
Lopez, S., Suarez, G., Jungreis, D., Obeid, I. & Picone, J. Automated identification of abnormal adult EEGs. https://doi.org/10.1109/SPMB.2015.7405423 (2016).
Sharan, R. V. & Berkovsky, S. Epileptic Seizure Detection Using Multi-Channel EEG Wavelet Power Spectra and 1-D Convolutional Neural Networks. in Proceedings of the Annual International Conference of the IEEE Engineering in Medicine and Biology Society, EMBS , 2020, vol. 2020-July. https://doi.org/10.1109/EMBC44109.2020.9176243 .
Li, M., Sun, X. & Chen, W. Patient-specific seizure detection method using nonlinear mode decomposition for long-term EEG signals. Med. Biol. Eng. Comput. https://doi.org/10.1007/s11517-020-02279-6 (2020).
Hu, X. et al. Scalp EEG classification using deep Bi-LSTM network for seizure detection. Comput. Biol. Med. https://doi.org/10.1016/j.compbiomed.2020.103919 (2020).
Ryu, S. et al. Pilot study of a single-channel EEG seizure detection algorithm using machine learning. Child’s Nerv. Syst. https://doi.org/10.1007/s00381-020-05011-9 (2021).
Altmann, A., Toloşi, L., Sander, O. & Lengauer, T. Permutation importance: A corrected feature importance measure. Bioinformatics https://doi.org/10.1093/bioinformatics/btq134 (2010).
Li, J. et al. Feature selection: A data perspective. ACM Comput. Surv. https://doi.org/10.1145/3136625 (2017).
Peng, G., Nourani, M., Harvey, J. & Dave, H. Feature Selection Using F-statistic Values for EEG Signal Analysis. in Proceedings of the Annual International Conference of the IEEE Engineering in Medicine and Biology Society, EMBS , 2020, vol. 2020-July. https://doi.org/10.1109/EMBC44109.2020.9176434 .
Tzimourta, K. D. et al. A robust methodology for classification of epileptic seizures in EEG signals. Health Technol. https://doi.org/10.1007/s12553-018-0265-z (2019).
Raghu, S., Sriraam, N., Temel, Y., Rao, S. V. & Kubben, P. L. EEG based multi-class seizure type classification using convolutional neural network and transfer learning. Neural Netw. https://doi.org/10.1016/j.neunet.2020.01.017 (2020).
Download references
Acknowledgements
This study was supported by the facilities the provided by New Jersey Institute of Technology, NJ, USA; Florida International University, FL, USA; University of Cincinnati, OH, USA, and Khulna University of Engineering & Technology, Bangladesh.
Author information
Authors and affiliations.
Department of Biomedical Engineering (BME), New Jersey Institute of Technology, Newark, NJ, USA
Torikul Islam
Department of Biomedical Engineering (BME), Khulna University of Engineering & Technology, Khulna, 9230, Bangladesh
Torikul Islam, Redwanul Islam, Monisha Basak, Amit Dutta Roy & Sk. Rahat Ali
Department of Biomedical Engineering (BME), Florida International University, Miami, FL, USA
Md. Adil Arman & Oleksii Shandra
Department of Biomedical Engineering (BME), University of Cincinnati, Cincinnati, OH, USA
Samanta Paul
You can also search for this author in PubMed Google Scholar
Contributions
T.I.: Conceptualization, Investigation, Formal analysis, Methodology, Software, Validation, Resources, Visualization, Project administration, Writing—original draft, Writing—review & editing. R.I.: Investigation, Methodology, Software, Validation, Visualization. M.B.: Investigation, Methodology, Software, Validation, Visualization. A.D.R.: Conceptualization, Data curation, Supervision, Writing—review & editing. M.A.A.: Investigation. S.P.: Investigation. O.S.: Writing—review & editing. S.R.A.: Writing—review & editing.
Corresponding author
Correspondence to Torikul Islam .
Ethics declarations
Competing interests.
The authors declare no competing interests.
Additional information
Publisher's note.
Springer Nature remains neutral with regard to jurisdictional claims in published maps and institutional affiliations.
Supplementary Information
Supplementary information., rights and permissions.
Open Access This article is licensed under a Creative Commons Attribution 4.0 International License, which permits use, sharing, adaptation, distribution and reproduction in any medium or format, as long as you give appropriate credit to the original author(s) and the source, provide a link to the Creative Commons licence, and indicate if changes were made. The images or other third party material in this article are included in the article's Creative Commons licence, unless indicated otherwise in a credit line to the material. If material is not included in the article's Creative Commons licence and your intended use is not permitted by statutory regulation or exceeds the permitted use, you will need to obtain permission directly from the copyright holder. To view a copy of this licence, visit http://creativecommons.org/licenses/by/4.0/ .
Reprints and permissions
About this article
Cite this article.
Islam, T., Islam, R., Basak, M. et al. Performance investigation of epilepsy detection from noisy EEG signals using base-2-meta stacking classifier. Sci Rep 14 , 10792 (2024). https://doi.org/10.1038/s41598-024-61338-2
Download citation
Received : 17 January 2024
Accepted : 04 May 2024
Published : 11 May 2024
DOI : https://doi.org/10.1038/s41598-024-61338-2
Share this article
Anyone you share the following link with will be able to read this content:
Sorry, a shareable link is not currently available for this article.
Provided by the Springer Nature SharedIt content-sharing initiative
- Base-2-Meta stacking classifier
- Machine learning
- Feature extraction
- Feature ranking
By submitting a comment you agree to abide by our Terms and Community Guidelines . If you find something abusive or that does not comply with our terms or guidelines please flag it as inappropriate.
Quick links
- Explore articles by subject
- Guide to authors
- Editorial policies
Sign up for the Nature Briefing newsletter — what matters in science, free to your inbox daily.

Epilepsy Society
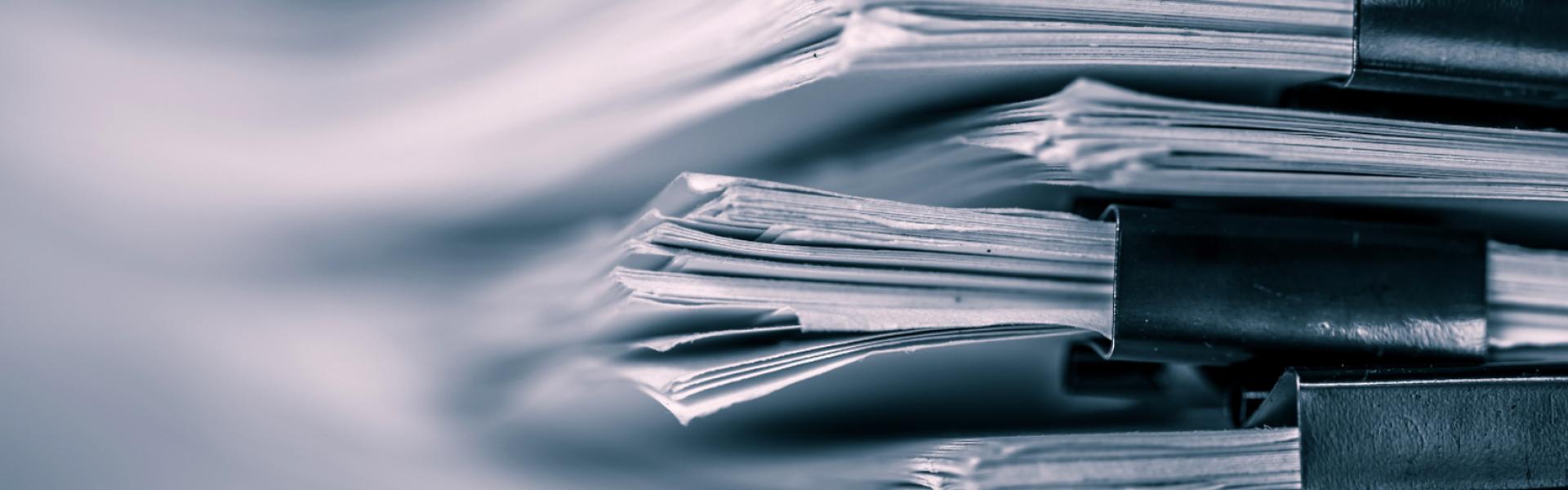
Research papers
Please find below a lay summary of research recently published in open access journal by staff based at the Chalfont Centre for Epilepsy.
breadcrumb navigation:
- What we do /
- current page Research papers
Published on 23 February 2020
Updated: 12 July 2022
Authored by Anonymous
Perampanel in routine clinical use across Europe: Pooled, multicenter, observational data.
Authors Rohracher A, Zimmermann G, Villanueva V, Garamendi I, Sander JW, et al.
Journal details Epilepsia. 2018; 59:1727-1739.
Lay summary One of the limitations of clinical trials is related to the difficulty to interpret or generalize the results because the studied population is very different from the population treated in normal life. In this report the researchers aimed to generate real-world evidence of health information from data reflecting routine clinical use over the past 4 years; from a wide variety of people with epilepsy, including those typically underrepresented such as the elderly members of the population who are being treated with the antiepileptic drug Perampanel (sold under the trade name Fycompa). Information was collected from over 2300 patients who had been prescribed this medicine from over 45 centres across Europe. The Perampanel related adverse side effects found in this study were similar to previous reports documenting routine clinical use, no new Perampanel related side effects were found in this study, adverse side effects such as dizziness were less frequent in people, than those events reported in the clinical trials. It was concluded that Perampanel was effective in routine clinical use in a wide variety of people whose epilepsy was not controlled.
The landscape of epilepsy-related GATOR1 variants
Authors Baldassari S, Picard F, Verbeek NE, van Kempen M, Brilstra EH, Lesca G, Conti V, Guerrini R, Bisulli F, Licchetta L, Pippucci T, Tinuper P, Hirsch E, de Saint Martin A, Chelly J, Rudolf G, Chipaux M, Ferrand-Sorbets S, Dorfmüller G, Sisodiya S, Balestrini S, Schoeler N, Hernandez- Hernandez L, Krithika S, et al
Journal details Genet Med. 2019; 21:398-408
Lay summary The objective of this study was to gain further understanding of the three genes that are involved in forming the GATOR1 protein complex (GAP activity towards rags complex 1); mutations in these genes are known to play a role in causing focal epilepsies. The researchers extracted DNA of 73 people with focal epilepsy and looked for changes in these three genes. The results of this study enabled detailed characterisation of epilepsy features related to GATOR1 protein complex. Additionally, it provided updated information to researchers and clinicians on all previously reported and novel GATOR1 epilepsy-related gene changes complete with new guidance for their clinical interpretation.
Genome-wide association study: Exploring the genetic basis for responsiveness to ketogenic dietary therapies for drug- resistant epilepsy.
Authors Schoeler NE, Leu C, Balestrini S, Mudge JM, Steward CA, Frankish A, Leung MA, Mackay M, Scheffer I, Williams R, Sander JW, Cross JH, Sisodiya SM.
Journal details Epilepsia. 2018; 59:1557-1566.
Lay summary Ketogenic dietary therapies (KDTs) are a group of high‐fat, low‐carbohydrate diets that have been used effectively as treatment options for people with drug resistant epilepsy since the early 1900s. We know that certain epilepsies, such as epilepsy with myoclonic‐atonic seizures, tuberous sclerosis complex, and Dravet syndrome, generally respond well to KDTs. However, KDTs are resource‐intensive, require dietary restriction, and can cause adverse side effects. Therefore the aim of this study was to identify a way to predict those who are likely to respond well and those who will not respond to KDTs in order to enable this dietary treatment earlier in the course of epilepsy.
In this study the researchers used a technique called genotyping in 272 patients to look for any specific signals such as changes in the DNA potentially associated with their responses to KDTs. A specific change in a gene called chromodomain Y like (CDYL) was found to be associated in individuals with poor response to KDT, where seizures were not under control. The CDYL gene is known to play a role in neural activities such as migration and therefore is a good candidate to study in more detail.
Effects of carbamazepine and lamotrigine on functional magnetic resonance imaging cognitive networks.
Authors Xiao F, Caciagli L, Wandschneider B, Sander JW, Sidhu M, Winston G, Burdett J, Trimmel K, Hill A, Vollmar C, Vos SB, Ourselin S, Thompson PJ, Zhou D, Duncan JS, Koepp MJ.
Journal details Epilepsia. 2018; 59:1362-1371
Lay summary This investigation took an imaging approach to study the effects of three antiepileptic drugs, Carbamazepine, Lamotrigine and Levetiracetam, on cognitive abilities of people with epilepsy. The 7 year records of functional magnetic resonance imaging (fMRI) brain scans of adult patients, who were fluent in English, were evaluated as part of their pre-surgical evaluation at the National Hospital for Neurology and Neurosurgery and Chalfont Centre for Epilepsy (UK). Activities in brain regions of patients and healthy volunteers were measured using their fMRI scans. Researchers found changes in patterns of activities involved in language tasks in patients on Lamotrigine and those on Carbamazepine showed more pronounced impairment of performance than those on lamotrigine. This study indicated that patients on Carbamazepine perform less well on a verbal fluency tests than those taking Lamotrigine and Levetiracetam. These important findings enhanced understanding of the effects of these medicines on the cognition of people with epilepsy.
Genome-wide mega-analysis identifies 16 loci and highlights diverse biological mechanisms in the common epilepsies
Authors Sander JW, Sisodiya SM.
Journal details International League Against Epilepsy Consortium on Complex Epilepsies. Nat Communications. 2018; 9:5269.
Lay summary This large study was conducted by the International League Against Epilepsy Consortium to help further the understanding of the genetics of the both focal and generalised epilepsies. The researchers compared the genomes of over 15,000 people with epilepsy with the genomes from over 29,000 healthy controls using a technique called genotyping.
Results of this study confirmed association of 16 regions of the genome with common epilepsies. One of these regions was a new association with juvenile myoclonic epilepsy and two novel regions were shown to be associated with focal epilepsy with hippocampal sclerosis. A total of 21 candidate epilepsy genes detected in these regions were studied further, to understand specific variants in these genes and the underlying biological mechanisms, and found a wide range of functions carried out by the products of these genes in the brain. Collectively, their findings provide new therapeutic leads that could potentially develop into drugs for treating common types of epilepsy.
MRI PhD theses
The following are PhD theses from the ES MRI Epilepsy Imaging Group:
- Vejay Vakharia. Computer assisted planning and robotics in epilepsy surgery.
- Bianca DeBlasi. Multi-parametric Imaging Using Hybrid PET/MR to Investigate the Epileptogenic Brain
- Lorenzo Caciagli - Neuroimaging of epilepsy: disease severity, cognitive comorbidities and endophenotypes
- Mark Nowell - Novel multimodality imaging in the planning and surgical treatment of epilepsy
- Christian Vollmar - Neuroimaging of functional and structural alterations in juvenile myoclonic epilepsy and frontal lobe epilepsy
- Britta Wandschneider - The effects of genes, antiepileptic drugs and risk of death on functional anatomy and cognitive networks in epilepsy
- Maria Feldmann - Imaging p-glycoprotein function: prediction of treatment response in mesial temporal lobe epilepsy
- Meneka Sidhu - Episodic memory in temporal lobe epilepsy
- Silvia Bonelli-Nauer - Cognitive functional MRI in temporal lobe epilepsy
- Helmut Laufs - EEG-fMRI signatures of spontaneous brain activity in healthy volunteers and epilepsy patients
- Rachel Thornton - Imaging brain networks in focal epilepsy : a prospective study of the clinical application of simultaneous EEG-fMRI in pre-surgical evaluation
- Gavin Winston - Translation of novel imaging techniques into clinical use for patients with epilepsy
- Mahinda Yogarajah - Imaging structural connections of the brain in epilepsy
- Umair Chaudhary - Haemodynamic correlates of interictal and ictal epileptic discharges and ictal semiology using simultaneous scalp video-EEG-fMRI and intracranial EEG-fMRI
- Robert Simister - Magnetic Resonance Spectroscopy as applied to epilepsy
- Serge Vulliemoz - Imaging functional and structural networks in the human epileptic brain
- Beate Diehl - Imaging correlates of the epileptogenic zone and functional deficit zone using diffusion tensor imaging (DTI)
- Khalid Hamandi - Functional MRI of focal and generalised interictal epileptiform discharges
- Afraim Salek-Haddadi - EEG-correlated functional MRI in epilepsy
- Rebecca Samson - Optimisation of quantitative magnetisation transfer (qMT) MRI to study restricted protons in the living human brain
- Robert Powell - Investigating brain structure and function in temporal lobe epilepsy
- Karsten Krakow - Imaging of epileptic activity using EEG-correlated functional MRI
- Rebecca Liu - Investigation of secondary cerebral damage in epilepsy
- Tejal Mitchell - Quantitative MRI in cerebral development disorders and epilepsy
- Fergus Rugg-Gunn - Imaging the neocortex in epilepsy with advanced MRI techniques
- Alex Everitt - The structural basis of the epilepsies: MRI and epidemiological studies
- Alexander Hammers - PET investigations in focal epilepsy
- Udo Wieshmann - New MR imaging techniques in epilepsy
- Matthias Koepp - Central benzodiazepine receptors in hippocampal sclerosis and idiopathic generalised epilepsies and opioid receptors in reading epilepsy
- Mark Richardson - Positron emission tomography investigation of cortical malformations causing epilepsy
- Wim van Paesschen - Quantitative MRI and hippocampal neuropathology of temporal lobe epilepsy
- Sanjay Sisodiya - Qualitative and quantitative analysis of MRI data from patients with epilepsy
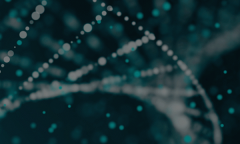
Read how we are working to understand the genetic architecture of each individual person's epilepsy through our world leading genomics research programme.
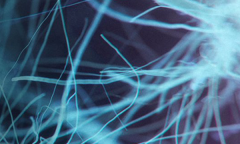
Neuroimaging
Neuroimaging enables us to look deep inside the brain to learn more about the impact of seizures on its structure and function.
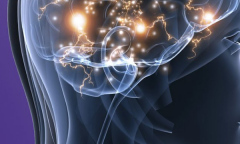
Neuropathology
The Epilepsy Society Brain and Tissue Bank is the first of its kind in the UK. It is dedicated to the study of epilepsy through brain and other tissue samples.
Enhanced Epileptic Seizure Detection Through Graph Spectral Analysis of EEG Signals
- Published: 18 May 2024
Cite this article
- Ramnivas Sharma ORCID: orcid.org/0000-0002-3411-6197 1 &
- Hemant Kumar Meena 1
72 Accesses
Explore all metrics
Epilepsy is a persistent health condition marked by unusual and highly synchronized electrical activity in the brain cells, resulting in recurring seizures. This paper proposes a novel real-time method to improve the detection of seizures using the spectral features of non-stationary electroencephalogram (EEG) signals. It is observed that the discrete wavelet transform (DWT)-based features do not consider the interrelationship among EEG signal components. This interrelationship has been well captured by the novel representation of EEG in the form of graph signals. Here, the spectral analysis of the graph signals is investigated by the graph-based Fourier transform (GFT). Then, GFT-based features have been selected and fed into different classifiers for analysis. The seizure detection rate in two publicly available EEG-based datasets, the University of Bonn (UB) and the Neurology Sleep Clinic New Delhi (NSC-ND), have been achieved with accuracy of 98.68% and 96.84%, respectively. The accuracy achieved is significantly better than the existing state-of-the-art techniques. This approach demonstrates the impact of utilizing the interrelationship among the EEG components, followed by enhanced feature selection based on GFT for the improved detection of seizures.
This is a preview of subscription content, log in via an institution to check access.
Access this article
Price excludes VAT (USA) Tax calculation will be finalised during checkout.
Instant access to the full article PDF.
Rent this article via DeepDyve
Institutional subscriptions
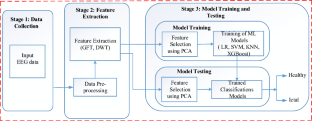
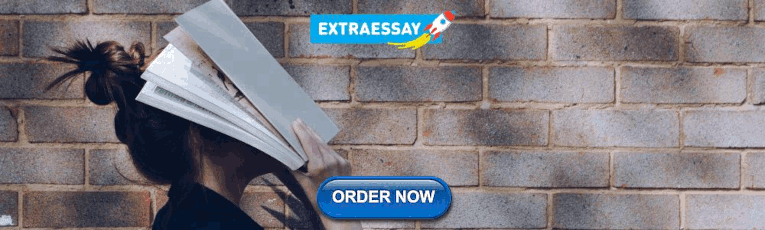
Similar content being viewed by others
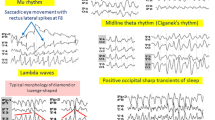
Overcoming traps and pitfalls leading to misinterpretation of normal EEG variants and variation of the background activity
Investigation of Alzheimer’s Disease EEG Frequency Components with Lempel-Ziv Complexity
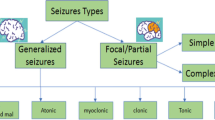
EEG seizure detection: concepts, techniques, challenges, and future trends
Data availability.
Publicly available data has been referred to in this paper.
U.R. Acharya, F. Molinari, S.V. Sree, S. Chattopadhyay, K.-H. Ng, J.S. Suri, Automated diagnosis of epileptic EEG using entropies. Biomed. Signal Process. Control 7 (4), 401–408 (2012)
Article Google Scholar
U.R. Acharya, S.L. Oh, Y. Hagiwara, J.H. Tan, H. Adeli, Deep convolutional neural network for the automated detection and diagnosis of seizure using EEG signals. Comput. Biol. Med. 100 , 270–278 (2018)
B. Akbarian, A. Erfanian, A framework for seizure detection using effective connectivity, graph theory, and multi-level modular network. Biomed. Signal Process. Control 59 , 101878 (2020)
R.G. Andrzejak, K. Lehnertz, F. Mormann, C. Rieke, P. David, C.E. Elger, Indications of nonlinear deterministic and finite-dimensional structures in time series of brain electrical activity: Dependence on recording region and brain state. Phys. Rev. E 64 (6), 061907 (2001)
A. Anuragi, D.S. Sisodia, R.B. Pachori, Automated fbse-ewt based learning framework for detection of epileptic seizures using time-segmented EEG signals. Comput. Biol. Med. 136 , 104708 (2021)
S.M. Beeraka, A. Kumar, M. Sameer, S. Ghosh, B. Gupta, Accuracy enhancement of epileptic seizure detection: a deep learning approach with hardware realization of stft. Circuits Syst. Signal Process. 41 , 461–484 (2022)
W. Bomela, S. Wang, C.-A. Chou, J.-S. Li, Real-time inference and detection of disruptive EEG networks for epileptic seizures. Sci. Rep. 10 (1), 8653 (2020)
M. Chakraborty, D. Mitra et al., Epilepsy seizure detection using kurtosis based vmd’s parameters selection and bandwidth features. Biomed. Signal Process. Control 64 , 102255 (2021)
G. Chen, Automatic EEG seizure detection using dual-tree complex wavelet-Fourier features. Expert Syst. Appl. 41 (5), 2391–2394 (2014)
T. Chen and C. Guestrin. Xgboost: A scalable tree boosting system, in proceedings of the 22nd acm sigkdd international conference on knowledge discovery and data mining , (San Francisco, California, 2016) pp. 785–794
M. Cheung, J. Shi, O. Wright, L.Y. Jiang, X. Liu, J.M. Moura, Graph signal processing and deep learning: convolution, pooling, and topology. IEEE Signal Process. Mag. 37 (6), 139–149 (2020)
N. Darjani, H. Omranpour, Phase space elliptic density feature for epileptic EEG signals classification using metaheuristic optimization method. Knowl.-Based Syst. 205 , 106276 (2020)
M. Defferrard, X. Bresson, P. Vandergheynst, Convolutional neural networks on graphs with fast localized spectral filtering. Adv. Neural. Inf. Process. Syst. 29 (2016)
N. Fatma, P. Singh, and M. K. Siddiqui. An in-depth examination of machine learning approaches for detecting epileptic seizures, in 2024 International Conference on Automation and Computation (AUTOCOM) , (IEEE, 2024) pp. 507–511
P. Ghaderyan, A. Abbasi, M.H. Sedaaghi, An efficient seizure prediction method using knn-based undersampling and linear frequency measures. J. Neurosci. Methods 232 , 134–142 (2014)
V. Gupta, R.B. Pachori, Epileptic seizure identification using entropy of fbse based EEG rhythms. Biomed. Signal Process. Control 53 , 101569 (2019)
U. Herwig, P. Satrapi, C. Schönfeldt-Lecuona, Using the international 10–20 EEG system for positioning of transcranial magnetic stimulation. Brain Topogr. 16 , 95–99 (2003)
M.-P. Hosseini, A. Hosseini, K. Ahi, A review on machine learning for EEG signal processing in bioengineering. IEEE Rev. Biomed. Eng. 14 , 204–218 (2020)
M. Hossin, M.N. Sulaiman, A review on evaluation metrics for data classification evaluations. Int. J. Data Min. Knowl. Manag. Process 5 (2), 1 (2015)
W. Hu, J. Cao, X. Lai, J. Liu, Mean amplitude spectrum based epileptic state classification for seizure prediction using convolutional neural networks. J. Ambient Intell. Hum. Comput. 14 (11), 15485–15495 (2019)
S. Jang, S.-E. Moon, and J.-S. Lee. EEG-based video identification using graph signal modeling and graph convolutional neural network, in 2018 IEEE international conference on acoustics, speech and signal processing (ICASSP) , (IEEE, 2018), pp. 3066–3070
K. Jindal, R. Upadhyay, H.S. Singh, Application of tunable-q wavelet transform based nonlinear features in epileptic seizure detection. Analog Integr. Circ. Sig. Process 100 , 437–452 (2019)
J. Jing, X. Pang, Z. Pan, F. Fan, Z. Meng, Classification and identification of epileptic EEG signals based on signal enhancement. Biomed. Signal Process. Control 71 , 103248 (2022)
V. Joshi, R.B. Pachori, A. Vijesh, Classification of ictal and seizure-free EEG signals using fractional linear prediction. Biomed. Signal Process. Control 9 , 1–5 (2014)
A.A. Khalil, M.I. El Sayeid, F.E. Ibrahim, A.A. Khalaf, E. Gemeay, H. Kasem, S.E.A. Khamis, G.M. El-Banby, W. El-Shafai, E.-S.M. El-Rabaie et al., Efficient frameworks for statistical seizure detection and prediction. J. Supercomput. 79 (16), 17824–17858 (2023)
A. Khosla, P. Khandnor, T. Chand, A comparative analysis of signal processing and classification methods for different applications based on EEG signals. Biocybern. Biomed. Eng. 40 (2), 649–690 (2020)
R. Li, X. Yuan, M. Radfar, P. Marendy, W. Ni, T.J. O’Brien, P.M. Casillas-Espinosa, Graph signal processing, graph neural network and graph learning on biological data: a systematic review. IEEE Rev. Biomed. Eng. 16 , 109–135 (2021)
Y. Liang, C. Chen, F. Li, D. Yao, P. Xu, L. Yu et al., Altered functional connectivity after epileptic seizure revealed by scalp EEG. Neural Plast. (2020). https://doi.org/10.1155/2020/8851415
J. Mateo-Sotos, A. Torres, J. Santos, O. Quevedo, C. Basar, A machine learning-based method to identify bipolar disorder patients. Circuits Syst. Signal Process. 41 (4), 2244–2265 (2022)
P. Mathur and V. K. Chakka. Graph signal processing of EEG signals for detection of epilepsy, in 2020 7th International Conference on Signal Processing and Integrated Networks (SPIN) , (IEEE, 2020), pp. 839–843
M. Nourelahi, A. Zamani, A. Talei, S. Tahmasebi, A model to predict breast cancer survivability using logistic regression. Middle East J. Cancer 10 (2), 132–138 (2019)
Google Scholar
M. Omidvar, A. Zahedi, H. Bakhshi, EEG signal processing for epilepsy seizure detection using 5-level db4 discrete wavelet transform, ga-based feature selection and ann/svm classifiers. J. Ambient Intell. Hum. Comput. 12 (11), 10395–10403 (2021)
R.J. Oweis, E.W. Abdulhay, Seizure classification in EEG signals utilizing Hilbert–Huang transform. Biomed. Eng. Online 10 , 1–15 (2011)
H. Peng, C. Lei, S. Zheng, C. Zhao, C. Wu, J. Sun, B. Hu, Automatic epileptic seizure detection via stein kernel-based sparse representation. Comput. Biol. Med. 132 , 104338 (2021)
S. Poorani, P. Balasubramanie, Seizure detection based on EEG signals using asymmetrical back propagation neural network method. Circuits Syst. Signal Process. 40 (9), 4614–4632 (2021)
S.M. Qaisar, S.F. Hussain, Effective epileptic seizure detection by using level-crossing EEG sampling sub-bands statistical features selection and machine learning for mobile healthcare. Comput. Methods Programs Biomed. 203 , 106034 (2021)
X. Qin, D. Xu, X. Dong, X. Cui, S. Zhang, EEG signal classification based on improved variational mode decomposition and deep forest. Biomed. Signal Process. Control 83 , 104644 (2023)
K. Rasheed, A. Qayyum, J. Qadir, S. Sivathamboo, P. Kwan, L. Kuhlmann, T. O’Brien, A. Razi, Machine learning for predicting epileptic seizures using EEG signals: a review. IEEE Rev. Biomed. Eng. 14 , 139–155 (2020)
E.A. Refaee, S. Shamsudheen, A computing system that integrates deep learning and the internet of things for effective disease diagnosis in smart health care systems. J. Supercomput. 78 (7), 9285–9306 (2022)
S.K. Rout, P.K. Biswal, An efficient error-minimized random vector functional link network for epileptic seizure classification using vmd. Biomed. Signal Process. Control 57 , 101787 (2020)
S. Rukmawan, F. Aszhari, Z. Rustam, J. Pandelaki, Cerebral infarction classification using the k-nearest neighbor and naive Bayes classifier. J. Phys. Conf. Ser. 1752 , 012045 (2021)
J. Saeedi, K. Faez, M.H. Moradi, Hybrid fractal-wavelet method for multi-channel EEG signal compression. Circuits Syst. Signal Process. 33 , 2583–2604 (2014)
N. Sairamya, S.T. George, D.N. Ponraj, M. Subathra, Detection of epileptic eeg signal using improved local pattern transformation methods. Circuits Syst. Signal Process. 37 , 5554–5575 (2018)
M. Savadkoohi, T. Oladunni, L. Thompson, A machine learning approach to epileptic seizure prediction using electroencephalogram EEG signal. Biocybern. Biomed. Eng. 40 (3), 1328–1341 (2020)
G. Sharma, A. Parashar, A.M. Joshi, DepHNN: a novel hybrid neural network for electroencephalogram EEG-based screening of depression. Biomed. Signal Process. Control 66 , 102393 (2021)
M. Shen, P. Wen, B. Song, Y. Li, An EEG based real-time epilepsy seizure detection approach using discrete wavelet transform and machine learning methods. Biomed. Signal Process. Control 77 , 103820 (2022)
D.I. Shuman, S.K. Narang, P. Frossard, A. Ortega, P. Vandergheynst, The emerging field of signal processing on graphs: Extending high-dimensional data analysis to networks and other irregular domains. IEEE Signal Process. Mag. 30 (3), 83–98 (2013)
T. Song, W. Zheng, P. Song, Z. Cui, EEG emotion recognition using dynamical graph convolutional neural networks. IEEE Trans. Affect. Comput. 11 (3), 532–541 (2018)
L. Stanković, M. Daković, E. Sejdić, Introduction to graph signal processing, in Vertex-frequency analysis of graph signals . ed. by L. Stanković, M. Daković, E. Sejdić (Springer, Berlin, 2019), pp.3–108
Chapter Google Scholar
P. Swami, T.K. Gandhi, B.K. Panigrahi, M. Tripathi, S. Anand, A novel robust diagnostic model to detect seizures in electroencephalography. Expert Syst. Appl. 56 , 116–130 (2016)
P. Swami, B. Panigrahi, S. Nara, M. Bhatia, T. Gandhi. EEG epilepsy datasets. (2016c). https://doi. org/10.13140/RG,2(14280.32006)
E. Tuncer, E.D. Bolat, Classification of epileptic seizures from electroencephalogram EEG data using bidirectional short-term memory (bi-lstm) network architecture. Biomed. Signal Process. Control 73 , 103462 (2022)
J. Wu, T. Zhou, T. Li, Detecting epileptic seizures in EEG signals with complementary ensemble empirical mode decomposition and extreme gradient boosting. Entropy 22 (2), 140 (2020)
W. Zhao, W. Zhao, W. Wang, X. Jiang, X. Zhang, Y. Peng, B. Zhang, G. Zhang, A novel deep neural network for robust detection of seizures using EEG signals. Comput. Math. Methods Med. (2020). https://doi.org/10.1155/2020/9689821
Download references
No Funding is involved.
Author information
Authors and affiliations.
Department of Electrical Engineering, Malaviya National Institute of Technology, Jaipur, Rajasthan, 302017, India
Ramnivas Sharma & Hemant Kumar Meena
You can also search for this author in PubMed Google Scholar
Contributions
Ramnivas Sharma: Original draft, Software, Review and editing of the paper, Formal analysis, and Results obtained.
Corresponding author
Correspondence to Ramnivas Sharma .
Ethics declarations
Conflict of interest.
No competing financial or interpersonal conflicts.
Ethical Approval
Not involve any studies with animals or humans.
Consent to Participants
Human participants are not involved.
Additional information
Publisher's note.
Springer Nature remains neutral with regard to jurisdictional claims in published maps and institutional affiliations.
Rights and permissions
Springer Nature or its licensor (e.g. a society or other partner) holds exclusive rights to this article under a publishing agreement with the author(s) or other rightsholder(s); author self-archiving of the accepted manuscript version of this article is solely governed by the terms of such publishing agreement and applicable law.
Reprints and permissions
About this article
Sharma, R., Meena, H.K. Enhanced Epileptic Seizure Detection Through Graph Spectral Analysis of EEG Signals. Circuits Syst Signal Process (2024). https://doi.org/10.1007/s00034-024-02715-0
Download citation
Received : 01 November 2023
Revised : 25 April 2024
Accepted : 26 April 2024
Published : 18 May 2024
DOI : https://doi.org/10.1007/s00034-024-02715-0
Share this article
Anyone you share the following link with will be able to read this content:
Sorry, a shareable link is not currently available for this article.
Provided by the Springer Nature SharedIt content-sharing initiative
- EEG signals
- Discrete wavelet transform
- Graph Fourier transform
- Machine learning algorithms
- Find a journal
- Publish with us
- Track your research

An official website of the United States government
The .gov means it’s official. Federal government websites often end in .gov or .mil. Before sharing sensitive information, make sure you’re on a federal government site.
The site is secure. The https:// ensures that you are connecting to the official website and that any information you provide is encrypted and transmitted securely.
- Publications
- Account settings
Preview improvements coming to the PMC website in October 2024. Learn More or Try it out now .
- Advanced Search
- Journal List
- Int J Environ Res Public Health

Epileptic Seizures Detection Using Deep Learning Techniques: A Review
Afshin shoeibi.
1 Faculty of Electrical Engineering, Biomedical Data Acquisition Lab (BDAL), K. N. Toosi University of Technology, Tehran 1631714191, Iran; ri.ca.mu.liam@imessahgdivan
2 Computer Engineering Department, Ferdowsi University of Mashhad, Mashhad 9177948974, Iran; [email protected] (D.A.); ri.ca.mu@inahuor (M.R.)
Marjane Khodatars
3 Mashhad Branch, Islamic Azad University, Mashhad 91735413, Iran; moc.liamg@enajram1sratadohk
Navid Ghassemi
Mahboobeh jafari.
4 Electrical and Computer Engineering Faculty, Semnan University, Semnan 3513119111, Iran; [email protected]
Parisa Moridian
5 Faculty of Engineering, Science and Research Branch, Islamic Azad University, Tehran 1477893855, Iran; moc.oohay@naidiromasirap
Roohallah Alizadehsani
6 Institute for Intelligent Systems Research and Innovation (IISRI), Deakin University, Waurn Ponds, VIC 3217, Australia; ua.ude.nikaed@inashedazilar (R.A.); ri.ca.smum@hemiezohk (F.K.); [email protected] (A.K.); [email protected] (S.N.)
Maryam Panahiazar
7 Institute for Computational Health Sciences, School of Medicine, University of California, San Francisco, CA 94143, USA; [email protected]
Fahime Khozeimeh
8 Faculty of Electrical Engineering, Gonabad Branch, Islamic Azad University, Gonabad 6518115743, Iran; moc.liamg@erazfessa
Hossein Hosseini-Nejad
9 Faculty of Electrical and Computer Engineering, K. N. Toosi University of Technology, Tehran 1631714191, Iran; ri.ca.utnk.dtee@dahzen_iniessoh
Abbas Khosravi
Amir f. atiya.
10 Department of Computer Engineering, Faculty of Engineering, Cairo University, Cairo 12613, Egypt; ude.oirac@ayita
Diba Aminshahidi
Sadiq hussain.
11 System Administrator at Dibrugarh University, Assam 786004, India; ni.ca.urbid@qidas
Modjtaba Rouhani
Saeid nahavandi, udyavara rajendra acharya.
12 Department of Biomedical Engineering, School of Science and Technology, Singapore University of Social Sciences, Singapore 599494, Singapore; gs.ude.pn@ura
13 Department of Electronics and Computer Engineering, Ngee Ann Polytechnic, Singapore 599489, Singapore
14 Department of Bioinformatics and Medical Engineering, Taichung City 41354, Taiwan
Associated Data
Not applicable.
A variety of screening approaches have been proposed to diagnose epileptic seizures, using electroencephalography (EEG) and magnetic resonance imaging (MRI) modalities. Artificial intelligence encompasses a variety of areas, and one of its branches is deep learning (DL). Before the rise of DL, conventional machine learning algorithms involving feature extraction were performed. This limited their performance to the ability of those handcrafting the features. However, in DL, the extraction of features and classification are entirely automated. The advent of these techniques in many areas of medicine, such as in the diagnosis of epileptic seizures, has made significant advances. In this study, a comprehensive overview of works focused on automated epileptic seizure detection using DL techniques and neuroimaging modalities is presented. Various methods proposed to diagnose epileptic seizures automatically using EEG and MRI modalities are described. In addition, rehabilitation systems developed for epileptic seizures using DL have been analyzed, and a summary is provided. The rehabilitation tools include cloud computing techniques and hardware required for implementation of DL algorithms. The important challenges in accurate detection of automated epileptic seizures using DL with EEG and MRI modalities are discussed. The advantages and limitations in employing DL-based techniques for epileptic seizures diagnosis are presented. Finally, the most promising DL models proposed and possible future works on automated epileptic seizure detection are delineated.
1. Introduction
Epilepsy is a noncommunicable disease and one of the most common neurological disorders of humans, usually associated with sudden attacks [ 1 ]. Sudden attacks of seizures are a swift and early abnormality in the electrical activity of the brain that disrupts the part or whole body [ 2 ]. Various kinds of epileptic seizures are affecting around 60 million people worldwide [ 3 ]. These attacks occasionally provoke cognitive disorders that can cause severe physical injury to the patient. Moreover, people with epileptic seizures sometimes suffer emotional distress due to embarrassment and lack of appropriate social status. Hence, early detection of epileptic seizures can help the patients and improve their quality of life.
Screening techniques in the diagnosis of epileptic seizures comprise two important categories of functional and structural neuroimaging modalities [ 4 , 5 , 6 , 7 , 8 , 9 ]. The functional neuroimaging modality provides important information about brain function during epileptic seizure occurrence for physicians and neurologists [ 4 , 5 , 6 , 7 , 8 , 9 ]. The structural neuroimaging modalities provide physicians with substantial information about the brain structure of individuals with epileptic seizures [ 4 , 5 , 6 , 7 , 8 , 9 ]. The most important functional neuroimaging techniques are EEG [ 5 ], magnetoencephalography (MEG) [ 6 ], positron emission tomography (PET) [ 7 ], single-photon emission computed tomography (SPECT) [ 7 , 10 ], functional MRI (fMRI) [ 4 , 11 ], electrocorticography (ECoG) [ 12 ], and functional near-infrared spectroscopy (fNIRS) [ 13 ]. In contrast, structural MRI (sMRI) and diffusion tensor imaging (DTI) are among the most significant structural neuroimaging techniques [ 4 , 14 ]. In the diagnosis of epileptic seizures, functional neuroimaging modalities are more commonly applied than structural modalities [ 4 , 5 , 6 , 7 , 8 , 9 ]. Research on the diagnosis of epileptic seizures has indicated that EEG modalities are the most popular among physicians.
The EEG signals are widely preferred as they are economical, portable, and show clear rhythms in the frequency domain [ 8 , 9 ]. The EEG provides the voltage variations produced by the ionic current of neurons in the brain, which indicate the brain’s bioelectric activity [ 15 ]. They need to be recorded for a long period of time to detect epileptic seizures. In addition, these signals are recorded in multiple channels, making the analysis complex. The EEG signals are also prone to artifacts generated by main power supply, electrode movement, and muscle tremor [ 16 ]. This will pose challenges to the physicians to diagnose epileptic seizures using noisy EEG signals. To resolve these difficulties, much research is being carried out to diagnose and predict epileptic seizures based on EEG modalities and other techniques such as MRI coupled with AI techniques [ 17 , 18 ]. AI techniques in the field of epileptic seizures diagnosis have employed conventional machine learning and DL methods [ 19 , 20 , 21 , 22 ].
Many machine learning algorithms have been developed using statistical, time, frequency, time-frequency domain and nonlinear parameters to detect epileptic seizures [ 23 , 24 ]. In conventional machine learning techniques, the selection of features and classifiers is done by trial-and-error method [ 25 , 26 ]. One needs to have sound knowledge of signal processing and data mining techniques to develop an accurate model. Such models perform well for limited data. Nowadays, with the increase in the availability of data, machine learning techniques may not perform very well. Hence, the DL techniques, which are the state-of-art methods, have been employed [ 27 , 28 ]. DL models, unlike conventional machine learning techniques, require huge data in the training phase [ 29 ]. This is because these models have a large number of feature spaces, and in case of lack of data, they face the problem of overfitting [ 29 ].
In conventional machine learning algorithms, most simulations were executed in the Matlab software environment, but the DL models are usually developed using Python programming language with numerous open-source toolboxes. The python language with more freely available DL toolboxes has helped the researchers to develop novel automated systems, and there is greater accessibility of computation resource to everyone thanks to cloud computing. Figure 1 shows that the TensorFlow and one of its high-level APIs, Keras, are widely used for epileptic seizure detection using DL in reviewed works due to their versatility and applicability.

Number of times each DL tool was used for automated detection of epileptic seizure by various studies.
Since 2016, substantial research has been done to detect epilepsy using DL models such as convolutional neural networks (CNNs), recurrent neural networks (RNNs), deep belief networks (DBNs), Autoencoders (AEs), CNN-RNNs, and CNN-AEs [ 30 , 31 , 32 , 33 ]. The number of studies in this area using DL is growing as new efficient models are proposed. Figure 2 provides the overview of number of studies conducted using various DL models from 2014 to 2021 in detecting epileptic seizures.

Number of studies conducted using various DL models from 2014 until now (2021).
It can be noted from Figure 2 that various DL models have been exploited in the diagnosis of epileptic seizures. Compared to other DL techniques, 2D-CNN and 1D-CNN models are the most widely used in epileptic seizures detection. Researchers have mostly employed 2D-CNN models to diagnose epilepsy. In the diagnosis of epileptic seizures using 2D-CNN models, EEG signals are first converted into two-dimensional (2D) images using preprocessing methods such as short-time Fourier transform (STFT). Next, these images are applied to 2D-CNN networks. The second category comprises 1D-CNN models, which have achieved a special place among researchers for epileptic seizures detection. In this work, EEG signals are first preprocessed (noise removal and normalization) and then applied to 1D-CNN networks. Simple implementation and high efficiency are among the most important advantages of this type of network.
The keywords “EEG”, “MRI”, “Epilepsy”, “Epileptic Seizures”, and “Deep Learning” were used to search articles. These keywords were searched in various citation databases such as IEEE, Elsevier, Springer, Wiley, and ArXiv. Google Scholar was also used to search further. Figure 3 shows the number of accepted papers in each citation database. It is observed that the IEEE citation database contains the most accepted articles.

Search strategy used.
The main aims of this study are as follows:
- Providing information on available EEG datasets;
- Reviewing works done using various DL models for automated detection of epileptic seizures with various modality signals;
- Introducing future challenges on the detection of epileptic seizures;
- Analyzing the best performing model for various modalities of data.
Epileptic seizures detection using DL is discussed in Section 2 . Section 3 describes the non-EEG-based epileptic seizure detection. Hardware used for epileptic seizures detection is provided in Section 4 . Discussion on the paper is outlined in Section 5 . The challenges faced by employing DL methods for epileptic seizure detection are summarized in Section 6 . Finally, the conclusion and future work are delineated in Section 7 .
2. Epileptic Seizures Detection Based on DL Techniques
Figure 4 illustrates the working of a computer-aided diagnosis system (CADS) for epileptic seizures using DL architectures. The input to the DL model can be EEG, MEG, ECoG, fNIRS, PET, SPECT, and MRI. Then, the signal is subjected to the preprocessing to remove the noise. These eliminated signals are used to develop the DL models. The performance of the model is evaluated using accuracy, sensitivity, and specificity. Additionally, a table combining all the works conducted on epileptic seizure detection using DL is presented in the table form in Appendix A of the paper.

Block diagram of a DL-based CAD system for epileptic seizures.
2.1. Dataset
Datasets play an important role in developing accurate and robust CADS. Multiple EEG datasets, namely, Freiburg [ 34 ], CHB-MIT [ 35 ], Kaggle [ 36 ], Bonn [ 37 ], Flint-Hills [ 26 ], Bern-Barcelona [ 38 ], Hauz Khas [ 26 ], and Zenodo [ 39 ], are available to develop the automated epileptic seizure detection systems. The signals from these datasets are recorded either intracranial or from the scalp of humans or animals.
2.1.1. Fribourg
The EEG dataset contains invasive EEG signals from 21 patients suffering from re-fractory focal epilepsy which were recorded during pre-surgical epilepsy monitoring at the epilepsy center of the University Hospital Fribourg. To provide direct recording from focal area, reduction of artifacts and achieving higher Signal to Noise Ratio (SNR), the in-tra-cortical grid, strip, and depth electrodes were used. The EEG signals were recorded using 128-channel Neurofile NT system with 6 contacts electrodes (three focal and three extra focal) and digitized by a 16bits A/D with sample rate of 256 Hz. For each patient, there are ictal and interictal data, the former contains seizures with at least 50 minutes’ of the pre-ictal region and the latter contains about 24 h of EEG data without seizure [ 34 ].
2.1.2. CHB-MIT
The database comprises 844 h of continuous recording of scalp EEG signals with 163 seizures from 23 children, recorded according to intentional 10–20 standard electrode positions and sampled at 256 samples per second. The inter-ictal region is defined as the period between at least 4 h before the onset seizure and 4 h after the seizure ended. There are two types of seizures, called combined and main seizures, available in this database. The former are multiple seizures close to each other, while the later are great seizures considered for prediction. Generally, the prediction is meaningful for patients having less than 10 seizures per day. In this database, there are sufficient data available (at least three main seizures and 3 h inter-ictal recording) from 13 patients [ 35 ].
2.1.3. Kaggle
The database is the epileptic seizures prediction challenge of the American Epilepsy Society and contains intracranial EEG signals from five dogs and two patients with 48 seizures and 627 h total duration. The EEG signals of dogs were acquired by 16 implantable electrodes, which were sampled at 400 KHz, while the EEG signals from patient 1 and patient 2 were recorded using 15 deep and 24 subdural electrodes, respectively, with sample rate of 5 KHz. In this database, 10 min segments of pre-ictal and inter-ictal data are available, and for each seizure, six pre-ictal segments (with 10 s distance) up to five minutes before seizure onset are accessible. The inter-ictal segments are selected randomly at least one week before each seizure [ 36 ].
2.1.4. Bonn
Bonn database consists of five datasets, A, B, C, D, and E, each containing 100 single-channel EEG signals of 23.6 s duration. The EEG signals were digitized at a sample rate of 173.61 Hz by 12-bit A/D converter. Datasets A and B have the normal signals of five volunteers with eyes opened and closed states, respectively. The EEG signals of datasets C and D are related to pre-ictal region and were recorded from epileptogenic and left area of hippocampus, respectively. The EEG signals of E dataset are related to ictal region. Signals of datasets A and B were recorded using 10–20 scalp EEG standard, while the signals of C and D were intracranial EEG recorded using depth electrodes, and the signals of E were provided using both depth and strip electrodes. Depth electrodes are located symmetrically on hippocampus, while strip electrodes are located on lateral and base sections of neo cortex [ 37 ].
2.1.5. Flint-Hills
The database presents electrocardiography signals with total duration of 1419 h and sample rate of 249 Hz. In addition, meta information about 59 seizures and information related to the position of electrodes are presented. The signals of this database were obtained using 48 to 64 electrodes for each patient [ 26 ].
2.1.6. Bern Barcelona
Barcelona database was collected from the brain department of Bern Hospital of Barcelona and contains intracranial EEG of patients with focal epilepsy. Subjects were monitored for several days, and no antiepileptic drugs were used to determine seizures and possible surgery. The signals were acquired using AD-Tech intracortical electrodes, and one extra reference electrode based on 10–20 standard between PZ and FZ positions was used. The database contained two types of EEG signals: focal and extra focal EEG signals. Every dataset contained 3750 pairs of simultaneous recorded signals with duration of 20 s and sample rate of 512 Hz. The database consists of total 83 h EEG data from five patients with different ages [ 38 ].
2.1.7. Hauz Khas
The database was collected at a brain center in Delhi, India and comprises of scalp EEG signals of 10 patients, recorded with AS40 system and sampled at a rate of 200 Hz in Hauz Khas neurons. The signals were filtered using band-pass filter with pass frequency of 0.5–70 Hz and classified as pre-ictal, inter-ictal, and ictal classes by neurologist experts [ 26 ].
2.1.8. Zenodo
This dataset contains multichannel EEG recordings of 79 human neonates collected in Helsinki University Hospital, with the median recording duration of 74 min. The EEG data were annotated by three experts, and every expert has annotated about 460 seizures, 39 neonates had seizure and 22 neonates were seizure-free in consensus [ 26 ].
The supplementary information on each dataset is listed in Table 1 . Figure 5 shows the number of times each dataset employed epileptic seizures detection using DL techniques. It can be observed that the Bonn dataset is most widely used for automated detection of seizure using DL methods.

Usage of various datasets for automated detection of seizure using DL techniques by various studies.
Review of popular and available EEG datasets for epileptic seizures detection.
2.2. Preprocessing
In developing CADS using DL models with EEG signals, the preprocessing involves three steps: noise removal, normalization, and signal preparation for DL network applications [ 29 , 40 ]. In the noise removal step, finite impulse response (FIR) or infinite impulse response (IIR) filters are usually used to eliminate extra signal noise. Normalization is then performed using various schemes such as the z-score technique. Finally, different time domain, frequency, and time–frequency methods are employed to prepare the signals for the deployment of deep networks.
2.3. Review of Deep Learning Techniques
In contrast to conventional neural networks, or so-called shallow networks, deep neural networks are structures with more than two hidden layers. This increase in the size of the networks results in a massive rise in the number of parameters of the network, requiring appropriate methods for learning, and also measures to avoid overfitting of the learned network. Convolutional networks use filters convolved with input patterns instead of multiplying a weight vector (matrix), which reduces the number of trainable parameters dramatically.
Furthermore, other methods are suggested to help the network to learn, as well [ 41 ]. Pooling layers reduce the size of the input pattern to the next convolutional layer. Batch normalization, dropout, early stopping, unsupervised or semi unsupervised learning, and regularization techniques prevent the learned network from overfitting and increase the learning ability and speed. The AE and DBN are employed as unsupervised learning and then fine-tuned to avoid overfitting for limited labeled data. Long short-term memory (LSTM) and gated recurrent units (GRU) are RNNs capable of revealing the long-term time dependencies of data samples.
2.3.1. Convolutional Neural Networks (CNNs)
CNNs are one class of the most popular DL networks to which most of the researches in machine learning have been devoted [ 30 ]. They were initially presented for image-processing applications, but have recently been adopted to one- and two-dimensional architectures for diagnosis and prediction of diseases using biological signals [ 42 ]. This class of DL networks is widely used for the detection of epileptic seizures using EEG signals. In two-dimensional convolutional neural networks (2D-CNN), the one-dimensional (1D) EEG signals are first transformed into two-dimensional plots by employing visualization methods such as spectrogram [ 43 ], higher-order bispectrum [ 44 , 45 ], and wavelet transforms, and are then applied to the input of the convolutional network. In 1D architectures, the EEG signals are applied in the one-dimensional form to the input of convolutional networks. In these networks, changes are made to the core architecture of 2D-CNN that makes it capable of processing the 1D-EEG signals. Therefore, since both 2D and one-dimensional convolutional neural networks (1D-CNNs) are used in the field of epileptic seizures detection, they are investigated separately.
A. 2D Convolutional Neural Networks (2D-CNNs)
Nowadays, deep 2D networks are used for various medical applications such as diagnosis of COVID-19 in CT and X-ray [ 46 , 47 ], and autism spectrum disorders from MRI modalities [ 48 ]. First, in 2012, Krizovsky et al. [ 49 ] suggested this network to solve image classification problems, and then quickly used similar networks for different tasks such as medical image classification, in an effort to obviate the difficulties of previous networks and solve more intricate problems with better performance. Figure 6 shows a general form of a 2D-CNN used for epileptic seizure detection. The application of 2D-CNN architectures is arguably the most important architecture in the deep neural nets. More information about visualization and preprocessing method can be found in Appendix A .

A typical 2D-CNN for epileptic seizure detection.
In one study [ 50 ], the SeizNet 16-layer convolutional network is introduced, with additional dropout layers and batch normalization (BN) behind each convolutional layer having a structure similar to that of VGG-Net. The authors in [ 51 ] presented a new 2D-CNN model that can extract the spectral and temporal characteristics of EEG signals and used them to learn the general structure of seizures. Zuo et al. [ 52 ] developed the diagnosis of higher-frequency oscillations (HFO) epilepsy from 16-layer 2D-CNN and EEG signals. A DL framework called SeizureNet that uses convolution layers with dense connections is proposed in [ 53 ]. A novel DL model called the temporal graph convolutional network (TGCN) has been introduced by Covert et al. [ 54 ], comprising of five architectures with 14, 18, 22, 23, and 26 layers. Bouaziz et al. [ 55 ] split the EEG signals of CHB-MIT with 23 channels into 2 s time windows and then converted them into density images (spatial representation), which were fed as inputs to the CNN network.
FeiFei Li, Professor of Stanford University, created a dataset of labeled images of real-world objects and termed her project as ImageNet [ 56 ]. ImageNet organizes a computer vision competition called ILSVRC annually to solve the image classification problems. Alex Krizhevsky revolutionized the image classification world with his algorithm, AlexNet, which won the 2012 ImageNet challenge and started the whole DL era [ 49 ]. AlexNet won the competition by achieving the top-5 test accuracy of 84.6%. Taqi et al. [ 57 ] used the AlexNet network to diagnose focal epileptic seizures. This proposed network used the feature extraction approach and eventually applied the Softmax layer for classification purposes and achieved 100% accuracy. In another study, the AlexNet network was employed [ 58 ]. They transformed the 1D signal to 2D image by passing through the Signal2Image (S2I) module. The several methods used in this are signal as image, spectrogram, one-layer 1D-CNN, and two-layer 1D-CNN.
A research team at Oxford proposed the visual geometry group (VGG) model in 2014 [ 59 ]. They configured various models, and one such model was VGG-16, which was submitted to the ILSVRC 2014 competition. The VCG-16 comprises 16 layers and delivered an excellent performance for image classification problems. Ahmedt-Aristizabal et al. [ 60 ] performed VGG-16 architecture to diagnose epilepsy from facial images. Their proposed approach attempted to extract and classify semiological patterns of facial states automatically. After recording the images, the proposed VGG architecture is trained primarily by well-known datasets, followed by various networks such as 1D-CNN and LSTM in the last few layers. In [ 58 ], the VGG network used one-dimensional and two-dimensional signals. To train the models, Adam’s optimizer and a cross-entropy error function were used. They used the batch size and number of epochs as 20 and 100, respectively. The idea of detecting epileptic seizures on the sEEG signal plots was examined by Emami et al. [ 61 ]. In the preprocessing step, the signals were segmented into different time windows and VGG-16 was used for classification, using small (3 × 3) convolution filters to efficiently detect small EEG signal changes. This architecture was pre-trained by applying an ImageNet dataset to differentiate 1000 classes, and the last two layers had 4096 and 1000 dimensional vectors. They modified these last two layers to have 32 and 2 dimensions, respectively, to detect seizure and non-seizure classes.
D. GoogleNet
GoogLeNet won the 2014 ImageNet competition with 93.3% top-5 test accuracy [ 62 ]. This 22-layer network was called GoogLeNet to honor Yann Lecun, who designed LeNet. Before the introduction of GoogLeNet, it was stated that by going deep, one could achieve better accuracy and results. Nevertheless, the google team proposed an architecture called inception, which achieved better performance by not going deep but by better design. It represented a robust design by using filters of different sizes on the same image. In the field of EEG signal processing to diagnose epileptic seizures, this architecture has recently received the attention of researchers. Taqi et al. [ 57 ] used this network in their preliminary studies to diagnose epileptic seizures. Their model was used to extract features from the Bern-Barcelona dataset and achieved excellent results.
Microsoft’s ResNet won the ImageNet challenge with 96.4% accuracy by applying a 152-layer network that utilized a ResNet module [ 63 ]. In this network, residual blocks capable of training deep architecture were introduced by using skip connections that copied inputs of each layer to the next layer. The idea was to learn something different and new in the next layer. So far, little research has been accomplished on the implementation of ResNet networks to diagnose epilepsy, but this may grow significantly in the coming days. Bizopoulos et al. [ 58 ] introduced two ResNet and DenseNet architectures to diagnose epileptic seizures and attained good results. They showed that S2I-DenseNet based model with an average of 70 epochs was sufficient to gain the best accuracy of 85.3%. A summary of related works done using 2D-CNNs is shown in Table 2 . A sketch of accuracy accuracy (%) obtained by various authors is shown in Figure 7 .

Sketch of accuracy (%) obtained by various authors using 2D-CNN models for seizure detection.
Summary of related works done using 2D-CNNs.
F. 1D—Convolutional Neural Network (1D-CNN)
1D-CNNs are intrinsically suitable for processing of biological signals such as EEG for epileptic seizures detection [ 2 ]. These architectures present a more straightforward structure, and a single pass of them is faster as compared with CNN with 2D architecture, due to fewer parameters. The most important superiority of 1D to 2D architectures is the possibility of employing pooling and convolutional layers with a larger size. In addition to that, signals are 1D in nature, and using preprocessing methods to transform them to 2D may lead to information loss. Figure 8 shows a general form of a 1D-CNN used for epileptic seizure detection.

Typical sketch of the 1D-CNN model that can be used for epileptic seizure detection.
The authors in [ 58 ] conducted experiments using 1D-LeNet, AlexNet, VGGnet, ResNet, and DenseNet architectures, and applied well-known 2D architectures in 1D space in the first study in this section. In [ 80 ], 1D-CNN was used for feature extraction procedure. The researchers in [ 81 ] used 1D-CNN for other work. They used a CHB-MIT dataset, and the signals from each channel were segmented into 4 s intervals; overlapping segments were also accepted to increase the data and accuracy. Combining CNNs with conventional feature extraction methods was explored in [ 82 ]; they used the empirical mode decomposition (EMD) method for feature extraction, and CNN was used to acquire high accuracy in the multiclass classification tasks. In [ 83 ], a framework for the diagnosis of epileptic seizures is presented that combined the capability of interpreting probabilistic graphical models (PGMs) with advances in DL. The authors in [ 84 ] submitted a 1D-CNN architecture-defined CNN-BP (standing for CNN bipolar). In this work, they used the data from patients monitored with combined foramen ovale (FO) electrodes and EEG surface electrodes. A new scheme to classify EEG signals based on temporal convolution neural networks (TCNN) was introduced by Zhang et al. [ 85 ]. Table 3 shows the summary of related works done using 1D-CNNs. Figure 9 shows the sketch of accuracy (%) obtained by various authors using 1D-CNN models for epileptic seizures detection.

Sketch of accuracy (%) versus authors obtained using 1D-CNN models for seizure detection.
Summary of related works done using 1D-CNNs.
2.3.2. Recurrent Neural Networks (RNNs)
Sequential data such as text, signals, and videos show characteristics such as variable and great length, which makes them not suitable for simple DL methods [ 41 ]. However, these data form a significant part of the information in the world, compelling the need for DL-based schemes to process these types of data. RNNs are the solution suggested to overcome the mentioned challenges, and are widely used for physiological signals. Figure 10 shows a general form of RNN used for epileptic seizure detection. In the following section, an overview of popular RNN models are presented in addition to the reviewed papers.

Sample RNN model that can be used for seizure detection.
A. Long Short-Term Memory (LSTM)
The main problem of a simple RNN is short-term memory. RNN may leave out key information as it has a hard time transporting information from earlier time steps to the next steps in long-sequence data. Another drawback of RNN is the vanishing gradient problem [ 30 , 31 , 32 , 33 ]. The problem arises because of the shrinking of gradients as it back-propagates. To solve the short-term memory problem, LSTM gates were created [ 30 ]. The flow of information can be regulated through gates. The gates can preserve the long sequence of necessary data, and throw away the undesired ones. The building block of LSTM is the cell state and its gates.
In this section, Golmohammadi et al. [ 68 ] evaluated two LSTM architectures with three and four layers together with the Softmax classifier in their investigation and obtained satisfactory results. In [ 92 ], three-layer LSTMs are used for feature extraction and classification. The sigmoid active function is used in the last fully connected (FC) layer for classification. According to directed experiments in [ 98 ], they employed two architectures: LSTM and GRU. The LSTM GRU model architecture is composed of a layer of Reshape, four layers of LSTM/GRU with the activator, and one layer of FC with sigmoid activator. In another work, Yao et al. [ 102 ] practiced ten different and independently ameliorated RNN (IndRNN) architectures and achieved the best accuracy using Dense IndRNN with attention (DIndRNN) with 31 layers.
B. Gated Recurrent Unit (GRU)
One variation of LSTM is GRU, which combines the input and forgets gates into one update gate [ 30 , 31 , 32 , 33 ]. It merges the input and forgets gates and also makes some other modifications. The gating signals are decreased to two. One is the reset gate, and another is the updating gate. These two gates decide which information is necessary to pass to the output. In one experiment, Chen et al. [ 92 ] used a three-layer GRU network with sigmoid classifier and yielded 96.67% accuracy. Talathi et al. have used a new CADS based on GRU for epileptic seizure detection [ 103 ]. In the proposed method, during the preprocessing, the input signals are split into time windows and spectrogram are obtained from them. Then, these plots are fed to a four-layer GRU network with a Softmax FC layer in the classification stage; 98% accuracy was achieved. In another study, Roy et al. [ 104 ] employed a five-layer GRU network with Softmax classifier and achieved remarkable results. Table 4 provides the summary of related works done using RNNs. Figure 11 shows the sketch of accuracy (%) obtained by various authors using RNN models for seizure detection.

Sketch of accuracy (%) obtained by authors using RNN models for seizure detection.
Summary of related works done using RNNs.
2.3.3. Autoencoders (AEs)
AE is an unsupervised machine learning model for which the input is the same as output [ 30 , 31 , 32 , 33 ]. Input is compressed to a latent-space representation, and then the output is obtained from the representation. Therefore, in AE, the compression and decompression functions are coupled with the neural network. AE consists of three parts, i.e., encoder, code, and decoder. AE networks are most commonly used for feature extraction or dimensionality reduction in the brain signal processing. Figure 12 shows a general form of an AE used for epileptic seizures detection. As the first research in this section, Rajaguru et al. [ 113 ] separately surveyed the multilayer AE (MAE) and expectation-maximization with principal component analysis (EM-PCA) methods to diminish the representation dimensions and then employed the GA for classification. They obtained an average classification accuracy of 93.78% when MAEs were applied for dimensionality reduction and combined with GA as classifier. In another work, it was proposed to design an automated system based on AEs for the diagnosis of epilepsy using the EEG signals [ 114 ]. First, Harmonic wavelet packet transform (HWPT) was used to decompose the signal into frequency sub-bands, and then fractal features, including box-counting (BC), multiresolution BC (MRBC), and Katz fractal dimension (KFD), were extracted from each of the sub-bands.

Sample AE network that may be used for seizure detection.
A. Other Types of AEs
To create a more robust representation, a number of schemes such as denoising AE (DAE) (which tries to recreate input from a corrupted form of it) [ 41 ], stacked AE (SAE) (stacking a few AEs on top of each other to go deeper) [ 41 ], and sparse AEs (SpAE) (which attempts to harness from sparse representations) [ 41 ] have been applied. These methods might pursue other objectives as well, for example, the DAE can be used to recover the corrupted input.
Works in this section begin with Golmohammadi et al. [ 68 ], who presented various deep networks, one of which is stacked denoising AE (SDAE). Their architecture in this section consists of three layers, and the final results demonstrated good performance of their approach. Qiu et al. [ 115 ] exerted the windowed signal, z-score normalization step of preprocessing EEG signals and imported preprocessed data into the denoising sparse AE (DSpAE) network. In their experiment, they achieved an outstanding performance of 100% accuracy. In [ 116 ], a high-performance automated EEG analysis system based on principles of machine learning and big data is presented, which consists of several parts. At first, the signal features are extracted by linear predictive cepstral coefficients (LPCC) coefficients, then three paths are applied for precise detection. The first pass is sequential decoding using hidden Markov models (HMMs), the second pass is composed of both temporal and spatial context analysis based on DL, and in the third pass, a probabilistic grammar is employed.
In another study, Yan et al. [ 117 ] proposed a feature extraction and classification method based on SpAE and support vector machine (SVM). In this approach, first, the feature extraction of the input EEG signals is performed using SAE, and, finally, the classification is performed by SVM. Another SAE architecture was proposed by Yuan et al. [ 118 ], which is namedWave2Vec. In the preprocessing stage, the signals were first framed, and in the deep network segment, the SAE with Softmax was applied and achieved 93.92% accuracy. Following the experiments of Yuan et al., in [ 119 ], different stacked sparse denoising AE (SSpDAE) architectures have been tested and compared. In this work, feature extraction is accomplished by the SSpDAE network and finally classification by Softmax. They obtained an accuracy of 93.64%. Table 5 provides the summary of related works done using AEs. In addition, Figure 13 shows the comparison of the accuracies obtained by different researchers.

Sketch of accuracy (%) versus authors obtained using AE models for seizure detection.
Summary of related works done using AEs.
2.3.4. Deep Belief Networks (DBNs)
Restricted Boltzmann machines (RBM) is a variant of deep Boltzmann machines (DBM) and an undirected graphical model [ 30 ]. The unrestricted Boltzmann machines may also have connections between the hidden units. Stacking the RBMs forms a DBN; RBM is the building block of DBN. DBNs are unsupervised probabilistic hybrid generative DL models comprising latent and stochastic variables in multiple layers [ 30 , 31 , 32 , 33 ]. Furthermore, a variation of DBN is called convolutional DBN (CDBN), which could successfully scale the high-dimensional model and uses the spatial information of the nearby pixels [ 30 , 31 , 32 , 33 ]. DBNs are probabilistic, generative, unsupervised DL models which contain visible and multiple layers of hidden units [ 30 , 31 , 32 , 33 ]. Xuyen et al. [ 129 ] used DBN to identify epileptic spikes in EEG data. The proposed architecture in their study consisted of three hidden layers and achieved an accuracy of 96.87%. In another study, Turner et al. [ 130 ] applied the DBN network to diagnose epilepsy and found promising results.
2.3.5. Convolutional Recurrent Neural Networks (CNN-RNNs)
The highly efficient combination of DL networks used to predict and detect epileptic seizures from EEG signals is the CNN-RNN architecture. Adding convolutional layers to RNN helps to find spatially nearby patterns effectively as RNN characteristic is more suitable for time-series data. In [ 68 ], they applied numerous preprocessing schemes; then, a modified CNN-LSTM architecture was proposed comprising 13 layers and the sigmoid was used for the last layer. Finally, the proposed approach demonstrated better performance.
Roy et al. [ 69 ] used different CNN-RNN hybrid architectures to improve the experimental results. Their first network comprised a one-dimensional seven-layer CNN-GRU convolution architecture, and the second one is a three-dimensional (3D) CNN-GRU network. In another work, Roy et al. [ 104 ] concentrated on natural and abnormal brain activities and suggested four different DL architectures. The proposed ChronoNet model was developed using previous models. It achieved 90.60% and 86.57% training and test accuracies, respectively.
Fang et al. [ 131 ] used the Inception-V3 network. At the outset, a preliminary training was used on this network. Then, to fine-tune this architecture, an RNN- based network called spatial temporal GRU (ST-GRU) was applied, and achieved 77.30% accuracy. Choi et al. [ 132 ] proposed a multiscale 3D-CNN with RNN model for the detection of epileptic seizures. The CNN module output is applied as the input of the RNN module. The RNN module consists of a unilateral GRU layer that extracts the temporal feature of epileptic seizures, which are finally classified using an FC layer. At the end of this section, generalized information from the CNN-RNN research is presented in Table 6 and Figure 14 , respectively.

Sketch of accuracy (%) versus different researchers obtained using CNN-RNN models for seizure detection.
Summary of related works done using CNN-RNNs.
2.3.6. Convolutional Autoencoders (CNN-AEs)
In addition to finding nearby patterns, convolutional layers can reduce the number of parameters in structures such as AEs. These two reasons make their combination suitable for many tasks such as unsupervised feature extraction for epileptic seizure detection. A novel approach based on CNN-AE was presented by Yuan et al. [ 136 ]. At the feature extraction stage, two deep approaches, AE and 2D-CNN, were used to extract the supervised and unsupervised features, respectively. The unsupervised features were obtained directly from the input signals, and the supervised features were acquired from the spectrogram of the signals. Finally, the Softmax classifier was utilized for classification and achieved 94.37% accuracy. In another investigation, Yuan et al. [ 137 ] proposed an approach called deep fusional attention network (DFAN), which can extract channel-aware representations from multichannel EEG signals. They developed a fusional attention layer that utilized a fusional gate to fully integrate multiview information to quantify the contribution of each biomedical channel dynamically. A multiview convolution encoding layer, in combination with CNN, has also been used to train the integrated DL model. Table 7 provides the summary of related works done using CNN-AEs, and Figure 15 shows the accuracies (%) obtained by different researchers.

Sketch of accuracy (%) versus authors obtained using CNN-AE models for seizure detection.
Summary of related works done using CNN-AEs.
3. Non-EEG-Based Epileptic Seizures Detection
3.1. medical imaging.
Various DL models were developed to detect epileptic seizure using sMRI, fMRI, and PET scans with or without EEG signals [ 141 , 142 , 143 , 144 , 145 , 146 , 147 , 148 ]. These models outperformed the conventional models in terms of automatic detection and monitoring of the disease. However, due to the nature and difficulties in using imaging methods, these models are mostly practiced for localization and detection of seizure.
The authors of [ 141 ] proposed automatic localization and detection of focal cortical dysplasia (FCD) from the MRI modality using a CNN model. The diagnosis of FCD rate is only 50% despite the progress in the analytics of MRI modalities. Gill et al. [ 142 ] proposed a CNN-based algorithm with feature learning capability to detect FCD automatically. The authors [ 143 ] designed DeepIED based on DL and EEG-fMRI scans for epilepsy patients, combining the general linear model with EEG-fMRI techniques to estimate the epileptogenic zone. Hosseini et al. [ 144 ] proposed an edge computing autonomic framework for evaluation, regulation, and monitoring of epileptic brain. The epileptogenic network estimated the epilepsy using rs-fMRI and EEG. Shiri et al. [ 148 ] presented a technique for direct attenuation correction of PET images by applying emission data via CNN-AE. Nineteen radiomic features from 83 brain regions were evaluated for image quantification via Hammersmith atlas. Finally, the summary of related works done using medical imaging methods and DL is shown in Table 8 .
Summary of related works done using MRI modalities and DL.
3.2. Other Neuroimaging Modalities
Ravi Prakash et al. [ 135 ] introduced an algorithm based on DL for ECoG-based functional mapping (ECoG-FM) for eloquent language cortex identification. However, the success rate of ECoG-FM is low as compared with electro-cortical stimulation mapping (ESM). In another work, Rosas-Romero et al. [ 149 ] have used fNIRS to detect epileptic seizure and obtained better performance than achieved using conventional EEG signals.
4. Rehabilitation Systems for Epileptic Seizures Detection
The high performance and robustness to noise have made the DL techniques suitable for commercial products. Nowadays various commercial products have been developed in the field of DL, one of which is DL applications and hardware for diagnosing epileptic seizures. In the first study investigated, the brain–computer interface (BCI) system was developed using an AE for epileptic seizure detection by Hosseini et al. [ 127 ]. In another study, Singh et al. [ 128 ] indicated a utilitarian product for the diagnosis of epileptic seizures, which comprised the user segment and the cloud segment. The block diagram of the proposed system presented by Singh et al. is shown in Figure 16 .

Block diagram of proposed epileptic seizure detection system using DL methods with EEG signals.
Kiral-Kornek et al. [ 150 ] demonstrated that DL in combination with neuromorphic hardware could help in developing a wearable, real-time, always-on, patient-specific seizure warning system with low power consumption and reliable long-term performance.
5. Discussion
Nowadays, many people worldwide have epileptic seizures and suffer from these neurological disorders. Early detection of epileptic seizures is of substantial importance because it directly affects the patients’ quality of life and can enhance their self-confidence at all stages of life. So far, much research has been accomplished to diagnose epileptic seizures using AI techniques. The objective of these studies is to assist physicians in accurate epileptic seizures diagnosis. AI research involves conventional machine learning [ 151 ] and DL [ 152 , 153 , 154 , 155 , 156 ] scopes. Until recently, many machine learning methods that were adopted to automatically detect seizures could not be seriously used for a variety of real-time diagnostic aid tools for epileptic seizures due to their disadvantages. DL is one of the state-of-the-art fields of epileptic seizure detection that has been employed for epileptic seizure detection since 2016. In recent years, the research growth in epileptic seizure diagnosis using DL is proceeding rapidly due to the simultaneous development of DL toolboxes as well as graphics processing units (GPUs). Applying DL techniques to diagnose epileptic seizures gives doctors hope that in the not-too-distant future a variety of rehabilitation tools will be developed for patients with epileptic seizures. Table A1 in the Appendix shows the overview of works done in this area. It also shows the type of dataset used, implementation tool, preprocessing, DL network, and evaluation methods utilized.
As shown in this study, various DL structures are applied for epileptic seizure detection, yet none of them has superiority over others. The best structure should be chosen carefully based on the dataset and problem characteristics, such as the need for real-time detection or minimum acceptable accuracy or even the use of pre-trained models. There are many databases available with different models. Hence, it is difficult to compare them as they have been developed using different datasets and models. Overall, one of the most important advantages of DL algorithms is their high performance. Hence, such models have been widely used for many applications. Another advantage of DL methods is that they are robust to noise. Therefore, noise removal can be omitted in many applications. However, they need more data to train, and training takes time. Developing a robust model is time consuming and requires huge data.
6. Challenges
There are several challenges in diagnosing epileptic seizures using neuroimaging modalities and DL procedures. Inaccessibility of datasets with high registration time is the first challenge in this area. The datasets available for diagnosing epileptic seizures have a finite registration time (or recording), making it difficult to conduct serious (or important) research in the field of epileptic seizures. The complete datasets are not shared in the public domain, only a portion of the data may be available. Hence, real-time diagnosis of epileptic seizures is still challenging. However, research in the field of real-time epileptic seizures diagnosis has been performed, using clinical data [ 157 , 158 , 159 ].
Due to the lack of accessible datasets, researchers have not yet been able to present a DL-based CADS for diagnosing epileptic seizures with optimum performance. Additionally, it is not possible to combine the available EEG datasets to enhance the efficiency of DL networks. This is because each of the datasets presented possesses different sampling frequencies, and in order to achieve higher detection accuracy, it is not pragmatic to integrate them to feed to DL networks.
Table 1 shows all available EEG datasets used for epileptic seizure detection. However, other neuroimaging modalities such as MRI are used for epileptic seizures detection. In [ 141 , 142 , 143 , 144 , 145 , 146 , 147 , 148 ], MRI modalities coupled with DL methods have been used to diagnose epileptic seizures. Datasets with non-MRI modalities are not available, and this has led to limited research in this area. Therefore, providing datasets from other neuroimaging modalities is important to conduct research.
Nowadays, DL models have made considerable advancements [ 160 , 161 , 162 , 163 , 164 ]. This has resulted in the development of computer hardware [ 165 , 166 ] that is expensive and not easily accessible to the researchers. Researchers working in the field of epileptic seizures detection/prediction do not always have access to high-power hardware to implement novel DL models. Although powerful computing servers are available by Google, constraints such as the amount of data that can be uploaded to these servers and execution time are still the challenges.
7. Conclusion and Future Works
In recent years, a lot of research has been done in the epileptic seizures detection field using artificial intelligence methods [ 167 , 168 , 169 , 170 , 171 , 172 , 173 , 174 , 175 ]. In this paper, a comprehensive review of works done in the field of epileptic seizure detection using various DL techniques such as CNNs, RNNs, and AEs is presented. Various screening methods have been developed using EEG and MRI modalities. We have investigated the epileptic seizures detection using DL-based practical and applied hardware methods. It is very encouraging that much of the future research will concentrate on hardware—practical applications aid in the accurate detection of such diseases. The functional hardware has also been utilized to boost the performance of detection strategies. Furthermore, the models can be placed in the cloud by hospitals. Therefore, handheld applications, mobile or wearable devices, may be equipped with such models, and cloud servers will perform the computations; by taking benefit from predictive models, these devices can be used to avert patients in a timely manner. Alert messages may be generated to the family, relatives, the concerned hospital, and doctor in the detection of epileptic seizures through the handheld devices or wearables, and thus the patient can be provided with proper treatment in time. Moreover, a cap with EEG electrodes in it can obtain the EEG signals, which can be sent to the model kept in the cloud to achieve real-time detection. Additionally, if we can detect early stage of seizure using interictal periods of EEG signals, the patient can take medication immediately and prevent seizure. This field of research requires more research that combines different screening methods for more precise and fast detection of epileptic seizures and also applies semi supervised and unsupervised methods to further overcome the dataset size limits. Finally, having publicly available comprehensive datasets can help to develop an accurate and robust model that can detect the seizure in the early stage.
Table A1 shows the detailed summary of DL methods employed for automated detection of epileptic seizures.
Summary of DL methods employed for automated detection of epileptic seizures.
Author Contributions
Contributed to prepare the first draft: A.S., M.K., N.G., M.J., R.A., P.M., F.K., D.A., H.H.-N., A.Z., M.R., and S.H.; contributed to editing the final draft: S.N., M.P., A.F.A., A.K., A.S., and U.R.A.; contributed to all analysis of the data and produced the results accordingly: A.S., M.J., M.K., R.A., P.M., A.Z., and N.G.; searched for papers and then extracted data: S.H., A.S., M.K., and F.K.; Provided overall guidance and managed the project: S.N., M.P., A.F.A., A.K., A.S., and U.R.A. All authors have read and agreed to the published version of the manuscript.
This research received no external funding.
Informed Consent Statement
Data availability statement, conflicts of interest.
The authors declare no conflict of interest.
Publisher’s Note: MDPI stays neutral with regard to jurisdictional claims in published maps and institutional affiliations.
Help | Advanced Search
Computer Science > Computation and Language
Title: chameleon: mixed-modal early-fusion foundation models.
Abstract: We present Chameleon, a family of early-fusion token-based mixed-modal models capable of understanding and generating images and text in any arbitrary sequence. We outline a stable training approach from inception, an alignment recipe, and an architectural parameterization tailored for the early-fusion, token-based, mixed-modal setting. The models are evaluated on a comprehensive range of tasks, including visual question answering, image captioning, text generation, image generation, and long-form mixed modal generation. Chameleon demonstrates broad and general capabilities, including state-of-the-art performance in image captioning tasks, outperforms Llama-2 in text-only tasks while being competitive with models such as Mixtral 8x7B and Gemini-Pro, and performs non-trivial image generation, all in a single model. It also matches or exceeds the performance of much larger models, including Gemini Pro and GPT-4V, according to human judgments on a new long-form mixed-modal generation evaluation, where either the prompt or outputs contain mixed sequences of both images and text. Chameleon marks a significant step forward in a unified modeling of full multimodal documents.
Submission history
Access paper:.
- HTML (experimental)
- Other Formats

References & Citations
- Google Scholar
- Semantic Scholar
BibTeX formatted citation

Bibliographic and Citation Tools
Code, data and media associated with this article, recommenders and search tools.
- Institution
arXivLabs: experimental projects with community collaborators
arXivLabs is a framework that allows collaborators to develop and share new arXiv features directly on our website.
Both individuals and organizations that work with arXivLabs have embraced and accepted our values of openness, community, excellence, and user data privacy. arXiv is committed to these values and only works with partners that adhere to them.
Have an idea for a project that will add value for arXiv's community? Learn more about arXivLabs .
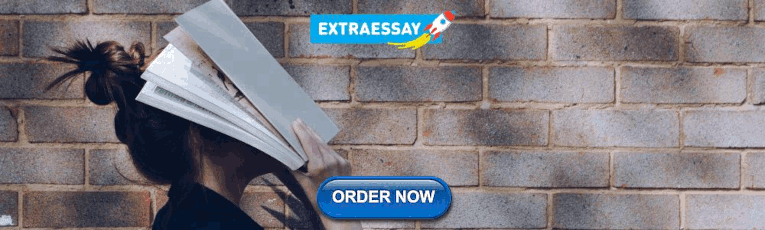
IMAGES
VIDEO
COMMENTS
Imprecise definitions, failure to use accepted terminology, or inappropriate use of terminology hamper our ability to study and advance the field of epilepsy. This article begins by discussing the pathophysiology and epidemiology of epilepsy, and then covers the accepted contemporary definitions and classifications of seizures and epilepsies.
Epilepsy syndromes are types of epi-"simple partial seizure" or "aura.". In the 2017 classification lepsy in which a clear demographic, seizure type(s), system, this type of seizure is more precisely termed "a electroencephalography (EEG) pattern, and prognosis focal aware seizure.". During a focal aware seizure, the are known.
Abstract. Epilepsy is a global health problem affecting approximately 50 million people worldwide. It is one of the most common chronic neurological diseases in the world and has serious physical ...
repercussions. Recently, ILAE stated a n ew. definition of epilepsy for clinical use which can be. considered if any of the following situations. experienced by patients: (a) Minimum occurring of ...
its burden, and to advocate for actions to address gaps in epilepsy knowledge, care and research. Raising epilepsy on the global public health agenda cannot be done alone. The adoption of the World Health Assembly resolution on epilepsy by Member States drew attention to the need for coordinated action at country level.
This paper reviews advances in epilepsy in recent years with an emphasis on therapeutics and underlying mechanisms, including status epilepticus, drug and surgical treatments. Lessons from rarer ...
145: 2436-49. Epilepsy research in 2022: clinical advances. In 2022, epilepsy research has made advances across. a range of clinically important areas, from self- management, genetics, imaging, and surgical planning to understanding febrile seizures and coma-related periodic patterns.
Epilepsy is one of the most common serious brain conditions, afecting over 70 million people worldwide. Its incidence has a bimodal distribution with the highest risk in infants and older age groups. Progress in genomic technology is exposing the complex genetic architecture of the common types of epilepsy, and is driving a paradigm shift.
Epilepsy Research provides for publication of high quality articles in both basic and clinical epilepsy research, with a special emphasis on translational research that ultimately relates to epilepsy as a human condition. The journal is intended to provide a forum for reporting the best and most rigorous epilepsy research from all disciplines ...
The diagnosis and treatment of seizures and epilepsy is a common task of the physician. Approximately 1 in 10 people will have a seizure during their lifetime. Epilepsy is the tendency to have unprovoked seizures. Epilepsy is the fourth most common neurological disorder and affects 1 in 26 people in the United States and 65 million people worldwide. Evaluation of a patient presenting with a ...
For assistance with manuscript submission, please contact: [email protected]. Free archive: Anyone may access any past or current articles without logging in. Editorial Office KCC Parktown 101-308, Mallijae-ro 185, Jung-Gu, Seoul, Korea TEL : +82 ...
We defined an epilepsy syndrome as "a characteristic cluster of clinical and EEG features, often supported by specific etiological findings (structural, genetic, metabolic, immune, and infectious).". The diagnosis of a syndrome in an individual with epilepsy frequently carries prognostic and treatment implications.
Abstract. This paper reviews advances in epilepsy in recent years with an emphasis on therapeutics and underlying mechanisms, including status epilepticus, drug and surgical treatments. Lessons from rarer epilepsies regarding the relationship between epilepsy type, mechanisms and choice of antiepileptic drugs (AED) are explored and data ...
Epilepsy syndromes, previously called "symptomatic localization-related," are those in which seizures arise in a focal brain region caused by an acquired or congenital lesion. Etiologies include tumor, scar (e.g., hippocampal sclerosis), cortical dysplasia, porencephalic cyst, and vascular malformation.
Epilepsy is one of the most common neurological disorders (~8 000 000 patients in the European Union). It has devastating behavioral, social, and occupational consequences, and is associated with accumulating brain damage and neurological deficits. Epilepsy comprises a large number of syndromes, which vary greatly with respect to their clinical ...
Research article Full text access Utilisation and polypharmacy aspects of antiepileptic drugs in elderly versus younger patients with epilepsy: A pharmacoepidemiological study of CNS-active drugs in Norway, 2004-2015
The pharmacological armamentarium against epilepsy has expanded considerably over the last three decades, and currently includes over 30 different antiseizure medications. Despite this large armamentarium, about one third of people with epilepsy fail to achieve sustained seizure freedom with currently available medications. This sobering fact, however, is mitigated by evidence that clinical ...
This paper reviews the epilepsy mentality disorder and the types of seizure, preprocessing operations that are performed on EEG data, a generally extracted feature from the signal, and a detailed view on classification procedures used in this problem and provide insights on the difficulties and future research directions in this innovative theme.
Spasm production injury certainly consciousness, heterogeneity. Epilepsy is a neurology disease a further overcome a large number of childr en and adolescents, with 80 percent of those affected ...
Epilepsy is a chronic neurological disease, characterized by spontaneous, unprovoked, recurrent seizures that may lead to long-term disability and premature death. Despite significant efforts made ...
Epilepsia. 2018; 59:1557-1566. Lay summary. Ketogenic dietary therapies (KDTs) are a group of high‐fat, low‐carbohydrate diets that have been used effectively as treatment options for people with drug resistant epilepsy since the early 1900s. We know that certain epilepsies, such as epilepsy with myoclonic‐atonic seizures, tuberous ...
Epilepsy is a persistent health condition marked by unusual and highly synchronized electrical activity in the brain cells, resulting in recurring seizures. This paper proposes a novel real-time method to improve the detection of seizures using the spectral features of non-stationary electroencephalogram (EEG) signals. It is observed that the discrete wavelet transform (DWT)-based features do ...
Genetic variants in the DEP domain containing 5 (DEPDC5) are one of the most common causes of inheritable drug-resistant temporal focal epilepsy. Epilepsies caused by DEPDC5 variants are often associated with brain malformations and sudden unexplained death in epilepsy (SUDEP). It has been reported that the DEPDC5 genes regulates cell growth via rapamycin complex 1 (mTORC1) pathways. Though ...
To assess whether post‐stroke epilepsy (PSE) is associated with neuroimaging findings of hemosiderin in a case-control study, and whether the addition of heMosiderin markers improves the risk stratification models of PSE, a double-blind, placebo-controlled study is conducted.
In recent years, a lot of research has been done in the epileptic seizures detection field using artificial intelligence methods [167,168,169,170,171,172,173,174,175]. In this paper, a comprehensive review of works done in the field of epileptic seizure detection using various DL techniques such as CNNs, RNNs, and AEs is presented.
Epilepsy is considered a spectrum disorder with highly diverse etiology, comprising structural, genetic, metabolic, autoimmune and infection-related causes. Structural causes (5, 7) refers to ...
View PDF HTML (experimental) Abstract: We present Chameleon, a family of early-fusion token-based mixed-modal models capable of understanding and generating images and text in any arbitrary sequence. We outline a stable training approach from inception, an alignment recipe, and an architectural parameterization tailored for the early-fusion, token-based, mixed-modal setting.
1)The paper identifies an impactful vulnerability in Ap-ple's Wi-Fi positioning system and demonstrates ways of exploiting it to track users, including in the context of military and natural disaster situations. 2)The paper collects a longitudinal dataset that could benefit future research. 3)The paper provides recommendations for remediation.
1. stroke as one of the most common serious neurologic disorders. About 50 million people world wide have epilepsy and 90% of them. 2. are from developing countries. In the past several years, our ...
Besides the Word documents and pdf files, the threat actor also collected files related to messaging apps such as Telegram and Viber. ... With deep investments in research and development, Bitdefender Labs discovers over 400 new threats each minute and validates around 40 billion daily threat queries. The company has pioneered breakthrough ...