Subscribe to the PwC Newsletter
Join the community, trending research, storydiffusion: consistent self-attention for long-range image and video generation.

This module converts the generated sequence of images into videos with smooth transitions and consistent subjects that are significantly more stable than the modules based on latent spaces only, especially in the context of long video generation.
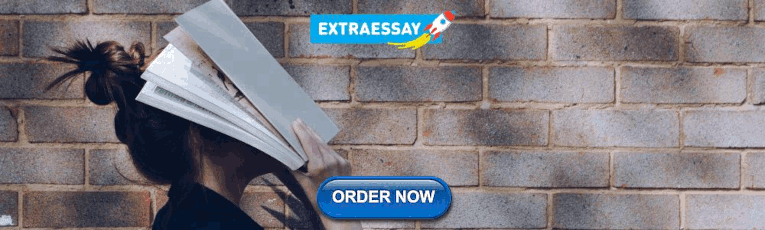
DeepSeek-V2: A Strong, Economical, and Efficient Mixture-of-Experts Language Model
MLA guarantees efficient inference through significantly compressing the Key-Value (KV) cache into a latent vector, while DeepSeekMoE enables training strong models at an economical cost through sparse computation.

Granite Code Models: A Family of Open Foundation Models for Code Intelligence
ibm-granite/granite-code-models • 7 May 2024
Increasingly, code LLMs are being integrated into software development environments to improve the productivity of human programmers, and LLM-based agents are beginning to show promise for handling complex tasks autonomously.

KAN: Kolmogorov-Arnold Networks
Inspired by the Kolmogorov-Arnold representation theorem, we propose Kolmogorov-Arnold Networks (KANs) as promising alternatives to Multi-Layer Perceptrons (MLPs).
QServe: W4A8KV4 Quantization and System Co-design for Efficient LLM Serving
The key insight driving QServe is that the efficiency of LLM serving on GPUs is critically influenced by operations on low-throughput CUDA cores.
Improving Diffusion Models for Virtual Try-on
Finally, we present a customization method using a pair of person-garment images, which significantly improves fidelity and authenticity.

Prometheus 2: An Open Source Language Model Specialized in Evaluating Other Language Models
prometheus-eval/prometheus-eval • 2 May 2024
Proprietary LMs such as GPT-4 are often employed to assess the quality of responses from various LMs.
ImageInWords: Unlocking Hyper-Detailed Image Descriptions
google/imageinwords • 5 May 2024
To address these issues, we introduce ImageInWords (IIW), a carefully designed human-in-the-loop annotation framework for curating hyper-detailed image descriptions and a new dataset resulting from this process.

Plan-and-Solve Prompting: Improving Zero-Shot Chain-of-Thought Reasoning by Large Language Models
assafelovic/gpt-researcher • 6 May 2023
To address the calculation errors and improve the quality of generated reasoning steps, we extend PS prompting with more detailed instructions and derive PS+ prompting.
Inf-DiT: Upsampling Any-Resolution Image with Memory-Efficient Diffusion Transformer
thudm/inf-dit • 7 May 2024
However, due to a quadratic increase in memory during generating ultra-high-resolution images (e. g. 4096*4096), the resolution of generated images is often limited to 1024*1024.

A free, AI-powered research tool for scientific literature
- Stephen D. Evans
- Economic Growth
New & Improved API for Developers
Introducing semantic reader in beta.
Stay Connected With Semantic Scholar Sign Up What Is Semantic Scholar? Semantic Scholar is a free, AI-powered research tool for scientific literature, based at the Allen Institute for AI.
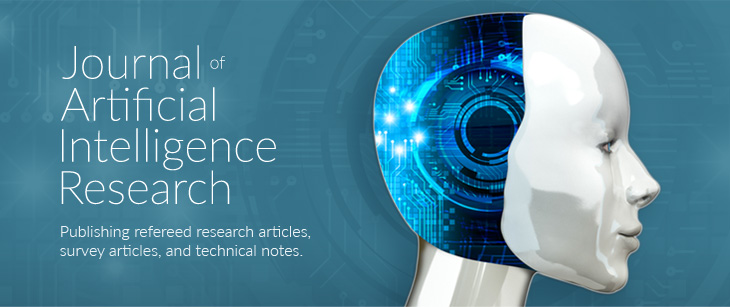
The Journal of Artificial Intelligence Research (JAIR) is dedicated to the rapid dissemination of important research results to the global artificial intelligence (AI) community. The journal’s scope encompasses all areas of AI, including agents and multi-agent systems, automated reasoning, constraint processing and search, knowledge representation, machine learning, natural language, planning and scheduling, robotics and vision, and uncertainty in AI.
Current Issue
Vol. 79 (2024)
Published: 2024-01-10
Bt-GAN: Generating Fair Synthetic Healthdata via Bias-transforming Generative Adversarial Networks
Collision avoiding max-sum for mobile sensor teams, usn: a robust imitation learning method against diverse action noise, structure in deep reinforcement learning: a survey and open problems, a map of diverse synthetic stable matching instances, digcn: a dynamic interaction graph convolutional network based on learnable proposals for object detection, iterative train scheduling under disruption with maximum satisfiability, removing bias and incentivizing precision in peer-grading, cultural bias in explainable ai research: a systematic analysis, learning to resolve social dilemmas: a survey, a principled distributional approach to trajectory similarity measurement and its application to anomaly detection, multi-modal attentive prompt learning for few-shot emotion recognition in conversations, condense: conditional density estimation for time series anomaly detection, performative ethics from within the ivory tower: how cs practitioners uphold systems of oppression, learning logic specifications for policy guidance in pomdps: an inductive logic programming approach, multi-objective reinforcement learning based on decomposition: a taxonomy and framework, can fairness be automated guidelines and opportunities for fairness-aware automl, practical and parallelizable algorithms for non-monotone submodular maximization with size constraint, exploring the tradeoff between system profit and income equality among ride-hailing drivers, on mitigating the utility-loss in differentially private learning: a new perspective by a geometrically inspired kernel approach, an algorithm with improved complexity for pebble motion/multi-agent path finding on trees, weighted, circular and semi-algebraic proofs, reinforcement learning for generative ai: state of the art, opportunities and open research challenges, human-in-the-loop reinforcement learning: a survey and position on requirements, challenges, and opportunities, boolean observation games, detecting change intervals with isolation distributional kernel, query-driven qualitative constraint acquisition, visually grounded language learning: a review of language games, datasets, tasks, and models, right place, right time: proactive multi-robot task allocation under spatiotemporal uncertainty, principles and their computational consequences for argumentation frameworks with collective attacks, the ai race: why current neural network-based architectures are a poor basis for artificial general intelligence, undesirable biases in nlp: addressing challenges of measurement.
Generative AI: A Review on Models and Applications
Ieee account.
- Change Username/Password
- Update Address
Purchase Details
- Payment Options
- Order History
- View Purchased Documents
Profile Information
- Communications Preferences
- Profession and Education
- Technical Interests
- US & Canada: +1 800 678 4333
- Worldwide: +1 732 981 0060
- Contact & Support
- About IEEE Xplore
- Accessibility
- Terms of Use
- Nondiscrimination Policy
- Privacy & Opting Out of Cookies
A not-for-profit organization, IEEE is the world's largest technical professional organization dedicated to advancing technology for the benefit of humanity. © Copyright 2024 IEEE - All rights reserved. Use of this web site signifies your agreement to the terms and conditions.
Tackling the most challenging problems in computer science
Our teams aspire to make discoveries that positively impact society. Core to our approach is sharing our research and tools to fuel progress in the field, to help more people more quickly. We regularly publish in academic journals, release projects as open source, and apply research to Google products to benefit users at scale.
Featured research developments
Mitigating aviation’s climate impact with Project Contrails
Consensus and subjectivity of skin tone annotation for ML fairness
A toolkit for transparency in AI dataset documentation
Building better pangenomes to improve the equity of genomics
A set of methods, best practices, and examples for designing with AI
Learn more from our research
Researchers across Google are innovating across many domains. We challenge conventions and reimagine technology so that everyone can benefit.
Publications
Google publishes over 1,000 papers annually. Publishing our work enables us to collaborate and share ideas with, as well as learn from, the broader scientific community.
Research areas
From conducting fundamental research to influencing product development, our research teams have the opportunity to impact technology used by billions of people every day.
Tools and datasets
We make tools and datasets available to the broader research community with the goal of building a more collaborative ecosystem.
Meet the people behind our innovations
Our teams collaborate with the research and academic communities across the world
Partnerships to improve our AI products

The best AI tools for research papers and academic research (Literature review, grants, PDFs and more)
As our collective understanding and application of artificial intelligence (AI) continues to evolve, so too does the realm of academic research. Some people are scared by it while others are openly embracing the change.
Make no mistake, AI is here to stay!
Instead of tirelessly scrolling through hundreds of PDFs, a powerful AI tool comes to your rescue, summarizing key information in your research papers. Instead of manually combing through citations and conducting literature reviews, an AI research assistant proficiently handles these tasks.
These aren’t futuristic dreams, but today’s reality. Welcome to the transformative world of AI-powered research tools!
This blog post will dive deeper into these tools, providing a detailed review of how AI is revolutionizing academic research. We’ll look at the tools that can make your literature review process less tedious, your search for relevant papers more precise, and your overall research process more efficient and fruitful.
I know that I wish these were around during my time in academia. It can be quite confronting when trying to work out what ones you should and shouldn’t use. A new one seems to be coming out every day!
Here is everything you need to know about AI for academic research and the ones I have personally trialed on my YouTube channel.
My Top AI Tools for Researchers and Academics – Tested and Reviewed!
There are many different tools now available on the market but there are only a handful that are specifically designed with researchers and academics as their primary user.
These are my recommendations that’ll cover almost everything that you’ll want to do:
Want to find out all of the tools that you could use?
Here they are, below:
AI literature search and mapping – best AI tools for a literature review – elicit and more
Harnessing AI tools for literature reviews and mapping brings a new level of efficiency and precision to academic research. No longer do you have to spend hours looking in obscure research databases to find what you need!
AI-powered tools like Semantic Scholar and elicit.org use sophisticated search engines to quickly identify relevant papers.
They can mine key information from countless PDFs, drastically reducing research time. You can even search with semantic questions, rather than having to deal with key words etc.
With AI as your research assistant, you can navigate the vast sea of scientific research with ease, uncovering citations and focusing on academic writing. It’s a revolutionary way to take on literature reviews.
- Elicit – https://elicit.org
- Litmaps – https://www.litmaps.com
- Research rabbit – https://www.researchrabbit.ai/
- Connected Papers – https://www.connectedpapers.com/
- Supersymmetry.ai: https://www.supersymmetry.ai
- Semantic Scholar: https://www.semanticscholar.org
- Laser AI – https://laser.ai/
- Inciteful – https://inciteful.xyz/
- Scite – https://scite.ai/
- System – https://www.system.com
If you like AI tools you may want to check out this article:
- How to get ChatGPT to write an essay [The prompts you need]
AI-powered research tools and AI for academic research
AI research tools, like Concensus, offer immense benefits in scientific research. Here are the general AI-powered tools for academic research.
These AI-powered tools can efficiently summarize PDFs, extract key information, and perform AI-powered searches, and much more. Some are even working towards adding your own data base of files to ask questions from.
Tools like scite even analyze citations in depth, while AI models like ChatGPT elicit new perspectives.
The result? The research process, previously a grueling endeavor, becomes significantly streamlined, offering you time for deeper exploration and understanding. Say goodbye to traditional struggles, and hello to your new AI research assistant!
- Consensus – https://consensus.app/
- Iris AI – https://iris.ai/
- Research Buddy – https://researchbuddy.app/
- Mirror Think – https://mirrorthink.ai
AI for reading peer-reviewed papers easily
Using AI tools like Explain paper and Humata can significantly enhance your engagement with peer-reviewed papers. I always used to skip over the details of the papers because I had reached saturation point with the information coming in.
These AI-powered research tools provide succinct summaries, saving you from sifting through extensive PDFs – no more boring nights trying to figure out which papers are the most important ones for you to read!
They not only facilitate efficient literature reviews by presenting key information, but also find overlooked insights.
With AI, deciphering complex citations and accelerating research has never been easier.
- Aetherbrain – https://aetherbrain.ai
- Explain Paper – https://www.explainpaper.com
- Chat PDF – https://www.chatpdf.com
- Humata – https://www.humata.ai/
- Lateral AI – https://www.lateral.io/
- Paper Brain – https://www.paperbrain.study/
- Scholarcy – https://www.scholarcy.com/
- SciSpace Copilot – https://typeset.io/
- Unriddle – https://www.unriddle.ai/
- Sharly.ai – https://www.sharly.ai/
- Open Read – https://www.openread.academy
AI for scientific writing and research papers
In the ever-evolving realm of academic research, AI tools are increasingly taking center stage.
Enter Paper Wizard, Jenny.AI, and Wisio – these groundbreaking platforms are set to revolutionize the way we approach scientific writing.
Together, these AI tools are pioneering a new era of efficient, streamlined scientific writing.
- Jenny.AI – https://jenni.ai/ (20% off with code ANDY20)
- Yomu – https://www.yomu.ai
- Wisio – https://www.wisio.app
AI academic editing tools
In the realm of scientific writing and editing, artificial intelligence (AI) tools are making a world of difference, offering precision and efficiency like never before. Consider tools such as Paper Pal, Writefull, and Trinka.
Together, these tools usher in a new era of scientific writing, where AI is your dedicated partner in the quest for impeccable composition.
- PaperPal – https://paperpal.com/
- Writefull – https://www.writefull.com/
- Trinka – https://www.trinka.ai/
AI tools for grant writing
In the challenging realm of science grant writing, two innovative AI tools are making waves: Granted AI and Grantable.
These platforms are game-changers, leveraging the power of artificial intelligence to streamline and enhance the grant application process.
Granted AI, an intelligent tool, uses AI algorithms to simplify the process of finding, applying, and managing grants. Meanwhile, Grantable offers a platform that automates and organizes grant application processes, making it easier than ever to secure funding.
Together, these tools are transforming the way we approach grant writing, using the power of AI to turn a complex, often arduous task into a more manageable, efficient, and successful endeavor.
- Granted AI – https://grantedai.com/
- Grantable – https://grantable.co/
Best free AI research tools
There are many different tools online that are emerging for researchers to be able to streamline their research processes. There’s no need for convience to come at a massive cost and break the bank.
The best free ones at time of writing are:
- Elicit – https://elicit.org
- Connected Papers – https://www.connectedpapers.com/
- Litmaps – https://www.litmaps.com ( 10% off Pro subscription using the code “STAPLETON” )
- Consensus – https://consensus.app/
Wrapping up
The integration of artificial intelligence in the world of academic research is nothing short of revolutionary.
With the array of AI tools we’ve explored today – from research and mapping, literature review, peer-reviewed papers reading, scientific writing, to academic editing and grant writing – the landscape of research is significantly transformed.
The advantages that AI-powered research tools bring to the table – efficiency, precision, time saving, and a more streamlined process – cannot be overstated.
These AI research tools aren’t just about convenience; they are transforming the way we conduct and comprehend research.
They liberate researchers from the clutches of tedium and overwhelm, allowing for more space for deep exploration, innovative thinking, and in-depth comprehension.
Whether you’re an experienced academic researcher or a student just starting out, these tools provide indispensable aid in your research journey.
And with a suite of free AI tools also available, there is no reason to not explore and embrace this AI revolution in academic research.
We are on the precipice of a new era of academic research, one where AI and human ingenuity work in tandem for richer, more profound scientific exploration. The future of research is here, and it is smart, efficient, and AI-powered.
Before we get too excited however, let us remember that AI tools are meant to be our assistants, not our masters. As we engage with these advanced technologies, let’s not lose sight of the human intellect, intuition, and imagination that form the heart of all meaningful research. Happy researching!
Thank you to Ivan Aguilar – Ph.D. Student at SFU (Simon Fraser University), for starting this list for me!

Dr Andrew Stapleton has a Masters and PhD in Chemistry from the UK and Australia. He has many years of research experience and has worked as a Postdoctoral Fellow and Associate at a number of Universities. Although having secured funding for his own research, he left academia to help others with his YouTube channel all about the inner workings of academia and how to make it work for you.
Thank you for visiting Academia Insider.
We are here to help you navigate Academia as painlessly as possible. We are supported by our readers and by visiting you are helping us earn a small amount through ads and affiliate revenue - Thank you!

2024 © Academia Insider

- Skip to main content
- Skip to primary sidebar
- Skip to footer

The Best of Applied Artificial Intelligence, Machine Learning, Automation, Bots, Chatbots
2020’s Top AI & Machine Learning Research Papers
November 24, 2020 by Mariya Yao

Despite the challenges of 2020, the AI research community produced a number of meaningful technical breakthroughs. GPT-3 by OpenAI may be the most famous, but there are definitely many other research papers worth your attention.
For example, teams from Google introduced a revolutionary chatbot, Meena, and EfficientDet object detectors in image recognition. Researchers from Yale introduced a novel AdaBelief optimizer that combines many benefits of existing optimization methods. OpenAI researchers demonstrated how deep reinforcement learning techniques can achieve superhuman performance in Dota 2.
To help you catch up on essential reading, we’ve summarized 10 important machine learning research papers from 2020. These papers will give you a broad overview of AI research advancements this year. Of course, there are many more breakthrough papers worth reading as well.
We have also published the top 10 lists of key research papers in natural language processing and computer vision . In addition, you can read our premium research summaries , where we feature the top 25 conversational AI research papers introduced recently.
Subscribe to our AI Research mailing list at the bottom of this article to be alerted when we release new summaries.
If you’d like to skip around, here are the papers we featured:
- A Distributed Multi-Sensor Machine Learning Approach to Earthquake Early Warning
- Efficiently Sampling Functions from Gaussian Process Posteriors
- Dota 2 with Large Scale Deep Reinforcement Learning
- Towards a Human-like Open-Domain Chatbot
- Language Models are Few-Shot Learners
- Beyond Accuracy: Behavioral Testing of NLP models with CheckList
- EfficientDet: Scalable and Efficient Object Detection
- Unsupervised Learning of Probably Symmetric Deformable 3D Objects from Images in the Wild
- An Image is Worth 16×16 Words: Transformers for Image Recognition at Scale
- AdaBelief Optimizer: Adapting Stepsizes by the Belief in Observed Gradients
Best AI & ML Research Papers 2020
1. a distributed multi-sensor machine learning approach to earthquake early warning , by kévin fauvel, daniel balouek-thomert, diego melgar, pedro silva, anthony simonet, gabriel antoniu, alexandru costan, véronique masson, manish parashar, ivan rodero, and alexandre termier, original abstract .
Our research aims to improve the accuracy of Earthquake Early Warning (EEW) systems by means of machine learning. EEW systems are designed to detect and characterize medium and large earthquakes before their damaging effects reach a certain location. Traditional EEW methods based on seismometers fail to accurately identify large earthquakes due to their sensitivity to the ground motion velocity. The recently introduced high-precision GPS stations, on the other hand, are ineffective to identify medium earthquakes due to their propensity to produce noisy data. In addition, GPS stations and seismometers may be deployed in large numbers across different locations and may produce a significant volume of data, consequently affecting the response time and the robustness of EEW systems.
In practice, EEW can be seen as a typical classification problem in the machine learning field: multi-sensor data are given in input, and earthquake severity is the classification result. In this paper, we introduce the Distributed Multi-Sensor Earthquake Early Warning (DMSEEW) system, a novel machine learning-based approach that combines data from both types of sensors (GPS stations and seismometers) to detect medium and large earthquakes. DMSEEW is based on a new stacking ensemble method which has been evaluated on a real-world dataset validated with geoscientists. The system builds on a geographically distributed infrastructure, ensuring an efficient computation in terms of response time and robustness to partial infrastructure failures. Our experiments show that DMSEEW is more accurate than the traditional seismometer-only approach and the combined-sensors (GPS and seismometers) approach that adopts the rule of relative strength.
Our Summary
The authors claim that traditional Earthquake Early Warning (EEW) systems that are based on seismometers, as well as recently introduced GPS systems, have their disadvantages with regards to predicting large and medium earthquakes respectively. Thus, the researchers suggest approaching an early earthquake prediction problem with machine learning by using the data from seismometers and GPS stations as input data. In particular, they introduce the Distributed Multi-Sensor Earthquake Early Warning (DMSEEW) system, which is specifically tailored for efficient computation on large-scale distributed cyberinfrastructures. The evaluation demonstrates that the DMSEEW system is more accurate than other baseline approaches with regard to real-time earthquake detection.

What’s the core idea of this paper?
- Seismometers have difficulty detecting large earthquakes because of their sensitivity to ground motion velocity.
- GPS stations are ineffective in detecting medium earthquakes, as they are prone to producing lots of noisy data.
- takes sensor-level class predictions from seismometers and GPS stations (i.e. normal activity, medium earthquake, large earthquake);
- aggregates these predictions using a bag-of-words representation and defines a final prediction for the earthquake category.
- Furthermore, they introduce a distributed cyberinfrastructure that can support the processing of high volumes of data in real time and allows the redirection of data to other processing data centers in case of disaster situations.
What’s the key achievement?
- precision – 100% vs. 63.2%;
- recall – 100% vs. 85.7%;
- F1 score – 100% vs. 72.7%.
- precision – 76.7% vs. 70.7%;
- recall – 38.8% vs. 34.1%;
- F1 score – 51.6% vs. 45.0%.
What does the AI community think?
- The paper received an Outstanding Paper award at AAAI 2020 (special track on AI for Social Impact).
What are future research areas?
- Evaluating DMSEEW response time and robustness via simulation of different scenarios in an existing EEW execution platform.
- Evaluating the DMSEEW system on another seismic network.

2. Efficiently Sampling Functions from Gaussian Process Posteriors , by James T. Wilson, Viacheslav Borovitskiy, Alexander Terenin, Peter Mostowsky, Marc Peter Deisenroth
Gaussian processes are the gold standard for many real-world modeling problems, especially in cases where a model’s success hinges upon its ability to faithfully represent predictive uncertainty. These problems typically exist as parts of larger frameworks, wherein quantities of interest are ultimately defined by integrating over posterior distributions. These quantities are frequently intractable, motivating the use of Monte Carlo methods. Despite substantial progress in scaling up Gaussian processes to large training sets, methods for accurately generating draws from their posterior distributions still scale cubically in the number of test locations. We identify a decomposition of Gaussian processes that naturally lends itself to scalable sampling by separating out the prior from the data. Building off of this factorization, we propose an easy-to-use and general-purpose approach for fast posterior sampling, which seamlessly pairs with sparse approximations to afford scalability both during training and at test time. In a series of experiments designed to test competing sampling schemes’ statistical properties and practical ramifications, we demonstrate how decoupled sample paths accurately represent Gaussian process posteriors at a fraction of the usual cost.
In this paper, the authors explore techniques for efficiently sampling from Gaussian process (GP) posteriors. After investigating the behaviors of naive approaches to sampling and fast approximation strategies using Fourier features, they find that many of these strategies are complementary. They, therefore, introduce an approach that incorporates the best of different sampling approaches. First, they suggest decomposing the posterior as the sum of a prior and an update. Then they combine this idea with techniques from literature on approximate GPs and obtain an easy-to-use general-purpose approach for fast posterior sampling. The experiments demonstrate that decoupled sample paths accurately represent GP posteriors at a much lower cost.
- The introduced approach to sampling functions from GP posteriors centers on the observation that it is possible to implicitly condition Gaussian random variables by combining them with an explicit corrective term.
- The authors translate this intuition to Gaussian processes and suggest decomposing the posterior as the sum of a prior and an update.
- Building on this factorization, the researchers suggest an efficient approach for fast posterior sampling that seamlessly pairs with sparse approximations to achieve scalability both during training and at test time.
- Introducing an easy-to-use and general-purpose approach to sampling from GP posteriors.
- avoid many shortcomings of the alternative sampling strategies;
- accurately represent GP posteriors at a much lower cost; for example, simulation of a well-known model of a biological neuron required only 20 seconds using decoupled sampling, while the iterative approach required 10 hours.
- The paper received an Honorable Mention at ICML 2020.
Where can you get implementation code?
- The authors released the implementation of this paper on GitHub .
3. Dota 2 with Large Scale Deep Reinforcement Learning , by Christopher Berner, Greg Brockman, Brooke Chan, Vicki Cheung, Przemysław “Psyho” Dębiak, Christy Dennison, David Farhi, Quirin Fischer, Shariq Hashme, Chris Hesse, Rafal Józefowicz, Scott Gray, Catherine Olsson, Jakub Pachocki, Michael Petrov, Henrique Pondé de Oliveira Pinto, Jonathan Raiman, Tim Salimans, Jeremy Schlatter, Jonas Schneider, Szymon Sidor, Ilya Sutskever, Jie Tang, Filip Wolski, Susan Zhang
On April 13th, 2019, OpenAI Five became the first AI system to defeat the world champions at an esports game. The game of Dota 2 presents novel challenges for AI systems such as long time horizons, imperfect information, and complex, continuous state-action spaces, all challenges which will become increasingly central to more capable AI systems. OpenAI Five leveraged existing reinforcement learning techniques, scaled to learn from batches of approximately 2 million frames every 2 seconds. We developed a distributed training system and tools for continual training which allowed us to train OpenAI Five for 10 months. By defeating the Dota 2 world champion (Team OG), OpenAI Five demonstrates that self-play reinforcement learning can achieve superhuman performance on a difficult task.
The OpenAI research team demonstrates that modern reinforcement learning techniques can achieve superhuman performance in such a challenging esports game as Dota 2. The challenges of this particular task for the AI system lies in the long time horizons, partial observability, and high dimensionality of observation and action spaces. To tackle this game, the researchers scaled existing RL systems to unprecedented levels with thousands of GPUs utilized for 10 months. The resulting OpenAI Five model was able to defeat the Dota 2 world champions and won 99.4% of over 7000 games played during the multi-day showcase.

- The goal of the introduced OpenAI Five model is to find the policy that maximizes the probability of winning the game against professional human players, which in practice implies maximizing the reward function with some additional signals like characters dying, resources collected, etc.
- While the Dota 2 engine runs at 30 frames per second, the OpenAI Five only acts on every 4th frame.
- At each timestep, the model receives an observation with all the information available to human players (approximated in a set of data arrays) and returns a discrete action , which encodes the desired movement, attack, etc.
- A policy is defined as a function from the history of observations to a probability distribution over actions that are parameterized as an LSTM with ~159M parameters.
- The policy is trained using a variant of advantage actor critic, Proximal Policy Optimization.
- The OpenAI Five model was trained for 180 days spread over 10 months of real time.

- defeated the Dota 2 world champions in a best-of-three match (2–0);
- won 99.4% of over 7000 games during a multi-day online showcase.
- Applying introduced methods to other zero-sum two-team continuous environments.
What are possible business applications?
- Tackling challenging esports games like Dota 2 can be a promising step towards solving advanced real-world problems using reinforcement learning techniques.
4. Towards a Human-like Open-Domain Chatbot , by Daniel Adiwardana, Minh-Thang Luong, David R. So, Jamie Hall, Noah Fiedel, Romal Thoppilan, Zi Yang, Apoorv Kulshreshtha, Gaurav Nemade, Yifeng Lu, Quoc V. Le
We present Meena, a multi-turn open-domain chatbot trained end-to-end on data mined and filtered from public domain social media conversations. This 2.6B parameter neural network is simply trained to minimize perplexity of the next token. We also propose a human evaluation metric called Sensibleness and Specificity Average (SSA), which captures key elements of a human-like multi-turn conversation. Our experiments show strong correlation between perplexity and SSA. The fact that the best perplexity end-to-end trained Meena scores high on SSA (72% on multi-turn evaluation) suggests that a human-level SSA of 86% is potentially within reach if we can better optimize perplexity. Additionally, the full version of Meena (with a filtering mechanism and tuned decoding) scores 79% SSA, 23% higher in absolute SSA than the existing chatbots we evaluated.
In contrast to most modern conversational agents, which are highly specialized, the Google research team introduces a chatbot Meena that can chat about virtually anything. It’s built on a large neural network with 2.6B parameters trained on 341 GB of text. The researchers also propose a new human evaluation metric for open-domain chatbots, called Sensibleness and Specificity Average (SSA), which can capture important attributes for human conversation. They demonstrate that this metric correlates highly with perplexity, an automatic metric that is readily available. Thus, the Meena chatbot, which is trained to minimize perplexity, can conduct conversations that are more sensible and specific compared to other chatbots. Particularly, the experiments demonstrate that Meena outperforms existing state-of-the-art chatbots by a large margin in terms of the SSA score (79% vs. 56%) and is closing the gap with human performance (86%).

- Despite recent progress, open-domain chatbots still have significant weaknesses: their responses often do not make sense or are too vague or generic.
- Meena is built on a seq2seq model with Evolved Transformer (ET) that includes 1 ET encoder block and 13 ET decoder blocks.
- The model is trained on multi-turn conversations with the input sequence including all turns of the context (up to 7) and the output sequence being the response.
- making sense,
- being specific.
- The research team discovered that the SSA metric shows high negative correlation (R2 = 0.93) with perplexity, a readily available automatic metric that Meena is trained to minimize.
- Proposing a simple human-evaluation metric for open-domain chatbots.
- The best end-to-end trained Meena model outperforms existing state-of-the-art open-domain chatbots by a large margin, achieving an SSA score of 72% (vs. 56%).
- Furthermore, the full version of Meena, with a filtering mechanism and tuned decoding, further advances the SSA score to 79%, which is not far from the 86% SSA achieved by the average human.
- “Google’s “Meena” chatbot was trained on a full TPUv3 pod (2048 TPU cores) for 30 full days – that’s more than $1,400,000 of compute time to train this chatbot model.” – Elliot Turner, CEO and founder of Hyperia .
- “So I was browsing the results for the new Google chatbot Meena, and they look pretty OK (if boring sometimes). However, every once in a while it enters ‘scary sociopath mode,’ which is, shall we say, sub-optimal” – Graham Neubig, Associate professor at Carnegie Mellon University .

- Lowering the perplexity through improvements in algorithms, architectures, data, and compute.
- Considering other aspects of conversations beyond sensibleness and specificity, such as, for example, personality and factuality.
- Tackling safety and bias in the models.
- further humanizing computer interactions;
- improving foreign language practice;
- making interactive movie and videogame characters relatable.
- Considering the challenges related to safety and bias in the models, the authors haven’t released the Meena model yet. However, they are still evaluating the risks and benefits and may decide otherwise in the coming months.
5. Language Models are Few-Shot Learners , by Tom B. Brown, Benjamin Mann, Nick Ryder, Melanie Subbiah, Jared Kaplan, Prafulla Dhariwal, Arvind Neelakantan, Pranav Shyam, Girish Sastry, Amanda Askell, Sandhini Agarwal, Ariel Herbert-Voss, Gretchen Krueger, Tom Henighan, Rewon Child, Aditya Ramesh, Daniel M. Ziegler, Jeffrey Wu, Clemens Winter, Christopher Hesse, Mark Chen, Eric Sigler, Mateusz Litwin, Scott Gray, Benjamin Chess, Jack Clark, Christopher Berner, Sam McCandlish, Alec Radford, Ilya Sutskever, Dario Amodei
Recent work has demonstrated substantial gains on many NLP tasks and benchmarks by pre-training on a large corpus of text followed by fine-tuning on a specific task. While typically task-agnostic in architecture, this method still requires task-specific fine-tuning datasets of thousands or tens of thousands of examples. By contrast, humans can generally perform a new language task from only a few examples or from simple instructions – something which current NLP systems still largely struggle to do. Here we show that scaling up language models greatly improves task-agnostic, few-shot performance, sometimes even reaching competitiveness with prior state-of-the-art fine-tuning approaches. Specifically, we train GPT-3, an autoregressive language model with 175 billion parameters, 10× more than any previous non-sparse language model, and test its performance in the few-shot setting. For all tasks, GPT-3 is applied without any gradient updates or fine-tuning, with tasks and few-shot demonstrations specified purely via text interaction with the model. GPT-3 achieves strong performance on many NLP datasets, including translation, question-answering, and cloze tasks, as well as several tasks that require on-the-fly reasoning or domain adaptation, such as unscrambling words, using a novel word in a sentence, or performing 3-digit arithmetic. At the same time, we also identify some datasets where GPT-3’s few-shot learning still struggles, as well as some datasets where GPT-3 faces methodological issues related to training on large web corpora. Finally, we find that GPT-3 can generate samples of news articles which human evaluators have difficulty distinguishing from articles written by humans. We discuss broader societal impacts of this finding and of GPT-3 in general.
The OpenAI research team draws attention to the fact that the need for a labeled dataset for every new language task limits the applicability of language models. Considering that there is a wide range of possible tasks and it’s often difficult to collect a large labeled training dataset, the researchers suggest an alternative solution, which is scaling up language models to improve task-agnostic few-shot performance. They test their solution by training a 175B-parameter autoregressive language model, called GPT-3 , and evaluating its performance on over two dozen NLP tasks. The evaluation under few-shot learning, one-shot learning, and zero-shot learning demonstrates that GPT-3 achieves promising results and even occasionally outperforms the state of the art achieved by fine-tuned models.

- The GPT-3 model uses the same model and architecture as GPT-2, including the modified initialization, pre-normalization, and reversible tokenization.
- However, in contrast to GPT-2, it uses alternating dense and locally banded sparse attention patterns in the layers of the transformer, as in the Sparse Transformer .
- Few-shot learning , when the model is given a few demonstrations of the task (typically, 10 to 100) at inference time but with no weight updates allowed.
- One-shot learning , when only one demonstration is allowed, together with a natural language description of the task.
- Zero-shot learning , when no demonstrations are allowed and the model has access only to a natural language description of the task.
- On the CoQA benchmark, 81.5 F1 in the zero-shot setting, 84.0 F1 in the one-shot setting, and 85.0 F1 in the few-shot setting, compared to the 90.7 F1 score achieved by fine-tuned SOTA.
- On the TriviaQA benchmark, 64.3% accuracy in the zero-shot setting, 68.0% in the one-shot setting, and 71.2% in the few-shot setting, surpassing the state of the art (68%) by 3.2%.
- On the LAMBADA dataset, 76.2 % accuracy in the zero-shot setting, 72.5% in the one-shot setting, and 86.4% in the few-shot setting, surpassing the state of the art (68%) by 18%.
- The news articles generated by the 175B-parameter GPT-3 model are hard to distinguish from real ones, according to human evaluations (with accuracy barely above the chance level at ~52%).
- “The GPT-3 hype is way too much. It’s impressive (thanks for the nice compliments!) but it still has serious weaknesses and sometimes makes very silly mistakes. AI is going to change the world, but GPT-3 is just a very early glimpse. We have a lot still to figure out.” – Sam Altman, CEO and co-founder of OpenAI .
- “I’m shocked how hard it is to generate text about Muslims from GPT-3 that has nothing to do with violence… or being killed…” – Abubakar Abid, CEO and founder of Gradio .
- “No. GPT-3 fundamentally does not understand the world that it talks about. Increasing corpus further will allow it to generate a more credible pastiche but not fix its fundamental lack of comprehension of the world. Demos of GPT-4 will still require human cherry picking.” – Gary Marcus, CEO and founder of Robust.ai .
- “Extrapolating the spectacular performance of GPT3 into the future suggests that the answer to life, the universe and everything is just 4.398 trillion parameters.” – Geoffrey Hinton, Turing Award winner .
- Improving pre-training sample efficiency.
- Exploring how few-shot learning works.
- Distillation of large models down to a manageable size for real-world applications.
- The model with 175B parameters is hard to apply to real business problems due to its impractical resource requirements, but if the researchers manage to distill this model down to a workable size, it could be applied to a wide range of language tasks, including question answering, dialog agents, and ad copy generation.
- The code itself is not available, but some dataset statistics together with unconditional, unfiltered 2048-token samples from GPT-3 are released on GitHub .
6. Beyond Accuracy: Behavioral Testing of NLP models with CheckList , by Marco Tulio Ribeiro, Tongshuang Wu, Carlos Guestrin, Sameer Singh
Although measuring held-out accuracy has been the primary approach to evaluate generalization, it often overestimates the performance of NLP models, while alternative approaches for evaluating models either focus on individual tasks or on specific behaviors. Inspired by principles of behavioral testing in software engineering, we introduce CheckList, a task-agnostic methodology for testing NLP models. CheckList includes a matrix of general linguistic capabilities and test types that facilitate comprehensive test ideation, as well as a software tool to generate a large and diverse number of test cases quickly. We illustrate the utility of CheckList with tests for three tasks, identifying critical failures in both commercial and state-of-art models. In a user study, a team responsible for a commercial sentiment analysis model found new and actionable bugs in an extensively tested model. In another user study, NLP practitioners with CheckList created twice as many tests, and found almost three times as many bugs as users without it.
The authors point out the shortcomings of existing approaches to evaluating performance of NLP models. A single aggregate statistic, like accuracy, makes it difficult to estimate where the model is failing and how to fix it. The alternative evaluation approaches usually focus on individual tasks or specific capabilities. To address the lack of comprehensive evaluation approaches, the researchers introduce CheckList , a new evaluation methodology for testing of NLP models. The approach is inspired by principles of behavioral testing in software engineering. Basically, CheckList is a matrix of linguistic capabilities and test types that facilitates test ideation. Multiple user studies demonstrate that CheckList is very effective at discovering actionable bugs, even in extensively tested NLP models.

- The primary approach to the evaluation of models’ generalization capabilities, which is accuracy on held-out data, may lead to performance overestimation, as the held-out data often contains the same biases as the training data. Moreover, this single aggregate statistic doesn’t help much in figuring out where the NLP model is failing and how to fix these bugs.
- The alternative approaches are usually designed for evaluation of specific behaviors on individual tasks and thus, lack comprehensiveness.
- CheckList provides users with a list of linguistic capabilities to be tested, like vocabulary, named entity recognition, and negation.
- Then, to break down potential capability failures into specific behaviors, CheckList suggests different test types , such as prediction invariance or directional expectation tests in case of certain perturbations.
- Potential tests are structured as a matrix, with capabilities as rows and test types as columns.
- The suggested implementation of CheckList also introduces a variety of abstractions to help users generate large numbers of test cases easily.
- Evaluation of state-of-the-art models with CheckList demonstrated that even though some NLP tasks are considered “solved” based on accuracy results, the behavioral testing highlights many areas for improvement.
- helps to identify and test for capabilities not previously considered;
- results in more thorough and comprehensive testing for previously considered capabilities;
- helps to discover many more actionable bugs.
- The paper received the Best Paper Award at ACL 2020, the leading conference in natural language processing.
- CheckList can be used to create more exhaustive testing for a variety of NLP tasks.
- Such comprehensive testing that helps in identifying many actionable bugs is likely to lead to more robust NLP systems.
- The code for testing NLP models with CheckList is available on GitHub .
7. EfficientDet: Scalable and Efficient Object Detection , by Mingxing Tan, Ruoming Pang, Quoc V. Le
Model efficiency has become increasingly important in computer vision. In this paper, we systematically study neural network architecture design choices for object detection and propose several key optimizations to improve efficiency. First, we propose a weighted bi-directional feature pyramid network (BiFPN), which allows easy and fast multi-scale feature fusion; Second, we propose a compound scaling method that uniformly scales the resolution, depth, and width for all backbone, feature network, and box/class prediction networks at the same time. Based on these optimizations and EfficientNet backbones, we have developed a new family of object detectors, called EfficientDet, which consistently achieve much better efficiency than prior art across a wide spectrum of resource constraints. In particular, with single-model and single-scale, our EfficientDet-D7 achieves state-of-the-art 52.2 AP on COCO test-dev with 52M parameters and 325B FLOPs, being 4×–9× smaller and using 13×–42× fewer FLOPs than previous detectors. Code is available on https://github.com/google/automl/tree/master/efficientdet .
The large size of object detection models deters their deployment in real-world applications such as self-driving cars and robotics. To address this problem, the Google Research team introduces two optimizations, namely (1) a weighted bi-directional feature pyramid network (BiFPN) for efficient multi-scale feature fusion and (2) a novel compound scaling method. By combining these optimizations with the EfficientNet backbones, the authors develop a family of object detectors, called EfficientDet . The experiments demonstrate that these object detectors consistently achieve higher accuracy with far fewer parameters and multiply-adds (FLOPs).

- A weighted bi-directional feature pyramid network (BiFPN) for easy and fast multi-scale feature fusion. It learns the importance of different input features and repeatedly applies top-down and bottom-up multi-scale feature fusion.
- A new compound scaling method for simultaneous scaling of the resolution, depth, and width for all backbone, feature network, and box/class prediction networks.
- These optimizations, together with the EfficientNet backbones, allow the development of a new family of object detectors, called EfficientDet .
- the EfficientDet model with 52M parameters gets state-of-the-art 52.2 AP on the COCO test-dev dataset, outperforming the previous best detector with 1.5 AP while being 4× smaller and using 13× fewer FLOPs;
- with simple modifications, the EfficientDet model achieves 81.74% mIOU accuracy, outperforming DeepLabV3+ by 1.7% on Pascal VOC 2012 semantic segmentation with 9.8x fewer FLOPs;
- the EfficientDet models are up to 3× to 8× faster on GPU/CPU than previous detectors.
- The paper was accepted to CVPR 2020, the leading conference in computer vision.
- The high level of interest in the code implementations of this paper makes this research one of the highest-trending papers introduced recently.
- The high accuracy and efficiency of the EfficientDet detectors may enable their application for real-world tasks, including self-driving cars and robotics.
- The authors released the official TensorFlow implementation of EfficientDet.
- The PyTorch implementation of this paper can be found here and here .
8. Unsupervised Learning of Probably Symmetric Deformable 3D Objects from Images in the Wild , by Shangzhe Wu, Christian Rupprecht, Andrea Vedaldi
We propose a method to learn 3D deformable object categories from raw single-view images, without external supervision. The method is based on an autoencoder that factors each input image into depth, albedo, viewpoint and illumination. In order to disentangle these components without supervision, we use the fact that many object categories have, at least in principle, a symmetric structure. We show that reasoning about illumination allows us to exploit the underlying object symmetry even if the appearance is not symmetric due to shading. Furthermore, we model objects that are probably, but not certainly, symmetric by predicting a symmetry probability map, learned end-to-end with the other components of the model. Our experiments show that this method can recover very accurately the 3D shape of human faces, cat faces and cars from single-view images, without any supervision or a prior shape model. On benchmarks, we demonstrate superior accuracy compared to another method that uses supervision at the level of 2D image correspondences.
The research group from the University of Oxford studies the problem of learning 3D deformable object categories from single-view RGB images without additional supervision. To decompose the image into depth, albedo, illumination, and viewpoint without direct supervision for these factors, they suggest starting by assuming objects to be symmetric. Then, considering that real-world objects are never fully symmetrical, at least due to variations in pose and illumination, the researchers augment the model by explicitly modeling illumination and predicting a dense map with probabilities that any given pixel has a symmetric counterpart. The experiments demonstrate that the introduced approach achieves better reconstruction results than other unsupervised methods. Moreover, it outperforms the recent state-of-the-art method that leverages keypoint supervision.

- no access to 2D or 3D ground truth information such as keypoints, segmentation, depth maps, or prior knowledge of a 3D model;
- using an unconstrained collection of single-view images without having multiple views of the same instance.
- leveraging symmetry as a geometric cue to constrain the decomposition;
- explicitly modeling illumination and using it as an additional cue for recovering the shape;
- augmenting the model to account for potential lack of symmetry – particularly, predicting a dense map that contains the probability of a given pixel having a symmetric counterpart in the image.
- Qualitative evaluation of the suggested approach demonstrates that it reconstructs 3D faces of humans and cats with high fidelity, containing fine details of the nose, eyes, and mouth.
- The method reconstructs higher-quality shapes compared to other state-of-the-art unsupervised methods, and even outperforms the DepthNet model, which uses 2D keypoint annotations for depth prediction.

- The paper received the Best Paper Award at CVPR 2020, the leading conference in computer vision.
- Reconstructing more complex objects by extending the model to use either multiple canonical views or a different 3D representation, such as a mesh or a voxel map.
- Improving model performance under extreme lighting conditions and for extreme poses.
- The implementation code and demo are available on GitHub .
9. An Image is Worth 16×16 Words: Transformers for Image Recognition at Scale , by Alexey Dosovitskiy, Lucas Beyer, Alexander Kolesnikov, Dirk Weissenborn, Xiaohua Zhai, Thomas Unterthiner, Mostafa Dehghani, Matthias Minderer, Georg Heigold, Sylvain Gelly, Jakob Uszkoreit, Neil Houlsby
While the Transformer architecture has become the de-facto standard for natural language processing tasks, its applications to computer vision remain limited. In vision, attention is either applied in conjunction with convolutional networks, or used to replace certain components of convolutional networks while keeping their overall structure in place. We show that this reliance on CNNs is not necessary and a pure transformer can perform very well on image classification tasks when applied directly to sequences of image patches. When pre-trained on large amounts of data and transferred to multiple recognition benchmarks (ImageNet, CIFAR-100, VTAB, etc.), Vision Transformer attain excellent results compared to state-of-the-art convolutional networks while requiring substantially fewer computational resources to train.
The authors of this paper show that a pure Transformer can perform very well on image classification tasks. They introduce Vision Transformer (ViT) , which is applied directly to sequences of image patches by analogy with tokens (words) in NLP. When trained on large datasets of 14M–300M images, Vision Transformer approaches or beats state-of-the-art CNN-based models on image recognition tasks. In particular, it achieves an accuracy of 88.36% on ImageNet, 90.77% on ImageNet-ReaL, 94.55% on CIFAR-100, and 77.16% on the VTAB suite of 19 tasks.

- When applying Transformer architecture to images, the authors follow as closely as possible the design of the original Transformer designed for NLP.
- splitting images into fixed-size patches;
- linearly embedding each of them;
- adding position embeddings to the resulting sequence of vectors;
- feeding the patches to a standard Transformer encoder;
- adding an extra learnable ‘classification token’ to the sequence.
- Similarly to Transformers in NLP, Vision Transformer is typically pre-trained on large datasets and fine-tuned to downstream tasks.
- 88.36% on ImageNet;
- 90.77% on ImageNet-ReaL;
- 94.55% on CIFAR-100;
- 97.56% on Oxford-IIIT Pets;
- 99.74% on Oxford Flowers-102;
- 77.16% on the VTAB suite of 19 tasks.

- The paper is trending in the AI research community, as evident from the repository stats on GitHub .
- It is also under review for ICLR 2021 , one of the key conferences in deep learning.
- Applying Vision Transformer to other computer vision tasks, such as detection and segmentation.
- Exploring self-supervised pre-training methods.
- Analyzing the few-shot properties of Vision Transformer.
- Exploring contrastive pre-training.
- Further scaling ViT.
- Thanks to their efficient pre-training and high performance, Transformers may substitute convolutional networks in many computer vision applications, including navigation, automatic inspection, and visual surveillance.
- The PyTorch implementation of Vision Transformer is available on GitHub .
10. AdaBelief Optimizer: Adapting Stepsizes by the Belief in Observed Gradients , by Juntang Zhuang, Tommy Tang, Sekhar Tatikonda, Nicha Dvornek, Yifan Ding, Xenophon Papademetris, James S. Duncan
Most popular optimizers for deep learning can be broadly categorized as adaptive methods (e.g. Adam) or accelerated schemes (e.g. stochastic gradient descent (SGD) with momentum). For many models such as convolutional neural networks (CNNs), adaptive methods typically converge faster but generalize worse compared to SGD; for complex settings such as generative adversarial networks (GANs), adaptive methods are typically the default because of their stability. We propose AdaBelief to simultaneously achieve three goals: fast convergence as in adaptive methods, good generalization as in SGD, and training stability. The intuition for AdaBelief is to adapt the step size according to the “belief” in the current gradient direction. Viewing the exponential moving average (EMA) of the noisy gradient as the prediction of the gradient at the next time step, if the observed gradient greatly deviates from the prediction, we distrust the current observation and take a small step; if the observed gradient is close to the prediction, we trust it and take a large step. We validate AdaBelief in extensive experiments, showing that it outperforms other methods with fast convergence and high accuracy on image classification and language modeling. Specifically, on ImageNet, AdaBelief achieves comparable accuracy to SGD. Furthermore, in the training of a GAN on Cifar10, AdaBelief demonstrates high stability and improves the quality of generated samples compared to a well-tuned Adam optimizer. Code is available at https://github.com/juntang-zhuang/Adabelief-Optimizer .
The researchers introduce AdaBelief , a new optimizer, which combines the high convergence speed of adaptive optimization methods and good generalization capabilities of accelerated stochastic gradient descent (SGD) schemes. The core idea behind the AdaBelief optimizer is to adapt step size based on the difference between predicted gradient and observed gradient: the step is small if the observed gradient deviates significantly from the prediction, making us distrust this observation, and the step is large when the current observation is close to the prediction, making us believe in this observation. The experiments confirm that AdaBelief combines fast convergence of adaptive methods, good generalizability of the SGD family, and high stability in the training of GANs.
- The idea of the AdaBelief optimizer is to combine the advantages of adaptive optimization methods (e.g., Adam) and accelerated SGD optimizers. Adaptive methods typically converge faster, while SGD optimizers demonstrate better generalization performance.
- If the observed gradient deviates greatly from the prediction, we have a weak belief in this observation and take a small step.
- If the observed gradient is close to the prediction, we have a strong belief in this observation and take a large step.
- fast convergence, like adaptive optimization methods;
- good generalization, like the SGD family;
- training stability in complex settings such as GAN.
- In image classification tasks on CIFAR and ImageNet, AdaBelief demonstrates as fast convergence as Adam and as good generalization as SGD.
- It outperforms other methods in language modeling.
- In the training of a WGAN , AdaBelief significantly improves the quality of generated images compared to Adam.
- The paper was accepted to NeurIPS 2020, the top conference in artificial intelligence.
- It is also trending in the AI research community, as evident from the repository stats on GitHub .
- AdaBelief can boost the development and application of deep learning models as it can be applied to the training of any model that numerically estimates parameter gradient.
- Both PyTorch and Tensorflow implementations are released on GitHub.
If you like these research summaries, you might be also interested in the following articles:
- GPT-3 & Beyond: 10 NLP Research Papers You Should Read
- Novel Computer Vision Research Papers From 2020
- AAAI 2021: Top Research Papers With Business Applications
- ICLR 2021: Key Research Papers
Enjoy this article? Sign up for more AI research updates.
We’ll let you know when we release more summary articles like this one.
- Email Address *
- Name * First Last
- Natural Language Processing (NLP)
- Chatbots & Conversational AI
- Computer Vision
- Ethics & Safety
- Machine Learning
- Deep Learning
- Reinforcement Learning
- Generative Models
- Other (Please Describe Below)
- What is your biggest challenge with AI research? *
Reader Interactions
About Mariya Yao
Mariya is the co-author of Applied AI: A Handbook For Business Leaders and former CTO at Metamaven. She "translates" arcane technical concepts into actionable business advice for executives and designs lovable products people actually want to use. Follow her on Twitter at @thinkmariya to raise your AI IQ.
May 16, 2021 at 8:13 pm
Merci pour ces informations massives
March 16, 2024 at 10:58 pm
It is perfect time to make a few plans for the longer trrm and it is time to be happy. I’ve learn this submit annd iff I may I desire to counnsel you some interesting things or advice.
Maybe you could write next articles referring to this article. I want to learn more things about it!
Here is my web page … Eleanore
March 21, 2024 at 5:48 pm
2020’s Top AI & Machine Learning Research Papers mytgpczlq http://www.gabu6e0lozi87m5i503901r03g7p5ec4s.org/ [url=http://www.gabu6e0lozi87m5i503901r03g7p5ec4s.org/]umytgpczlq[/url] amytgpczlq
March 25, 2024 at 11:22 pm
I’m excited to see where you’ll go next. illplaywithyou
Leave a Reply
You must be logged in to post a comment.
About TOPBOTS
- Expert Contributors
- Terms of Service & Privacy Policy
- Contact TOPBOTS
Your Writing Assistant for Research
Unlock Your Research Potential with Jenni AI
Are you an academic researcher seeking assistance in your quest to create remarkable research and scientific papers? Jenni AI is here to empower you, not by doing the work for you, but by enhancing your research process and efficiency. Explore how Jenni AI can elevate your academic writing experience and accelerate your journey toward academic excellence.

Loved by over 1 million academics

Academia's Trusted Companion
Join our academic community and elevate your research journey alongside fellow scholars with Jenni AI.

Effortlessly Ignite Your Research Ideas
Unlock your potential with these standout features
Boost Productivity
Save time and effort with AI assistance, allowing you to focus on critical aspects of your research. Craft well-structured, scholarly papers with ease, backed by AI-driven recommendations and real-time feedback.
Get started

Overcome Writer's Block
Get inspiration and generate ideas to break through the barriers of writer's block. Jenni AI generates research prompts tailored to your subject, sparking your creativity and guiding your research.
Unlock Your Full Writing Potential
Jenni AI is designed to boost your academic writing capabilities, not as a shortcut, but as a tool to help you overcome writer's block and enhance your research papers' quality.

Ensure Accuracy
Properly format citations and references, ensuring your work meets academic standards. Jenni AI offers accurate and hassle-free citation assistance, including APA, MLA, and Chicago styles.
Our Commitment: Academic Honesty
Jenni AI is committed to upholding academic integrity. Our tool is designed to assist, not replace, your effort in research and writing. We strongly discourage any unethical use. We're dedicated to helping you excel in a responsible and ethical manner.
How it Works
Sign up for free.
To get started, sign up for a free account on Jenni AI's platform.
Prompt Generation
Input your research topic, and Jenni AI generates comprehensive prompts to kickstart your paper.
Research Assistance
Find credible sources, articles, and relevant data with ease through our powerful AI-driven research assistant.
Writing Support
Draft and refine your paper with real-time suggestions for structure, content, and clarity.
Citation & References
Let Jenni AI handle your citations and references in multiple styles, saving you valuable time.
What Our Users Say
Discover how Jenni AI has made a difference in the lives of academics just like you

· Aug 26
I thought AI writing was useless. Then I found Jenni AI, the AI-powered assistant for academic writing. It turned out to be much more advanced than I ever could have imagined. Jenni AI = ChatGPT x 10.

Charlie Cuddy
@sonofgorkhali
· 23 Aug
Love this use of AI to assist with, not replace, writing! Keep crushing it @Davidjpark96 💪

Waqar Younas, PhD
@waqaryofficial
· 6 Apr
4/9 Jenni AI's Outline Builder is a game-changer for organizing your thoughts and structuring your content. Create detailed outlines effortlessly, ensuring your writing is clear and coherent. #OutlineBuilder #WritingTools #JenniAI

I started with Jenni-who & Jenni-what. But now I can't write without Jenni. I love Jenni AI and am amazed to see how far Jenni has come. Kudos to http://Jenni.AI team.

· 28 Jul
Jenni is perfect for writing research docs, SOPs, study projects presentations 👌🏽

Stéphane Prud'homme
http://jenni.ai is awesome and super useful! thanks to @Davidjpark96 and @whoisjenniai fyi @Phd_jeu @DoctoralStories @WriteThatPhD
Frequently asked questions
How much does jenni ai cost, how can jenni ai assist me in writing complex academic papers, can jenni ai handle different types of academic papers, such as essays, research papers, and dissertationss jenni ai maintain the originality of my work, how does artificial intelligence enhance my academic writing with jenni ai.
Can Jenni AI help me structure and write a comprehensive literature review?
Will using Jenni AI improve my overall writing skills?
Can Jenni AI assist with crafting a thesis statement?
What sets Jenni AI apart as an AI-powered writing tool?
Can I trust Jenni AI to help me maintain academic integrity in my work?
Choosing the Right Academic Writing Companion
Get ready to make an informed decision and uncover the key reasons why Jenni AI is your ultimate tool for academic excellence.
Feature Featire
COMPETITORS
Enhanced Writing Style
Jenni AI excels in refining your writing style and enhancing sentence structure to meet academic standards with precision.
Competitors may offer basic grammar checking but often fall short in fine-tuning the nuances of writing style.
Academic Writing Process
Jenni AI streamlines the academic writing process, offering real-time assistance in content generation and thorough proofreading.
Competitors may not provide the same level of support, leaving users to navigate the intricacies of academic writing on their own.
Scientific Writing
Jenni AI is tailored for scientific writing, ensuring the clarity and precision needed in research articles and reports.
Competitors may offer generic writing tools that lack the specialized features required for scientific writing.
Original Content and Academic Integrity
Jenni AI's AI algorithms focus on producing original content while preventing plagiarism, ensuring academic integrity.
Competitors may not provide robust plagiarism checks, potentially compromising academic integrity.
Valuable Tool for Technical Writing
Jenni AI extends its versatility to technical writing, aiding in the creation of clear and concise technical documents.
Some competitors may not be as well-suited for technical writing projects.
User-Friendly Interface
Jenni AI offers an intuitive and user-friendly interface, making it easy for both novice and experienced writers to utilize its features effectively.
Some competitors may have steeper learning curves or complex interfaces, which can be time-consuming and frustrating for users.
Seamless Citation Management
Jenni AI simplifies the citation management process, offering suggestions and templates for various citation styles.
Competitors may not provide the same level of support for correct and consistent citations.
Ready to Revolutionize Your Research Writing?
Sign up for a free Jenni AI account today. Unlock your research potential and experience the difference for yourself. Your journey to academic excellence starts here.
Ask a question, get an answer backed by real research
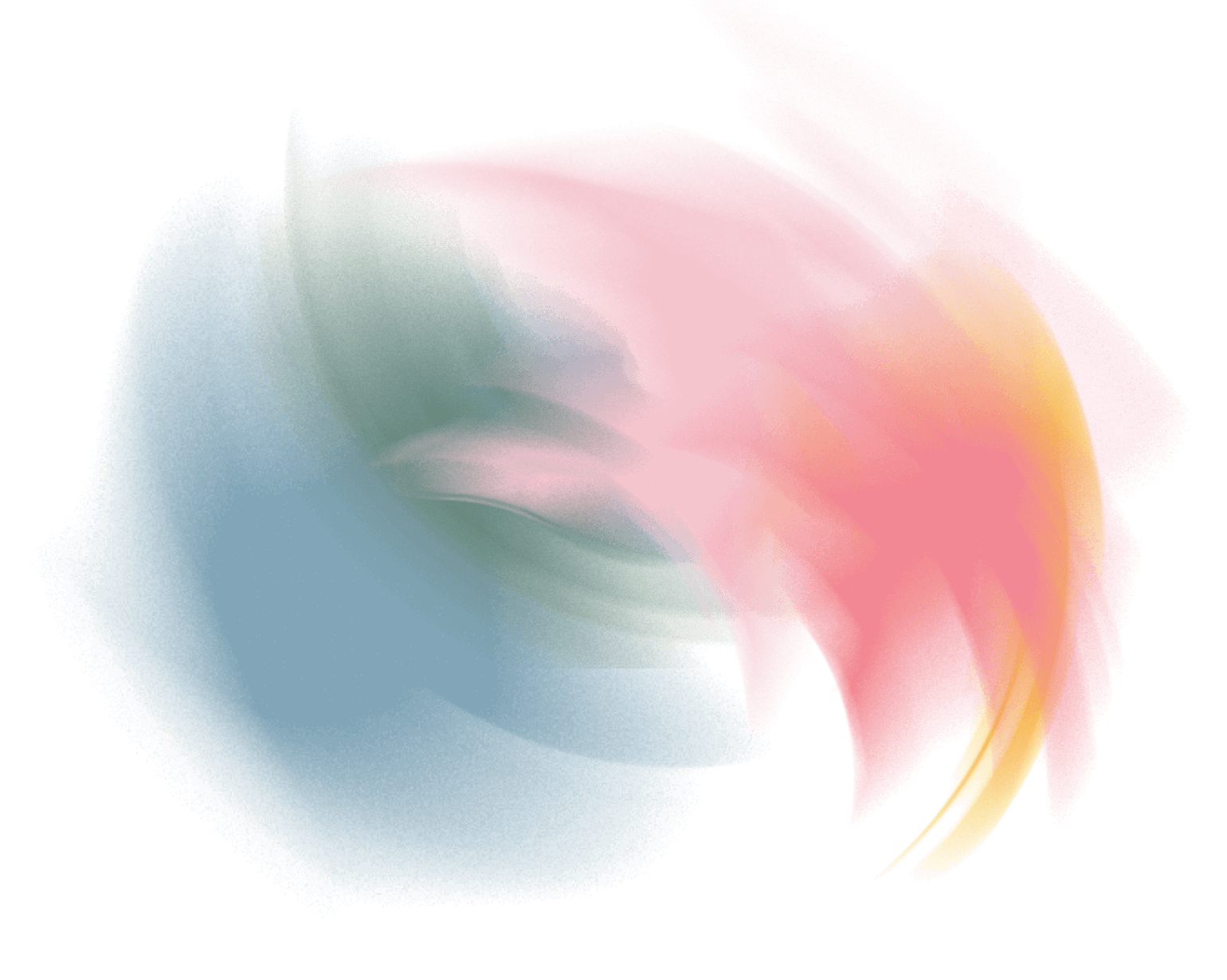
1.2b citation statements extracted and analyzed
187 m articles, book chapters, preprints, and datasets.
Trusted by leading Universities, Publishers, and Corporations across the world.
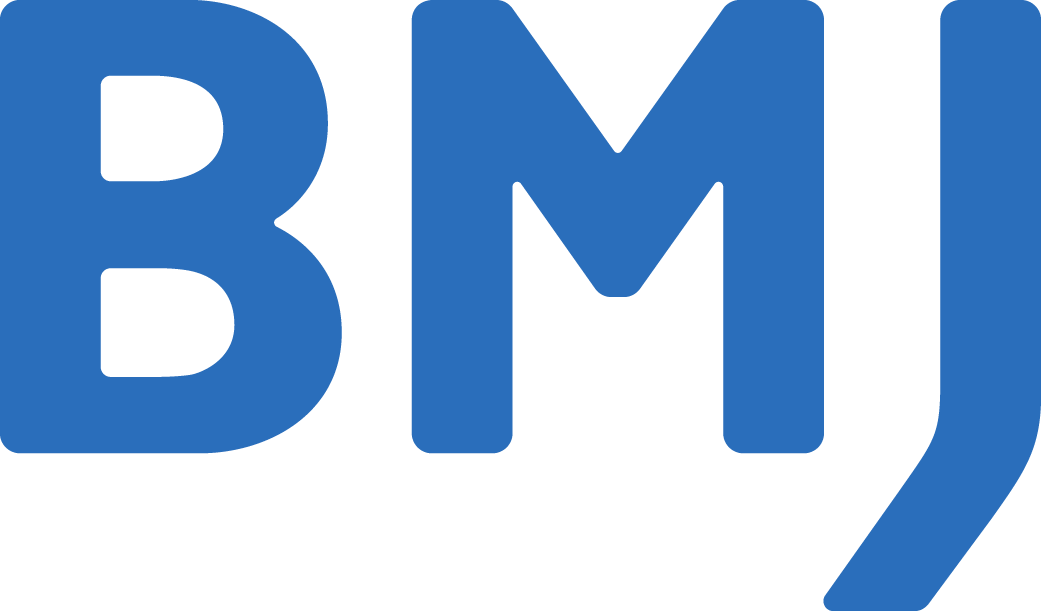
Read what research articles say about each other
scite is an award-winning platform for discovering and evaluating scientific articles via Smart Citations. Smart Citations allow users to see how a publication has been cited by providing the context of the citation and a classification describing whether it provides supporting or contrasting evidence for the cited claim.
Extracted citations in a report page
Never waste time looking for and evaluating research again.
Our innovative index of Smart Citations powers new features built to make research intuitive and trustworthy for anyone engaging with research.
Search Citation Statements
Find information by searching across a mix of metadata (like titles & abstracts) as well as Citation Statements we indexed from the full-text of research articles.
Create Custom Dashboards
Build and manage collections of articles of interest -- from a manual list, systematic review, or a search -- and get aggregate insights, notifications, and more.
Reference Check
Evaluate how references from your manuscript were used by you or your co-authors to ensure you properly cite high quality references.
Journal Metrics
Explore pre-built journal dashboards to find their publications, top authors, compare yearly scite Index rankings in subject areas, and more.
Large Language Model (LLM) Experience for Researchers
Assistant by scite gives you the power of large language models backed by our unique database of Smart Citations to minimize the risk of hallucinations and improve the quality of information and real references.
Use it to get ideas for search strategies, build reference lists for a new topic you're exploring, get help writing marketing and blog posts, and more.
Assistant is built with observability in mind to help you make more informed decisions about AI generated content.
Here are a few examples to try:
"How many rats live in NYC?"
"How does the structure of a protein affect its function?"
Awards & Press
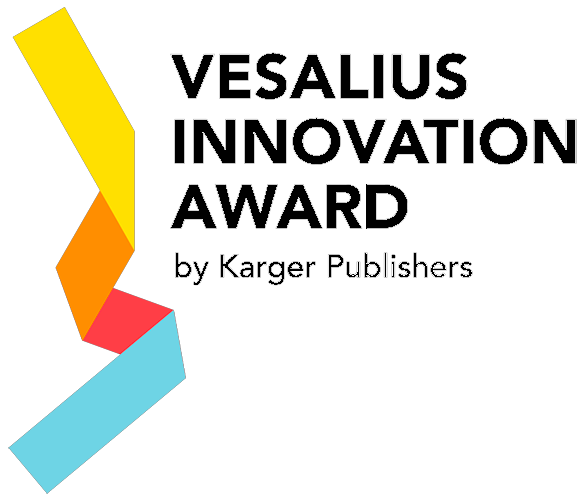
Trusted by researchers and organizations around the world
Over 650,000 students, researchers, and industry experts use scite
See what they're saying
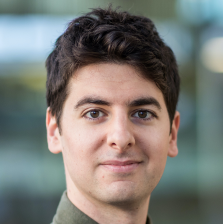
scite is an incredibly clever tool. The feature that classifies papers on whether they find supporting or contrasting evidence for a particular publication saves so much time. It has become indispensable to me when writing papers and finding related work to cite and read.
Emir Efendić, Ph.D
Maastricht University
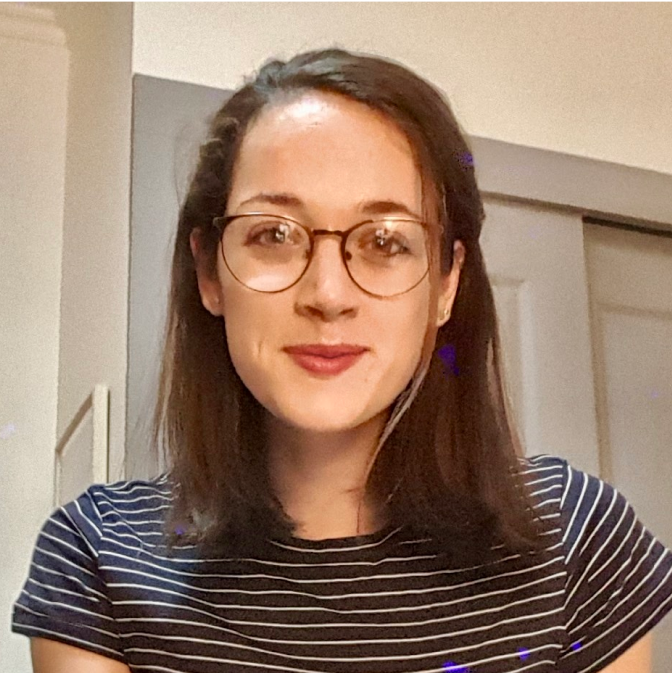
As a PhD student, I'm so glad that this exists for my literature searches and papers. Being able to assess what is disputed or affirmed in the literature is how the scientific process is supposed to work, and scite helps me do this more efficiently.
Kathleen C McCormick, Ph.D Student
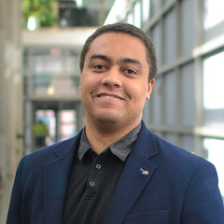
scite is such an awesome tool! It’s never been easier to place a scientific paper in the context of the wider literature.
Mark Mikkelsen, Ph.D
The Johns Hopkins University School of Medicine
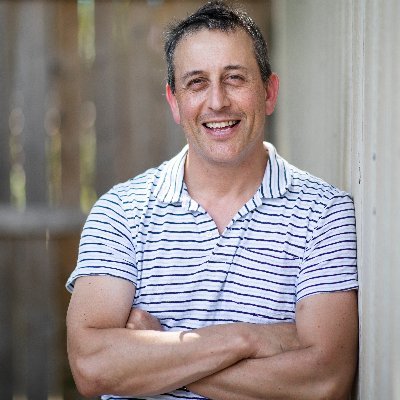
This is a really cool tool. I just tried it out on a paper we wrote on flu/pneumococcal seasonality... really interesting to see the results were affirmed by other studies. I had no idea.
David N. Fisman, Ph.D
University of Toronto
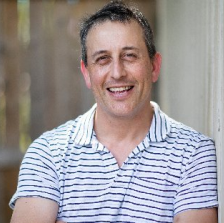
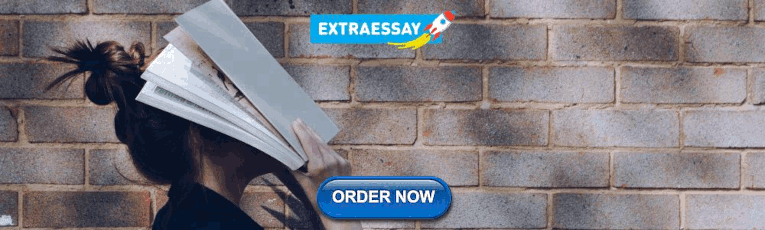
Do better research
Join scite to be a part of a community dedicated to making science more reliable.
scite is a Brooklyn-based organization that helps researchers better discover and understand research articles through Smart Citations–citations that display the context of the citation and describe whether the article provides supporting or contrasting evidence. scite is used by students and researchers from around the world and is funded in part by the National Science Foundation and the National Institute on Drug Abuse of the National Institutes of Health.
Contact Info
10624 S. Eastern Ave., Ste. A-614
Henderson, NV 89052, USA
Blog Terms and Conditions API Terms Privacy Policy Contact Cookie Preferences Do Not Sell or Share My Personal Information
Copyright © 2024 scite LLC. All rights reserved.
Made with 💙 for researchers
Part of the Research Solutions Family.
Analyze research papers at superhuman speed
Search for research papers, get one sentence abstract summaries, select relevant papers and search for more like them, extract details from papers into an organized table.
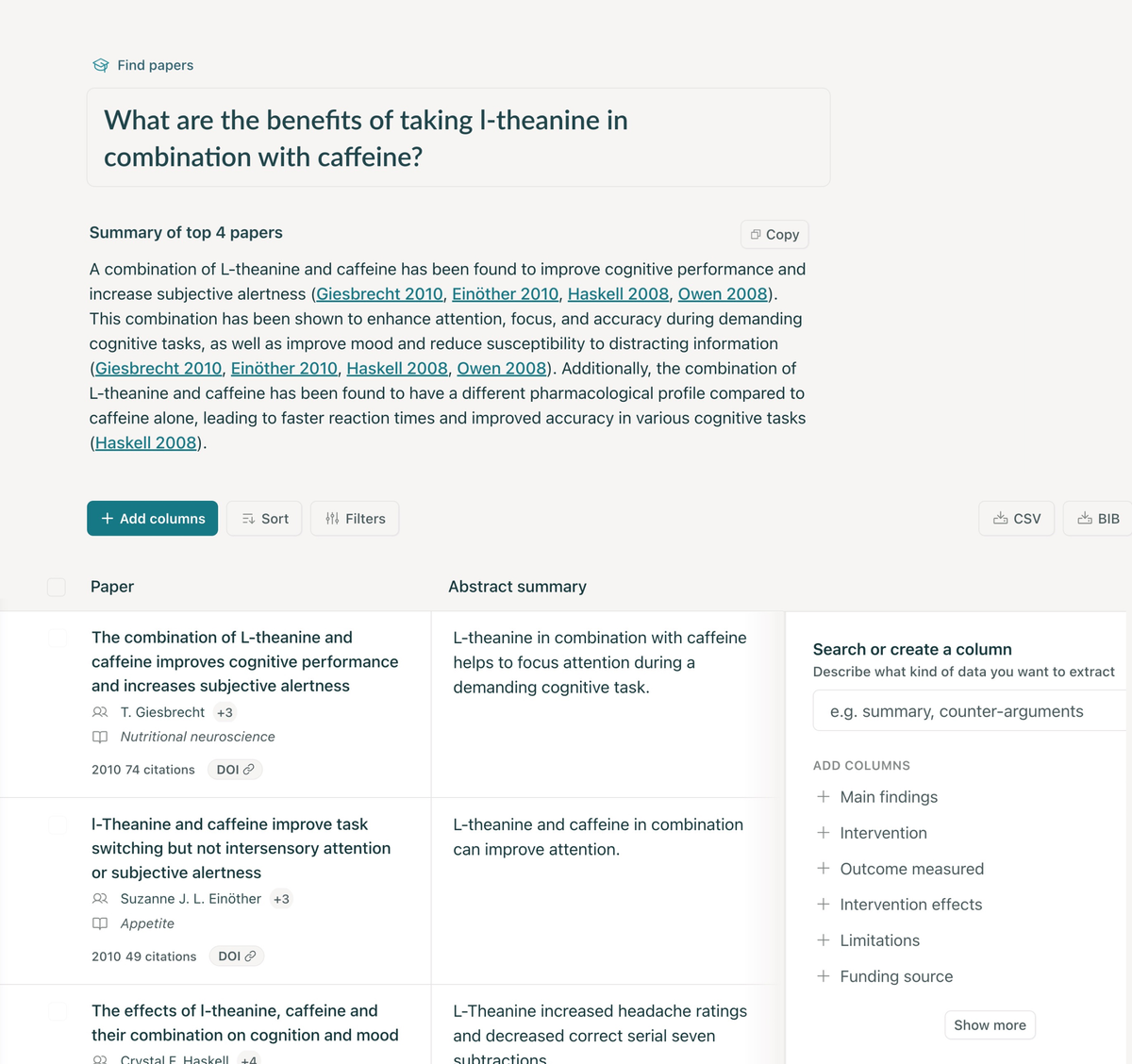
Find themes and concepts across many papers
Don't just take our word for it.
.webp)
Tons of features to speed up your research
Upload your own pdfs, orient with a quick summary, view sources for every answer, ask questions to papers, research for the machine intelligence age, pick a plan that's right for you, get in touch, enterprise and institutions, custom pricing, common questions. great answers., how do researchers use elicit.
Over 2 million researchers have used Elicit. Researchers commonly use Elicit to:
- Speed up literature review
- Find papers they couldn’t find elsewhere
- Automate systematic reviews and meta-analyses
- Learn about a new domain
Elicit tends to work best for empirical domains that involve experiments and concrete results. This type of research is common in biomedicine and machine learning.
What is Elicit not a good fit for?
Elicit does not currently answer questions or surface information that is not written about in an academic paper. It tends to work less well for identifying facts (e.g. “How many cars were sold in Malaysia last year?”) and theoretical or non-empirical domains.
What types of data can Elicit search over?
Elicit searches across 125 million academic papers from the Semantic Scholar corpus, which covers all academic disciplines. When you extract data from papers in Elicit, Elicit will use the full text if available or the abstract if not.
How accurate are the answers in Elicit?
A good rule of thumb is to assume that around 90% of the information you see in Elicit is accurate. While we do our best to increase accuracy without skyrocketing costs, it’s very important for you to check the work in Elicit closely. We try to make this easier for you by identifying all of the sources for information generated with language models.
What is Elicit Plus?
Elicit Plus is Elicit's subscription offering, which comes with a set of features, as well as monthly credits. On Elicit Plus, you may use up to 12,000 credits a month. Unused monthly credits do not carry forward into the next month. Plus subscriptions auto-renew every month.
What are credits?
Elicit uses a credit system to pay for the costs of running our app. When you run workflows and add columns to tables it will cost you credits. When you sign up you get 5,000 credits to use. Once those run out, you'll need to subscribe to Elicit Plus to get more. Credits are non-transferable.
How can you get in contact with the team?
Please email us at [email protected] or post in our Slack community if you have feedback or general comments! We log and incorporate all user comments. If you have a problem, please email [email protected] and we will try to help you as soon as possible.
What happens to papers uploaded to Elicit?
When you upload papers to analyze in Elicit, those papers will remain private to you and will not be shared with anyone else.
How accurate is Elicit?
Training our models on specific tasks, searching over academic papers, making it easy to double-check answers, save time, think more. try elicit for free..
Thank you for visiting nature.com. You are using a browser version with limited support for CSS. To obtain the best experience, we recommend you use a more up to date browser (or turn off compatibility mode in Internet Explorer). In the meantime, to ensure continued support, we are displaying the site without styles and JavaScript.
- View all journals
- Explore content
- About the journal
- Publish with us
- Sign up for alerts
- NEWS FEATURE
- 27 September 2023
- Correction 10 October 2023
AI and science: what 1,600 researchers think
- Richard Van Noorden &
- Jeffrey M. Perkel
You can also search for this author in PubMed Google Scholar
Artificial-intelligence (AI) tools are becoming increasingly common in science, and many scientists anticipate that they will soon be central to the practice of research, suggests a Nature survey of more than 1,600 researchers around the world.
Access options
Access Nature and 54 other Nature Portfolio journals
Get Nature+, our best-value online-access subscription
24,99 € / 30 days
cancel any time
Subscribe to this journal
Receive 51 print issues and online access
185,98 € per year
only 3,65 € per issue
Rent or buy this article
Prices vary by article type
Prices may be subject to local taxes which are calculated during checkout
Nature 621 , 672-675 (2023)
doi: https://doi.org/10.1038/d41586-023-02980-0
Updates & Corrections
Correction 10 October 2023 : An earlier version of this story erroneously affiliated Kedar Hippalgaonkar with the National University of Singapore.
Reprints and permissions
Supplementary Information
- AI survey methodology (docx)
- AI survey questions (pdf)
- AI survey results (xlsx)
Related Articles
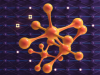
- Machine learning
- Mathematics and computing
- Computer science
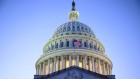
The US Congress is taking on AI —this computer scientist is helping
News Q&A 09 MAY 24
Accurate structure prediction of biomolecular interactions with AlphaFold 3
Article 08 MAY 24
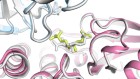
Major AlphaFold upgrade offers boost for drug discovery
News 08 MAY 24
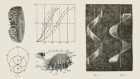
The dream of electronic newspapers becomes a reality — in 1974
News & Views 07 MAY 24
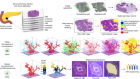
3D genomic mapping reveals multifocality of human pancreatic precancers
Article 01 MAY 24
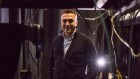
AI’s keen diagnostic eye
Outlook 18 APR 24
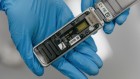
Powerful ‘nanopore’ DNA sequencing method tackles proteins too
Technology Feature 08 MAY 24
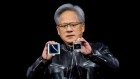
Who’s making chips for AI? Chinese manufacturers lag behind US tech giants
News 03 MAY 24
Staff Scientist
A Staff Scientist position is available in the laboratory of Drs. Elliot and Glassberg to study translational aspects of lung injury, repair and fibro
Maywood, Illinois
Loyola University Chicago - Department of Medicine
W3-Professorship (with tenure) in Inorganic Chemistry
The Institute of Inorganic Chemistry in the Faculty of Mathematics and Natural Sciences at the University of Bonn invites applications for a W3-Pro...
53113, Zentrum (DE)
Rheinische Friedrich-Wilhelms-Universität
Principal Investigator Positions at the Chinese Institutes for Medical Research, Beijing
Studies of mechanisms of human diseases, drug discovery, biomedical engineering, public health and relevant interdisciplinary fields.
Beijing, China
The Chinese Institutes for Medical Research (CIMR), Beijing
Research Associate - Neural Development Disorders
Houston, Texas (US)
Baylor College of Medicine (BCM)
Staff Scientist - Mitochondria and Surgery
Sign up for the Nature Briefing newsletter — what matters in science, free to your inbox daily.
Quick links
- Explore articles by subject
- Guide to authors
- Editorial policies
Help | Advanced Search
Computer Science > Artificial Intelligence
Title: capabilities of gemini models in medicine.
Abstract: Excellence in a wide variety of medical applications poses considerable challenges for AI, requiring advanced reasoning, access to up-to-date medical knowledge and understanding of complex multimodal data. Gemini models, with strong general capabilities in multimodal and long-context reasoning, offer exciting possibilities in medicine. Building on these core strengths of Gemini, we introduce Med-Gemini, a family of highly capable multimodal models that are specialized in medicine with the ability to seamlessly use web search, and that can be efficiently tailored to novel modalities using custom encoders. We evaluate Med-Gemini on 14 medical benchmarks, establishing new state-of-the-art (SoTA) performance on 10 of them, and surpass the GPT-4 model family on every benchmark where a direct comparison is viable, often by a wide margin. On the popular MedQA (USMLE) benchmark, our best-performing Med-Gemini model achieves SoTA performance of 91.1% accuracy, using a novel uncertainty-guided search strategy. On 7 multimodal benchmarks including NEJM Image Challenges and MMMU (health & medicine), Med-Gemini improves over GPT-4V by an average relative margin of 44.5%. We demonstrate the effectiveness of Med-Gemini's long-context capabilities through SoTA performance on a needle-in-a-haystack retrieval task from long de-identified health records and medical video question answering, surpassing prior bespoke methods using only in-context learning. Finally, Med-Gemini's performance suggests real-world utility by surpassing human experts on tasks such as medical text summarization, alongside demonstrations of promising potential for multimodal medical dialogue, medical research and education. Taken together, our results offer compelling evidence for Med-Gemini's potential, although further rigorous evaluation will be crucial before real-world deployment in this safety-critical domain.
Submission history
Access paper:.
- HTML (experimental)
- Other Formats

References & Citations
- Google Scholar
- Semantic Scholar
BibTeX formatted citation

Bibliographic and Citation Tools
Code, data and media associated with this article, recommenders and search tools.
- Institution
arXivLabs: experimental projects with community collaborators
arXivLabs is a framework that allows collaborators to develop and share new arXiv features directly on our website.
Both individuals and organizations that work with arXivLabs have embraced and accepted our values of openness, community, excellence, and user data privacy. arXiv is committed to these values and only works with partners that adhere to them.
Have an idea for a project that will add value for arXiv's community? Learn more about arXivLabs .
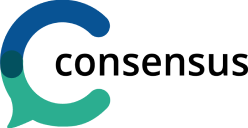
- Universities & students
- How to search
- How it works
- Start a new search
- Blog & updates
AI Search Engine for Research
Consensus is a search engine that uses AI to find insights in research papers
& start searching now!
Why Consensus?
Consensus responsibly uses AI to help you conduct effective research
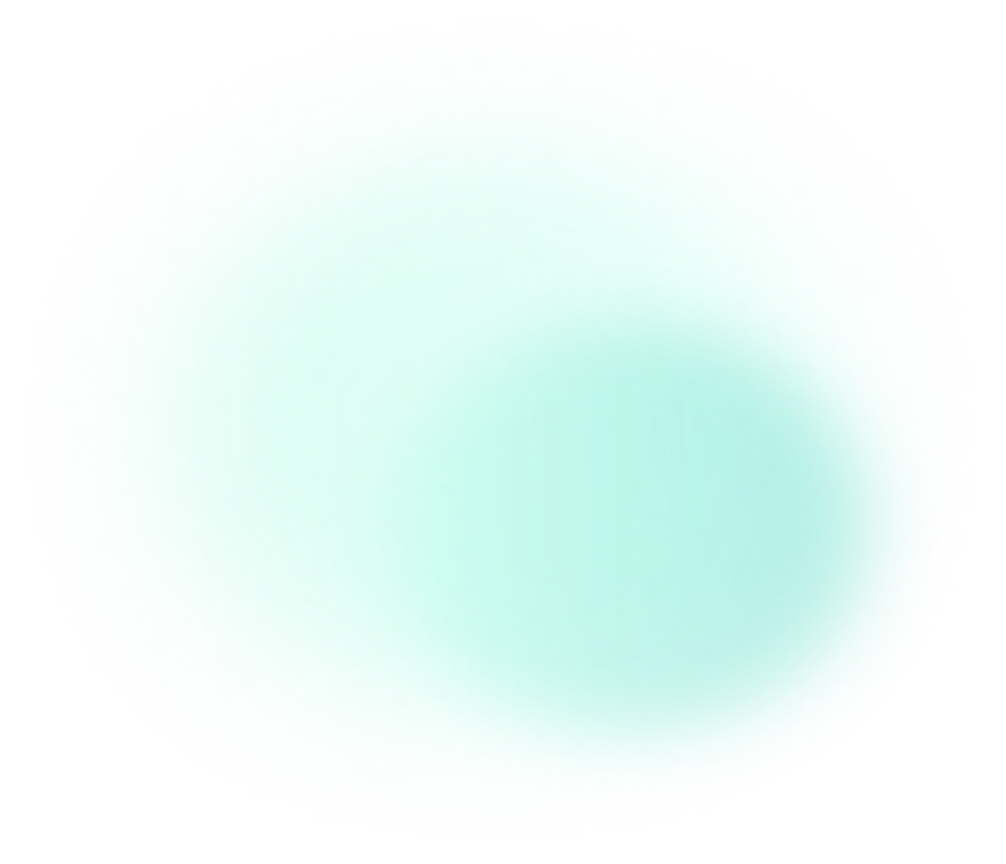
Search through over 200 million scientific papers without having to keyword match
All of our results are tied to actual studies, we cite our sources and we will never show you ads
Proprietary and purpose-built features that leverage GPT4 and other LLMs to summarize results for you
Used by researchers at the world’s top institutions
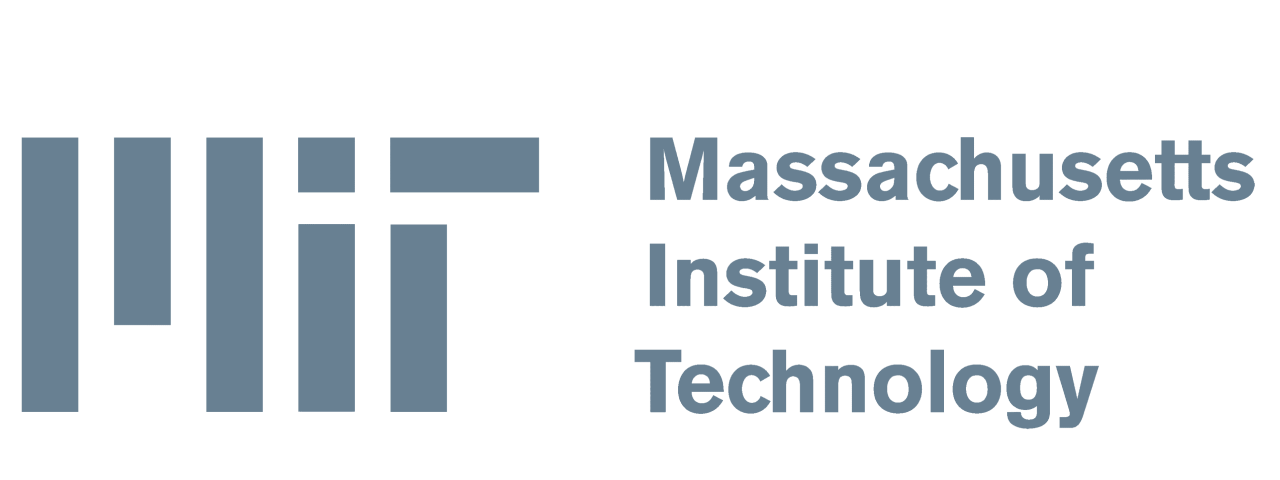
researchers, students, doctors, professionals and evidence-conscious consumers choose Consensus
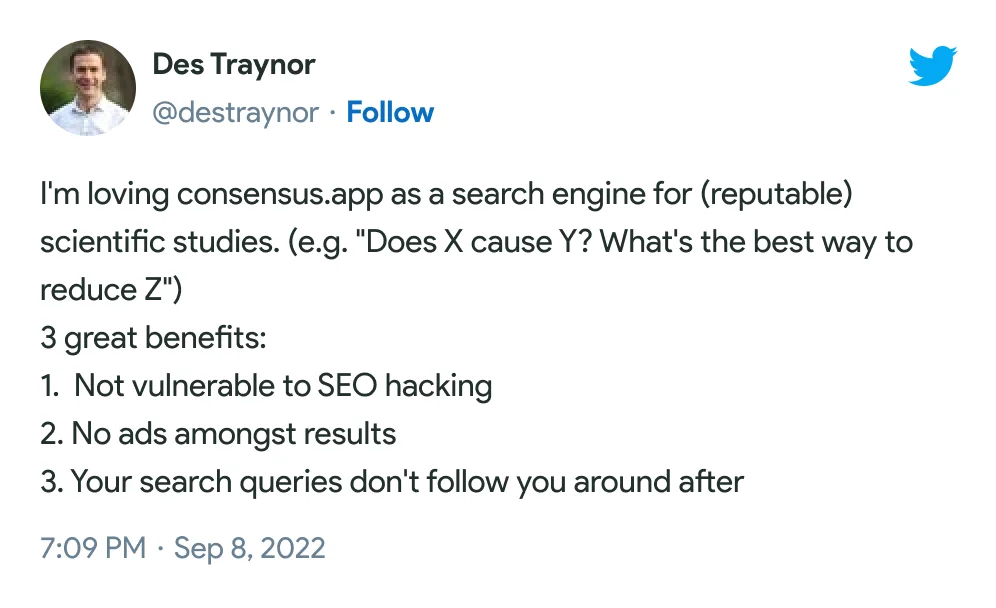
Consensus has been Featured in
Consensus helps.
Find supporting evidence for your paper
Researchers
Efficiently conduct literature reviews
Quickly find answers to patients’ questions
Instantly find expert quotes for presentations
Content Creators
Source peer-reviewed insights for your blog
Health and fitness enthusiasts
Check the viability of supplements and routines
Consensus vs ChatGPT
ChatGPT is built to have a conversation with you. Consensus is purpose-built to help you conduct effective research.
Results pulled directly from peer-reviewed studies
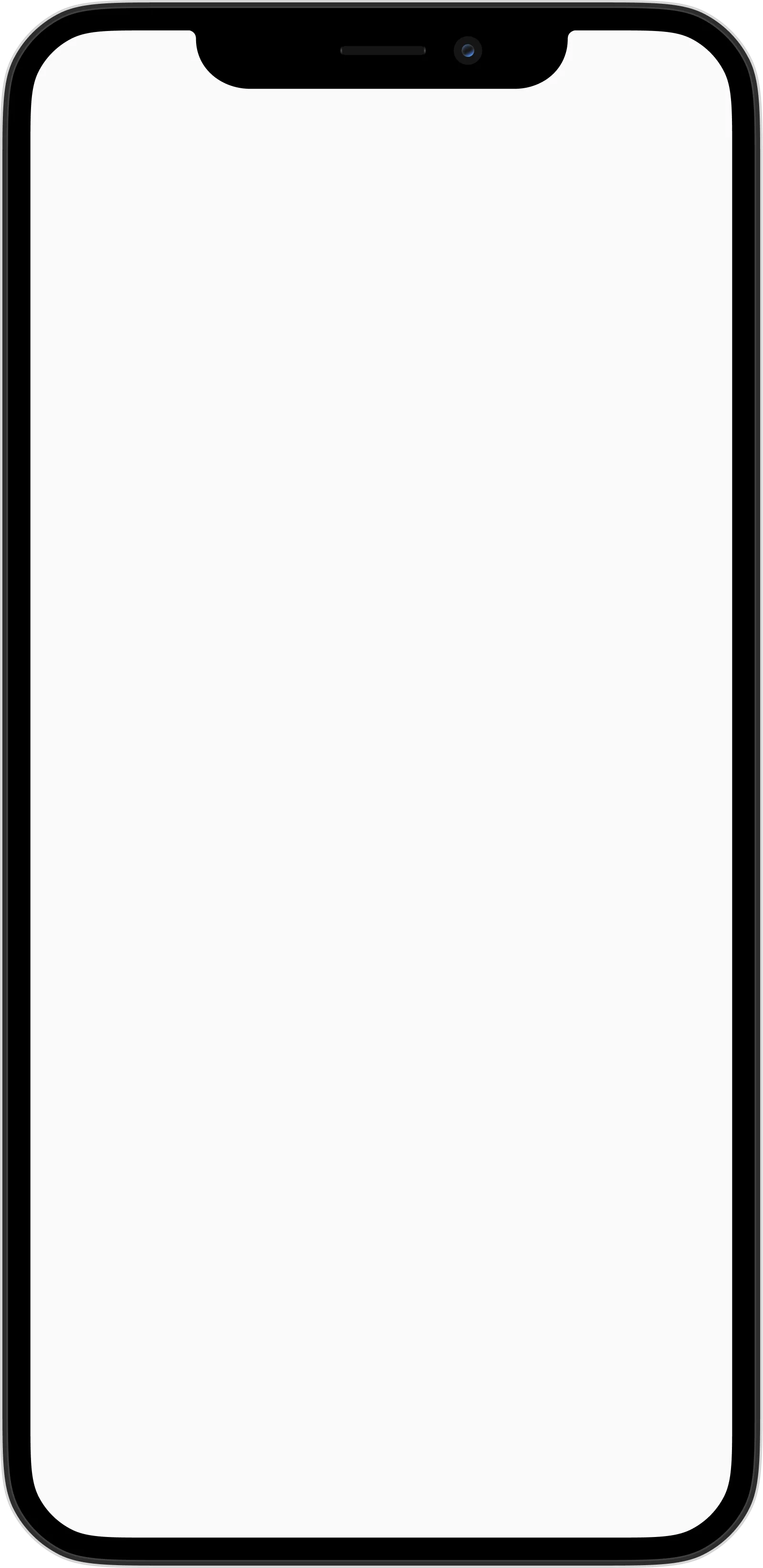
Fully machine-generated, trained on the entire internet
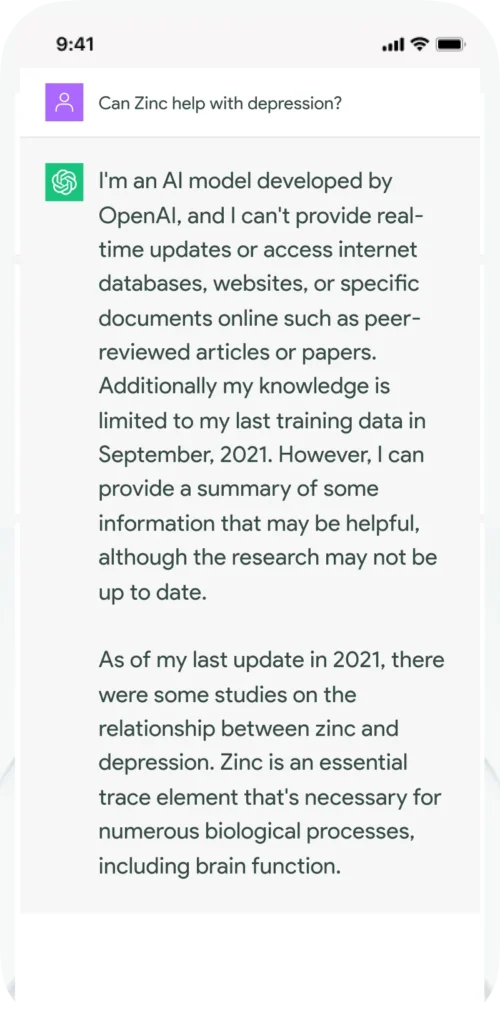
Our mission is to democratize expert knowledge
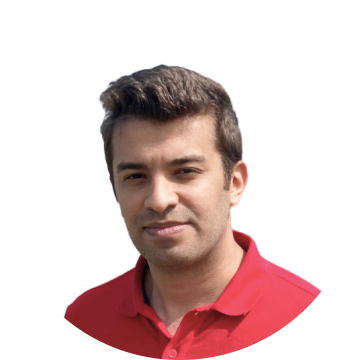
Sign up for our free BETA

Special Features
Vendor voice.
Some scientists can't stop using AI to write research papers
If you read about 'meticulous commendable intricacy' there's a chance a boffin had help.
Linguistic and statistical analyses of scientific articles suggest that generative AI may have been used to write an increasing amount of scientific literature.
Two academic papers assert that analyzing word choice in the corpus of science publications reveals an increasing usage of AI for writing research papers. One study , published in March by Andrew Gray of University College London in the UK, suggests at least one percent – 60,000 or more – of all papers published in 2023 were written at least partially by AI.
A second paper published in April by a Stanford University team in the US claims this figure might range between 6.3 and 17.5 percent, depending on the topic.
Both papers looked for certain words that large language models (LLMs) use habitually, such as “intricate,” “pivotal,” and “meticulously." By tracking the use of those words across scientific literature, and comparing this to words that aren't particularly favored by AI, the two studies say they can detect an increasing reliance on machine learning within the scientific publishing community.
In Gray's paper, the use of control words like "red," "conclusion," and "after" changed by a few percent from 2019 to 2023. The same was true of other certain adjectives and adverbs until 2023 (termed the post-LLM year by Gray).
In that year use of the words "meticulous," "commendable," and "intricate," rose by 59, 83, and 117 percent respectively, while their prevalence in scientific literature hardly changed between 2019 and 2022. The word with the single biggest increase in prevalence post-2022 was “meticulously”, up 137 percent.
The Stanford paper found similar phenomena, demonstrating a sudden increase for the words "realm," "showcasing," "intricate," and "pivotal." The former two were used about 80 percent more often than in 2021 and 2022, while the latter two were used around 120 and almost 160 percent more frequently respectively.
- Beyond the hype, AI promises leg up for scientific research
- AI researchers have started reviewing their peers using AI assistance
- Boffins deem Google DeepMind's material discoveries rather shallow
- Turns out AI chatbots are way more persuasive than humans
The researchers also considered word usage statistics in various scientific disciplines. Computer science and electrical engineering were ahead of the pack when it came to using AI-preferred language, while mathematics, physics, and papers published by the journal Nature, only saw increases of between five and 7.5 percent.
The Stanford bods also noted that authors posting more preprints, working in more crowded fields, and writing shorter papers seem to use AI more frequently. Their paper suggests that a general lack of time and a need to write as much as possible encourages the use of LLMs, which can help increase output.
Potentially the next big controversy in the scientific community
Using AI to help in the research process isn't anything new, and lots of boffins are open about utilizing AI to tweak experiments to achieve better results. However, using AI to actually write abstracts and other chunks of papers is very different, because the general expectation is that scientific articles are written by actual humans, not robots, and at least a couple of publishers consider using LLMs to write papers to be scientific misconduct.
Using AI models can be very risky as they often produce inaccurate text, the very thing scientific literature is not supposed to do. AI models can even fabricate quotations and citations, an occurrence that infamously got two New York attorneys in trouble for citing cases ChatGPT had dreamed up.
"Authors who are using LLM-generated text must be pressured to disclose this or to think twice about whether doing so is appropriate in the first place, as a matter of basic research integrity," University College London’s Gray opined.
The Stanford researchers also raised similar concerns, writing that use of generative AI in scientific literature could create "risks to the security and independence of scientific practice." ®
Narrower topics
- Large Language Model
- Machine Learning
- Neural Networks
- Tensor Processing Unit
Broader topics
- Self-driving Car
Send us news
Other stories you might like
Big brains divided over training ai with more ai: is model collapse inevitable, deepmind spinoff isomorphic claims alphafold 3 predicts bio-matter down to the dna, with run:ai acquisition, nvidia aims to manage your ai kubes, easing the cloud migration journey.
Google Search results polluted by buggy AI-written code frustrate coders
Forget the ai doom and hype, let's make computers useful, politicians call for ban on 'killer robots' and the curbing of ai weapons, intel's neuromorphic 'owl brain' swoops into sandia labs, investment analyst accuses palantir of ai washing, mitre promises a cute little 17-pflops ai super for the rest of uncle sam's agencies, add ai servers to the list of idevices apple silicon could soon power, warren buffett voices ai fears, likens tech to atom bomb.

- Advertise with us
Our Websites
- The Next Platform
- Blocks and Files
Your Privacy
- Cookies Policy
- Privacy Policy
- Ts & Cs

Copyright. All rights reserved © 1998–2024

- Data Science
- Data Analysis
- Data Visualization
- Machine Learning
- Deep Learning
- Computer Vision
- Artificial Intelligence
- AI ML DS Interview Series
- AI ML DS Projects series
- Data Engineering
- Web Scrapping
- Top 10 Free AI Tools for Video Editing
- Top 10 AI Tools for Sales (Free and Paid)
- Top 10 Best AI Tools for Startups in 2024
- Top 12 AI Testing Tools for Test Automation in 2024
- 10 Best AI Tools for Small Business Owners
- 10 Best AI Tools for Academic Research in 2024
- Top 10 Free AI Writing Tools for Content Creators
- 10 Best AI Tools for Lawyers (Free + Paid)
- Top 10 AI Content Creation Tools
- Top 10 AI Photo Editing Tools for Beginners in 2024 (Free & Easy!)
- Top 12 AI Tools for Digital Marketing: 2024 Edition
- Top 10 AI Tools for Data Analysis
- 10 Free AI Detection Tools
- 10 Best AI Tools for Contract Review
- Top AI-Powered Image Enhancing Tools
- Top 10 AI Tools for Podcasters: Exclusive List [2024]
- Top 10 Keyword Tool.io Alternatives for Keyword Research (Free) - 2024
- 10 AI Tools to Create Amazing Infographics
- Top 7 Best AI Tools for Accounting
Top 10 AI Tools for Creating Research Papers
AI tools are very easy to use and are of great help. Creating research papers requires high-end information that an AI can provide. AI tools designed specifically to help in the process of writing research papers have emerged as invaluable resources for researchers. These tools increase advanced algorithms and natural language processing (NLP) techniques to assist researchers at different stages of the writing process.
Use the tools that highlight the key features and are useful for the research. It helps in generating reviews on literature and also offers solutions to challenges.
PDFgear Copilot
Connected papers, research rabbit, faqs – top 10 ai tools for creating research papers.
Here is the list of top 10 AI tools for creating research papers:
QuillBot is an AI tool that can paraphrase sentences while the meaning remains the same. It employs advanced algorithms to generate alternative wordings, making it useful for writers seeking to enhance clarity or avoid plagiarism. QuillBot offers an easy to use interface and supports multiple languages, catering to diverse writing needs.
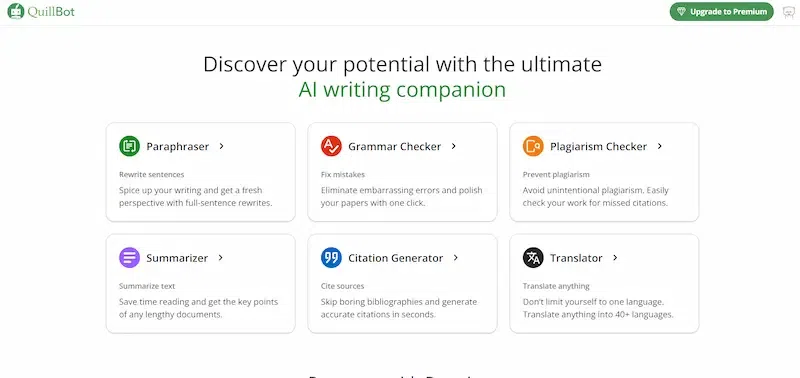
- Users can discover academic literature by providing personalized recommendations and search tools.
- Helps in organizing research materials using tags, annotating articles for easy retrieval.
- Offers multiple features for collaboration with colleagues.
- Helps in enhancing the writing quality.
- Helps in preventing plagiarism.
- Limited context is provided.
- Accuracy is limited.
- Free plan available.
- Monthly at $9.95 per month.
- Yearly at $4.17 per month.
Link: https://quillbot.com/
Bit AI is an AI tool that generates content which can be used for articles and blog posts. It uses machine language to understand and create content similar to human writing styles. It makes content accessible to a vast audience.
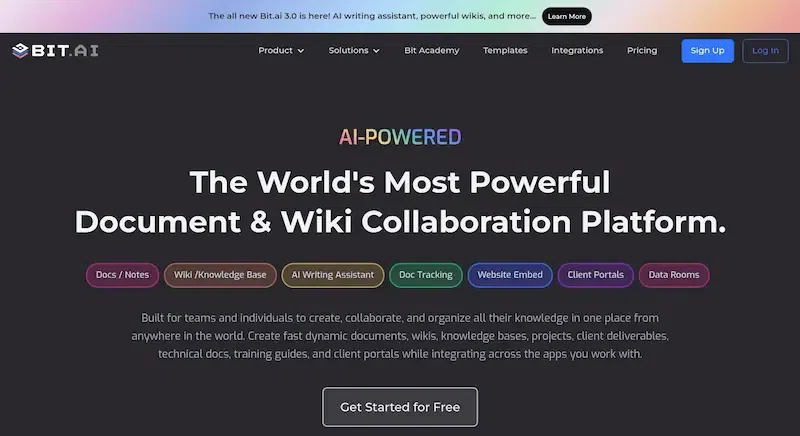
- Helps in summarizing the articles using machine learning, and extracts key information.
- Mimics the human writing styles that appear natural and engaging.
- Simplifies the complex texts and makes it highly accessible on different platforms.
- Highly versatile in providing content.
- Helps in saving time.
- Highly dependent on database access.
- Limitations in understanding the issues.
- Free plan available
- Pro at $12 per month
- Business at $20 per month.
Link: https://bit.ai/
Scite is an AI tool that analyzes academic literature to evaluate the reliability of scientific claims. It employs citation analysis and natural language processing techniques to identify supporting or contradicting evidence for research findings. Scite helps researchers assess the credibility of research articles and make informed decisions based on evidence.
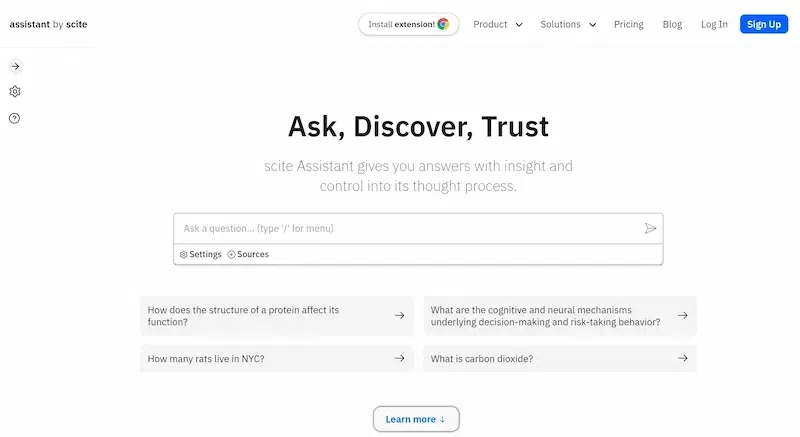
- Analysis academic literatures to enhance reliability.
- Identifies supporting evidences to research findings and helps in creating credibility in the content collected.
- Provides evidence based research and content for exploring in this vast range.
- Helps in research and explorations of literature.
- Evidence based decision making.
- Highly dependent on citation data.
- Limited scope in content.
- Individual at $10 per month
- Team can be customized.
Link: https://scite.ai/
PDFGear CoPilot is an AI tool that processes documents and converts converts PDF files into various formats with high accuracy. Offers a wide range of features like text extraction, image recognition, and document conversion, facilitating easy integration of PDF content into different applications. It manages documents and enhances productivity.
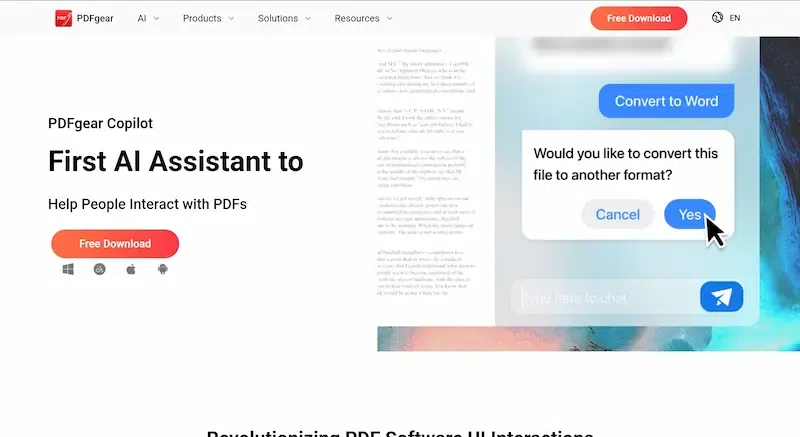
- Automates the analysis of research papers and provides useful insights.
- Saves time in performing operations.
- Free download for android
Link: https://www.pdfgear.com/pdf-copilot/
Concessus is an AI tool that is highly collaborative. Offers a wide range of features like document sharing, discussion forums, and project management tools, enabling teams to collaborate effectively. It enhances communication and productivity in content among the researchers.
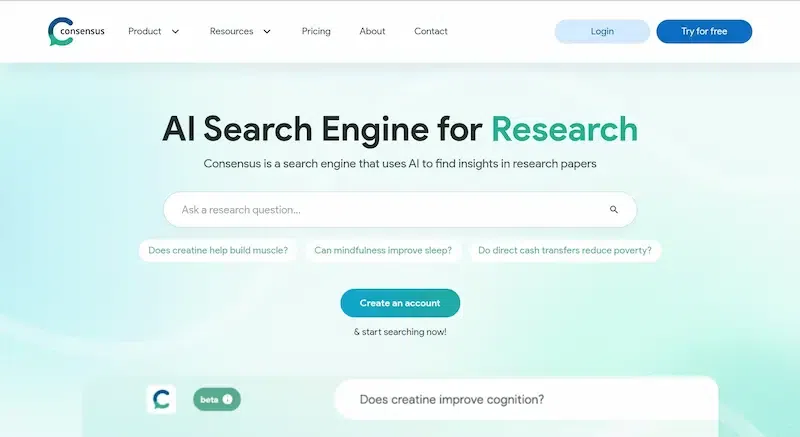
- Provides various tools for document sharing and discussion forums for collaboration.
- Allows sharing of knowledge and resources withing teams and enhances communication.
- Allows organization of research papers, and tracks progress.
- Helps in improving productivity by shatying organised.
- Offers collaboration between users.
- Highly dependent on data integration.
- Learning curve is steeper.
- Premium at $8.99 per month
- Teams at $9.99 per month
- Enterprise is customized.
Link: https://consensus.app/home/
Connected Papers is an AI research tool that visualizes the connections between research papers. It analyzes networks to identify related works and clusters them into interactive visualizations. It helps researchers explore literature, discover new connections, and gain insights into the structure of academic knowledge.
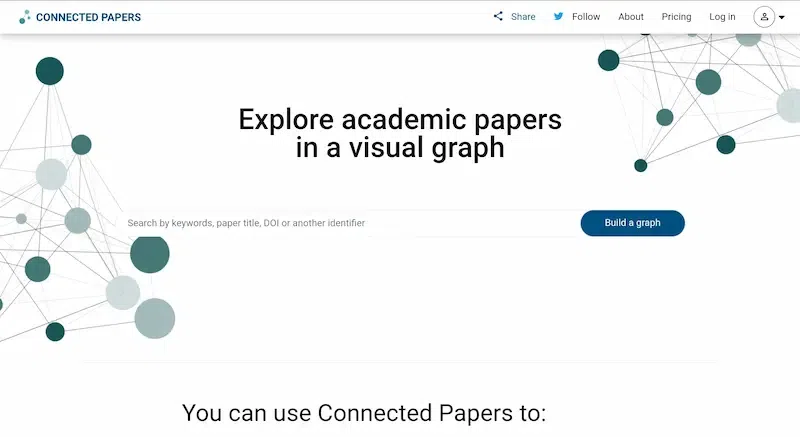
- Analysis networks and allows creating connection between research papers and identify related work.
- Generates interactive visualization of clusters to helps users in exploration of new litrature.
- Users can navigate among related litrature in an easy to use interface.
- Simplifies the research process.
- Highly accessible to a vast audience.
- Limited data coverage is provided.
- Highly complex citation.
- Academic at $3 per month.
- Business at $10 per month.
Link: https://www.connectedpapers.com/
Litmaps is a literature mapping AI tool that helps researchers organize and visualize literature. It enables users to create interactive maps of research topics, authors, and citations, facilitating exploration and analysis of academic knowledge available online. Helps in reviewing litrature and provides evidence based decision making.
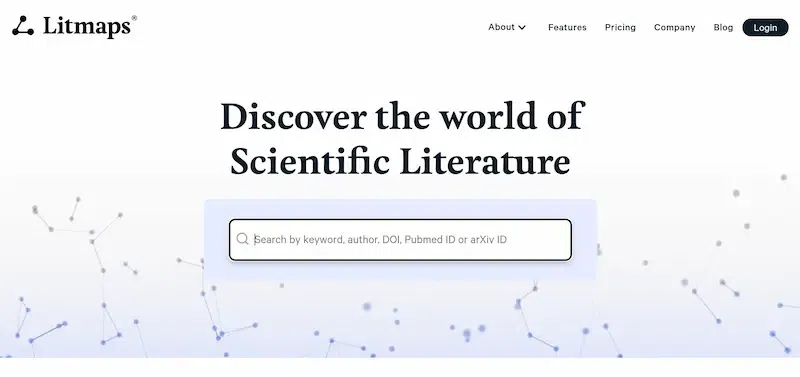
- Allows users to create interactive maps of research topics and provides visualization of academic literature.
- Provides tools for organizing research papers and managing large collection of literature.
- Allows users to collaborate among themselves and eases knowledge sharing and editing easy.
- Helps in visualization of content and mapping.
- Easy for collaboration and sharing knowledge.
- Highly dependent on data sources.
- Free for students, businesses, teams and organizations.
- Pro at $10 per month.
- Teams are customized.
Link: https://www.litmaps.com/
Jenni is an AI tool used to help in virtual research. Designed to help users find and organize articles. It employs natural language processing to understand user queries and retrieve relevant literature from academic databases. It helps in the research process and provides personalized recommendations.
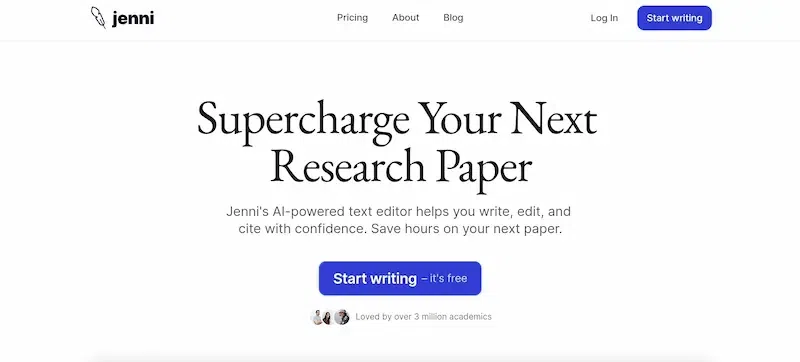
- Acts on virtual research assistant that helps in finding and organising the articles.
- Has an natural language processor to understand user issues and provide relevant information.
- Provides personalized recommendations and management tools.
- Enhances productivity by focusing on recommendations and managing tools.
- It is very time saving.
- Unlimited at $20 per month.
Link: https://jenni.ai/
Paper pal is an AI tool that can be used for creating research papers. It analyzes the articles and summarizes them. It uses machine learning to extract information from papers and generate summaries.
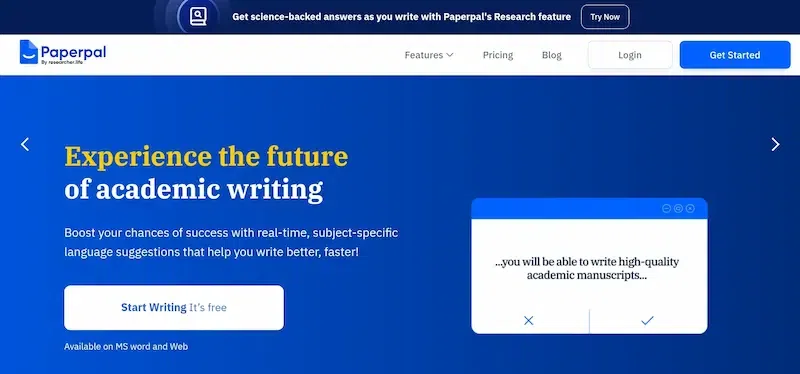
- Accuracy is compromised at times.
- Context is lost in summaries.
- Prime at $9 per month
Link: http://paperpal.com
Research Rabbit is an AI tool for creating research papers. It helps in discovering and organizing important data required for creating research papers. It helps in reviewing processes and offers personalized recommendations.
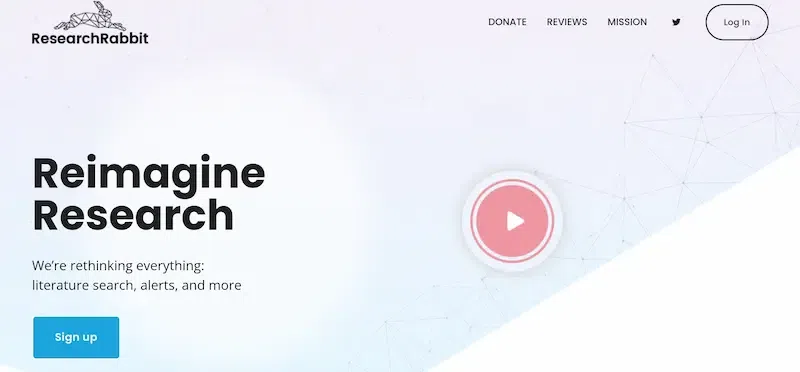
- Enhances the literature exploration by personalized recommendation.
- Allows collaboration and sharing of knowledge.
- Plans can be customized.
Link: https://www.researchrabbit.ai/https://www.researchrabbit.ai/
The development of AI tools for research paper creation has completely enhanced the efficiency of content written by writers. These tools improve writing quality, speed up the research process, and promote collaboration by utilizing artificial intelligence. They relieve researchers of some of the administrative load so they can concentrate on the important aspects of their work, such as idea generation and citation formatting.
These tools will surely become more and more essential to academic pursuits as AI develops, completely changing the way information is produced, exchanged, and shared in academic communities all over the world.
What kind of research paper creation tools can AI offer?
Artificial intelligence (AI) tools for research paper creation are software programmes that help researchers with different parts of the writing process by leveraging natural language processing and artificial intelligence algorithms.
How will AI enhance research papers?
With capabilities like automatic literature searches, article summaries, grammar and style recommendations, and citation management, artificial intelligence (AI) technologies improve the drafting of research papers.
Can one trust AI technologies to write academically?
Although AI writing tools can be helpful, their dependability is dependent on a number of aspects, including the user’s comprehension of how to interpret the ideas they provide, the quality of the algorithms they use, and the accuracy of the data sources they use.
Can AI tools take the role of human researchers?
Artificial intelligence (AI) tools support human researchers by automating some jobs and helping with research and writing, rather than taking the place of them.
To what extent may AI techniques be used for authoring research papers?
Researchers all over the world can easily use a multitude of AI tools for writing research papers through downloaded software or online platforms. While some tools are available for free with restricted functions, others demand a one-time purchase or membership in order to access all features.
Please Login to comment...
Similar reads, improve your coding skills with practice.
What kind of Experience do you want to share?
- 5 Best AI Research Paper Summarizers (May 2024)
Unite.AI is committed to rigorous editorial standards. We may receive compensation when you click on links to products we review. Please view our affiliate disclosure .
Table Of Contents
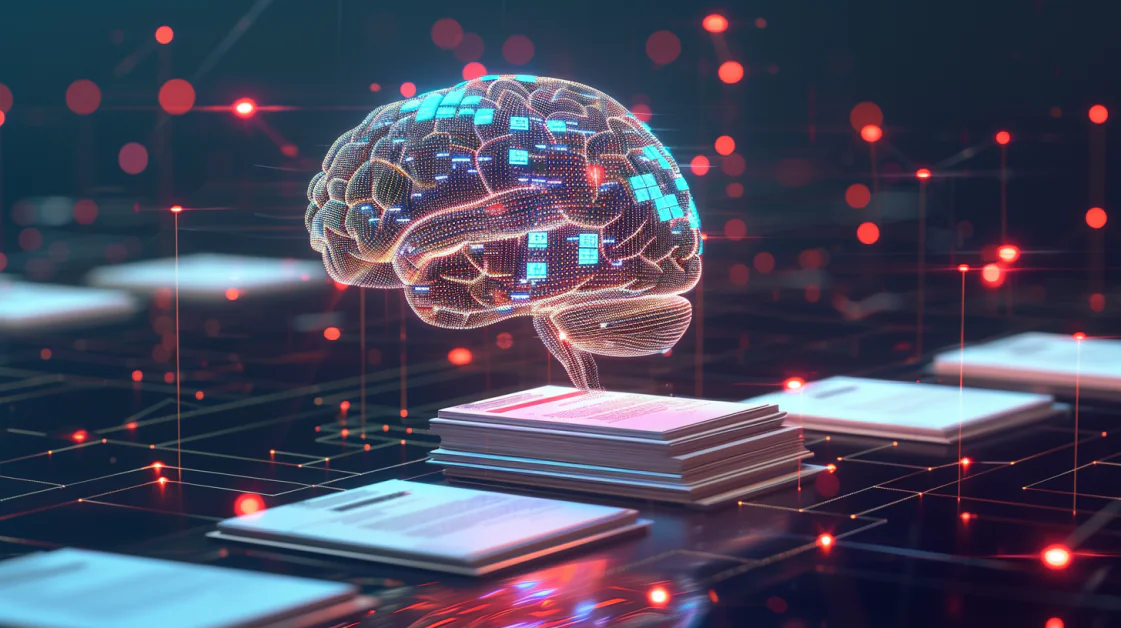
In the fast-paced world of academic research, keeping up with the ever-growing body of literature can be a daunting task. Researchers and students often find themselves inundated with lengthy research papers, making it challenging to quickly grasp the core ideas and insights. AI-powered research paper summarizers have emerged as powerful tools, leveraging advanced algorithms to condense lengthy documents into concise and readable summaries.
In this article, we will explore the top AI research paper summarizers, each designed to streamline the process of understanding and synthesizing academic literature:
1. Tenorshare AI PDF Tool
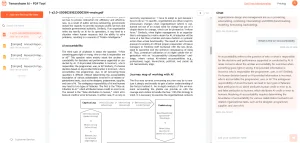
Tenorshare AI PDF Tool is a cutting-edge solution that harnesses the power of artificial intelligence to simplify the process of summarizing research papers. With its user-friendly interface and advanced AI algorithms, this tool quickly analyzes and condenses lengthy papers into concise, readable summaries, allowing researchers to grasp the core ideas without having to read the entire document.
One of the standout features of Tenorshare AI PDF Tool is its interactive chat interface, powered by ChatGPT. This innovative functionality enables users to ask questions and retrieve specific information from the PDF document, making it easier to navigate and understand complex research papers. The tool also efficiently extracts critical sections and information, such as the abstract, methodology, results, and conclusions, streamlining the reading process and helping users focus on the most relevant parts of the document.
Key features of Tenorshare AI PDF Tool:
- AI-driven summarization that quickly condenses lengthy research papers
- Interactive chat interface powered by ChatGPT for retrieving specific information
- Automatic extraction of critical sections and information from the paper
- Batch processing capabilities for handling multiple PDF files simultaneously
- Secure and private, with SSL encryption and the option to delete uploaded files

Elicit is an AI-powered research assistant that improves the way users find and summarize academic papers. With its intelligent search capabilities and advanced natural language processing, Elicit helps researchers quickly identify the most relevant papers and understand their core ideas through automatically generated summaries.
By simply entering keywords, phrases, or questions, users can leverage Elicit's AI algorithms to search through its extensive database and retrieve the most pertinent papers. The tool offers various filters and sorting options, such as publication date, study types, and citation count, enabling users to refine their search results and find exactly what they need. One of Elicit's most impressive features is its ability to generate concise summaries of the top papers related to the search query, capturing the key findings and conclusions and saving researchers valuable time.
Key features of Elicit:
- Intelligent search that understands the context and meaning of search queries
- Filters and sorting options for refining search results
- Automatic summarization of the top papers related to the search query
- Detailed paper insights, including tested outcomes, participant information, and trustworthiness assessment
- Inline referencing for transparency and accuracy verification
3. QuillBot

QuillBot is an AI-powered writing platform that offers a comprehensive suite of tools to enhance and streamline the writing process, including a powerful Summarizer tool that is particularly useful for condensing research papers. By leveraging advanced natural language processing and machine learning algorithms, QuillBot's Summarizer quickly analyzes lengthy articles, research papers, or documents and generates concise summaries that capture the core ideas and key points.
One of the key advantages of QuillBot's Summarizer is its ability to perform extractive summarization, which involves identifying and extracting the most critical sentences and information from the research paper while maintaining the original context. Users can customize the summary length to be either short (key sentences) or long (paragraph format) based on their needs, and the output can be generated in either a bullet point list format or as a coherent paragraph. This flexibility allows researchers to tailor the summary to their specific requirements and preferences.
Key features of QuillBot's Summarizer:
- AI-powered extractive summarization that identifies and extracts key information
- Customizable summary length (short or long) to suit different needs
- Bullet point or paragraph output for flexible formatting
- Improved reading comprehension by condensing the paper into its core concepts
- Integration with other QuillBot tools, such as Paraphraser and Grammar Checker, for further enhancement
4. Semantic Scholar

Semantic Scholar is a free, AI-powered research tool developed by the Allen Institute for AI that improves the way researchers search for and discover scientific literature. By employing advanced natural language processing, machine learning, and machine vision techniques, Semantic Scholar provides a smarter and more efficient way to navigate the vast landscape of academic publications.
One of the standout features of Semantic Scholar is its ability to generate concise, one-sentence summaries of research papers, capturing the essence of the content and allowing researchers to quickly grasp the main ideas without reading lengthy abstracts. This feature is particularly useful when browsing on mobile devices or when time is limited. Additionally, Semantic Scholar highlights the most important and influential citations within a paper, helping researchers focus on the most relevant information and understand the impact of the research.
Key features of Semantic Scholar:
- Concise one-sentence summaries of research papers for quick comprehension
- Identification of the most influential citations within a paper
- Personalized paper recommendations through the “Research Feed” feature
- Semantic Reader for in-line citation cards with summaries and “skimming highlights”
- Personal library management with the ability to save and organize papers
5. IBM Watson Discovery

IBM Watson Discovery is a powerful AI-driven tool designed to analyze and summarize large volumes of unstructured data, including research papers, articles, and scientific publications. By harnessing the power of cognitive computing, natural language processing, and machine learning, Watson Discovery enables researchers to quickly find relevant information and gain valuable insights from complex documents.
One of the key strengths of IBM Watson Discovery is its ability to understand the context, concepts, and relationships within the text, allowing it to identify patterns, trends, and connections that may be overlooked by human readers. This makes it easier to navigate and summarize complex research papers, as the tool can highlight important entities, relationships, and topics within the document. Users can create customizable queries, filter, and categorize data to generate summaries of the most relevant research findings, and the tool's advanced search capabilities enable precise searches and retrieval of specific information from large document libraries.
Key features of IBM Watson Discovery:
- Cognitive capabilities that understand context, concepts, and relationships within the text
- Customizable queries and filtering for generating summaries of relevant research findings
- Relationship identification to highlight important entities, relationships, and topics
- Significant time-saving by automating the discovery of information and insight
Empowering Researchers with AI-Driven Summarization Tools
The emergence of AI-powered research summarizers has transformed the way researchers and academics approach scientific literature. By leveraging advanced natural language processing, machine learning, and cognitive computing, these innovative tools enable users to quickly find, understand, and summarize complex research papers, saving valuable time and effort.
Each of these AI research summarizers offers unique features and benefits that cater to researchers' diverse needs. As these tools continue to evolve and improve, they will undoubtedly play an increasingly crucial role in empowering researchers to navigate the ever-expanding universe of scientific knowledge more efficiently and effectively.
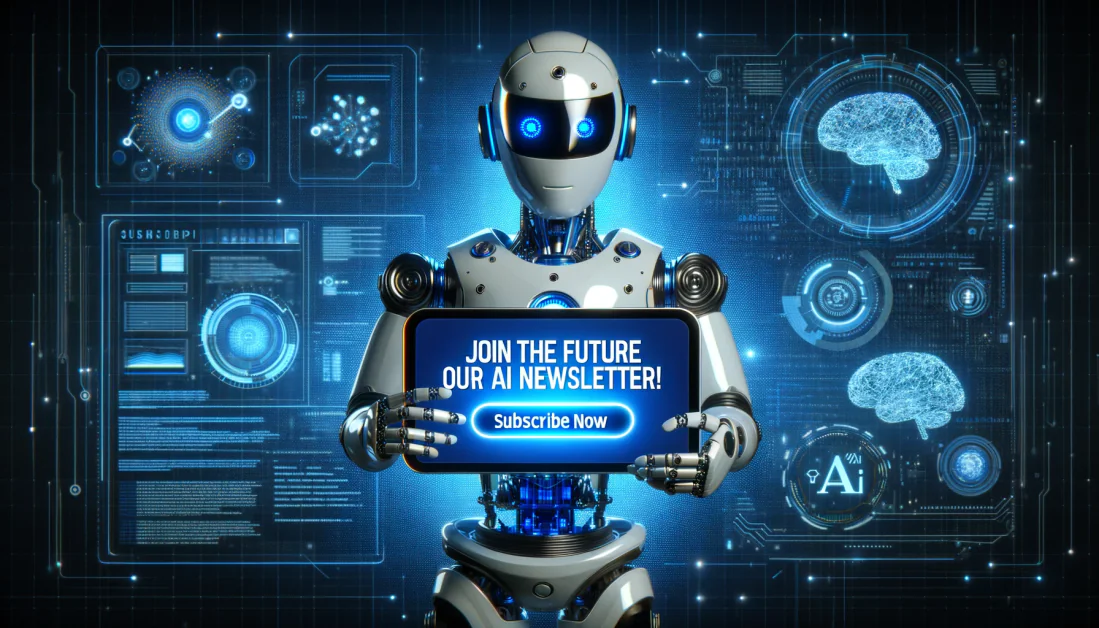
5 Best AI SOP (Standard Operating Procedures) Generators in 2024
Alex McFarland is an AI journalist and writer exploring the latest developments in artificial intelligence. He has collaborated with numerous AI startups and publications worldwide.
You may like
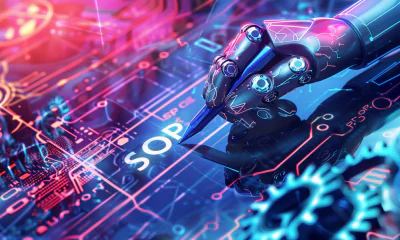
5 Best B2B Customer Support Tools (May 2024)
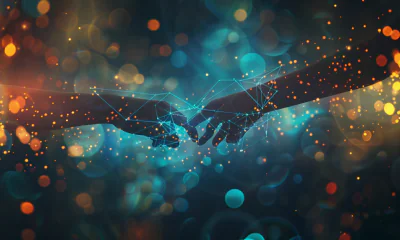
5 Best AI Apps for Couples (May 2024)
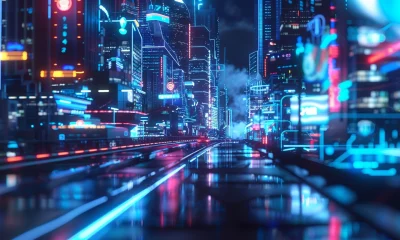
10 Best AI Shopify Tools (May 2024)
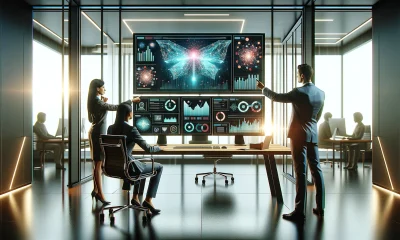
10 Best AI Business Plan Generators (May 2024)
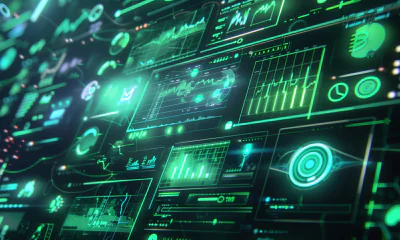
10 Best AI Tools for Google Sheets (May 2024)
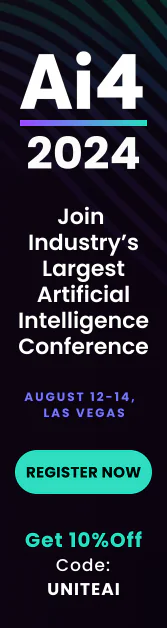
Recent Posts
- Itamar Friedman, CEO & Co-Founder of CodiumAI – Interview Series
- Hungry for Data: How Supply Chain AI Can Reach its Inflection Point
- Hostinger Review – Is This The Most Affordable Premium Webhost?
- What is AlphaFold 3? The AI Model Poised to Transform Biology
AI Assistance in Scientific Research Raises Concerns
Research indicates that generative AI is being used in scientific writing at a significant rate some of the researchers are treating it as a valid approach that can pose a threat to real research and the true nature of scholarly work.
AI’s growing influence on scientific writing
Scholars have discovered that the volume of AI-produced writing is quite substantial compared to other kinds of writing, like journals and books. Such analysis based on linguistics hints that the use of words typically associated with large language models (LLMs) like “intricate,” “pivotal,” and “meticulously” has increased substantially in the text.
The data collected by Andrew Gray from University College London reveal that after 2023, just 1% of papers in certain fields are assisted by AI. Subsequently, in April, another study from Stanford University found that the number of biased reviews falls between 6.3 and 17.5 percent based on the research subject.
Detecting AI influence
Language tests, and statistical analysis were amongst the tools used to link words or phrases to AI assistance. Despite the fact that modifying words, like ‘red’,’result’, and ‘after’ observed less variation till 2023 and then spikes in the use of some adjectives and adverbs associated with LLM-generated content begin to happen.
Precisely, the words “meticulous,” “commendable,” and “intricate” increased that much by 117%, having hit the highest rate post-2022. The Stanford study observed a Shift in Language usage in Artificial Intelligence, which indicated that AI language continues to improve in its usage in all scientific disciplines.
The research also disclosed that AI linguistic discrimination is consistent with the disciplinary disparities in AI adoption. Fields like computer science and electrical engineering are in the teaching front of AI charter language. However, fields such as mathematics, physics, or Nature didn’t feature more dramatic shifts but rather more conservative raises.
Ethical challenges in AI-assisted academic writing
The authors, being more prolific in preprints, working in the research areas where the competition is high, and whetting an appetite for short papers, were shown to be more prone to AI-assisted writing. It is evident that this pattern throws light on the presumed relationship between time limitation and the increased amount of published content as the result of AI-generated content.
AI has been a key facilitator in speeding up research processes. However, it still raises issues of ethics when the technology is used in diverse tasks such as abstracts and other sections of scientific papers. Certain publishers consider it plagiarism, and to some extent unethical, if employed agents of LLMs discuss a scientific paper in which they are not the sole human authors.
The necessary nature of avoiding inaccuracies in AI-generated text, such as imagined quotations and examples, is yet a key feature of scholars’ communication, one should not fail to be transparent and honest. Authors who employ LLM-driven material are required to let the readers know about the research method they used to maintain research integrity and standard acts.
With AI’s increasing influence in academic writing, the architects of the academic community are confronted with the serious challenge of solving ethical implications and ensuring the reliability of research articles. AI is a great technology that significantly facilitates research activities, but honesty and integrity still ought to be maintained in order to preserve scientific integrity.
Learning from Ricardo and Thompson: Machinery and Labor in the Early Industrial Revolution, and in the Age of AI
David Ricardo initially believed machinery would help workers but revised his opinion, likely based on the impact of automation in the textile industry. Despite cotton textiles becoming one of the largest sectors in the British economy, real wages for cotton weavers did not rise for decades. As E.P. Thompson emphasized, automation forced workers into unhealthy factories with close surveillance and little autonomy. Automation can increase wages, but only when accompanied by new tasks that raise the marginal productivity of labor and/or when there is sufficient additional hiring in complementary sectors. Wages are unlikely to rise when workers cannot push for their share of productivity growth. Today, artificial intelligence may boost average productivity, but it also may replace many workers while degrading job quality for those who remain employed. As in Ricardo’s time, the impact of automation on workers today is more complex than an automatic linkage from higher productivity to better wages.
The authors are co-directors of the MIT Shaping the Future of Work Initiative, which was established through a generous gift from the Hewlett Foundation. Relevant disclosures are available at shapingwork.mit.edu/power-and-progress, under “Policy Summary.” For their outstanding work, we thank Gavin Alcott (research and drafting), Julia Regier (editing), and Hilary McClellen (fact-checking). We also thank Joel Mokyr for his helpful comments. The views expressed herein are those of the authors and do not necessarily reflect the views of the National Bureau of Economic Research.
We are grateful to David Autor for useful comments. We gratefully acknowledge financial support from Toulouse Network on Information Technology, Google, Microsoft, IBM, the Sloan Foundation and the Smith Richardson Foundation.
MARC RIS BibTeΧ
Download Citation Data
More from NBER
In addition to working papers , the NBER disseminates affiliates’ latest findings through a range of free periodicals — the NBER Reporter , the NBER Digest , the Bulletin on Retirement and Disability , the Bulletin on Health , and the Bulletin on Entrepreneurship — as well as online conference reports , video lectures , and interviews .
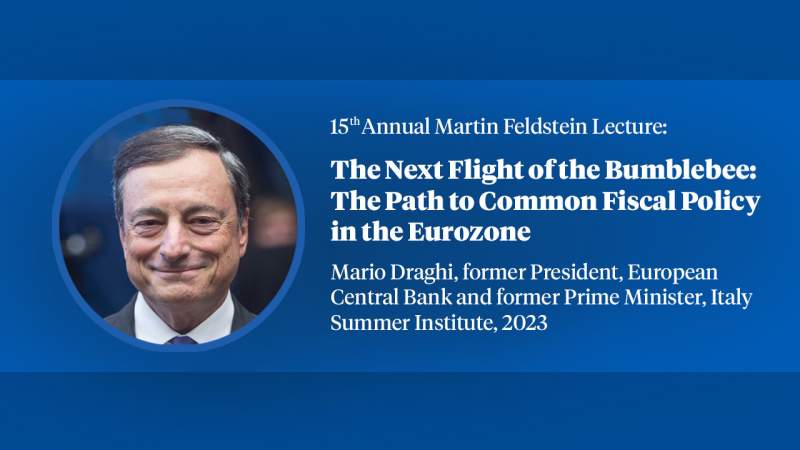
- Work & Careers
- Life & Arts
Become an FT subscriber
Try unlimited access Only $1 for 4 weeks
Then $75 per month. Complete digital access to quality FT journalism on any device. Cancel anytime during your trial.
- Global news & analysis
- Expert opinion
- Special features
- FirstFT newsletter
- Videos & Podcasts
- Android & iOS app
- FT Edit app
- 10 gift articles per month
Explore more offers.
Standard digital.
- FT Digital Edition
Premium Digital
Print + premium digital, weekend print + standard digital, weekend print + premium digital.
Essential digital access to quality FT journalism on any device. Pay a year upfront and save 20%.
- Global news & analysis
- Exclusive FT analysis
- FT App on Android & iOS
- FirstFT: the day's biggest stories
- 20+ curated newsletters
- Follow topics & set alerts with myFT
- FT Videos & Podcasts
- 20 monthly gift articles to share
- Lex: FT's flagship investment column
- 15+ Premium newsletters by leading experts
- FT Digital Edition: our digitised print edition
- Weekday Print Edition
- Videos & Podcasts
- Premium newsletters
- 10 additional gift articles per month
- FT Weekend Print delivery
- Everything in Standard Digital
- Everything in Premium Digital
Complete digital access to quality FT journalism with expert analysis from industry leaders. Pay a year upfront and save 20%.
- 10 monthly gift articles to share
- Everything in Print
Terms & Conditions apply
Explore our full range of subscriptions.
Why the ft.
See why over a million readers pay to read the Financial Times.
International Edition
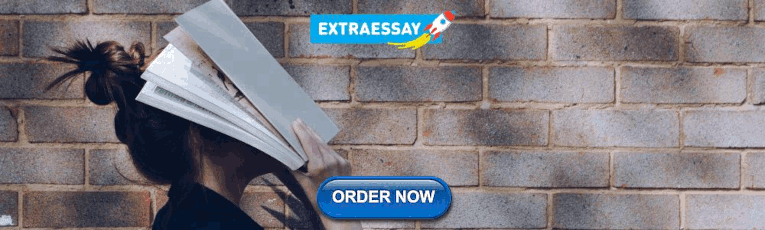
IMAGES
VIDEO
COMMENTS
Compared to both open-source and proprietary models, InternVL 1. 5 shows competitive performance, achieving state-of-the-art results in 8 of 18 benchmarks. Ranked #6 on Visual Question Answering on MM-Vet. Papers With Code highlights trending Machine Learning research and the code to implement it.
Semantic Reader is an augmented reader with the potential to revolutionize scientific reading by making it more accessible and richly contextual. Try it for select papers. Semantic Scholar uses groundbreaking AI and engineering to understand the semantics of scientific literature to help Scholars discover relevant research.
Subjects: Artificial Intelligence (cs.AI); Computer Vision and Pattern Recognition (cs.CV) [21] arXiv:2405.04015 [ pdf , ps , html , other ] Title: Certified Policy Verification and Synthesis for MDPs under Distributional Reach-avoidance Properties
The journal of Artificial Intelligence (AIJ) welcomes papers on broad aspects of AI that constitute advances in the overall field including, but not limited to, cognition and AI, automated reasoning and inference, case-based reasoning, commonsense reasoning, computer vision, constraint processing, ethical AI, heuristic search, human interfaces, intelligent robotics, knowledge representation ...
Artificial Intelligence | Explore the latest full-text research PDFs, articles, conference papers, preprints and more on ARTIFICIAL INTELLIGENCE. Find methods information, sources, references or ...
Artificial intelligence (AI) is being increasingly integrated into scientific discovery to augment and accelerate research, helping scientists to generate hypotheses, design experiments, collect ...
Pioneering research on the path to AGI. We believe our research will eventually lead to artificial general intelligence, a system that can solve human-level problems. Building safe and beneficial AGI is our mission. "Safely aligning powerful AI systems is one of the most important unsolved problems for our mission.
The Journal of Artificial Intelligence Research (JAIR) is dedicated to the rapid dissemination of important research results to the global artificial intelligence (AI) community. The journal's scope encompasses all areas of AI, including agents and multi-agent systems, automated reasoning, constraint processing and search, knowledge ...
Gemma Conroy, Hepeng Jia, Benjamin Plackett &. Andy Tay. As artificial intelligence (AI) becomes ubiquitous in fields such as medicine, education and security, there are significant ethical and ...
The number of AI and robotics papers published in the 82 high-quality science journals in the Nature Index (Count) has been rising year-on-year — so rapidly that it resembles an exponential ...
Generative Artificial Intelligence (AI) stands as a transformative paradigm in machine learning, enabling the creation of complex and realistic data from latent representations. This review paper comprehensively surveys the landscape of Generative AI, encompassing its foundational concepts, diverse models, training methodologies, applications, challenges, recent advancements, evaluation ...
Mar 8, 2021. 5. Each year scientists from around the world publish thousands of research papers in AI but only a few of them reach wide audiences and make a global impact in the world. Below are the top-10 most impactful research papers published in top AI conferences during the last 5 years. The ranking is based on the number of citations and ...
FEATURED RESEARCH. AI for the benefit of humanity. EXAMPLES OF OUR WORK. Improving skin tone evaluation in machine learning to uphold our AI principles. Discover Discover. Generative AI. ... Google publishes over 1,000 papers annually. Publishing our work enables us to collaborate and share ideas with, as well as learn from, the broader ...
AI was implemented and examined in a wide variety of subject areas, such as science, medicine, arts, sports, engineering, mathematics, technologies, foreign language, business, history and more (See Table 3).The largest number of AIEd research studies (n = 14) were in engineering, computer science, information technology (IT), or informatics, followed by mathematics (n = 8), foreign language ...
AI for scientific writing and research papers. In the ever-evolving realm of academic research, AI tools are increasingly taking center stage. Enter Paper Wizard, Jenny.AI, and Wisio - these groundbreaking platforms are set to revolutionize the way we approach scientific writing.
The Best of Applied Artificial Intelligence, Machine Learning, Automation, Bots, Chatbots. 2020's Top AI & Machine Learning Research Papers. November 24, 2020 by Mariya Yao. Despite the challenges of 2020, the AI research community produced a number of meaningful technical breakthroughs. GPT-3 by OpenAI may be the most famous, but there are ...
Are you an academic researcher seeking assistance in your quest to create remarkable research and scientific papers? Jenni AI is here to empower you, not by doing the work for you, but by enhancing your research process and efficiency. Explore how Jenni AI can elevate your academic writing experience and accelerate your journey toward academic ...
Clicking on the title of the citing paper takes you directly to the publication. ... Assistant is built with observability in mind to help you make more informed decisions about AI generated content. ... scite is a Brooklyn-based organization that helps researchers better discover and understand research articles through Smart Citations ...
Use AI to search, summarize, extract data from, and chat with over 125 million papers. Used by over 2 million researchers in academia and industry. ... Elicit uses language models to extract data from and summarize research papers. As a new technology, language models sometimes make up inaccurate answers. We improve accuracy by:
Artificial-intelligence (AI) tools are becoming increasingly common in science, and many scientists anticipate that they will soon be central to the practice of research, suggests a Nature survey ...
Excellence in a wide variety of medical applications poses considerable challenges for AI, requiring advanced reasoning, access to up-to-date medical knowledge and understanding of complex multimodal data. Gemini models, with strong general capabilities in multimodal and long-context reasoning, offer exciting possibilities in medicine. Building on these core strengths of Gemini, we introduce ...
ChatGPT for Research. Consensus is an AI-powered search engine that finds and summarizes scientific research papers. Just ask a question!
SciSpace is an incredible (AI-powered) tool to help you understand research papers better. It can explain and elaborate most academic texts in simple words. Mushtaq Bilal, PhD Researcher @ Syddansk Universitet. Loved by 1 million+ researchers from. Browse papers by years View all papers.
Two academic papers assert that analyzing word choice in the corpus of science publications reveals an increasing usage of AI for writing research papers. One study , published in March by Andrew Gray of University College London in the UK, suggests at least one percent - 60,000 or more - of all papers published in 2023 were written at ...
Top 10 AI Tools for Creating Research Papers. Here is the list of top 10 AI tools for creating research papers: QuillBot. QuillBot is an AI tool that can paraphrase sentences while the meaning remains the same. It employs advanced algorithms to generate alternative wordings, making it useful for writers seeking to enhance clarity or avoid ...
Tenorshare AI PDF Tool is a cutting-edge solution that harnesses the power of artificial intelligence to simplify the process of summarizing research papers. With its user-friendly interface and advanced AI algorithms, this tool quickly analyzes and condenses lengthy papers into concise, readable summaries, allowing researchers to grasp the ...
The data collected by Andrew Gray from University College London reveal that after 2023, just 1% of papers in certain fields are assisted by AI. Subsequently, in April, another study from Stanford ...
Today, artificial intelligence may boost average productivity, but it also may replace many workers while degrading job quality for those who remain employed. As in Ricardo's time, the impact of automation on workers today is more complex than an automatic linkage from higher productivity to better wages.
According to a Financial Times analysis of hundreds of LinkedIn profiles as well as public job postings and research papers, the $2.7tn company has undertaken a hiring spree over recent years to ...