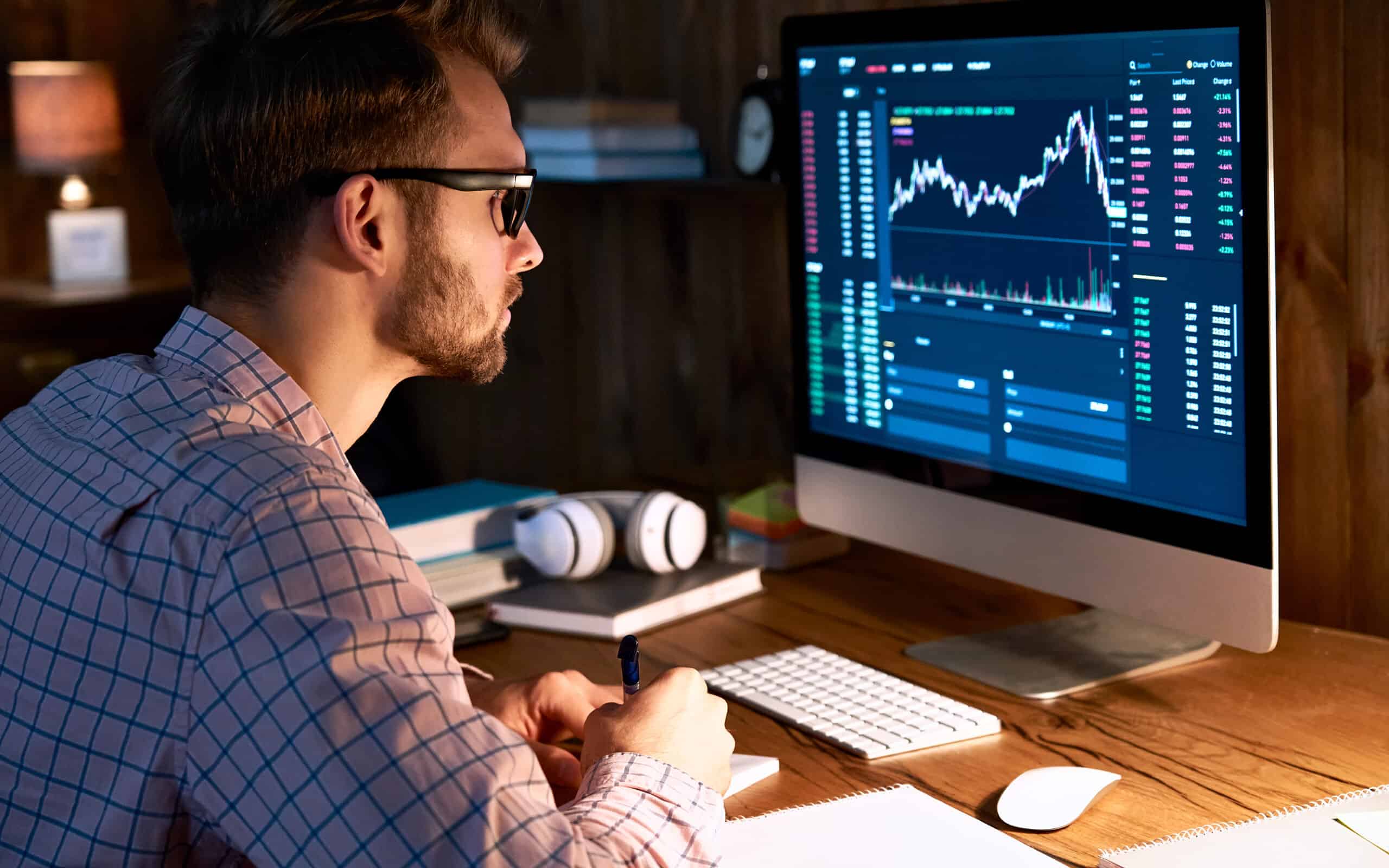
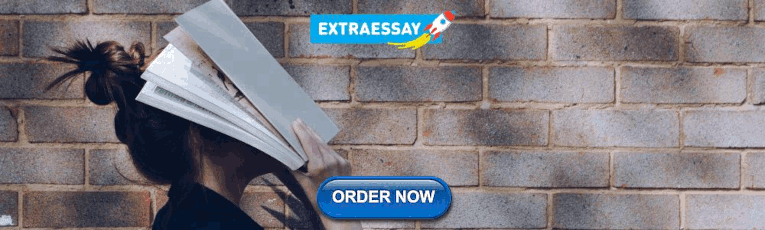
The Power of Special Cause Variation: Learning from Process Changes
Updated: July 28, 2023 by Marilyn Monda
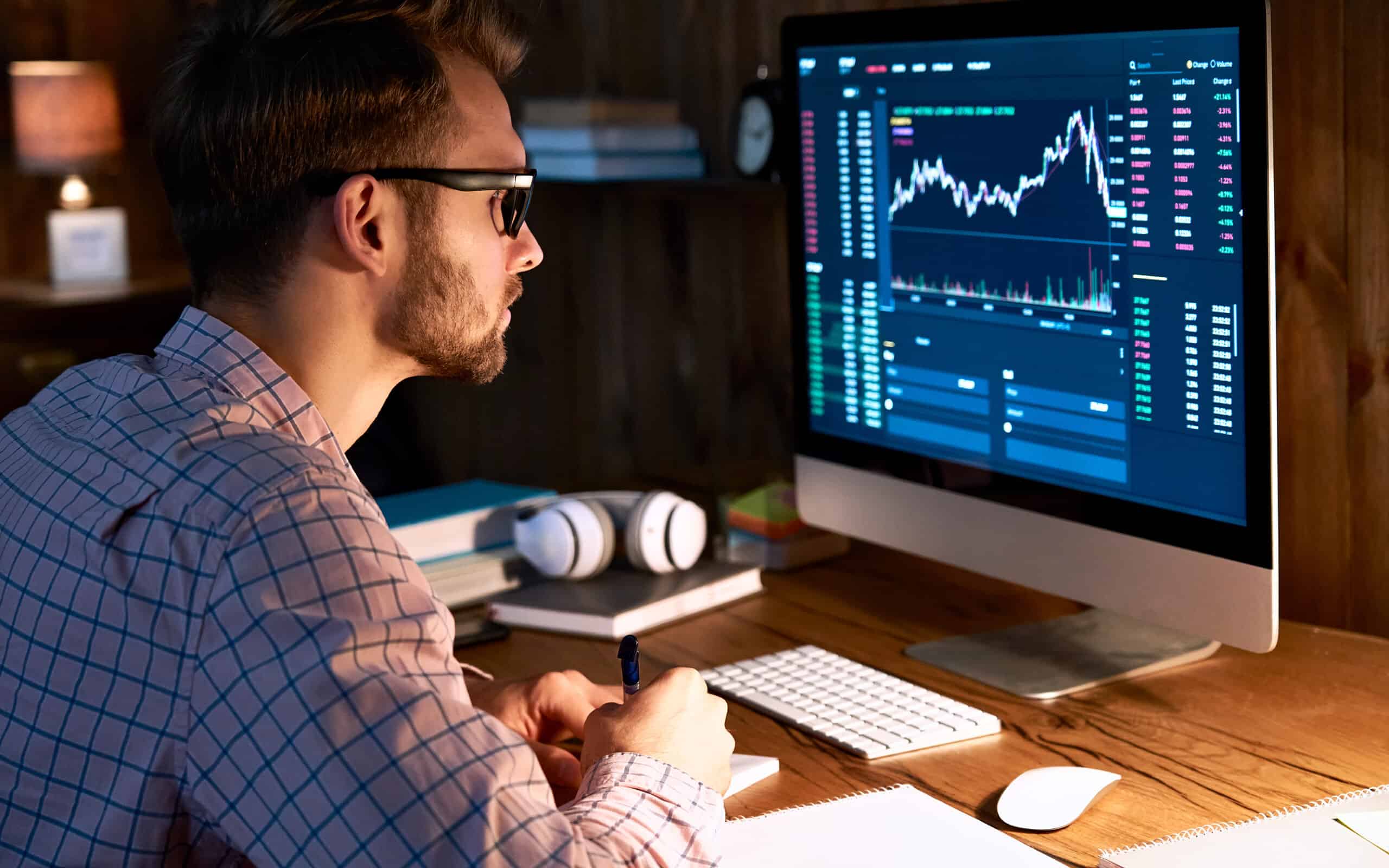
I love to see special cause variation! That’s because I know I’m about to learn something important about my process. A special cause is a signal that the process outcome is changing — and not always for the better.
Overview: What is special cause variation?
A control chart can show two different types of variation: common cause variation (random variation from the various process components) and special cause variation.
Special cause variation is present when the control chart of a process measure shows either plotted point(s) outside the control limits or a non-random pattern of variation.
When a control chart shows special cause variation, a process measure is said to be out-of-control or unstable. Common types of special cause variation signals include:
- A point outside of the upper control limit or lower control limit
- A trend: 6 or 7 points increasing or decreasing
- A cycle or repeating pattern
- A run: 8 or more points on either side of the average
A special cause of variation is assignable to a defect, fault, mistake, delay, breakdown, accident, and/or shortage in the process. When special causes are present, process quality is unpredictable.
Special causes are a signal for you to act to make the process improvements necessary to bring the process measure back into control.
RELATED: COMMON CAUSE VARIATION VS. SPECIAL CAUSE VARIATION
Drawbacks of special cause variation .
The source of a special cause can be difficult to find if you are not plotting the control chart in real time. Unless you have annotated data or a good memory, control charts made from historical data won’t aid your investigation into the source of the special cause.
If a process measure has never been charted, it is almost certain that it will be out of control. When you first start studying a process with a control chart, you will usually see a variety of special causes. To find the sources, begin a study of the status of critical process components.
When a special cause source cannot be found, it will become common to the process. As time goes on, the special causes repeat and cease being special. They then increase the natural or common cause variation in the process.
Why is special cause variation important to understand?
Let’s define quality as minimum variation around an appropriate target. The study of variation using a control chart is one way to tell if the process variation is increasing or if the center is moving away from the desired target over time.
A special cause is assignable to a process component that has changed or is changing. Investigation into the source of a special cause will:
- Let you know when to act to adjust or improve the process.
- Keep you from making the mistake of missing an opportunity to improve a process. If the ignored special cause repeats, you still don’t know how to fix it.
- Provide data to suggest or evaluate a process improvement.
If no special cause variation exists, that is, the process is in control, you should leave the process alone! Making process changes when there is no special cause present is called Tampering and can increase the variation of the process, lowering its quality.
An industry example of special cause variation
In this example, a control chart was used to monitor the number of data entry errors on job applications. Each day a sample of applications was reviewed. The number of errors found were plotted on a control chart.
One day, a point was plotted outside the control limit. Upon investigation, the manager noticed it occurred when a new worker started. It was found the worker wasn’t trained.
The newly trained worker continued data entry. A downward trend of errors followed, indicating the training was a source for the special cause!
The manager issued guidelines for new worker training. Since then, there have been three new workers without the error count spiking.
3 best practices when thinking about special cause variation
Special causes are signals that you need to act to move your process measure back into control.
Identify the source
When a special cause of variation exists, make a timely effort to identify its source. A good starting point is to check if any process component changed near to the time the special cause was seen. Also, you could ask process experts to brainstorm why the special cause samples were out of control.
For example, a trend up in screw thickness could be caused by a gage going out of calibration.
Make improvements at the source
Implement improvements to the source of special cause variation. Once you make improvements to the source of the special cause (like re-calibrating that gage), watch what happens as the next thickness samples are plotted. If the plot moves back toward stability, you know you found the issue!
Document everything
As you identify recurring special causes and their sources, document them on a control plan so process operators know what to do if they see the special cause again.
For our gage, the control plan could direct a worker to recalibrate the next time the screw thickness trends up, sending the process back to stability.
Frequently Asked Questions (FAQ) about special cause variation
- Are special causes always bad news?
No. A special cause can indicate either an increase or decrease in the quality of the process measure.
If the special cause shows increased process quality (for example, a decrease in cycle time), then you should make its source common to the process.
- If a process is in control (no special causes) is it also capable?
Not always. Control and capability are two different assessments. Your process measure can be stable (in control) and still not meet the customer specification (capable).
Once a process measure is in control, you can then assess its capability against the customer target and specification limits. If the data is within customer limits and on target, the process is considered both in control and capable.
Final thoughts on special causes
Every process measure will show variation, you will never attain zero variability. Still, it is important to understand the nature of variability so that you can use it to better improve and control your process outcomes.
The special cause variation signal is the key to finding those critical process components that are the sources of variation needing improvement. Use special cause variation to unlock the path to process control.
About the Author
Marilyn Monda
Table of Contents
Types of variance, common cause variation, common cause variation examples, special cause variation, special cause variation example, choose the right program, common cause variation vs. special cause variation.
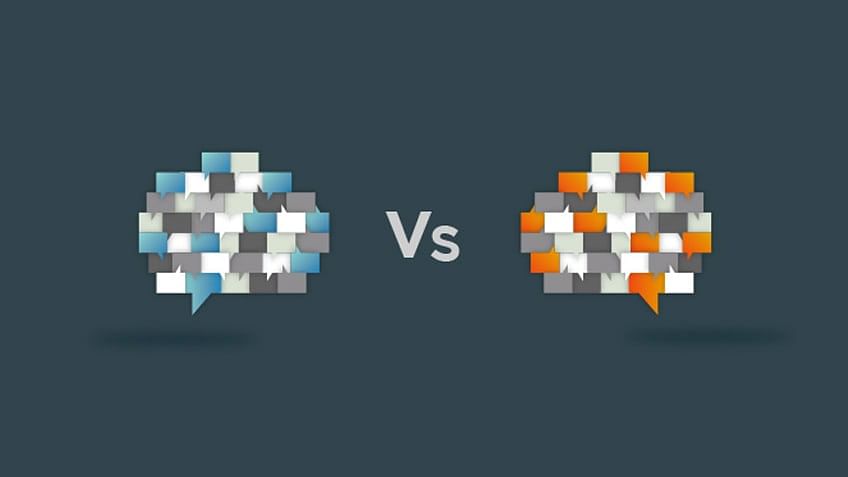
Every piece of data which is measured will show some degree of variation: no matter how much we try, we could never attain identical results for two different situations - each result will be different, even if the difference is slight. Variation may be defined as “the numerical value used to indicate how widely individuals in a group vary.”
In other words, variance gives us an idea of how data is distributed about an expected value or the mean. If you attain a variance of zero, it indicates that your results are identical - an uncommon condition. A high variance shows that the data points are spread out from each other—and the mean, while a smaller variation indicates that the data points are closer to the mean. Variance is always nonnegative.
Are you looking forward to making a mark in the Project Management field? If yes, enroll in the PMP Certification Program now and get a step closer to your career goal!
Change is inevitable, even in statistics. You’ll need to know what kind of variation affects your process because the course of action you take will depend on the type of variance. There are two types of Variance: Common Cause Variation and Special Cause Variation. You’ll need to know about Common Causes Variation vs Special Causes Variation because they are two subjects that are tested on the PMP Certification and CAPM Certification exams.
Common Cause Variation, also referred to as “Natural Problems, “Noise,” and “Random Cause” was a term coined by Harry Alpert in 1947. Common causes of variance are the usual quantifiable and historical variations in a system that are natural. Though variance is a problem, it is an inherent part of a process—variance will eventually creep in, and it is not much you can do about it. Specific actions cannot be taken to prevent this failure from occurring. It is ongoing, consistent, and predictable.
Characteristics of common causes variation are:
- Variation predictable probabilistically
- Phenomena that are active within the system
- Variation within a historical experience base which is not regular
- Lack of significance in individual high and low values
This variation usually lies within three standard deviations from the mean where 99.73% of values are expected to be found. On a control chart, they are indicated by a few random points that are within the control limit. These kinds of variations will require management action since there can be no immediate process to rectify it. You will have to make a fundamental change to reduce the number of common causes of variation. If there are only common causes of variation on your chart, your process is said to be “statistically stable.”
When this term is applied to your chart, the chart itself becomes fairly stable. Your project will have no major changes, and you will be able to continue process execution hassle-free.
Become a Data Science & Business Analytics Professional
- 28% Annual Job Growth By 2026
- 11.5 M Expected New Jobs For Data Science By 2026
Data Scientist
- Industry-recognized Data Scientist Master’s certificate from Simplilearn
- Dedicated live sessions by faculty of industry experts
Big Data Engineer
- Live interaction with IBM leadership
- 8X higher live interaction in live online classes by industry experts
Here's what learners are saying regarding our programs:
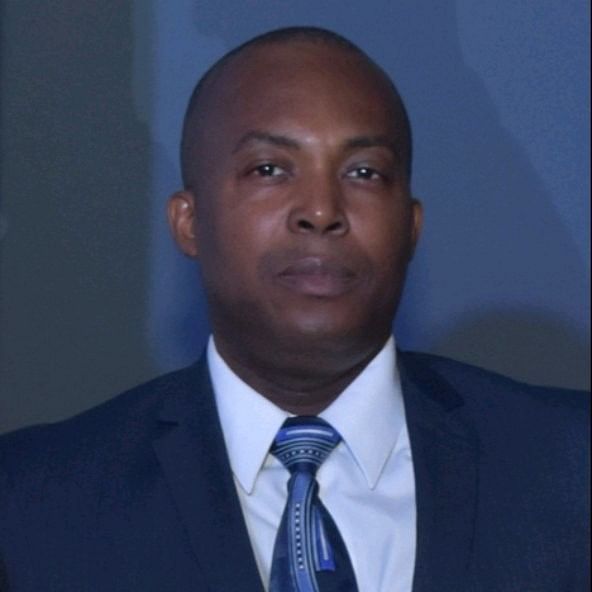
A.Anthony Davis
Simplilearn has one of the best programs available online to earn real-world skills that are in demand worldwide. I just completed the Machine Learning Advanced course, and the LMS was excellent.
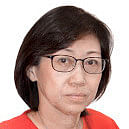
I encountered problems when trying to solve the projects in my Data Science class for Python. The Teaching Assistant was accommodating and provided step-by-step guidance through web chat sessions to resolve the issues.
Consider an employee who takes a little longer than usual to complete a specific task. He is given two days to do a task, and instead, he takes two and a half days; this is considered a common cause variation. His completion time would not have deviated very much from the mean since you would have had to consider the fact that he could submit it a little late.
Here’s another example: you estimate 20 minutes to get ready and ten minutes to get to work. Instead, you take five minutes extra to get ready because you had to pack lunch and 15 additional minutes to get to work because of traffic.
Other examples that relate to projects are inappropriate procedures, which can include the lack of clearly defined standard procedures, poor working conditions, measurement errors, normal wear and tear, computer response times, etc. These are all common cause variation.
Special Cause Variation, on the other hand, refers to unexpected glitches that affect a process. The term Special Cause Variation was coined by W. Edwards Deming and is also known as an “Assignable Cause.” These are variations that were not observed previously and are unusual, non-quantifiable variations.
These causes are sporadic, and they are a result of a specific change that is brought about in a process resulting in a chaotic problem. It is not usually part of your normal process and occurs out of the blue. Causes are usually related to some defect in the system or method. However, this failure can be corrected by making changes to affected methods, components, or processes.
Characteristics of special cause variation are:
- New and unanticipated or previously neglected episode within the system
- This kind of variation is usually unpredictable and even problematic
- The variation has never happened before and is thus outside the historical experience base
On a control chart, the points lie beyond the preferred control limit or even as random points within the control limit. Once identified on a chart, this type of problem needs to be found and addressed immediately you can help prevent it from recurring.
Let’s say you are driving to work, and you estimate arrival in 10 minutes every day. One day, it took you 20 minutes to arrive at work because you were caught in the traffic from an accident zone and were held up.
Examples relating to project management are if machine malfunctions, computer crashes, there is a power cut, etc. These kinds of random things that can happen during a project are examples of special cause variation.
One way to evaluate a project’s health is to track the difference between the original project plan and what is happening. The use of control charts helps to differentiate between the common cause variation and the special cause variation, making the process of making changes and amends easier.
Learn new trends, emerging practices, tailoring considerations, and core competencies required of a Project Management professional with the PMP Certification course .
Unlock your project management potential with Simplilearn's comprehensive training. Gain the skills and knowledge needed to lead successful projects, boost efficiency, and exceed goals. Choose the right project management course today and advance your career with confidence.
Program Name PMP® Certification Training Course PMP Plus Post Graduate Program In Project Management Geo All Geos All Geos All Geos University PMI Simplilearn University of Massachusetts Amherst Course Duration 90 Days of Flexible Access to Online Classes 36 Months 6 Months Coding experience reqd No No No Skills you wll learn 8+ PM skills including Work Breakdown Structure, Gantt Charts, Resource Allocation, Leadership and more. 6 courses including Project Management, Agile Scrum Master, Implementing a PMO, and More 9+ skills including Project Management, Quality Management, Agile Management, Design Thinking and More. Additional Benefits Experiential learning through case studies Global Teaching Assistance 35PDUs Learn by working on real-world problems 24x7 Learning support from mentors Earn 60+ PDU’s 3 year course access Cost $$ $$$$ $$$$ Explore Program Explore Program Explore Program
This article has explained special cause variation vs common cause variation which are the two important concepts in project management when it comes to data validation. Simplilearn offers multiple Project Management training courses like the Post Graduate Program in Project Management and learning paths that can help aspiring project managers get the education they need to pass not only exams like the PMP certification and CAPM® but also real-world knowledge useful for any project management career.
PMP is a registered mark of the Project Management Institute, Inc.
Our Project Management Courses Duration And Fees
Project Management Courses typically range from a few weeks to several months, with fees varying based on program and institution.
Get Free Certifications with free video courses
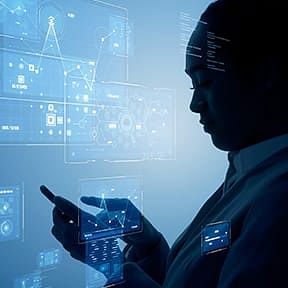
Data Science & Business Analytics
Introduction to Data Science
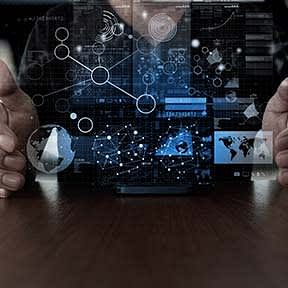
Introduction to Big Data Tools for Beginners
Learn from Industry Experts with free Masterclasses
Data Scientist vs Data Analyst: Breaking Down the Roles
Learner Spotlight: Watch How Prasann Upskilled in Data Science and Transformed His Career
Open Gates to a Successful Data Scientist Career in 2024 with Simplilearn Masters program
Recommended Reads
Four Proven Reasons Why Gamification Improves Employee Training
10 Major Causes of Project Failure
Data Analyst vs. Data Scientist: The Ultimate Comparison
Free eBook: Top 25 Interview Questions and Answers: Big Data Analytics
Root Cause Analysis: All You Need to Know
A Comprehensive Comparison of NFT Vs. Crypto
Get Affiliated Certifications with Live Class programs
- PMP, PMI, PMBOK, CAPM, PgMP, PfMP, ACP, PBA, RMP, SP, and OPM3 are registered marks of the Project Management Institute, Inc.
Lean Six Sigma Training Certification

- Facebook Instagram Twitter LinkedIn YouTube
- (877) 497-4462
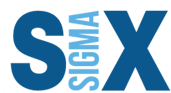
What is Special Cause Variation? How to Identify It?
March 8th, 2024
Variations are inherent in any process and the sources of these variations play a crucial role in determining process performance and stability. While common cause variations are predictable and result from the process design itself, special cause variation arise due to unexpected factors and lead to process instability.
Managing these special cause variations by identifying and eliminating their root causes is an integral part of quality management frameworks like Six Sigma and Lean. By doing so, processes can achieve stability and enhanced capability.
This enables improved competitiveness through cost reduction, lead time reduction, improved quality, and higher customer satisfaction.
Thus, organizations invest significant efforts in detecting and managing special causes of variation in their business processes.
Understanding Special Cause Variation
Special cause variation refers to fluctuations in a process that happen because of unique or unusual factors that are not normally present.
These unnatural variations are unpredictable and assignable to a specific cause. They indicate that the process has become unstable and is producing defects or undesirable outcomes.
Difference from Common Cause Variation
Common cause variation refers to natural or expected variability that exists in any process. This type of variation happens due to minor, ubiquitous causes that are inherent in the process design.
Common causes lead to random scattering of data points around the mean. On the other hand, special causes produce data points that stand out from the normal pattern of variation and signal that the process is out of control.
While common cause variation indicates a stable process, special cause variation implies instability requiring investigation and corrective action.
Importance in Quality Management
Effective management of special cause variation is crucial for organizations looking to enhance their quality management programs.
By properly identifying and addressing special causes, companies can boost competitiveness, better meet customer expectations, and positively impact financials.
Enhancing Competitiveness with Special Cause Variation
In today’s business landscape, quality has become a key competitive differentiator across industries. Companies that demonstrate consistent product/service quality and continuous improvement initiatives can gain an edge.
The ability to rapidly detect and mitigate special causes of variation enables organizations to enhance process stability .
This prevents the occurrence of unexpected defects and non-conformances which can erode quality perception. Maintaining robust quality standards is essential to stay ahead.
Connecting with Quality-Focused Customers
Customers are increasingly assessing providers based on their quality management record and commitment to zero defects.
By tackling special cause variations through root cause analysis , corrective actions, and control mechanisms. etc. companies can limit unexpected issues that lead to customer dissatisfaction.
This accountability and proactive quality management culture aligns with customer requirements and values.
Communicating such initiatives and results also reassures customers regarding consistency. This helps strengthen loyalty and presents partnership opportunities with quality-focused customers.
Identifying Special Cause Variation
Special cause variation is often difficult to detect without the right analysis tools. Identifying special causes requires going beyond typical process monitoring to specialized statistical techniques.
There are three main methods for recognizing when variation is due to special causes:
Control Charts
Control charts are the most common way to identify special cause variation. Control charts plot data over time with statistically derived upper and lower control limits.
When data points fall outside these control limits , it indicates something unexpected has happened to the process.
This unexpected variance points to special causes rather than expected normal variation.
Common control charts used to monitor special causes include Xbar-R charts , Xbar-S charts , Individual charts, moving range charts, and P charts.
Statistical process control software makes it easy to generate control charts and receive alerts when special causes occur.
Statistical Process Control
Statistical process control (SPC) is an analytical methodology that makes extensive use of control charts.
SPC carefully monitors processes to distinguish between expected and unexpected variations using statistical methods.
By visually separating normal and special cause variations on control charts, SPC provides objective evidence that can pinpoint the timing and magnitude of special causes.
SPC also calculates metrics like Cp and Cpk that quantify overall process variation and process capability.
Tracking these metrics provides further insight into process stability versus areas with heightened special cause variation.
Root Cause Analysis
When control charts or SPC identify special cause variation, the next step is to determine the root cause. Root cause analysis gets to the underlying reason why the unexpected process change happened.
Various tools like the 5 Whys , fishbone diagrams , and failure mode analysis help uncover root causes. Tracing special cause variation back to the root cause is crucial for addressing problems permanently.
Otherwise, the same special cause could keep affecting the process unpredictably.
Implications of Special Cause Variation
Special cause variation can have major implications if left unchecked.
Understanding and properly dealing with special causes is crucial for organizations that want to achieve process stability, improve customer satisfaction, and boost financial performance.
Process Stability with Special Cause Variation
The presence of special cause variation indicates an unstable process. The output and performance metrics of an unstable process fluctuate unpredictably.
This makes the process unreliable and difficult to control. Identifying and eliminating special causes is the first step toward stabilizing a process.
Stable processes have consistent output and are predictable, easier to monitor, and simpler to improve.
Customer Satisfaction
Customers expect consistent quality and on-time delivery of products and services. An unstable process leads to unpredictable product quality and delivery delays which frustrates customers. Fixing special causes improves process stability and capability.
This enables organizations to reliably meet customer expectations and increase satisfaction levels. Satisfied customers lead to repeat purchases and valuable word-of-mouth publicity.
Financial Performance
Unstable processes lead to increased waste, rework, and returns. The additional effort and resources needed to fix these issues drive up costs and hurt profitability.
Special cause variation can also result in production and shipment delays that mean missed revenue opportunities and penalties.
Eliminating special causes reduces costs and improves process efficiency . This directly translates into increased profit margins, higher ROI, and stronger financial performance.
Strategies for Management of Special Cause Variation
Effectively managing special cause variation is critical for organizations looking to improve quality, reduce costs, and boost customer satisfaction.
When a process experiences special cause variation, it indicates an unexpected change that needs to be addressed. Implementing targeted strategies can help get processes back into a state of statistical control.
Corrective Actions
- Once a special cause is identified through statistical process control methods, structured problem-solving methodologies like PDCA (Plan-Do-Check-Act) or DMAIC (Define, Measure, Analyze, Improve, Control) can be utilized to develop corrective actions.
- Brainstorming sessions to determine potential causes and solutions can provide useful insights from team members. Fishbone diagrams are an effective tool to visually map out causes.
- Pilot testing proposed improvements on a small scale first to evaluate potential impact.
- Verify the effectiveness of corrective actions by monitoring the process over time using control charts. Special causes should be removed and variation should return to baseline common cause levels.
Continual Improvement with Special Cause Variation
- Schedule periodic reviews of processes, even in the absence of special causes, to identify opportunities for incremental improvements.
- Utilize lean tools like 5S , poka-yoke , and process mapping to reduce waste and enhance process stability.
- Keep the workforce engaged by encouraging them to share improvement ideas and recognize implementation efforts.
- Consider automating certain process steps to reduce variability induced by human errors.
- Review process performance metrics regularly to ensure improvements are sustained over longer periods.
- Conduct refresher training for employees on methods like statistical process control, Six Sigma, and hypothesis testing.
By taking a structured approach combining short-term corrective actions and long-term continual improvement , organizations can effectively manage special cause variation events.
This drives greater consistency and stability in processes, directly enhancing product quality and customer satisfaction.
SixSigma.us offers both Live Virtual classes as well as Online Self-Paced training. Most option includes access to the same great Master Black Belt instructors that teach our World Class in-person sessions. Sign-up today!
Virtual Classroom Training Programs Self-Paced Online Training Programs
SixSigma.us Accreditation & Affiliations
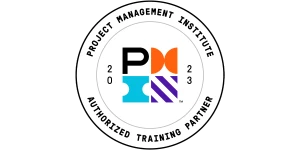
Monthly Management Tips
- Be the first one to receive the latest updates and information from 6Sigma
- Get curated resources from industry-experts
- Gain an edge with complete guides and other exclusive materials
- Become a part of one of the largest Six Sigma community
- Unlock your path to become a Six Sigma professional
" * " indicates required fields
Volume 8 Supplement 1
Proceedings of Advancing the Methods in Health Quality Improvement Research 2012 Conference
- Proceedings
- Open access
- Published: 19 April 2013
Understanding and managing variation: three different perspectives
- Michael E Bowen 1 , 2 , 3 &
- Duncan Neuhauser 4
Implementation Science volume 8 , Article number: S1 ( 2013 ) Cite this article
28k Accesses
4 Citations
13 Altmetric
Metrics details
Presentation
Managing variation is essential to quality improvement. Quality improvement is primarily concerned with two types of variation – common-cause variation and special-cause variation. Common-cause variation is random variation present in stable healthcare processes. Special-cause variation is an unpredictable deviation resulting from a cause that is not an intrinsic part of a process. By careful and systematic measurement, it is easier to detect changes that are not random variation.
The approach to managing variation depends on the priorities and perspectives of the improvement leader and the intended generalizability of the results of the improvement effort. Clinical researchers, healthcare managers, and individual patients each have different goals, time horizons, and methodological approaches to managing variation; however, in all cases, the research question should drive study design, data collection, and evaluation. To advance the field of quality improvement, greater understanding of these perspectives and methodologies is needed [ 1 ].
Clinical researcher perspective
The primary goal of traditional randomized controlled trials (RCTs) (ie a comparison of treatment A versus placebo) is to determine treatment or intervention efficacy in a specified population when all else is equal. In this approach, researchers seek to maximize internal validity. Through randomization, researchers seek to balance variation in baseline factors by randomizing patients, clinicians, or organizations to experimental and control groups. Researchers may also increase understanding of variation within a specific study using approaches such as stratification to examine for effect modification. Although the generalizability of outcomes in all research designs is limited by the study population and setting, this can be particularly challenging in traditional RCTs. When inclusion criteria are strict, study populations are not representative of “real world” patients, and the applicability of study findings to clinical practice may be unclear. Traditional RCTs are limited in their ability to evaluate complex processes that are purposefully and continually changing over time because they evaluate interventions in rigorously controlled conditions over fixed time frames [ 2 ]. However, using alternative designs such as hybrid, effectiveness studies discussed in these proceedings or pragmatic RCTs, researchers can rigorously answer a broader range of research questions [ 3 ].
Healthcare manager perspective
Healthcare managers seek to understand and reduce variation in patient populations by monitoring process and outcome measures. They utilize real-time data to learn from and manage variation over time. By comparing past, present, and desired performance, they seek to reduce undesired variation and reinforce desired variation. Additionally, managers often implement best practices and benchmark performance against them. In this process, efficient, time-sensitive evaluations are important. Run charts and Statistical Process Control (SPC) methods leverage the power of repeated measures over time to detect small changes in process stability and increase the statistical power and rapidity with which effects can be detected [ 1 ].
Patient perspective
While the clinical researcher and healthcare manager are interested in understanding and managing variation at a population level, the individual patient wants to know if a particular treatment will allow one to achieve health outcomes similar to those observed in study populations. Although the findings of RCTs help form the foundation of evidence-based practice and managers utilize these findings in population management, they provide less guidance about the likelihood of an individual patient achieving the average benefits observed across a population of patients. Even when RCT findings are statistically significant, many trial participants receive no benefit. In order to understand if group RCT results can be achieved with individual patients, a different methodological approach is needed. “N-of-1 trials” and the longitudinal factorial design of experiments allow patients and providers to systematically evaluate the independent and combined effects of multiple disease management variables on individual health outcomes [ 4 ]. This offers patients and providers the opportunity to collect, analyze, and understand data in real time to improve individual patient outcomes.
Advancing the field of improvement science and increasing our ability to understand and manage variation requires an appreciation of the complementary perspectives held and methodologies utilized by clinical researchers, healthcare managers, and patients. To accomplish this, clinical researchers, healthcare managers, and individual patients each face key challenges.
Recommendations
Clinical researchers are challenged to design studies that yield generalizable outcomes across studies and over time. One potential approach is to anchor research questions in theoretical frameworks to better understand the research problem and relationships among key variables. Additionally, researchers should expand methodological and analytical approaches to leverage the statistical power of multiple observations collected over time. SPC is one such approach. Incorporation of qualitative research and mixed methods can also increase our ability to understand context and the key determinants of variation.
Healthcare managers are challenged to identify best practices and benchmark their processes against them. However, the details of best practices and implementation strategies are rarely described in sufficient detail to allow identification of the key drivers of process improvement and adaption of best practices to local context. By advocating for transparency in process improvement and urging publication of improvement and implementation efforts, healthcare managers can enhance the spread of best practices, facilitate improved benchmarking, and drive continuous healthcare improvement.
Individual patients and providers are challenged to develop the skills needed to understand and manage individual processes and outcomes. As an example, patients with hypertension are often advised to take and titrate medications, modify dietary intake, and increase activity levels in a non-systematic manner. The longitudinal factorial design offers an opportunity to rigorously evaluate the impact of these recommendations, both in isolation and in combination, on disease outcomes [ 1 ]. Patients can utilize paper, smart phone applications, or even electronic health record portals to sequentially record their blood pressures. Patients and providers can then apply simple SPC rules to better understand variation in blood pressure readings and manage their disease [ 5 ].
As clinical researchers, healthcare managers, and individual patients strive to improve healthcare processes and outcomes, each stakeholder brings a different perspective and set of methodological tools to the improvement team. These perspectives and methods are often complementary such that it is not which methodological approach is “best” but rather which approach is best suited to answer the specific research question. By combining these perspectives and developing partnerships with organizational managers, improvement leaders can demonstrate process improvement to key decision makers in the healthcare organization. It is through such partnerships that the future of quality improvement research is likely to find financial support and ultimate sustainability.
Neuhauser D, Provost L, Bergman B: The meaning of variation to healthcare managers, clinical and health-services researchers, and individual patients. BMJ Qual Saf. 2011, 20 (Suppl 1): i36-40. 10.1136/bmjqs.2010.046334.
Article PubMed Central PubMed Google Scholar
Neuhauser D, Diaz M: Quality improvement research: are randomised trials necessary?. Qual Saf Health Care. 2007, 16: 77-80. 10.1136/qshc.2006.021584.
Article PubMed Central CAS PubMed Google Scholar
Eccles M, Grimshaw J, Campbell M, Ramsay C: Research designs for studies evaluating the effectiveness of change and improvement strategies. Quality and Safety in Health Care. 2003, 12: 47-52. 10.1136/qhc.12.1.47.
Olsson J, Terris D, Elg M, Lundberg J, Lindblad S: The one-person randomized controlled trial. Qual Manag Health Care. 2005, 14: 206-216.
Article PubMed Google Scholar
Hebert C, Neuhauser D: Improving hypertension care with patient-generated run charts: physician, patient, and management perspectives. Qual Manag Health Care. 2004, 13: 174-177.
Download references
Author information
Authors and affiliations.
VA National Quality Scholars Fellowship, Tennessee Valley Healthcare System, Nashville, Tennessee, 37212, USA
Michael E Bowen
Division of General Internal Medicine, Department of Medicine, University of Texas Southwestern Medical Center, Dallas, Texas, 75390, USA
Division of Outcomes and Health Services Research, Department of Clinical Sciences, University of Texas Southwestern Medical Center, Dallas, Texas, 75390, USA
Department of Epidemiology and Biostatistics, Case Western Reserve University, Cleveland, Ohio, 44106, USA
Duncan Neuhauser
You can also search for this author in PubMed Google Scholar
Corresponding author
Correspondence to Michael E Bowen .
Rights and permissions
This article is published under license to BioMed Central Ltd. This is an Open Access article distributed under the terms of the Creative Commons Attribution License ( http://creativecommons.org/licenses/by/2.0 ), which permits unrestricted use, distribution, and reproduction in any medium, provided the original work is properly cited.
Reprints and permissions
About this article
Cite this article.
Bowen, M.E., Neuhauser, D. Understanding and managing variation: three different perspectives. Implementation Sci 8 (Suppl 1), S1 (2013). https://doi.org/10.1186/1748-5908-8-S1-S1
Download citation
Published : 19 April 2013
DOI : https://doi.org/10.1186/1748-5908-8-S1-S1
Share this article
Anyone you share the following link with will be able to read this content:
Sorry, a shareable link is not currently available for this article.
Provided by the Springer Nature SharedIt content-sharing initiative
- Statistical Process Control
- Clinical Researcher
- Healthcare Manager
- Healthcare Process
- Quality Improvement Research
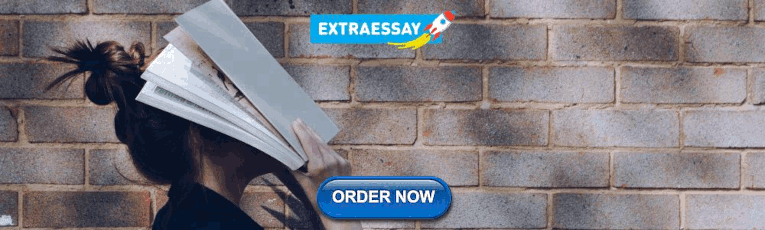
Implementation Science
ISSN: 1748-5908
- Submission enquiries: Access here and click Contact Us
- General enquiries: [email protected]
Log in using your username and password
- Search More Search for this keyword Advanced search
- Latest content
- Current issue
- BMJ Journals More You are viewing from: Google Indexer
You are here
- Volume 20, Issue Suppl 1
- The meaning of variation to healthcare managers, clinical and health-services researchers, and individual patients
- Article Text
- Article info
- Citation Tools
- Rapid Responses
- Article metrics

- Duncan Neuhauser 1 ,
- Lloyd Provost 2 ,
- Bo Bergman 3
- 1 Department of Epidemiology and Biostatistics, Case Western Reserve University, Cleveland, Ohio, USA
- 2 Associates in Process Improvement, Austin, Texas, USA
- 3 Centre for Health Improvement, Chalmers University of Technology, Gothenburg, Sweden
- Correspondence to Charles Elton Blanchard Professor Duncan Neuhauser, Department of Epidemiology and Biostatistics, Medical School, Case Western Reserve University, 10900 Euclid Ave, Cleveland Ohio 44106-4249, USA; dvn{at}case.edu
Healthcare managers, clinical researchers and individual patients (and their physicians) manage variation differently to achieve different ends. First, managers are primarily concerned with the performance of care processes over time. Their time horizon is relatively short, and the improvements they are concerned with are pragmatic and ‘holistic.’ Their goal is to create processes that are stable and effective. The analytical techniques of statistical process control effectively reflect these concerns. Second, clinical and health-services researchers are interested in the effectiveness of care and the generalisability of findings. They seek to control variation by their study design methods. Their primary question is: ‘Does A cause B, everything else being equal?’ Consequently, randomised controlled trials and regression models are the research methods of choice. The focus of this reductionist approach is on the ‘average patient’ in the group being observed rather than the individual patient working with the individual care provider. Third, individual patients are primarily concerned with the nature and quality of their own care and clinical outcomes. They and their care providers are not primarily seeking to generalise beyond the unique individual. We propose that the gold standard for helping individual patients with chronic conditions should be longitudinal factorial design of trials with individual patients. Understanding how these three groups deal differently with variation can help appreciate these three approaches.
- Control charts
- evidence-based medicine
- quality of care
- statistical process control
This is an open-access article distributed under the terms of the Creative Commons Attribution Non-commercial License, which permits use, distribution, and reproduction in any medium, provided the original work is properly cited, the use is non commercial and is otherwise in compliance with the license. See: http://creativecommons.org/licenses/by-nc/2.0/ and http://creativecommons.org/licenses/by-nc/2.0/legalcode .
https://doi.org/10.1136/bmjqs.2010.046334
Statistics from Altmetric.com
Request permissions.
If you wish to reuse any or all of this article please use the link below which will take you to the Copyright Clearance Center’s RightsLink service. You will be able to get a quick price and instant permission to reuse the content in many different ways.
Introduction
Health managers, clinical researchers, and individual patients need to understand and manage variation in healthcare processes in different time frames and in different ways. In short, they ask different questions about why and how healthcare processes and outcomes change ( table 1 ). Confusing the needs of these three stakeholders results in misunderstanding.
- View inline
Meaning of variation to managers, researchers and individual patients: questions, methods and time frames
Health managers
Our extensive experience in working with healthcare managers has taught us that their primary goal is to maintain and improve the quality of care processes and outcomes for groups of patients. Ongoing care and its improvement are temporal, so in their situation, learning from variation over time is essential. Data are organised over time to answer the fundamental management question: is care today as good as or better than it was in the past, and how likely is it to be better tomorrow? In answering that question, it becomes crucial to understand the difference between common-cause and special-cause variation (as will be discussed later). Common-cause variation appears as random variation in all measures from healthcare processes. 1 Special-cause variation appears as the effect of causes outside the core processes of the work. Management can reduce this variation by enabling the easy recognition of special-cause variation and by changing healthcare processes—by supporting the use of clinical practice guidelines, for example—but common-cause variation can never be eliminated.
The magnitude of common-cause variation creates the upper and lower control limits in Shewhart control charts. 2–5 Such charts summarise the work of health managers well. Figure 1 shows a Shewhart control chart (p-chart) developed by a quality-improvement team whose aim was to increase compliance with a new care protocol. The clinical records of eligible patients discharged (45–75 patients) were evaluated each week by the team, and records indicating that the complete protocol was followed were identified. The baseline control chart showed a stable process with a centre line (average performance) of 38% compliance. The team analysed the aspects of the protocol that were not followed and developed process changes to make it easier to complete these particular tasks. After successfully adapting the changes to the local environment (indicated by weekly points above the upper control limit in the ‘Implementing Changes’ period), the team formally implemented the changes in each unit. The team continued to monitor the process and eventually developed updated limits for the chart. The updated chart indicated a stable process averaging 83%.
- Download figure
- Open in new tab
- Download powerpoint
Annotated Shewhart control chart—using protocol.
This control chart makes it clear that a stable but inferior process was operating for the first 11 weeks and, by inference, probably before that. The annotated changes (testing, adapting and implementing new processes of care) are linked to designed tests of change which are special (assignable) causes of variation, in this case, to improvement after week 15, after which a new better stable process has taken hold. Note that there is common-cause (random) variation in both the old and improved processes.
After updating the control limits, the chart reveals a new stable process with no special-cause variation, which is to say, no points above or below the control limits (the dotted lines). Note that the change after week 15 cannot easily be explained by chance (random, or common-cause, variation), since the probability of 13 points in a row occurring by chance above the baseline control limit is one divided by 2 to the 13th power. This is the same likelihood that in flipping a coin 13 times, it will come up heads every time. This level of statistical power to exclude randomness as an explanation is not to be found in randomised controlled trials (RCTs). Although there is no hard-and-fast rule about the number of observations over time needed to demonstrate process stability and establish change, we believe a persuasive control chart requires 20–30 or more observations.
The manager's task demonstrates several important characteristics. First is the need to define the key quality characteristics, and choose among them for focused improvement efforts. The choice should be made based on the needs of patients and families. The importance of these quality characteristics to those being served means that speed in learning and improvement is important. Indeed, for the healthcare manager, information for improvement must be as rapid as possible (in real time). Year-old research data are not very helpful here; just-in-time performance data in the hands of the decision-makers provide a potent opportunity for rapid improvement. 6
Second, managerial change is holistic; that is, every element of an intervention that might help to improve and can be done is put to use, sometimes incrementally, but simultaneously if need be. Healthcare managers are actively working to promote measurement of process and clinical outcomes, take problems in organisational performance seriously, consider the root causes of those problems, encourage the formation of problem solving clinical micro-system teams and promote the use of multiple, evolving Plan–Do–Study–Act (PDSA) tests of change.
This kind of improvement reasoning can be applied to a wide range of care processes, large and small. For example, good surgery is the appropriate combination of hundreds of individual tasks, many of which could be improved in small ways. Aggregating these many smaller changes may result in important, observable improvement over time. The protocol-driven, randomised trial research approach is a powerful tool for establishing efficacy but has limitations for evaluating and improving such complex processes as surgery, which are continually and purposefully changing over time. The realities of clinical improvement call for a move from after-the-fact quality inspection to building quality measures into medical information systems, thereby creating real-time quality data for providers to act upon. Caring for populations of similar patients in similar ways (economies of scale) can be of particular value, because the resulting large numbers and process stability can help rapidly demonstrate variation in care processes 7 ; very tight control limits (minimal common-cause variation) allow special-cause variation to be detected more quickly.
Clinical and health-services researchers
While quality-management thinking tends towards the use of data plotted over time in control-chart format, clinical researchers think in terms of true experimental methods, such as RCTs. Health-services researchers, in contrast, think in terms of regression analysis as their principal tool for discovering explainable variation in processes and outcomes of care. The data that both communities of researchers use are generally collected during fixed periods of time, or combined across time periods; neither is usually concerned with the analysis of data over time.
Take, for example, the question of whether age and sex are associated with the ability to undertake early ambulation after hip surgery. Clinical researchers try to control for such variables through the use of entry criteria into a trial, and random assignment of patients to experimental or control group. The usual health-services research approach would be to use a regression model to predict the outcome (early ambulation), over hundreds of patients using age and sex as independent variables. Such research could show that age and sex predict outcomes and are statistically significant, and that perhaps 10% of the variance is explained by these two independent variables. In contrast, quality-improvement thinking is likely to conclude that 90% of the variance is unexplained and could be common-cause variation. The health-services researcher is therefore likely to conclude that if we measured more variables, we could explain more of this variance, while improvement scientists are more likely to conclude that this unexplained variance is a reflection of common-cause variation in a good process that is under control.
The entry criteria into RCTs are carefully defined, which makes it a challenge to generalise the results beyond the kinds of patients included in such studies. Restricted patient entry criteria are imposed to reduce variation in outcomes unrelated to the experimental intervention. RCTs focus on the difference between point estimates of outcomes for entire groups (control and experimental), using statistical tests of significance to show that differences between the two arms of a trial are not likely to be due to chance.
Individual patients and their healthcare providers
The question an individual patient asks is different from those asked by manager and researcher, namely ‘How can I get better?’ The answer is unique to each patient; the question does not focus on generalising results beyond this person. At the same time, the question the patient's physician is asking is whether the group results from the best clinical trials will apply in this patient's case. This question calls for a different inferential approach. 8–10 The cost of projecting general findings to individual patients could be substantial, as described below.
Consider the implications of a drug trial in which 100 patients taking a new drug and 100 patients taking a placebo are reported as successful because 25 drug takers improved compared with 10 controls. This difference is shown as not likely to be due to chance. (The drug company undertakes a multimillion dollar advertising campaign to promote this breakthrough.) However, on closer examination, the meaning of these results for individual patients is not so clear. To begin with, 75 of the patients who took the drug did not benefit. And among those 25 who benefited, some, perhaps 15, responded extremely well, while the size of the benefit in the other 10 was much smaller. To have only the 15 ‘maximum responders’ take this drug instead of all 100 could save the healthcare system 85% of the drug's costs (as well as reduce the chance of unnecessary adverse drug effects); those ‘savings’ would, of course, also reduce the drug company's sales proportionally. These considerations make it clear that looking at more than group results could potentially make an enormous difference in the value of research studies, particularly from the point of view of individual patients and their providers.
In light of the above concerns, we propose that the longitudinal factorial study design should be the gold standard of evidence for efficacy, particularly for assessing whether interventions whose efficacy has been established through controlled trials are effective in individual patients for whom they might be appropriate ( box 1 ). Take the case of a patient with hypertension who measures her blood pressure at least twice every day and plots these numbers on a run chart. Through this informal observation, she has learnt about several factors that result in the variation in her blood pressure readings: time of day, the three different hypertension medicines she takes (not always regularly), her stress level, eating salty French fries, exercise, meditation (and, in her case, saying the rosary), and whether she slept well the night before. Some of these factors she can control; some are out of her control.
Longitudinal factorial design of experiments for individual patients
The six individual components of this approach are not new, but in combination they are new 8 9
One patient with a chronic health condition; sometimes referred to as an ‘N-of-1 trial.’
Care processes and health status are measured over time. These could include daily measures over 20 or more days, with the patient day as the unit of analysis.
Whenever possible, data are numerical rather than simple clinical observation and classification.
The patient is directly involved in making therapeutic changes and collecting data.
Two or more inputs (factors) are experimentally and concurrently changed in a predetermined fashion.
Therapeutic inputs are added or deleted in a predetermined, systematic way. For example: on day 1, drug A is taken; on day 2, drug B; on day 3, drug A and B; day 4, neither. For the next 4 days, this sequence could be randomly reordered.
Since she is accustomed to monitoring her blood pressure over time, she is in an excellent position to carry out an experiment that would help her optimise the effects of these various influences on her hypertension. Working with her primary care provider, she could, for example, set up a table of randomly chosen dates to make each of several of these changes each day, thereby creating a systematically predetermined mix of these controllable factors over time. This factorial design allows her to measure the effects of individual inputs on her blood pressure, and even interactions among them. After an appropriate number of days (perhaps 30 days, depending on the trade-off between urgency and statistical power), she might conclude that one of her three medications has no effect on her hypertension, and she can stop using it. She might also find that the combination of exercise and consistently low salt intake is as effective as either of the other two drugs. Her answers could well be unique to her. Planned experimental interventions involving single patients are known as ‘N-of-1’ trials, and hundreds have been reported. 10 Although longitudinal factorial design of experiments has long been used in quality engineering, as of 2005 there appears to have been only one published example of its use for an individual patient. 8 9 This method of investigation could potentially become widely used in the future to establish the efficacy of specific drugs for individual patients, 11 and perhaps even required, particularly for very expensive drug therapies for chronic conditions. Such individual trial results could be combined to obtain generalised knowledge.
This method can be used to show (1) the independent effect of each input on the outcome, (2) the interaction effect between the inputs (perhaps neither drug A or B is effective on its own, but in combination they work well), (3) the effect of different drug dosages and (4) the lag time between treatment and outcome. This approach will not be practical if the outcome of interest occurs years later. This method will be more practical with patient access to their medical record where they could monitor all five of Bergman's core health processes. 12
Understanding variation is one of the cornerstones of the science of improvement
This broad understanding of variation, which is based on the work of Walter Shewart in the 1920s, goes well beyond such simple issues as making an intended departure from a guideline or recognising a meaningful change in the outcome of care. It encompasses more than good or bad variation (meeting a target). It is concerned with more than the variation found by researchers in random samples from large populations.
Everything we observe or measure varies. Some variation in healthcare is desirable, even essential, since each patient is different and should be cared for uniquely. New and better treatments, and improvements in care processes result in beneficial variation. Special-cause variation should lead to learning. The ‘Plan–Do–Study’ portion of the Shewhart PDSA cycle can promote valuable change.
The ‘act’ step in the PDSA cycle represents the arrival of stability after a successful improvement has been made. Reducing unintended, and particularly harmful, variation is therefore a key improvement strategy. The more variation is controlled, the easier it is to detect changes that are not explained by chance. Stated differently, narrow limits on a Shewhart control chart make it easier and quicker to detect, and therefore respond to, special-cause variation.
The goal of statistical thinking in quality improvement is to make the available statistical tools as simple and useful as possible in meeting the primary goal, which is not mathematical correctness, but improvement in both the processes and outcomes of care. It is not fruitful to ask whether statistical process control, RCTs, regression equations or longitudinal factorial design of experiments is best in some absolute sense. Each is appropriate for answering different questions.
Forces driving this new way of thinking
The idea of reducing unwanted variation in healthcare represents a major shift in thinking, and it will take time to be accepted. Forces for this change include the computerisation of medical records leading to public reporting of care and outcome comparisons between providers and around the world. This in turn will promote pay for performance, and preferred provider contracting based on guideline use and good outcomes. This way of thinking about variation could spread across all five core systems of health, 12 including self-care and processes of healthy living.
- Bergman B ,
- Lifvergren S ,
- Gremyer I ,
- Hellstrom A ,
- Neuhauser D
- Neuhauser D ,
Competing interests None.
Provenance and peer review Not commissioned; externally peer reviewed.
Read the full text or download the PDF:
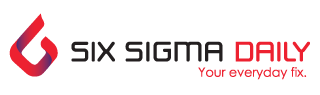
Common-Cause vs. Special-Cause
Common-cause variation is where no one, or combination of factors is unduly affected the process variation (random variation). special-cause variation is when one or more factors are affecting the process variation in a non-random way. with special-cause variation, one should be able to identify, or put their finger on the reason behind the unexpected variation..
Common-cause variation is where no one, or combination of factors is unduly affected the process variation (random variation). Special-cause variation is when one or more factors are affecting the process variation in a non-random way. With special-cause variation, one should be able to identify, or put their finger on the reason behind the unexpected variation .
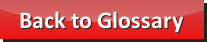

- Why This Book Is Needed
- Articles List, Additional Concepts
- Examples: 1-Page Summaries
- Examples: Concept Flow Diagram
- Examples: Compare and Contrast Tables
- Examples: Cartoons
- Example: Which to Use When Article
- Sample Articles
- Communicate
Common Cause - Special Cause
We hope you enjoyed this lesson.
Get the Statistical Process Control course for more great video tutorials.
Start free trial
Cool lesson, huh? Share it with your friends
Facebook Twitter LinkedIn WhatsApp Email
- Lesson resources Resources
- Quick reference Reference
About this lesson
Exercise files.
Download this lesson’s related exercise files.
Quick reference
SPC Control Charts are designed to differentiate between special cause variation and common cause variation. In order to understand the importance of this and the implication for control, this lesson explains and illustrates the difference.
When to use
Common cause variation is always present in a process. Special cause variation is present in an unstable process. Whenever a process manager seeks to control a process, he or she needs to separate the variation into the appropriate categories so that appropriate actions can be taken.
Instructions
SPC control charts are used to identify the differences between common cause variation and special cause variation. Once the process manager has determined the root cause for special cause variation and eliminated it, the remaining common cause variation is placed under statistical control in order to maintain a predictable process. For this reason, it is important that we clearly understand the differences between these two types of variation.
Common Cause
Common cause variation is the variation that is inherent in the design of the process characteristics. It is the typical variation between process operators, or the variation in equipment performance due to normal usage, it could even be differences in process performance due to environmental factors like heat, cold, or time of day. In one sense this variation is random, in another sense it is not. It is random to the extent that we don’t know if it will be higher or lower than the average value. However, the magnitude is not totally random. There is a normal range for the random variation that it will not exceed. Because of this, we can statistically model and predict the impact of the random variation on process performance. Finally, random variation can only be changed by making a fundamental process change so that the factors outlined earlier are different.
Special Cause
Special cause variation is not due to the inherent variation within the process design. It has as a unique root cause that is outside the inherent process operational characteristics. This root cause is not something the operator is normally monitoring and controlling. The occurrence of this root cause is unpredictable in timing and the magnitude of the impact is also unpredictable. That is why we say that the presence of a special cause variation leads to an unstable or unpredictable process. This unpredictability cannot be mathematically modeled and used to predict normal process performance. It is important to note that special cause variation is not always negative variation. Special causes may change important process parameters that reduce the inherent common cause variation within a process. This is referred to as the Hawthorne Effect – named after a research study conducted in Hawthorne, Illinois. In this study of worker productivity, it was determined that the primary influence on worker productivity was the special cause of having all the study specialists observing the worker’s behavior. Since they knew they were being studied, the worker changed their normal practice to be much more careful – but only when they were being watched.
Common Cause – Special Cause – Control Charts
Control charts are designed to differentiate between common cause variation and special cause variation. This is so the process managers and operators can remove the special cause variation which makes the process predictable. At that point, they can either maintain the normal variation or consider a fundamental change to the process to reduce the magnitude of the normal variation in the process. The operator should never chase the ups and downs of normal variation. Doing that will introduce process tampering which often leads to overcontrolling of the output and makes the overall performance even worse.
Hints & tips
- Identify and resolve special cause variation before attempting to make changes to the process and influencing common causes.
- Beware if tampering – it is an easy trap to fall into. It may give a short term benefit, due to the Hawthorne effect. But unless the process is fundamentally changed, it will go back to the original levels of common cause variation.…
- 00:04 Hi, I'm Ray Sheen.
- 00:06 Let's review the concepts of common cause and special cause variation.
- 00:11 A primary purpose of SPC charts is to identify the presence and
- 00:15 the magnitude of each of these variation categories.
- 00:19 I’ll start with what I mean by categories of variation.
- 00:23 All process variation can be divided into one of two categories.
- 00:27 The first is common cause variation.
- 00:30 This variation is always present, even in a stable process.
- 00:33 It’s inherent in the physical design and operation of the process.
- 00:37 Because it is always present, we can measure it and
- 00:40 establish a baseline of the normal variation using statistical techniques.
- 00:44 The specific instance of variation on any process run is random, but
- 00:49 is always within a set of boundaries that are predictable.
- 00:52 There are limits to this normal variation, which we can calculate and
- 00:55 plot on our control charts.
- 00:58 The second category of variation is special cause variation.
- 01:01 This is associated with unstable processes because it is not predictable.
- 01:06 It is due to something unusual.
- 01:08 Often, that something is poor process management by the business managers or
- 01:12 the process operators.
- 01:14 This type of variation is not predictable.
- 01:17 We can neither predict when it will occur or
- 01:19 what the magnitude of the value will be when it does occur.
- 01:22 Therefore, when it happens it creates an unexpected process performance, and
- 01:26 often requires special action to restore performance.
- 01:30 Let's look at each of these in a little more detail.
- 01:33 I'll start with common cause.
- 01:35 Common cause variation is always present and
- 01:37 is predictable with respect to the magnitude.
- 01:39 Therefore, it should be accounted for when setting process performance targets, and
- 01:44 allowed for within the tolerances on those performance targets.
- 01:48 Let me clarify the nature of randomness of common cause variation.
- 01:52 It is random with respect to any specific occurrence of variation.
- 01:56 Might be a little high or a little low as compared to normal performance, but
- 01:59 that variation is always within predictable magnitude range.
- 02:03 That means we can establish a normal range and a predictable variation.
- 02:08 What we can't do is eliminate common cause variation by taking some form of special
- 02:12 corrective action to chase the variation.
- 02:15 By that I mean if we follow the process and results are a little higher than
- 02:19 normal, we try to tweak the process to be a little lower to compensate.
- 02:23 Think about the young teenager learning to drive.
- 02:26 They over control the car while trying to stay in their lane and
- 02:29 end up going way outside the lines.
- 02:31 Trying to compensate for each occurrence of common cause variation creates
- 02:35 process tampering as a special cause, and normally results in an unstable process.
- 02:41 Okay, now let's look at special cause variation.
- 02:44 When Special Cause variation is present, we say the process is unstable.
- 02:48 That's because the process performance is no longer predictable.
- 02:51 Special Cause variation is not controllable by the process operator.
- 02:56 Therefore, they don't know what the process results will be on any given
- 02:59 run of the process.
- 03:00 Now, you may be thinking, well, that's true for common cause variation also.
- 03:04 The part of that is true for common cause variation is that
- 03:07 you don't know precisely what the process result will be, but you do
- 03:11 know that it will fall within predictable limits of common cause variation.
- 03:15 The problem with special cause is that it falls outside those limits
- 03:19 of common cause variation and you have no ability to predict how far outside.
- 03:24 Special Cause variation is unpredictable, but it's not random.
- 03:27 It has a clear cause that precipitates the variation.
- 03:30 There is a clear, identifiable root cause that if it had not occurred,
- 03:34 there would have been no Special Cause variation.
- 03:37 The good news about that is that sometimes we can find out
- 03:39 what created that underlying root cause and we can take actions to prevent it and
- 03:44 eliminate the Special Cause variation from occurring again.
- 03:48 One other point, the Special Cause variation is not always bad.
- 03:51 Sometimes it is special good.
- 03:53 This means that there is some root cause that is preventing normal cause variation
- 03:57 from occurring.
- 03:58 This can give us a false sense of the range for normal variation.
- 04:02 There's a famous research study conducted in Hawthorne, Illinois,
- 04:05 that illustrated this point.
- 04:07 The purpose of the study was to determine the effect of adding lighting
- 04:10 to the workplace of an industrial assembly line.
- 04:12 Well, first this test was done to establish a baseline without
- 04:15 lighting present.
- 04:16 Then lighting was added and the productivity was measured.
- 04:19 It had improved and
- 04:21 the sponsor of the research, a lighting company, was excited by the results.
- 04:25 However, the researchers did one more assessment.
- 04:28 They turned the lights off and measured productivity again, and
- 04:31 it had improved even more.
- 04:33 So the research said that the best productivity was
- 04:35 achieved when you installed lighting but turned it off.
- 04:39 Yeah, I agree.
- 04:40 That doesn't make much sense.
- 04:41 But with further study,
- 04:43 they found that while lighting helped, the biggest effect on productivity was
- 04:47 having all those researchers there in the factory collecting data all the time.
- 04:51 This was a special cause that disrupted the normal work habits of the people on
- 04:55 the production line.
- 04:56 So let's wrap this up with a comparison of common cause and Special Cause variation.
- 05:02 Common cause is the normal and predictable variation that occurs within a process.
- 05:07 It's predictable in the sense of the magnitude always falls within limits or
- 05:10 boundaries of process performance.
- 05:13 It is always present because it is inherent in the process design.
- 05:17 The predictable aspect allows us to mathematically model this variation,
- 05:20 which let's us establish defined limits for it.
- 05:23 One last point,
- 05:24 it cannot be eliminated by the process operators taking special action.
- 05:28 The only way to improve it is to fundamentally change the process
- 05:33 to one with different physical characteristics, and
- 05:35 therefore, lower levels of random variation.
- 05:39 In contrast, the special cause variation is unpredictable.
- 05:43 The process operator does not know when it will occur.
- 05:45 And if it does occur, the operator is unable to predict the process results
- 05:50 due to the impact of the special cause.
- 05:52 It is outside of the control of the process operator,
- 05:55 which is why we say it makes the process unstable.
- 05:59 The occurrence and magnitude cannot be mathematically predicted.
- 06:02 It is based upon some external unique root cause.
- 06:05 Now, although the operator cannot control the effect,
- 06:08 sometimes they can control the conditions that allow the unique root cause to occur.
- 06:13 In that case, it can be preventable.
- 06:16 This review of the principles of common cause variation and special cause
- 06:20 variation was necessary because we are about to see that SPC control charts
- 06:24 are designed to differentiate between these two types of variation.
Lesson notes are only available for subscribers.
PMI, PMP, CAPM and PMBOK are registered marks of the Project Management Institute, Inc.
© 2024 GoSkills Ltd. Skills for career advancement

Common Cause & Special Cause Variation Explained with Examples
Editorial Team
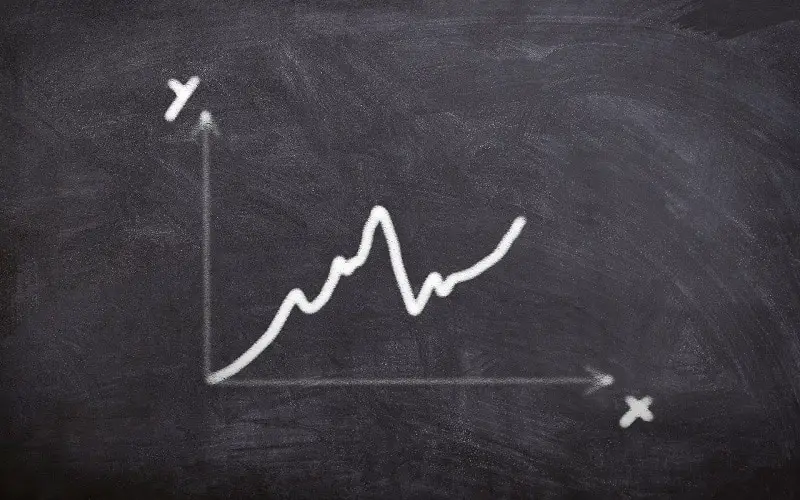
In any business operation, it is important to ensure consistency in products as well as repeatable results. Managers and workers alike have to be aware of the processes and methods on how to produce consistent outcomes at all costs. However, we cannot deny that producing exactly identical products or results is almost impossible as variance tends to exist. Variation is not necessarily a bad thing as long as it is within the standard of the critical to qualities (CTQs) specification limits.
Process variation is the occurrence when a system deviates from its fixed pattern and produces a result which differs from the usual ones. This is a major key as it concerns the consistencies of the transactional as well as the manufacturing of the business systems. Variation should be evaluated as it portrays the reliability of the business for the customers and stakeholders. Variation may also cost money hence it is crucial to keep variation at bay to prevent too much cost spent on variation. It is crucial to be able to distinguish the types of variance that occur in your business process since it will give the lead on what course of action to take. Mistakes in coming up with an effective reaction plan towards the variance may worsen the processes of the business.
There are two types of process variation which will be further elaborated in this article. The variations are known as common cause variation and special cause variation.
Common Cause Variation Definition
Common cause variation refers to the natural and measurable anomalies that occur in the system or business processes. It naturally exists within the system. While it is true that variance may bring a negative impact to business operations, we cannot escape from this aspect. It is inherent and will always be. In most cases, the common cause variant is constant, regular, and could be predicted within the business operations. The other term used to describe this variation is Natural Problems, Noise, or Random Cause. Common cause variance could be presented and analysed using histogram.
What is Common Cause Variation
There are several distinguishable characteristics of common cause variation. Firstly, the variation pattern is predictable. Common cause variation occurring is also an active event in the operations. it is controlled and is not significantly different from the usual phenomenon.
There are many factors and reasons for common cause variation and it is quite difficult to pinpoint and eliminate them. Some common cause variations are accepted within the business process and operations as long as they are within a tolerable level. Eradicating them is an arduous effort unless a drastic measure is implemented towards the operation.
Common Cause Variation Examples
There is a wide range of examples for common cause variation. Let’s take driving as an example. Usually, a driver is well aware of their destinations and the conditions of the path to reach the destination. Since they have been regularly using the same road, any defects or problems such as bumps, conditions of the road, and usual traffic are normal. They may not be able to precisely arrive at the destination at the same duration every time due to these common causes. However, the duration to arrive at the destination may not be largely differing day to day.
In terms of project-related variations, some of the examples include technical issues, human errors, downtime, high trafficking, poor computer response times, mistakes in standard procedures, and many more. Some other examples of common causes include poor design of products, outdated systems, and poor maintenance. Inconducive working conditions may also result in to common cause variants which could comprise of ventilation, temperature, humidity, noise, lighting, dirt, and so forth. Errors such as quality control and measurement could also be counted as common cause variation.
Special Cause Variation Definition
On the other hand, special cause variation refers to the unforeseen anomalies or variance that occurs within business operations. This variation, as the name suggests, is special in terms of being rare, having non-quantifiable patterns, and may not have been observed before. It is also known as Assignable Cause. Other opinions also mentioned that special cause variation is not only variance that happens for the first time, a previously overlooked or ignored problem could also be considered a special cause variation.
What is Special Cause Variation
Special cause variation is irregular occurrences and usually happens due to changes that were brought about in the business operations. It is not your mundane defects and may be very unpredictable. Most of the time, special cause variation happens following the flaws within the business processes or mechanism. While it may sound serious and taxing, there are ways to fix this which is by modifying the affected procedures or materials.
One of the characteristics of special cause variation is that it is uncontrolled and hardly predictable. The outcome of special causes variation is significantly different from the usual phenomenon. Since the issues are not predictable, it is usually problematic and may not even be recorded in the historical experience base.
Special Cause Variation Examples
As mentioned earlier, special cause variations are unexpected variants that occur due to factors that may affect the business system or operations. Let’s have an example of a special cause using the same scenario as previously elaborated for common cause variation example. The mentioned defects were common. Now, imagine if there is an unexpected accident that happens on the same road you usually take. Due to this accident, the time for the driver to arrive at the same destination may take longer than normal. Hence this accident is considered as a special cause variation. It is unexpected and results in a significantly different outcome, in this case, a longer time to arrive at the destination.
The example of special cause variation in the manufacturing sector includes environment, materials, manpower, technology, equipment, and many more. In terms of manpower, imagine a new employee is recruited into the team and still lacking in experience. The coaching and instructions should be adapted to consider that the person needs more training to be able to perform their tasks efficiently. Cases where a new supplier is needed in a short amount of time due to issues faced by the existing supplier are also unforeseen hence considered a special cause variation. Natural hazards that are beyond predictions may also be categorized into special cause variation. Some other examples include irregular traffic or fraud attack. An unexpected computer crash or malfunction in some of the components may also be considered as a special cause variation.
Common Cause and Special Cause Variation Detection
Control chart
One of the ways to keep track of common cause and special cause variation is by implementing control charts. When using control charts, the important aspect to be considered is firstly, establishing the average point of measurement. Next, establish the control limits. Usually, there are three standard deviations which are marked above and below the average point earlier. The last step is by determining which points exceed the upper and lower control limits established earlier. The points beyond the limits are special cause variation.
Before we get into the control chart of common cause and special cause variation, let’s have a look at the eight control chart rules first. If a process is stable, the points displayed in the chart will be near the average point and will not exceed the control limits.
However, it should be noted that not all rules are applicable to all types of control charts. That aside, it is quite tough to identify the causes of the patterns since special cause variation may be related to the specific type of processes. The table presented is the general rule that could be applied in most cases but is also subject to changes or differences. Studying the chart should be accompanied by knowledge and experiences in order to pinpoint the reasons for the patterns or variations.
A process is considered stable if special cause variation is not present, even if a common cause exists. A stable operation is important before it could be assessed or being improved. We could look at the stability or instability of the processes as displayed in control charts or run charts .
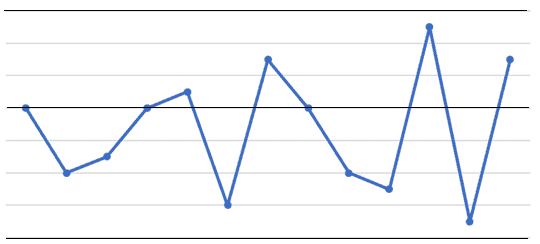
The points displayed in the chart above are randomly distributed and do not defy any of the eight rules listed earlier. This indicates that the process is stable.
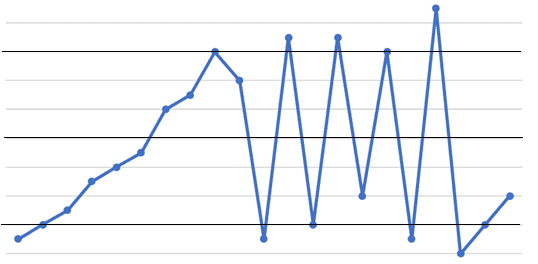
The chart presented above is an example of an unstable process. This is because some of the rules for control chart tests mentioned earlier are violated.
Simply, if the points are randomly distributed and are within the limit, they may be considered as the common cause variation. However, if there is a drastic irregularity or points exceeding the limit, you may want to analyse more into it to determine if it is a special cause variation.
Histogram is a type of bar graph that could be used to present the distribution of occurrences of data. It is easily understandable and analysed. A histogram provides information on the history of the processes done as well as forecasting the future performance of the operations. To ensure the reliability of the data presented in the histogram, it is essential for the process to be stable. As mentioned earlier, although affected by common cause variation, the processes are still considered stable, hence histogram may be used on this occasion, especially if the processes undergo regular measurement and assessment.
The data is considered to be normally distributed if it portrays a “bell” shape in the histogram. The data are grouped around the central value and this cluster is known as variation. There are several other examples of more complicated patterns, such as having several peaks in the histogram or a shortened histogram. Whenever these examples of complex structures appear in the histogram, it is fundamental to look into the data and operations more deeply.
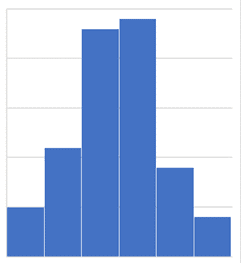
The above bar graph is an example of the histogram with a “bell” shape.
However, it should be noted that just because the histogram displays a “bell” shaped distribution, that does not mean the process is only experiencing common cause variation. A deeper analysis should be done to investigate if there were other underlying factors or causes that lead towards the pattern of the distribution displayed in the histogram.
Countering common cause and special cause variation
Once the causes of the variation have been pinpointed, here comes the attempt to combat and resolve it. Different measures are implemented to counter different types of variation, i.e. common cause variation and special cause variation. Common cause variation is quite tough to be completely eliminated. Drastic or long-term process modification could be used to counter common cause variation. A new method should be introduced and constantly conducted to achieve the long-term goal of eliminating the common cause variation. Some other effects may happen to the operations but as time passes, the cause may be gradually solved. As for special cause variation, it could be countered using contingency plans. Usually, additional processes are implemented into the usual operation in order to counter the special cause variation.
- What To Do If You Have “A Perfect Student” Syndrome?
- How to Effectively Find and Use the Best Possible Suppliers in 2022
- 5 Reasons to Screen Potential Employees
- Why It’s Important to Hire an InventHelp Patent Attorney?
most recent
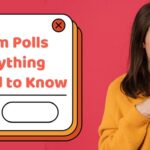
Tips & Guides
Instagram polls and everything you need to know.
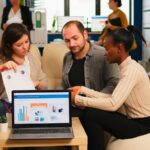
How to Overcome Challenges of ERP Integration
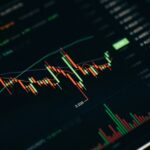
The Basics of Cryptocurrency Popularity
© 2024 Copyright ProjectPractical.com
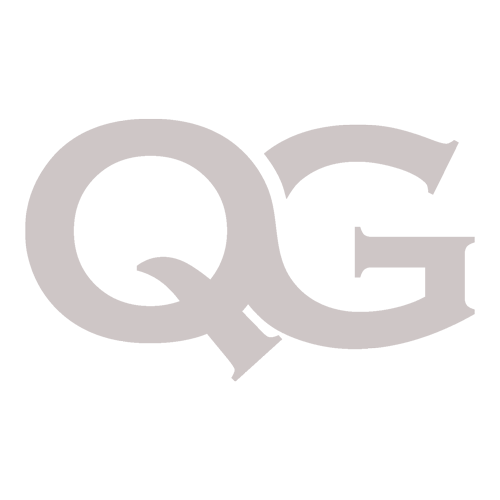
- ASQ® CQA Exam
- ASQ® CQE Exam
- ASQ® CSQP Exam
- ASQ® CSSYB Exam
- ASQ® CSSGB Exam
- ASQ® CSSBB Exam
- ASQ® CMQ/OE Exam
- ASQ® CQT Exam
- ASQ® CQPA Exam
- ASQ® CQIA Exam
- 7 Quality Tools
- Quality Gurus
- ISO 9001:2015
- Quality Cost
- Six Sigma Basics
- Risk Management
- Lean Manufacturing
- Design of Experiments
- Quality Acronyms
- Quality Awareness
- Quality Circles
- Acceptance Sampling
- Measurement System
- APQP + PPAP
- GD&T Symbols
- Project Quality (PMP)
- Full List of Quizzes >>
- Reliability Engineering
- Statistics with Excel
- Statistics with Minitab
- Multiple Regression
- Quality Function Deployment
- Benchmarking
- Statistical Process Control
- Quality Talks >> New
- Six Sigma White Belt
- Six Sigma Yellow Belt
- Six Sigma Green Belt
- Six Sigma Black Belt
- Minitab 17 for Six Sigma
- Casio fx-991MS Calculator
- CSSYB/LSSYB Mock Exam
- CSSGB/LSSGB Mock Exam
- CSSBB/LSSBB Mock Exam
- ASQ® CQA Preparation
- ASQ® CQE Preparation
- ASQ® CQPA Preparation
- ASQ® CQIA Preparation
- CQE Mock Exams
- CMQ/OE Mock Exams
- CQA Mock Exams
- CQIA Mock Exams
- CQPA Mock Exam
- CQT Mock Exam
- CQI Mock Exam
- CSQP Mock Exam
- Design of Experiments (DoE)
- Measurement System Analysis
- Statistics Using R
- Data Visualization with R
- Statistics Using Python
- Data Visualization with Python
- Regression with Minitab
- Logistic Regression
- Data Analysis Using Excel
- The Git Mindset
- Statistics Quiz
- Root Cause Analysis
- Kano Analysis
- Lean Management
- QMS Lead Auditor
- Quality Management
- ISO 9001:2015 Transition
- Project Quality Manager
- गुणवत्ता.org
- Summary Sheets
- Practice Tests
- QG Hall of Fame
- Testimonials – ASQ Exams Preparation
Difference Between , Qualitypedia , Six Sigma
- Special Cause vs. Common Cause Variation
** Unlock Your Full Potential **
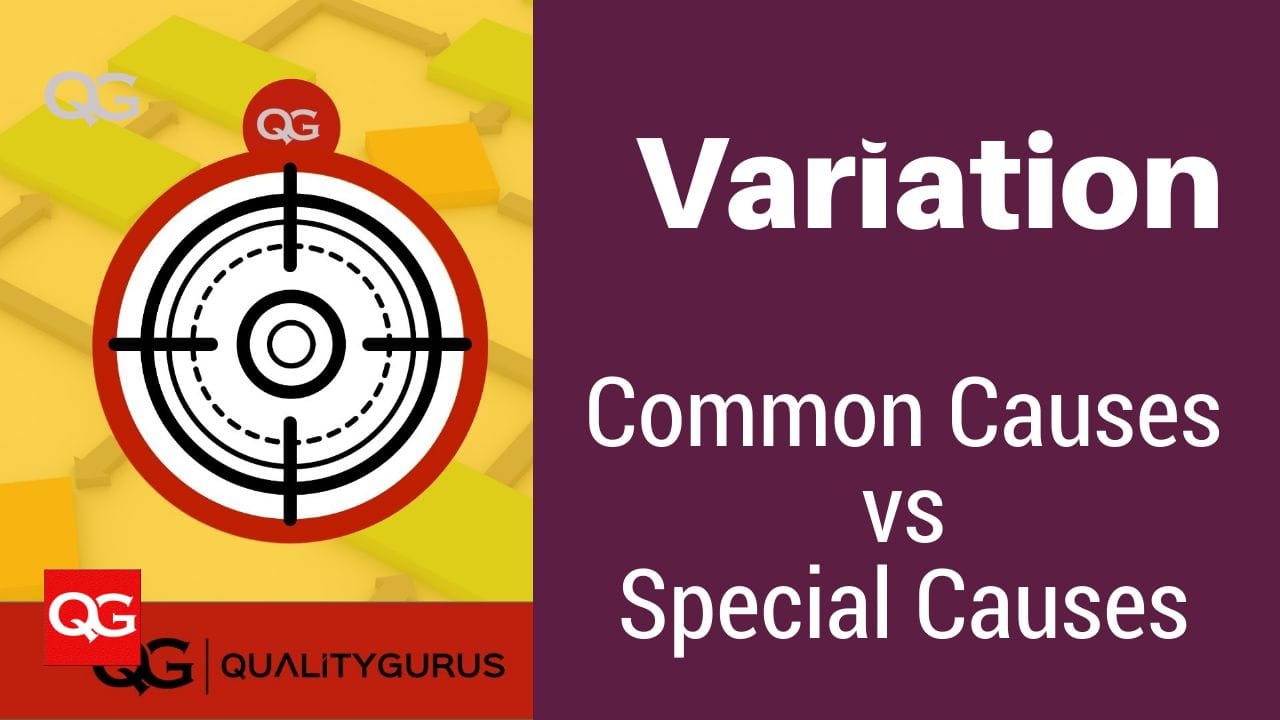
What is the variation?
Whatever measurement we take, there is always a variation between these measurements. No two items or measurements are precisely the same.
The problem with the variation is that it is the enemy of quality. Variation and quality do not go hand in hand. Variation reduction is one of the significant challenges of quality professionals.
Two types of variation, and why is it important to differentiate?
When dealing with variation, the challenge quality professionals face when to act and when not to act. Because if you act on each and every variation in the process and adjust the process, this will be a never-ending process. Dr. Deming called this "tempering the process." Rather than improving the quality, tempering, in fact, reduces the quality. Deming demonstrated the effect of tempering with the help of a funnel experiment.
The causes of variation can be classified into two categories:
- Common Causes
- Special Causes
Statistical Process Control Bootcamp
- $130 course for just $15.99 today!
- Learn Control Charts , Process Capability using Excel and Minitab
- 7+ hours of videos, slides & quizzes
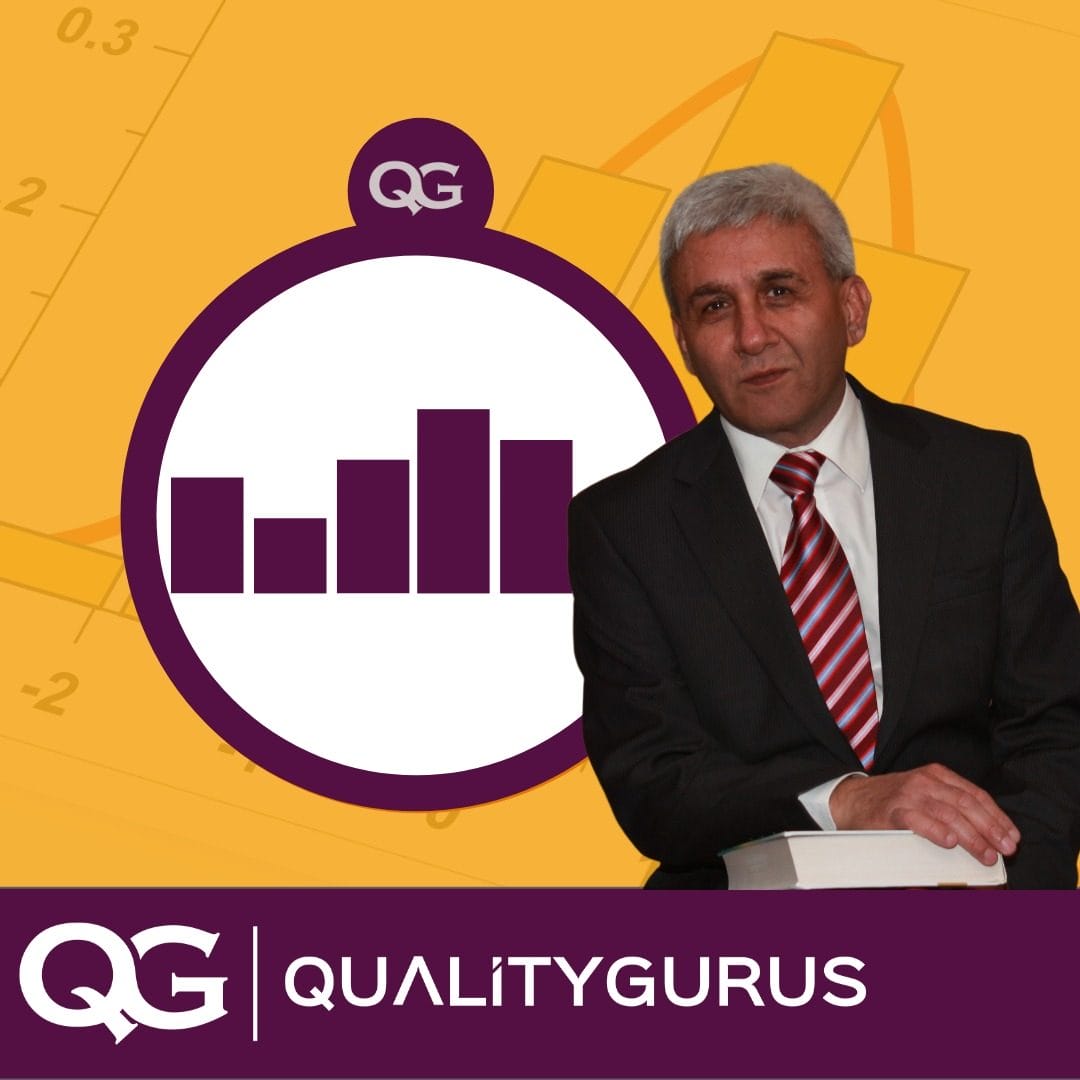
Common Cause Vs Special Cause: Types of Variation
Common cause variation is the natural variation in the process. It is a part of the process. There are "many" causes of this type of variation, and it is not easy to identify and remove these. You will need to live with them unless drastic action is taken, such as process re-engineering.
Common causes are also called n atural causes, noise, non-assignable and random causes .
Special cause variation, on the other hand, is the unexpected variation in the process. There is a specific cause that can be assigned to the variation. For that reason, this is also called as the assignable cause . You are required to take action to address these variations.
Special causes are also called assignable causes .
Seven Basic Quality Tools
- Get this $135 course for just $13.99 today!
- 3+ hours of videos, slides & quizzes
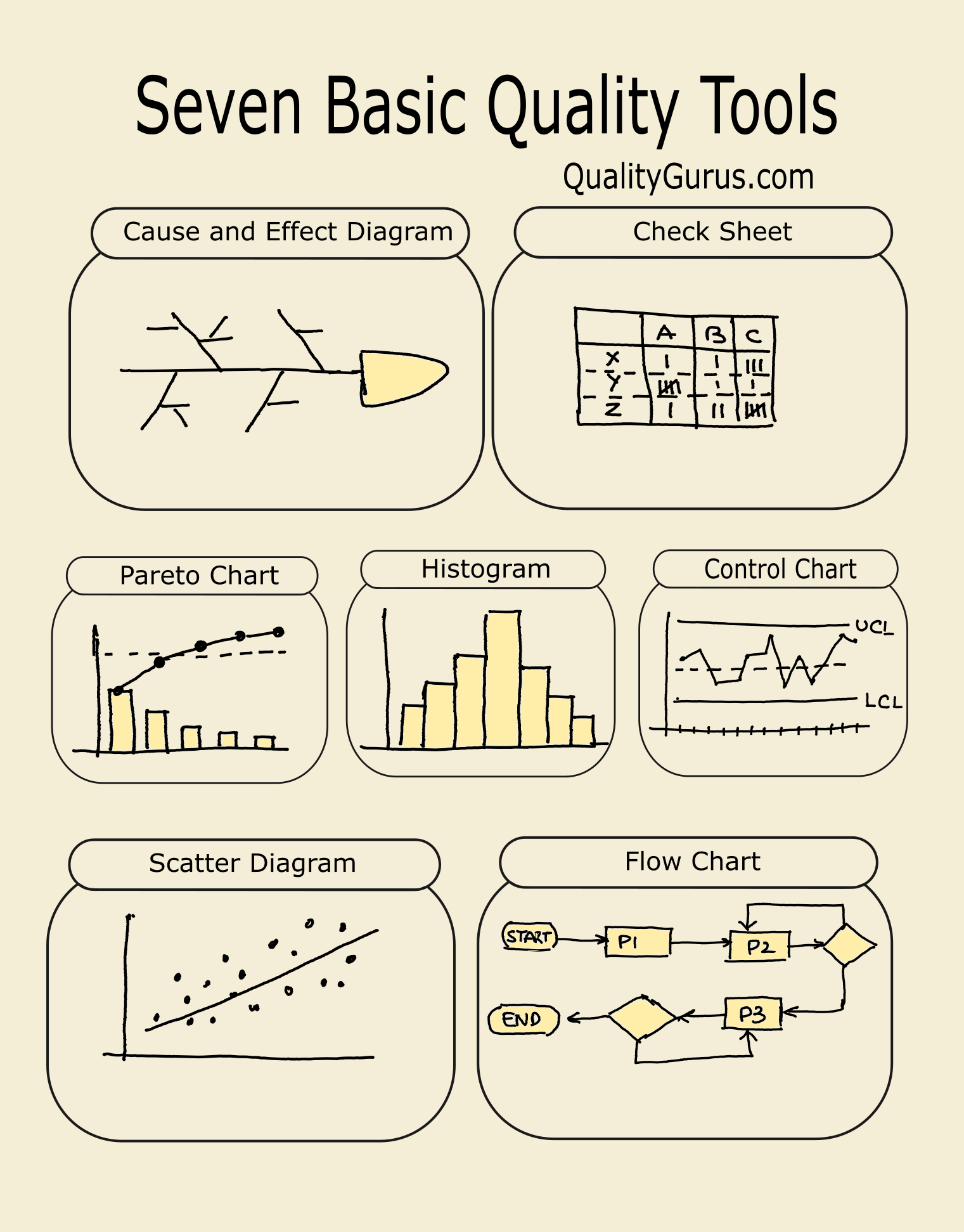
Control Charts to identify special causes
If the measurements of a process are normally distributed, then there is a 99.73% chance that the measurement will be within plus and minus three standard deviations. This is the basis of control charts .
If you plot the measurements on a Control Chart , then any measurements which are outside the plus and minus three standard deviation limits are expected to be because of a special cause. These limits are called as the Upper Control Limit (UCL) and the Lower Control Limits (LCL), Once you get such measurement, you are expected to investigate, do the root cause analysis , find out the reason for such deviation and take necessary actions.
Root Cause Analysis - Online Training
- 8+ hours of videos, slides & quizzes
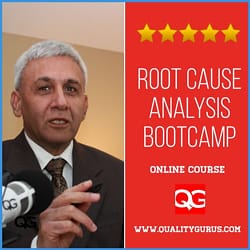
Similar Posts:
December 22, 2022
Understanding Probability: A Guide for Beginners
December 23, 2021
Converting VoC to CTQs
March 31, 2018
Benchmarking – Definition, Types and Steps
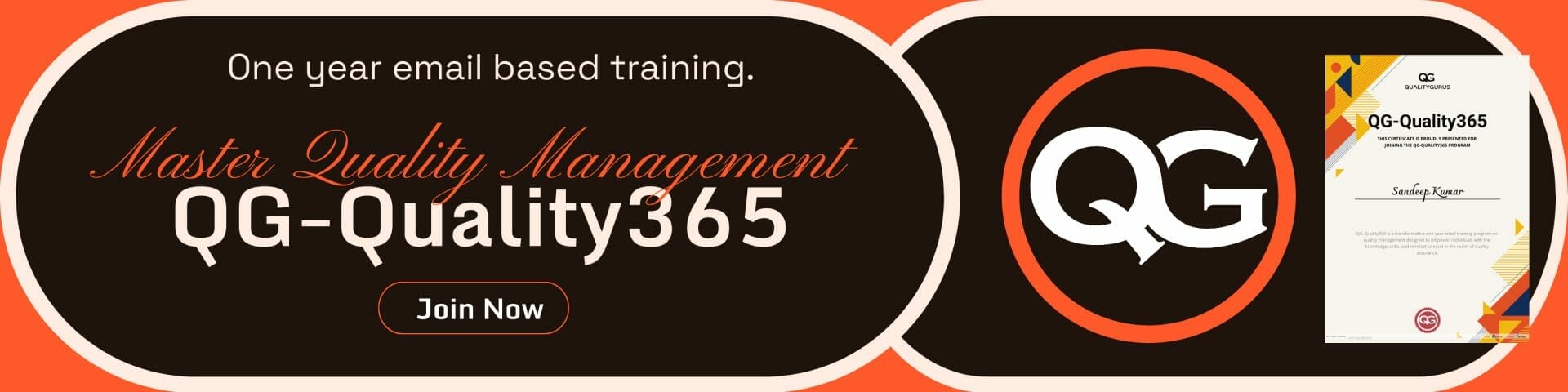
49 Courses on SALE!
- Pharmaceutical Engineering Magazine
- Online Exclusives
- Special Reports
- Facilities & Equipment
- Information Systems
- Product Development
- Production Systems
- Quality Systems
- Regulatory Compliance
- Research + Development
- Supply Chain Management
- White Papers
- iSpeak Blog
- Editorial Calendar
- Article of the Year
- Submit an Article
- Editorial Team
Embrace Special Cause Variation During Continued Process Verification (CPV)
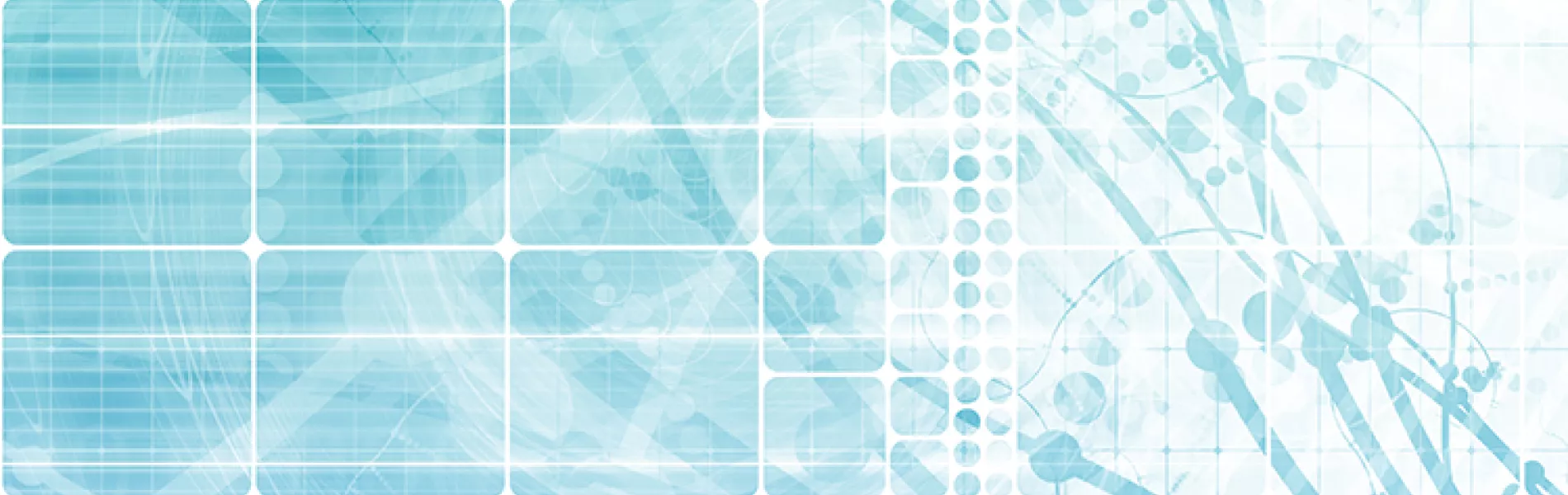
Understanding the fundamental assumption of independence (and the violation thereof) enables an appropriate response to control chart trend rule violations and challenges us to think differently about special cause variation in control charts.
To align with the 2011 US Food and Drug Administration (FDA) guidance on process validation, actions must be taken during the continued process verification (CPV) stage so that “[o] ngoing assurance is gained during routine production that the process remains in a state of control.” 1 Process monitoring during this stage provides valuable information that can be used to address process problems and identify opportunities for improvement. Successful implementation of CPV requires not only that decisions and actions be aligned with these goals; the use of statistical tools such as control charts must also be understood within the context of pharmaceutical/biologics manufacture and a risk-based approach to lifecycle process validation.
It is common practice to use Shewart control charts to monitor process behavior during the CPV stage. Quite often manufacturers apply a selection of the Western Electric or Nelson rules* to their control charts. 2 , 3 These rules were designed to signal significant process change and to justify action, often in real time, to address the change in process behavior.
Often not incorporated into the design and interpretation of these control charts, however, is the influence of the most important assumption underlying conventional interpretation: independent observations. 4 When observations are independent, variation is a result of random sources; the output of batch #1 is no more similar to batch #2 than it is to batch #25. Because this assumption is rarely met for pharmaceutical quality attributes, adaptation of typical control chart interpretation is critical; otherwise, CPV programs can be designed that not only misguide and waste resources, but actually hinder CPV goals.
Why is understanding this assumption so critical to a successful CPV program? Reach for almost any Lean Six Sigma reference to process control and you will find variability described as either “common cause,” due to typical, random sources of variability, or “special cause,” which reflects unexpected variability that is likely the result of a process change. Further description of the two types typically assigns an assessment of process control. Specifically, a process displaying only common cause variation is often said to be “in control” or “stable and predictable.” Special cause variation, in contrast, is generally described as unexpected or unnatural variation, and indicates that the process may be “unstable and unpredictable” or “out of control.” 5 , 6 , 7
The immediate translation of a statistical signal to potential loss of process control is valid only if the fundamental assumption of independence is met.
When a process is in a state of control and sources of variability have random influence across time, points on the control chart should have a random pattern. 4 , 8 Evidence of special cause variation can be an unexpected event, such as a single point outside of a control limit or a pattern not expected by random chance. In commonly used statistical software packages, such events can be associated with the Nelson rules, and identified on a control chart as a red symbol and a number identifying the specific pattern, commonly referred to as a statistical signal. This special cause designation is often associated with a call for action or investigation into the process to bring it back to a state of control. Thus, “red” is viewed as a problem. Some references are more extreme, stating that special causes must be eliminated before the control chart can be used as a monitoring tool. 8
It is critical to understand, however, that the immediate translation of a statistical signal to potential loss of process control is valid only if the fundamental assumption of independence is met. And in the usual manufacture of pharmaceutical and biological products, nonindependence is the norm, since typical sources of variability (raw materials, equipment, lab factors, etc.) are not used randomly. They meet the “typical” and “expected,” but not “random” criteria of the common cause variability definition.
In this scenario, patterns of variability that result from the nonrandom use of these typical sources may be identified as special cause variation. Practically speaking, this is a different situation than special cause variation that results from a true process upset, such as error in addition of raw materials. In the realm of non-independence, overreaction can occur when all special cause notations are automatically perceived as reflections of a process that is out of control.
* To distinguish between natural and unnatural variation, Western Electric expanded on the three standard error decision rules of Walter Shewhart. Based on these decision rules, Lloyd Nelson later formulated a set of tests for assignable causes.
- 1 US Food and Drug Administration. Guidance for Industry. “Process Validation: General Principles and Practices.” Revision 1. January 2011. http://www.fda.gov/downloads/Drugs/.../Guidances/UCM070336.pdf
- 2 Western Electric Co., Inc. Statistical Quality Control Handbook. Easton, Pennsylvania: Mack Printing Company, 1956.
- 3 Nelson, Lloyd S. “Technical Aids.” Journal of Quality Technology 16, no. 4 (October 1984).
- 4 a b Montgomery, Douglas C. Introduction to Statistical Quality Control, 7th ed. John Wiley & Sons, Inc., 2013.
- 5 George, Michael L., et al. The Lean Six Sigma Pocket Toolbook, McGraw-Hill, 2005.
- 6 Wedgewood, Ian D. Lean Sigma: A Practitioner’s Guide, Prentice Hall, 2007.
- 7 Brue, Greg. Six Sigma for Managers, McGraw-Hill, 2002.
- 8 a b Six Sigma Academy. The Black Belt Memory Jogger, 1st edition, GOAL/QPC, 2002.
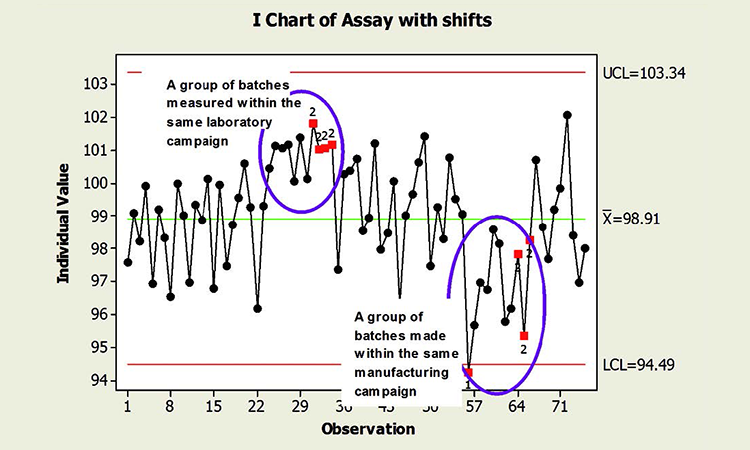
If non-independence precludes the conventional interpretation of trend rules and special cause variation, 4 , 5 does that mean basic control charts can’t be used? Certainly not. These tools, in their simplest forms, are very powerful, even in the presence of non-independence. The nonrandom use of variability sources (such as different raw material lots) is not the problem. The problem results when control chart interpretation is not appropriately modified from the classical statistical process control (SPC) paradigm in which independence is assumed.
When common cause sources of variability are not used randomly across time, “unlikely” patterns are really not so unlikely. For instance, the pattern of nine points on the same side of the control chart centerline can be expected. These patterns do not necessarily indicate that the process is out of control; it is often a reflection of the process as designed. The absence of statistical signals cannot be required to claim a state of control. Because of non-independence, the “state of control” may include results identified as special cause variation, as shown in Figure 1.
The figure shows two shifts in the data, one representing a group of batches measured in the same laboratory campaign, and another produced during the same manufacturing campaign. The observed behavior is not inherently unexpected; the process is not necessarily “out of control,” in the sense that the shifts in mean forecast instability and risk. The shifts may in fact be expected, since batches within the same laboratory and manufacturing campaign share common sources of variability that are quite likely different from the other campaigns represented. Even the point below the lower control limit is a potential artifact of non-independence. (See the discussion preceding the conclusion.)
Figure 2 is a chart of the same data in random order. Randomizing results reflects what could be expected if the common cause variables that influence individual campaigns were experienced randomly across time. Not surprisingly, no special cause variation is identified. Note, too, that the true process performance in Figure 2 is no better than that of Figure 1. Process performance and control cannot be measured by the amount of “red.” The charts could be interpreted incorrectly if the underlying data structure—and its effects on chart interpretation—were not understood.
Given this context, the appropriate response to this variation is not as straightforward as a simple textbook case, where a red symbol on a control chart becomes a call for action. Nor are the patterns and resulting signals in Figure 1 false alarms in the statistical sense; they do indicate performance that is statistically unexpected relative to overall performance. Thus, they can provide valuable information regarding the sources of variability, directly enabling the CPV goals of ongoing quality assurance and continual improvement.
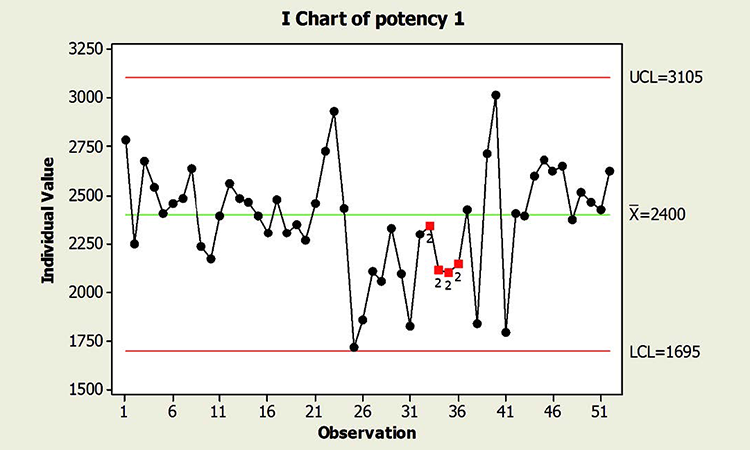
When textbook statistical interpretation of signals is not valid, a risk-based approach can define the urgency and appropriate reaction to special cause variability. Consider the following pair of charts: In each case, a shift in the mean is identified with a red number 2 following 9 points in a row on the same side of the mean. The magnitude of the shift relative to overall performance is about the same. But should reaction to these patterns also be the same?
In a presentation at the 2015 ISPE Statistician Forum on process validation, a member of the FDA Center for Drug Evaluation and Research, Office of Pharmaceutical Quality, noted that “…all signals are not created equally” and “… magnitude of the response depends on the severity of the signal …” 9 In this hypothetical situation, how might that advice be interpreted? Both figures reflect the same attribute (potency), and it is likely that potency would be rated as a high severity attribute. Does that mean that every signal must be investigated, and to the same level? What additional information might be useful to define the severity of the signal and an appropriate response in each scenario?
Consider the charts in Figure 4, which include more historical data prior to the shift, and specification limits (blue lines). This additional information is used to assess: 1) if the pattern is truly unexpected, and 2) risk.
- 9 Viehmann, Alex. “Process Monitoring Expectations and Establishing Acceptance Criteria.” Presented at the ISPE Statistician Forum, Silver Spring, MD, 14–15 April 2015.
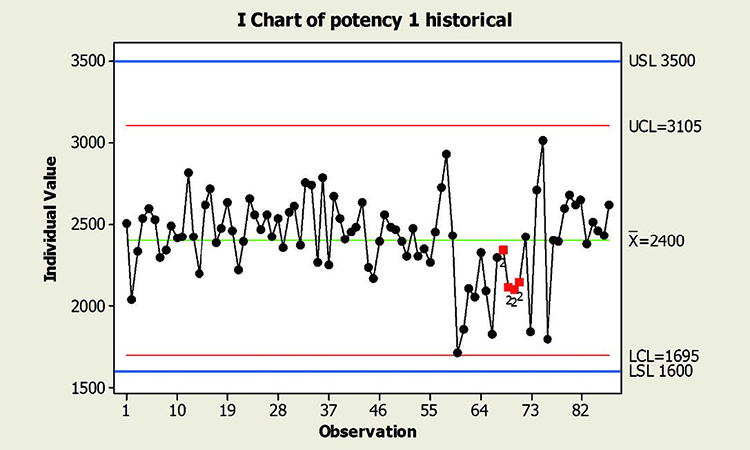
Using control charts in CPV requires a mindset change from typical application and interpretation within the SPC paradigm.
In addition, because this type of behavior has not been observed in the past, this shift is unexpected. And although the process has returned to normal behavior, we cannot know whether the factor that caused the shift will occur again; if it does, the risk of results outside specification is clearly apparent. In this situation, therefore, immediate attention is warranted.
This contrasts with the situation shown in the Potency 2 chart. Not only is the process trending well within specifications, shifts similar to the most recent one have been observed historically. Note that like the shift in assay in Figure 1, patterns identified in the Potency 2 data could indeed provide valuable information about sources of variability, and opportunity for continual improvement, thereby enabling a primary goal of CPV. The urgency for investigation, however, would not rise to the level warranted for the Potency 1 situation.
While process knowledge may certainly be gained through the evaluation of signals, the requirement that they be investigated regardless of the risk to patient and business can result in substantial, misdirected resources. Ultimately, this lack of prioritization serves neither the patient nor the business. Akin to the previous example, some manufacturers have designed decision trees or matrices to incorporate a risk-based approach to statistical signals in control charts and ensure an appropriate level of attention is given to observed signals.
Examples of decision rules include:
- Process capability (e.g., using process performance index)
- Distance of signal from specification
- Distance of signal from mean
- Number of batches affected
- Historical patterns of variability
Signal response is commensurate with the risk and opportunity identified considering those multiple elements, and can vary from a simple acknowledgment at the lowest level to a formal investigation documented within the quality system. The BioPhorum Operations Group CPV and Informatics Team formulated one such risk-based approach to signals, published in the January-February 2017 issue of this magazine. 10
Fearing unnecessary attention to signals and knowing that they are expected, some manufacturers choose to remove these signals from their charts. If the context were real-time SPC requiring rapid process-adjustment decisions, the number of signals may indeed pose a problem. But this does not pose the same risk in CPV, where immediate adjustments or decisions are not typically sought. And if the business process defines a risk-based approach to signals, overanalysis and/or overreaction should not occur.
When special cause variation triggers an appropriate risk-based response, the resulting visible patterns can help achieve CPV goals.
Questionable effectiveness is another reason some manufacturers choose to omit signals. For instance, specific statistical requirements can result in the situation of a shift that is detectable by a keen eye, but does not trigger a signal. The logic for omission continues: If signals are not triggered for all shifts and they can be misinterpreted as a lack of control, why use them at all? Indeed, careful review of charts for excursions and patterns by a process subject matter expert can be more effective than simple reliance on statistical signals. This careful review does not always exist, however, and even when it does the pattern of color from signals can aid interpretation. This is particularly true in early stages of process understanding, due either to the age of the product or the age of the CPV program.
There may indeed be cases where it’s reasonable to omit specific signals, as they provide little benefit or may even be detrimental. This decision should be considered carefully, however, by assessing what knowledge about variability might be forfeited, and recognizing that the biggest value of the charts can be the pattern they reveal. 11 If signals are viewed negatively due to inadequate training of users and reviewers, cumbersome reporting, or overreaction, more benefit may be realized by addressing these deficiencies than by widespread omission.
An additional note regarding both examples: These charts have “Shewhart” control limits derived from the average moving range, thus they reflect short-term standard deviation. In the context of nonrandom variability sources, this estimate tends to be less than the longer-term estimate computed by the typical standard deviation formulation of a sample. For this reason, Shewhart limits may be too narrow to bracket expected total variability, and more values may be expected to be outside the limits compared to the number expected if sources of variability are truly random. Hence, some manufacturers choose to derive limits based on the longer-term estimate of standard deviation. Others maintain the short-term estimate, and recognize this feature in their risk-based response to statistical signals.
Using control charts in CPV requires a mindset change from typical application and interpretation within the SPC paradigm. Indeed, while both paradigms utilize control charts, their intended use and assumed data structure are not the same. Thus, it should be expected that interpretation also differs. Because the fundamental assumption of independence integral to conventional SPC is not met in typical pharmaceutical or biopharmaceutical manufacture, response to variation identified as special cause is unfortunately not a simple application of statistical rules and common definitions.. Ignoring the influence of this assumption and imposing actions as if it had been in fact met is not only statistically inappropriate, it can result in wasted resources, improper focus, lost opportunities, and frustrated employees. None of these outcomes serve either the business or the patient. And while omitting the identification of “expected” special cause variation to avoid too many signals might be important in the context of real time SPC, it can inhibit the CPV goal of continued understanding of process variability.
Control chart interpretation within the context of CPV requires a combination of process knowledge, adequate statistical understanding, and a business process that incorporates them into a risk-based decision framework. When special cause variation triggers an appropriate risk-based response, the resulting visible patterns can be embraced to help achieve the goals of CPV.
Instead of dreading statistical signals in control charts, a new appreciation can be acquired where “red is the new black.”
- 10 Mark DiMartino, et al. “CPV Signal Responses in the Biopharmaceutical Industry.” Pharmaceutical Engineering 37, no. 1 (January-February 2017): 57–64
- 11 Chowdhury, Subir. Design for Six Sigma. Dearborn Trade Publishing, 2002.
Related Articles
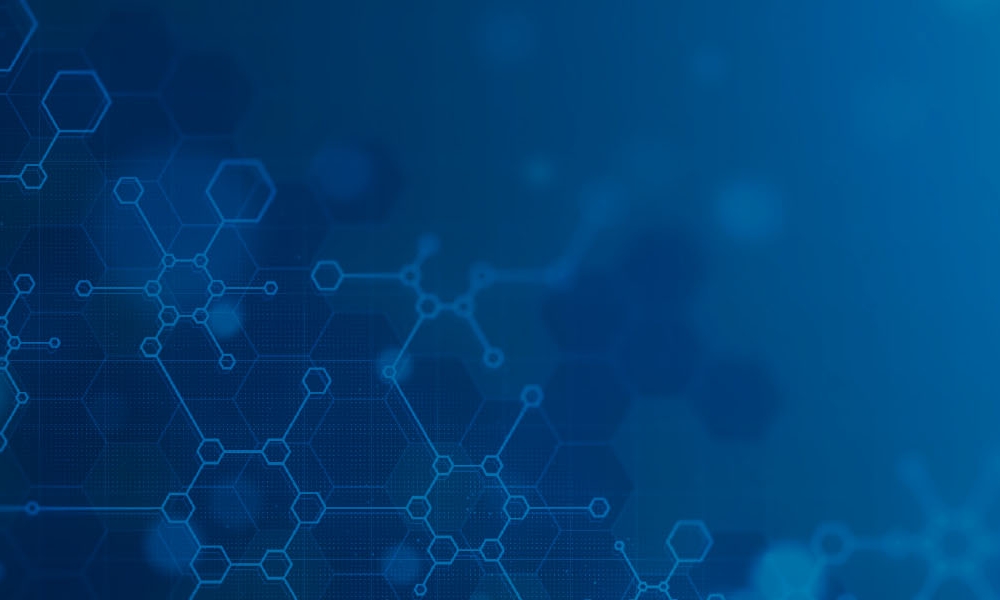
In the dynamic and highly regulated world of biopharmaceutical manufacturing, maintaining and ensuring quality is a critical success factor. An effective quality risk management (QRM) system is a key component in the overall quality management infrastructure of biopharmaceutical organizations. It offers a structured, scientific, and risk-based approach to decision-making, addressing potential...
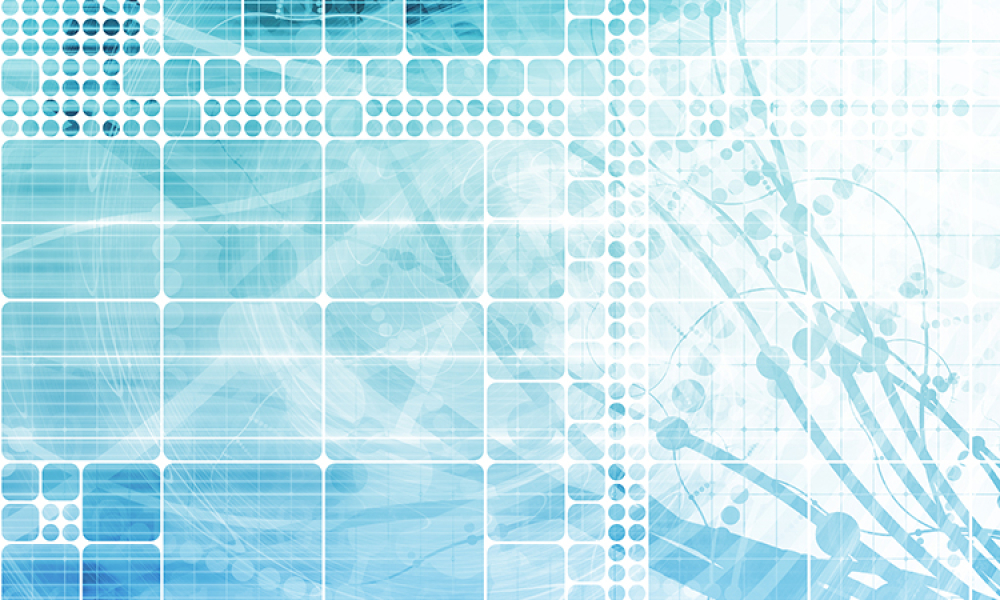
Pharmaceutical manufacturing facilities produce a variety of products, including highly potent products that require safety measures to prevent adverse health effects on patients and operators. To ensure safety, these facilities use containment equipment to minimize the risk of contamination. This article presents criteria for selecting containment equipment, considering both...
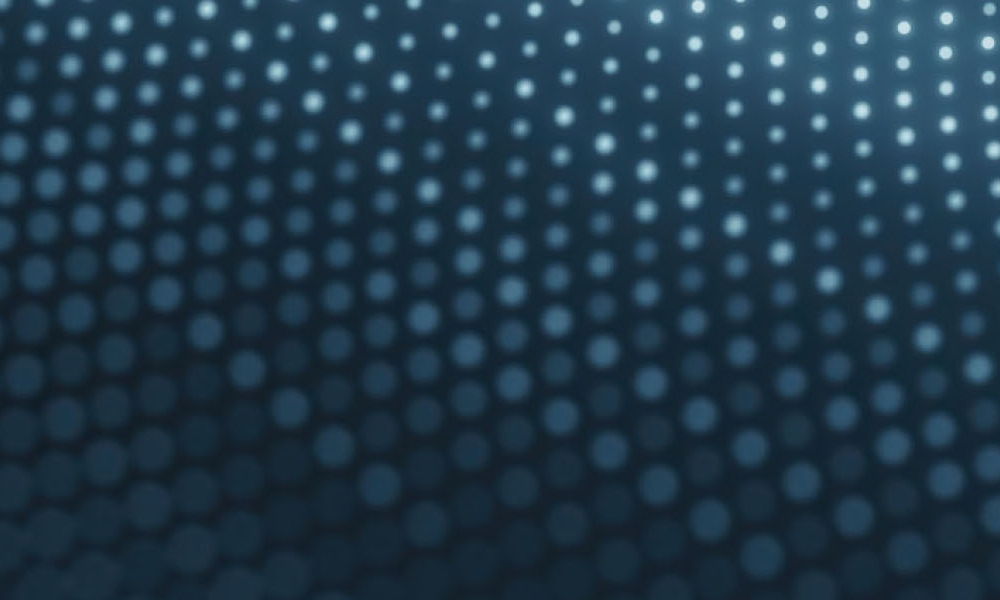
The world is beginning to grasp the huge challenge of achieving net-zero carbon emissions, or carbon neutrality, by 2050. Many countries have committed to achieving this ambitious goal. As a major global industry, the pharmaceutical sector has a significant role to play. For thermal energy–intensive industries, such as pharmaceutical manufacturing, the long-term future options to maintain...

Common Cause and Special Cause

Common Cause and Special Cause in Statistics: Understanding Variability
Statistics is a powerful tool for analyzing data and making informed decisions, but to do so effectively, it’s essential to understand the sources of variability within a process or system.
In the realm of statistical process control , two fundamental concepts come into play: common cause and special cause variation. These concepts help us differentiate between the everyday fluctuations in a process and the exceptional, identifiable factors that can lead to significant deviations from the norm.
Common Cause Variation
Common cause variation, often called random variation or systemic variation, is the inherent variability in any process. It is the everyday, expected variation that occurs when a system is stable and operating under normal conditions.
This type of variation is the result of numerous factors and interactions within a process, and it cannot be traced back to a specific source. Common cause variation is, in a sense, the “background noise” of a process.
Key characteristics of common cause variation include:
- Inherent to the Process: Common cause variation is an inherent part of a process and will always exist to some degree.
- Consistent Patterns: It typically follows consistent, predictable patterns, often resembling a bell-shaped curve (a normal distribution).
- Random and Unpredictable: It is random in nature and cannot be attributed to any specific factor or event. This makes it difficult to control or eliminate entirely.
- Small Fluctuations: Common cause variation results in small, manageable fluctuations around a process’s mean or average value.
Examples of common cause variation can include minor temperature fluctuations in a manufacturing process, small variations in delivery times, or slight variations in the weight of identical products produced on the same assembly line.
Special Cause Variation
Special cause variation, also known as assignable variation or non-random variation, is the opposite of common cause variation. It represents variability in a process that can be traced back to specific, identifiable causes. Unlike common cause variation, which is inherent to the process, special cause variation is due to external factors or events that disrupt the system’s normal functioning.
Key characteristics of special cause variation include:
- Identifiable Causes: Special cause variation can be linked to specific events, actions, or factors that are not part of the usual operation of the process.
- Erratic Patterns: Unlike the consistent patterns of common cause variation, it often exhibits erratic and unpredictable patterns.
- Large Fluctuations: Special cause variation results in significant deviations from a process’s mean or average value.
- Unusual Events: Examples of special cause variation can include equipment breakdowns, power outages, errors in data entry, or major shifts in market demand.
Differentiating Between Common Cause and Special Cause Variation
Distinguishing between common cause and special cause variation is crucial in process improvement and quality control. Understanding the source of variability in a process allows organizations to take appropriate actions.
Here are some guidelines for differentiation:
- Data Analysis: The first step is to collect and analyze data. If the variation observed falls within the expected range of common cause variation, it is likely due to inherent process variability. However, if the data points exhibit patterns or values that deviate significantly from the norm, special cause variation may be present.
- Statistical Tools: Various statistical techniques, such as control charts, can be used to monitor processes and identify abnormal data points that suggest special cause variation. Control charts help in distinguishing between natural process variation and unusual occurrences.
- Root Cause Analysis: When special cause variation is suspected, a thorough root cause analysis is essential. This involves investigating the specific factors that contributed to the variation and taking corrective actions to prevent its recurrence.
- Process Control: Once special cause variation is identified and addressed, process control measures can be put in place to minimize the risk of future occurrences.
How can we Minimize Common Cause Variation?
Minimizing common cause variation is a key goal in statistical process control and quality improvement. While common cause variation is inherent to any process and cannot be completely eliminated, there are several strategies and approaches that can help reduce its impact and maintain greater process stability. Here are some ways to minimize common cause variation:
- A thorough understanding of the process is the first step in minimizing common cause variation. You need to know how the process operates, what factors affect it, and the expected sources of variability.
- Implement process control tools, such as control charts, to continuously monitor the process. Control charts help in distinguishing between common cause and special cause variation. They visually represent the process’s performance over time, making it easier to detect trends or shifts.
- Develop and maintain standardized operating procedures for the process. SOPs ensure that everyone involved follows the same methods and practices, reducing variability in human factors and operational choices.
- Invest in the training and skill development of employees involved in the process. A well-trained workforce is less likely to introduce unnecessary variability through errors or inconsistent practices.
- Regularly maintain and calibrate equipment to minimize common cause variation associated with machinery or tools. Well-maintained equipment is more likely to produce consistent results.
- Use statistical techniques to understand the inherent variability in the process. By analyzing the process’s capability and identifying areas with excessive common cause variation, you can make data-driven decisions to improve it.
- Implement Lean Six Sigma principles to identify and eliminate waste and non-value-added steps in the process. This can help streamline operations and reduce variability.
- Use DOE methodologies to study the impact of various process factors on variability systematically. This approach can help optimize processes and identify which factors have the most significant impact on common cause variation.
- Form cross-functional teams to focus on process improvement. Teams can work together to identify sources of common cause variation, develop solutions, and ensure continuous process optimization.
- Make decisions based on data and evidence rather than intuition. Data-driven decisions allow for a better understanding of the process’s performance and the identification of areas where common cause variation can be reduced.
- Establish feedback loops to ensure that lessons learned from past performance are used to make continuous improvements. Regularly review and update process documentation, procedures, and best practices.
- Compare your process performance to industry benchmarks and best practices. Benchmarking can help identify areas where your process may be underperforming and experiencing excessive common cause variation.
- Encourage employees to provide feedback and suggestions for process improvement. They often have valuable insights into daily operations and can help identify and address common cause variation.
Minimizing common cause variation is an ongoing effort requiring a systematic process improvement approach. Organizations can reduce variability and enhance their processes’ overall quality and performance by consistently monitoring, analyzing, and making data-driven adjustments.
How does Common Cause and Special Cause Apply in Six Sigma Projects
Common cause and special cause variation are fundamental concepts in Six Sigma, a structured and data-driven methodology for process improvement. Understanding these concepts is crucial for identifying, analyzing, and addressing variations within processes to reduce defects and improve overall quality. Here’s how common cause and special cause apply in Six Sigma projects:
- Defining the Problem (Define Phase): In the Define phase of a Six Sigma project, the team identifies the problem that needs to be addressed. At this stage, it’s essential to differentiate between common cause and special cause variation. Common cause variation represents the inherent variability in the process, while special cause variation signifies exceptional factors causing deviations from the norm. This distinction helps in setting realistic improvement goals and understanding the scope of the project.
- Data Collection and Analysis (Measure Phase): The Measure phase involves collecting data to quantify the performance of the process and determine its capability. Six Sigma practitioners use statistical tools and control charts to identify patterns in the data. Control charts help distinguish between common cause and special cause variation. Common cause variation is typically represented by data points within control limits, while data points beyond these limits suggest special cause variation.
- Root Cause Analysis (Analyze Phase): Once special cause variation is identified in the Measure phase, the Analyze phase focuses on determining the specific causes of these exceptional variations. Root cause analysis techniques, such as the “5 Whys,” Fishbone diagrams, or Failure Modes and Effects Analysis (FMEA), are employed to understand the underlying factors responsible for special cause variation. Addressing these root causes is critical for process improvement.
- Improvement Actions (Improve Phase): In the Improve phase , the Six Sigma team devises and implements solutions to eliminate or mitigate the root causes of special cause variation. Improvement actions are carefully planned, tested, and validated to ensure that the process becomes more stable and predictable.
- Monitoring and Control (Control Phase): The Control phase is about sustaining the improvements made during the project. Common cause variation is continuously monitored through control charts, and process performance is measured against the new standards. The control plan, established in this phase, ensures that the process remains in control and that deviations due to common cause variation are promptly identified and addressed.
- Continuous Improvement: Six Sigma is inherently focused on continuous improvement. Common cause variation is always present but can be further reduced and managed through ongoing efforts. Teams conduct periodic reviews and data analysis to detect changes in process performance and address any new sources of special cause variation.
Six Sigma projects involve a structured approach to addressing both common cause and special cause variation. While common cause variation represents the natural variability in a process, special cause variation results from specific, identifiable issues.
A Six Sigma project aims to minimize both types of variation to improve process performance and quality. This requires a combination of data analysis, root cause analysis, process improvement efforts, and ongoing monitoring to ensure that the improvements are sustained over time.
In the world of statistics and quality control, understanding the concepts of common cause and special cause variation is vital for making informed decisions and improving processes. Common cause variation is the inherent, expected variation in a process, while special cause variation represents unusual and identifiable sources of variability. By distinguishing between these two types of variation, organizations can work towards greater process stability, predictability, and overall quality improvement.
Six Sigma Certifications
Six Sigma Resource Center
Common Cause and Special Cause Data (.pdf)
- All Certifications
- Accessibility

Connect With Us
Copyright MSI. All Rights Reserved.

- Quality Improvement Zone
- Quality Improvement journey
- Measurement
Understanding variation
This page explores what variation is, and why we need to understand it. We'll look at types of variation, how to look at variation, Statistical Process Control (SPC) charts and reacting to variation.
Leaders in the science of improvement should speak the language of variation. Knowledge about separating variation of outcomes of a process or system in to common and special causes helps to decide appropriate actions for that process or system. Inappropriate action may make things worse.
The Improvement Guide (Langley et al, page 81)
Measurements of all outcomes and processes will vary over time. Some variation is intentional, for example varying services for people depending on their needs. In contrast, unintended variation is due to differences not connected with different needs. For example we would want everyone to have a good experience. Unintended variation results in poor quality, waste and harm. This often forms the focus for improvement.
Often we look at data aggregated over long time periods, such as by year or by quarter. This hides the variation in the data. Measuring more often (hourly, daily, weekly or monthly) can be more informative. A central aspect of improvement science is looking at charts of data over time. Run charts and SPC charts can help us understand variation and decide whether a change is an improvement.
To tell whether a process or its outcome is improving, it is important to understand changes in the data. We need to understand whether these changes are due to actions we've taken, or due to random chance. Making decisions without understanding the causes of variation can make things worse.
The two types of variation defined by Shewhart and Deming are:
Common cause - those causes that are inherent in the system over time. Causes that affect everyone working in the system, and affect all outcomes of the system. These include random variation.
Special cause - those non-random causes that are not part of the system all the time. Causes that do not affect everyone, but arise because of specific circumstances.
SPC charts are a good way to separate common cause and special cause variation.
Run charts can signal whether the variation present is exhibiting random or non-random patterns. It cannot signal whether these are special or common cause. If there are non-random signals in your run charts, it is likely that special cause variation is present.
SPC charts minimise the chance of making either of two possible mistakes that can make things worse rather than better:
1. Inappropriately reacting to common cause variation, as if it were due to a special cause. This "tampering" may exacerbate the variation. If the system is stable and only common cause is present, but not working at an acceptable level, then it requires fundamental change. Reacting to individual data points is not helpful.
2. Inappropriately ignoring special cause variation, treating it as if it were common cause. We need to remove special cause variation to get stability and predictability. This will then give us the ability to understand the effect of any deliberate changes.
Process capability is about predicting what the future values of a measure are likely to be. The predicted range can be compared with the desired range to see if the process is capable of meeting its specifications. SPC methods help us to work out process capability. For information about how to calculate capability consult the Healthcare Data Guide.
A process could be stable and only showing common cause variation, but not capable. We would need to make a fundamental change if we wish to see an improvement. If we keep on doing what we've always done, we'll keep on getting what we've always got.
If we also detect special causes we need to investigate what might be happening and learn from them. Not all special cause variation is bad. If we notice a special cause which shows good performance we could consider testing it as a change to the process.
If we want to make a process better by changing something, we are introducing a special cause. If we notice special cause variation causing problems in how things work, we'll want to get rid of them.
Measurement plan
A measurement plan sets out details for each measure proposed for an improvement project.
A run chart is a line graph of data plotted over time. By collecting and charting data over time, you can find trends or patterns in the process.
SPC charts
Statistical Process Control (SPC) Charts are simple graphical tools that enable process performance monitoring.
Funnel plot
A funnel plot is a chart that helps to understand variation within a system.
Scatter plot
A graph in which the values of two variables are plotted along two axes, the pattern of the resulting points revealing any correlation present.
Pareto chart
A Pareto Chart is a tool to help you understand your system.
Histogram
A histogram is a plot that lets you discover, and show, the underlying frequency distribution (shape) of a set of continuous data.
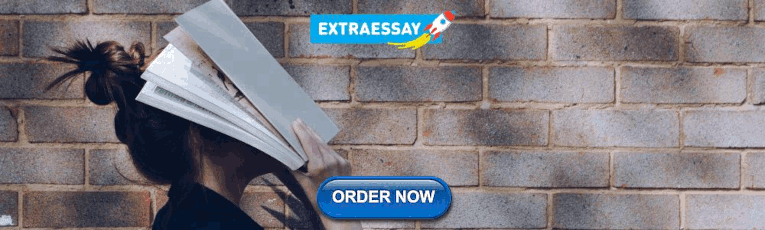
IMAGES
VIDEO
COMMENTS
A control chart can show two different types of variation: common cause variation (random variation from the various process components) and special cause variation. Special cause variation is present when the control chart of a process measure shows either plotted point(s) outside the control limits or a non-random pattern of variation. When a ...
Common and special causes are the two distinct origins of variation in a process, as defined in the statistical thinking and methods of Walter A. Shewhart and W. Edwards Deming. Briefly, "common causes", also called natural patterns, are the usual, historical, quantifiable variation in a system, while "special causes" are unusual, not ...
Common Cause Variation. Common Cause Variation, also referred to as "Natural Problems, "Noise," and "Random Cause" was a term coined by Harry Alpert in 1947. Common causes of variance are the usual quantifiable and historical variations in a system that are natural. Though variance is a problem, it is an inherent part of a process ...
Common cause variations are those causes that are inherent in the system or process. 4 Evidence of common cause variation can be seen visually in the OA's Fig 3, from January 2017 to October 2017, because the data points vary around the mean but remain between the upper and lower control limits (dotted lines). In contrast, special cause variations are causes of variations that are not ...
In answering that question, it becomes crucial to understand the difference between common-cause and special-cause variation (as will be discussed later). Common-cause variation appears as random variation in all measures from healthcare processes. 1 Special-cause variation appears as the effect of causes outside the core processes of the work ...
Common causes lead to random scattering of data points around the mean. On the other hand, special causes produce data points that stand out from the normal pattern of variation and signal that the process is out of control. ... Special cause variation is often difficult to detect without the right analysis tools. Identifying special causes ...
Special-cause variation is an unpredictable deviation resulting from a cause that is not an intrinsic part of a process. By careful and systematic measurement, it is easier to detect changes that are not random variation. The approach to managing variation depends on the priorities and perspectives of the improvement leader and the intended ...
If the data appear random and are contained within the control limits, the variation is likely common cause variation. ... Special cause variation in this system could occur if a machine fails, the truck used to transfer jobs moves more slowly, or an unexperienced operator works in this system. Human operators will try to make decisions and ...
Common-cause variation appears as random variation in all measures from healthcare processes.1 Special-cause variation appears as the effect of causes outside the core processes of the work. Management can reduce this variation by enabling the easy recognition of special-cause variation and by changing healthcare processes—by supporting the ...
Staff — January 4, 2013. Common-cause variation is where no one, or combination of factors is unduly affected the process variation (random variation). Special-cause variation is when one or more factors are affecting the process variation in a non-random way. With special-cause variation, one should be able to identify, or put their finger ...
Here are some common patterns which indicate non-random (Special Cause) Variation. A Sigma is a Standard Deviation. Trend: 6 consecutively increasing or 6 consecutively decreasing points; Shift in the Mean: 8 consecutive points on the same side of the Center Line;
Special cause variation is present in an unstable process. Whenever a process manager seeks to control a process, he or she needs to separate the variation into the appropriate categories so that appropriate actions can be taken. ... 01:48 Let me clarify the nature of randomness of common cause variation. 01:52 It is random with respect to any ...
The other term used to describe this variation is Natural Problems, Noise, or Random Cause. Common cause variance could be presented and analysed using histogram. What is Common Cause Variation. There are several distinguishable characteristics of common cause variation. Firstly, the variation pattern is predictable. ... Special Cause Variation ...
Common causes are also called natural causes, noise, non-assignable and random causes. Special cause variation, on the other hand, is the unexpected variation in the process. There is a specific cause that can be assigned to the variation. For that reason, this is also called as the assignable cause. You are required to take action to address ...
When a process is in a state of control and sources of variability have random influence across time, points on the control chart should have a random pattern.4, 8 Evidence of special cause variation can be an unexpected event, such as a single point outside of a control limit or a pattern not expected by random chance. In commonly used ...
Common cause variation, often called random variation or systemic variation, is the inherent variability in any process. It is the everyday, expected variation that occurs when a system is stable and operating under normal conditions. ... Special cause variation, also known as assignable variation or non-random variation, is the opposite of ...
It cannot signal whether these are special or common cause. If there are non-random signals in your run charts, it is likely that special cause variation is present. SPC charts minimise the chance of making either of two possible mistakes that can make things worse rather than better: 1. Inappropriately reacting to common cause variation, as if ...
Quality Glossary Definition: Variation. The Law of Variation is defined as the difference between an ideal and an actual situation. Variation or variability is most often encountered as a change in data, expected outcomes, or slight changes in production quality. Variation usually occurs in four separate areas: Special causes. Common causes.
[Federal Register Volume 89, Number 91 (Thursday, May 9, 2024)] [Rules and Regulations] [Pages 40066-40195] From the Federal Register Online via the Government Publishing Office [www.gpo.gov] [FR Doc No: 2024-09237] [[Page 40065]] Vol. 89 Thursday, No. 91 May 9, 2024 Part IV Department of Health and Human Services ----- 45 CFR Part 84 Nondiscrimination on the Basis of Disability in Programs or ...