Accessibility Links
- Skip to content
- Skip to search IOPscience
- Skip to Journals list
- Accessibility help
- Accessibility Help
Click here to close this panel.
Purpose-led Publishing is a coalition of three not-for-profit publishers in the field of physical sciences: AIP Publishing, the American Physical Society and IOP Publishing.
Together, as publishers that will always put purpose above profit, we have defined a set of industry standards that underpin high-quality, ethical scholarly communications.
We are proudly declaring that science is our only shareholder.
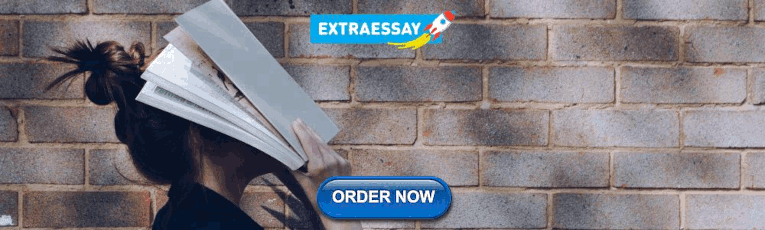
Sentiment analysis and prediction of Indian stock market amid Covid-19 pandemic
Chetan Gondaliya 1 , Ajay Patel 1 and Tirthank Shah 2
Published under licence by IOP Publishing Ltd IOP Conference Series: Materials Science and Engineering , Volume 1020 , 6th International Conference on Computers Management & Mathematical Sciences (ICCM 2020) 22nd-23rd November 2020, Nirjuli, India Citation Chetan Gondaliya et al 2021 IOP Conf. Ser.: Mater. Sci. Eng. 1020 012023 DOI 10.1088/1757-899X/1020/1/012023
Article metrics
5167 Total downloads
Share this article
Author e-mails.
Author affiliations
1 AMPICS, Ganpat University, Mehsana, Gujarat
2 Institute of Management, Nirma University, Ahmedabad, Gujarat
Buy this article in print
Outbreak and spread of the Covid-19 pandemic have touched to the core of our sentiments. Indian stock market has seen a roller coaster ride so far this year amid the Covid-19 pandemic. Sentiments have turned out to be a significant influence on the movement of the Indian stock market and pandemic has only added more steam. This study with the limelight on the Covid-19 pandemic is an endeavour to investigate the classification accuracy of selected ML algorithms under natural language processing for sentiment analysis and prediction for the Indian stock market. The study proposes the framework for sentiment analysis and prediction for the Indian stock market where six ML algorithms are put to test. Consequently, the study highlights the superior algorithms based on accuracy results. These superior algorithms can be potent input to build robust prediction models as a logical next step.
Export citation and abstract BibTeX RIS
Content from this work may be used under the terms of the Creative Commons Attribution 3.0 licence . Any further distribution of this work must maintain attribution to the author(s) and the title of the work, journal citation and DOI.
Testing the market efficiency in Indian stock market: evidence from Bombay Stock Exchange broad market indices
Journal of Economics, Finance and Administrative Science
ISSN : 2218-0648
Article publication date: 5 April 2022
Issue publication date: 13 December 2022
Despite volumes of research on the efficient market hypothesis (EMH) over the last six decades, the results are inconclusive as some studies supported the hypothesis, and some studies rejected it. The study aims to examine the market efficiency of the Indian stock market.
Design/methodology/approach
For analysis, nine Bombay Stock Exchange (BSE) broad market indices were selected covering the study period from 01 January 2011 to 31 December 2020. The data collected for this study are daily open, high, low and closing prices of selected indices. The tools used in this study are: (1) unit root test to check the stationarity of time series, (2) descriptive statistics, (3) autocorrelation and (4) runs test.
The empirical findings of the study reveal that BSE broad market indices do not follow a random walk and Indian stock market is as weak-form inefficient.
Research limitations/implications
The findings from this study provide several avenues for future research. One of the research implications is that anomalies in the statistical results by different academicians in the finance area need to be explained by future researchers.
Practical implications
Investment companies need to understand that extraordinary skills are required to beat the market to make abnormal returns. In an inefficient market where securities do not reflect the complete available information, it is challenging for the investment brokers to convince the customers about the portfolios they recommend to the public that the rate of return would be more than expected.
Social implications
As economic growth is related to the growth in the financial sector, developing countries like India depend on the accuracy of the information. In the presence of asymmetric information, the fluctuations in the stock market would have serious harmful consequences on the economy.
Originality/value
Amid several controversies surrounding the EMH testing, this study is a modest attempt to provide evidence that the Indian stock market is in weak-form inefficient. However, it is essential to link investors' behaviour and trends observed in the financial sector to fully understand the implications of EMH.
- Efficient market hypothesis
- Autocorrelation
- Indian stock market
Elangovan, R. , Irudayasamy, F.G. and Parayitam, S. (2022), "Testing the market efficiency in Indian stock market: evidence from Bombay Stock Exchange broad market indices", Journal of Economics, Finance and Administrative Science , Vol. 27 No. 54, pp. 313-327. https://doi.org/10.1108/JEFAS-04-2021-0040
Emerald Publishing Limited
Copyright © 2022, Rajesh Elangovan, Francis Gnanasekar Irudayasamy and Satyanarayana Parayitam
Published in Journal of Economics, Finance and Administrative Science . Published by Emerald Publishing Limited. This article is published under the Creative Commons Attribution (CC BY 4.0) licence. Anyone may reproduce, distribute, translate and create derivative works of this article (for both commercial and non-commercial purposes), subject to full attribution to the original publication and authors. The full terms of this licence maybe seen at http://creativecommons.org/licences/by/4.0/legalcode
1. Introduction
The efficient market hypothesis (EMH) postulates that an efficient market represents that all marketable securities and assets reflect newly released information immediately so that it is impossible to beat the market by using information and making extraordinary profits. The basic tenet of EMH is that stock returns are random in an efficient market. Second, investors cannot earn excess profits in an efficient market.
There has been a plethora of research on testing the EMH for over eight decades ( Brown, 2020 ; Chan et al. , 1997 ; Degutis and Novickytė, 2014 ; Fama, 1970 ; Jain et al. , 2020 ; Kelikume et al. , 2020 ; Patel et al. , 2018 ; Samsa, 2021 ; Shiller, 2003 ; Vidya, 2018 ). In addition, extensive academic research on EMH documented consensus that no one can make money by trading securities when the markets are efficient. However, the EMH assumes that capital markets come to equilibrium because the market is dominated by “informed and rational agents,” which may not be accurate. Therefore, there are many situations where EMH fails. That is the reason why several scholars time and again attempt to test EMH and see whether the market is efficient in weak, semi-strong and strong forms. Our study also takes the same path and attempt to test the EMH.
We are interested in studying EMH in the context of India because of the following reasons. First, extant research documented that the results were inconclusive, as some studies supported, and most studies did not support random walk theory. In this study, we attempt to examine the market efficiency in the Indian market during the decade 2010–2020, a period characterized by remarkable growth in the Indian economy because of increasing privatization and technological advancements. With the technology change, it is assumed that information is readily available to the investors to accurately predict the stock prices based on the available information immediately. It is also believed that stock prices reflect such information. So, the rationale for this study stems from the changing financial market, changing investors' behaviour, technological developments and the incredible rate of economic growth in India.
The second reason for this study is, in the world of availability of complete information, there is no reason why EMH should fail because the present-day prices reflect all the available information. So then, what are the reasons why EMH fails? Scholars in behavioural finance (e.g. Malkiel, 2003 ) argue that security prices reflect the behavioural intentions of investors. The third reason why we want to test EMH in India is that during the decade of 2010–2020, several economic reforms took place, and the Indian economy was characterized by phenomenon growth, though recession occurred for a brief period (around 2008–2009 because of the crash of real estate bubble in Western countries). Finally, we selected the Bombay Stock Exchange (BSE) in our study because of several reasons. First, BSE was established in 1875 and is the oldest stock exchange in Asia. Second, located in Dalal Street of Mumbai, BSE represents the financial capital of India. Third, BSE is the fastest, with a speed of around six microseconds, in the world accounting for speedy transactions. So, with these novel ideas, this study is conducted. In this paper, in the literature review, we explain the evolution of EMH and present the modern approach of EMH, followed by testing the EMH in the context of the Indian Stock market.
2. Literature review
After the formal introduction of EMH in the 1970s, Grossman (1976) has outlined the market efficiency paradox because of inconclusive results. The basic argument in the paradox is that the greater the belief in market efficiency by investors, the lesser the market efficiency. The intuitive logic behind this inefficiency is that if the investors believe that market is efficient, they will become lazy to collect information, which leads to market inefficiency. Though EMH has remained one of the most controversial research areas in finance and economics, some researchers argue that theory can be considered “half-true” ( Shiller, 2003 ), while the line of argument is that today's stock prices cannot determine the stock prices tomorrow because of volatility and information asymmetry. Therefore, contemporary researchers focus on analysing the stock prices in the presence of (vs absence) of information and adjusting for the announcements (such as mergers, amalgamations, stock splits) ( Jain et al. , 2020 ; Parthasarathy, 2016 ). In one of the studies, Fama and French (1988) argued that risk taken depends less on the company size and market return. Recently, some researchers focused on the “value effect” and documented that return was not necessarily associated with the amount of risk taken. Amid arguments in favour of EMH and contradictions against EMH, we test the hypothesis in the context of the Indian stock market in this study. As explained before, three forms of market efficiency were weak, semi-strong and strong. A market is said to be efficient when investors can predict future market values of individual securities based on the information available freely to all rational participants. The weak form represents a random walk where one cannot make profits by investing in the stocks where prices reflect perfect information. In the semi-strong form, the prices of financial assets reflect the available information in the market; but, in addition, prices change rapidly without any bias to new public information. The operational implication is that in semi-strong form, the investor cannot split the stock to obtain profitability significantly higher than they can achieve in a randomized portfolio of assets ( Samsa, 2021 ).
There are several studies conducted in the Indian context. For want of space, we mention only a few for reference. In the latest study by Jain et al. (2020) conducted on the efficiency of the stock market in India, from April 2010 to March 2019, based on BSE and National Stock Exchange, it was revealed that the Indian stock market is weak-form inefficient and therefore can be outperformed. In another study by Vidya (2018) , it was found that changes in stock market prices are random, and the market is efficient in the weak form. By performing runs test, Patel et al. (2018) found that from April 2015 to March 2018, the three-year daily closing points reveal that market is not efficient to adjust rapidly to the news, thus allowing the investors to outperform, especially when they keep in touch with the changes.
The objective of the study is to demonstrate whether the Indian stock market is weak-form efficient empirically. We determine this by examining whether the return series is stationary or not and identifying whether markets follow a normal distribution and by the descriptive statistics for BSE broad market indices for the return series. In this study, we have the following hypotheses.
The return series of BSE broad market indices are normally distributed.
There is no stationarity in the return series of BSE broad market indices.
The BSE broad market indices follow a random walk.
3.1 Research design
To examine the weak-form market efficiency in the Indian stock market, we have chosen the BSE broad market indices. As of 25 March 2021, BSE has 19 broad market indices and out of which nine indices were selected in this study covering the study period from 01 January 2011 to 31 December 2020. The selected indices are Standard and Poor Bombay Stock Exchange Sensitivity Index (S&P BSE SENSEX), Standard and Poor Bombay Stock Exchange All Capitalization (S&P BSE AllCap), S&P BSE 100, S&P BSE 200, S&P BSE 500, Standard and Poor Bombay Stock Exchange Large Market Capitalization (S&P BSE LargeCap), Standard and Poor Bombay Stock Exchange Mid-Capitalization (S&P BSE MidCap), S&P BSE SENSEX Next 50 and S&P BSE SmallCap. We selected nine indices because only these nine indices are available, and the information about the other ten indices is not available.
3.2 Data collection
The data collected for this empirical study are daily open, high, low and closing prices of BSE broad market indices. Instead of using the closing price itself, the researcher used the average of these four prices. The logic behind the average values of these four prices is that volatility in changes in prices is controlled to some extent. While prior researchers have used only closing prices, assuming trading is done at the closing price, Lodha and Sora (2015) recommend using the average of the four prices to nullify the fluctuations, and volatility is controlled at least partially. All the data have been collected from the official website of the BSE.
3.3 Analytical procedure
The tools used in this study are: (1) unit root test [The Augmented Dickey–Fuller [(ADF)] test to check the stationarity of time series, (2) descriptive statistics (average monthly returns, maximum, minimum, standard deviation, skewness, kurtosis and Jarque–Bera Test), (3) autocorrelation (measuring the linear relationship between lagged values of a time series) and (4) runs test (to check whether observations vary around a constant mean, have constant variance and are probabilistically independent). To calculate the daily returns, we used the formula [(LN (Today closing price/yesterday closing price) × 100]. These tools have been used by several researchers in the past ( Degutis and Novickytė, 2014 ; Harshita et al. , 2018 ; Titan, 2015 ). The procedures and techniques used in this study are consistent with the earlier research in the literature.
The ADF statistics used in the test should be a negative number, and the more the negative number, the stronger the rejection of the null hypothesis that there is a unit root. The runs test, a non-parametric test, is concerned with the price changes rather than the magnitude of price changes. It just considers whether the series consists of increasing values or decreasing values. The null hypothesis of the runs test is that the data set is from a random process.
First, we examined the descriptive statistics. Table 1 captures the results of descriptive statistics for BSE broad market indices. For the time-series data, it is essential to check the normality of the data which can be found by observing the descriptive statistics. Therefore, we did a preliminary analysis of the descriptive statistics, namely, mean, standard deviation, variance, minimum, maximum, skewness and kurtosis. The mean of BSE SENSEX and BSE 200 shows the maximum mean return of (0.0340). In order that the distribution is normal, the condition is that both skewness and kurtosis must be equal to 0 and 3, respectively. As can be seen from Table 1 , the value of skewness of the returns was found to be negative for all indices and therefore the distribution of the daily returns was asymmetrical. The value of Kurtosis is greater than 3 (which represents Leptokurtic distribution) for all the indices. Based on these descriptive statistics, we reject H01 and conclude that the distribution of returns is not normal.
To test the stationarity in the BSE broad market indices, an ADF test was conducted. Table 2 displays the results of the ADF test. The ADF test statistic values of intercept are less than critical values at a 1% significance level. Hence, H02 was rejected and concluded that there is no stationarity in the return series of BSE broad market indices. These results show that data has exhibited stationarity.
4.1 Autocorrelation
There are 16 lag periods associated with autocorrelation for all the indices (in Tables 3–11 ).
The results of autocorrelation for S&P BSE SENSEX are presented in Table 3 .
The first lag depicts an autocorrelation of 0.262 ( Q -statistic = 170.33, p < 0.05), suggesting that the stock market in India does not follow a random walk. It is also interesting to note that the autocorrelation values for the lags 6, 10, 11, 13, 15 and 16 were negative ( p < 0.05) and these results support that stock returns are not random.
The results of autocorrelation for S&P BSE AllCap are shown in Table 4 .
As shown in Table 4 , the first lag shows an autocorrelation of 0.182 ( Q -statistic = 81.897, p < 0.05) which suggests that stock returns in the Indian stock market do not follow a random walk. Further, the lags 6, 11, 13, 15 and 16 showed negative autocorrelations ( p < 0.05) corroborating that the stock returns are not random.
The results of autocorrelation for S&P BSE 100 are exhibited in Table 5 .
As can be seen in Table 5 , there are 16 lag periods related to the autocorrelation test. The first lag depicts an autocorrelation of 0.286 ( Q -statistic = 203.17, p < 0.05), and the lags 6, 11, 13, 15 and 16 had negative autocorrelations ( p < 0.05). These results suggest that stock returns on the Indian stock market are not random.
Table 6 shows the results of autocorrelation for S&P BSE 200.
As presented in Table 6 , there are 16 lag periods associated with the autocorrelation test. The first lag depicts an autocorrelation of 0.296 ( Q -statistic = 216.61, p < 0.05), and the lags 6, 11, 13, 15 and 16 had negative autocorrelations ( p < 0.05). These results indicate that stock returns in the Indian stock market are not random.
The results of autocorrelation for S&P BSE 500 are presented in Table 7 .
As can be seen in Table 7 , the first lag depicts an autocorrelation of 0.310 ( Q -statistic = 238.73, p < 0.05), and the lags 6,11,13,15 and 16 showed negative autocorrelations ( p < 0.05). These results corroborate that stock returns in the Indian stock market do not follow a random walk.
The results of the autocorrelation test for S&P BSE LargeCap are detailed in Table 8 .
As detailed in Table 8 , the first lag depicts an autocorrelation of 0.146 ( Q -statistic = 52.786, p < 0.05), and the lags 6, 11, 13, 15 and 16 had negative autocorrelations ( p < 0.05). These results indicate that the stock returns of the Indian stock market do not follow a random walk.
The results of the autocorrelation test for S&P BSE MidCap are presented in Table 9 .
As shown in Table 9 , there are 16 lag periods related to the autocorrelation test. The first lag depicts an autocorrelation of 0.299 ( Q -statistic = 221.42, p < 0.05), and the lags 6, 13, 14 and 15 had negative autocorrelations ( p < 0.05), thus documenting that stock returns in the Indian stock market are not random.
The results of the autocorrelation test for S&P BSE SENSEX NEXT50 are presented in Table 10 .
As can be seen in Table 10 , the first lag depicts an autocorrelation of 0.181 ( Q -statistic = 81.107, p < 0.05), and the lags 4, 5, 6, 11, 13, 15 and 16 all showed negative autocorrelations ( p < 0.05). These results reveal that stock returns on the Indian stock market are not random.
Table 11 shows the results of the autocorrelation test for S&P BSE SmallCap.
As Table 11 details, the first lag depicts an autocorrelation of 0.373 ( Q -statistic = 345.56, p < 0.05), and the lags, 13, 14 and 15 showed negative autocorrelations ( p < 0.05). The results document that stock returns on the Indian stock market are not random.
The results of runs test for BSE broad market indices are exhibited in Table 12 .
As shown in Table 12 , the Z value negative for all the BSE broad market indices and found that the critical value of Z for 95% level of confidence is ± 1.96. Hence, the null hypothesis, i.e. BSE broad market indices follow a random walk is rejected at a 5% level of significance. Moreover, the p -value is also 0.000 which is less than the alpha (0.05), and therefore, the null hypothesis is rejected. The runs test of this study indicates that BSE broad market indices do not follow a random walk and it is evident that the Indian stock market is in weak-form inefficient.
5. Discussion
The objective of this study is to test the EMH in the Indian stock market. The rationale for this study stems from the fact that though several studies on EMH were conducted in India, the results were mixed. This prompted us to study the EMH, especially during the last decade (2010–2020), a period characterized by unprecedented economic changes, and the Indian economy has been on the growth track. Our results were consistent with most of the previous studies. Our results are fascinating because even when much information is available because of technological improvements and transparency in the data, the EMH does not hold good.
First, the results indicate that the value of skewness returns distribution was negative for all indices. It is documented that the distribution of the daily returns is asymmetrical nature. The value of Kurtosis is greater than 3, representing Leptokurtic distribution for all the indices. The distribution is, therefore, was not normally distributed ( H01 ). The autocorrelation test reveals that the stock returns of the Indian stock market do not follow a random walk for all the indices. Second, the findings from this study indicated that the ADF test statistic values of intercept are less than critical values at a 1% significance level. Hence, the null hypothesis is rejected, and the results reveal that data has exhibited stationarity in nature ( H02 ). When the data are non-stationary, the time series contains unit root implying that the mean, variance and covariance are not constant over time. The ADF statistic contains significant negative values and corroborates that the series does not contain a unit root. If data contains the unit root, the data follows a random walk. The results from this study indicate that the unit root is not present, and the data showed stationarity. Finally, the runs test of this study indicates that BSE broad market indices do not follow a random walk, and it is evident that the Indian stock market is as weak-form inefficient ( H03 ). To sum, the results from our study do not support EMH as evidenced by critics of the theory. Asymmetrical distribution, Leptokurtic distribution and the runs test result show that BSE broad market indices do not follow a random walk. Therefore, the Indian stock market is weak-form inefficient.
5.1 Theoretical implications
The study has several theoretical implications. First, the results add to the growing body of literature on EMH, and the results are consistent with most of the earlier studies. Second, though some studies in the past have shown different and opposite effects (see Tables 1 and 2 ), our study provides a fresh look at the EMH during a decade of 2010–2020. Most importantly, the results from this study corroborate the findings conducted a decade back that tested the weak form of market efficiency of stock market returns in 14 countries (Pakistan, India, Sri Lanka, China, Korea, Hong Kong, Indonesia, Malaysia, Philippines, Singapore, Thailand, Taiwan, Japan and Australia), which documented that stock prices do not follow random walks in all countries of Asian-Pacific region ( Hamid et al. , 2010 ). Therefore, investors in these countries employed strategies of the arbitrage process to enjoy the stream of benefits. Thus, our results are consistent with the previous studies and vouch for the failure of the EMH hypothesis.
Third, from the theoretical standpoint, the results suggest diagnosing the plausible reasons for the failure of EMH in the Indian context, besides all other countries in the Asia–Pacific region. We argue that two fundamental reasons for the failure of EMH in the Indian context: the unpredictability of investors' behaviour and uncertainty about the investment. Many studies in the Western countries and the Middle East have concluded that the market should be seen in only relative terms. There is consensus among academicians that EMH fails to explain the excess volatility in security prices, unexpected bubbles in the stock market, irrational customers and overreactive behaviour. While analysing market efficiency, academicians need to consider the dynamics of changing markets that may tilt the results and vouch for EMH failure.
5.2 Practical implications
The research findings of this study have several implications for the stakeholders, including the stockbrokers and investors. First, the results reported in this study portrayed that the stock market in India exhibited the weak-form inefficient; the investment brokers and investors should exercise caution before selecting their investment portfolios. In India, being a thickly populated developing country, there is a wide variety of investors with varying financial requirements. Some investors seek a long-term return on their investment, whereas some prefer to have secured returns every month. Some investors are risk-takers, whereas some are risk-avoiders. Since people with different portfolio requirements influence the stock market, behavioural finance scholars suggest examining the effect of personality factors on investment behaviour. For example, some researchers documented that the personality characteristics of individuals have a significant impact on investment behaviour ( Isidore and Christie, 2017 ; Sadiq and Khan, 2019 ). Second, financial literacy plays a vital role in investment decisions. Therefore, in addition to the technical analysis of the stock market, the analysts need to consider the level of financial literacy, information access, subjective financial knowledge, risk propensity, etc., that may profoundly impact investor behaviour. As provided in this study, the results from stock market analysis guide the investors in making decisions and not getting lured by false promises of stockbrokers ( Aren and Aydemir, 2015 ; Barber et al. , 2021 ).
The findings from the study help investors to make correct investment decisions. The effectiveness of investment decisions largely depends on market efficiency and the investors' financial knowledge and various investment opportunities with varying degrees of return on investment. Although theoretically, rational decision-making considers identifying multiple available alternatives and choosing suitable options, it is not possible in reality because of information asymmetry and market anomalies ( Sitkin and Weingart, 1995 ), and investors make decisions based on the available information. Therefore, failure to consider the market anomalies may result in flawed decision-making. Moreover, in the present-day technological sophistication where information is readily available from multiple sources, it is expected that investors will have complete information. Despite this, the reasons for the failure of EMH need further investigation. Investors diversify their investments based on their financial knowledge, and available empirical evidence shows that increased financial knowledge influences financial management attitudes, resulting in healthy financial behaviours ( Borden et al. , 2008 ).
5.3 Future research agenda
This study offers several avenues for future research. The results from this research vouch against the EMH and call for further studies to identify the reasons for the rejection of EMH. Though it was documented that in Asian countries, stock markets failed to support EMH, and the researchers explained the possible causes for lack of support for EMH, the information was not adequately explained. During this study period (2011–2020), the market did not exhibit high volatility, and it is suggested to identify the reasons for not supporting the well-established random walk theory. As we omitted 11 indices, future researchers can focus on those indices to see if the results from the study can be replicated. Second, future research can focus on liquidity's role in asset pricing in the stock market ( Miralles-Quirós et al. , 2017 ) and see how liquidity affects the EMH in the Indian stock market. Third, sometimes the investors may make frugal decisions, either lack information or habituate to making fast decisions (called heuristic) that challenge the EMH. The essence is that decisions taken by irrational investors may lead to inefficiencies in the stock market ( Akerlof and Shiller, 2010 ; Lobao et al. , 2017 ). Future researchers can also throw light on the effect of heuristic decisions made by investors on the efficiency of the stock market. Finally, as financial literacy plays an essential role in the investor's behaviour, it would be interesting to put on the agenda of future research to study the impact of financial literacy and financial illiteracy on the effectiveness of the stock market ( Rasool and Ullah, 2020 ).
6. Conclusions
The basic tenet of EMH is that if the stock market is working efficiently, the prices will follow a random walk, and the prices will reflect the intrinsic values, and no one can benefit from trading. However, there is the possibility that investors may be reluctant to agree with this hypothesis. Hence, some investors tend to buy stock, and some others may sell the stock so that the price is not affected significantly ( Latham, 1985 ). Amid several studies that have been conducted in India, this study was a modest attempt to empirically examine whether the stock market in India is weak-form efficient. The data was collected (daily open, high, low and closing prices) on nine BSE broad market indices and analysed using the standard tests (unit root, descriptive statistics, autocorrelation and runs test) covering the period from 1 January 2011 to 31 December 2020. The results did not support random walk theory, thus contributing to supporting the theory against EMH. Therefore, it is concluded that the Indian stock market, based on the results from the study, is a weak-form inefficient.
EMH proposes that security prices reflect all the available information. If this hypothesis is true, the asset managers need to demonstrate extraordinary skills to convince the investors that the recommended stock outperforms other securities ( Brown, 2020 ). Since the rapid growth of technology makes information accessible in no time, prices must reflect all information. EMH must be more valid in the present-day context than when Fama (1970) postulated the hypothesis. While the academicians do not consider the costs involved in acquiring information, the investment analyses do think the cost involved, and hence Fama (1970) argued that prices reflect the information only to the extent the expenses do not outweigh the benefits. One of the implications of EMH, as critics argue, is that there will be equilibrium in capital markets because of the presence of rational and informed agents. However, in real life, not all traders are perfectly rational. So, in the imperfect world, as behavioural financial scholars contend, EMH is valid only in theory (in spirit) but not accurate in practice. Some examples are that the financial crisis around 2008 around the world was a total failure of EMH, as the stock market largely depends on behavioural needs rather than financial arithmetic ( Malkiel, 2003 ). As things stand now, as there is no consensus among financial management scholars and economists about all three forms of EMH, there is a near-consensus that EMH is a theory simple to describe but difficult to put to the empirical test. Despite the onslaught against the EMH over the last two decades, the puzzling set of stock market anomalies could be considered chance events; some supporters argue that it is hard to find profit even if the market is highly volatile ( Roll, 1994 ). Lack of finding support for EMH may be considered as a shortcoming of the basic model. It would not be an exaggeration that the EMH has not lost its charisma and is expected to be on the agenda of financial economists.
Descriptive statistics for BSE broad market indices
Note(s): AllCap, All Capitalization; BSE, Bombay Stock Exchange; LargeCap, Large Market Capitalization; MidCap, Mid-Capitalization; S.D., Standard Deviation; SENSEX, Sensitivity Index
Source(s): Compiled from EViews 10
Akerlof , G.A. and Shiller , R.J. ( 2010 ), Animal Spirits: How Human Psychology Drives the Economy, and Why it Matters for Global Capitalism , Princeton University Press , Princeton, NJ .
Aren , S. and Aydemir , S.D. ( 2015 ), “ The factors influencing given investment choices of individuals ”, Procedia - Social and Behavioral Sciences , Vol. 210 , pp. 126 - 135 .
Barber , B.M. , Morse , A. and Yasuda , A. ( 2021 ), “ Impact investing ”, Journal of Financial Economics , Vol. 139 No. 1 , pp. 162 - 185 .
Borden , L.M. , Lee , S. , Serido , J. and Collins , D. ( 2008 ), “ Changing college students' financial knowledge, attitudes, and behavior through seminar participation ”, Journal of Family and Economic Issues , Vol. 29 No. 1 , pp. 23 - 40 .
Brown , S.J. ( 2020 ), “ The efficient market hypothesis, the financial analysts journal, and the professional status of investment management ”, Financial Analysts Journal , Vol. 76 No. 2 , pp. 5 - 14 , doi: 10.1080/0015198X.2020.1734375 .
Chan , K.C. , Gup , B.E. and Pan , M.-S. ( 1997 ), “ International stock market efficiency and integration: a study of eighteen nations ”, Journal of Business Finance and Accounting , Vol. 24 No. 6 , pp. 803 - 813 .
Degutis , A. and Novickytė , L. ( 2014 ), “ The efficient market hypothesis: a critical review of literature and methodology ”, Ekonomika , Vol. 93 No. 2 , pp. 7 - 23 .
Fama , E.F. ( 1970 ), “ Efficient capital markets: a review of theory and empirical work ”, The Journal of Finance , Vol. 25 No. 2 , pp. 383 - 417 .
Fama , E.F. and French , K.R. ( 1988 ), “ Permanent and temporary components of stock prices ”, Journal of Political Economy , Vol. 96 No. 2 , pp. 246 - 273 .
Grossman , S. ( 1976 ), “ On the efficiency of competitive stock markets where traders have diverse information ”, The Journal of Finance , Vol. 31 No. 2 , pp. 573 - 585 .
Hamid , K. , Suleman , M.T. , Ali , S. , Syed , Z. , Imdad , A. and Rana , S. ( 2010 ), “ Testing the weak form of efficient market hypothesis: empirical evidence from Asia-Pacific markets ”, International Research Journal of Finance and Economics , Vol. 58 No. 1 , pp. 121 - 133 .
Harshita , Singh , S. and Yadav , S.S. ( 2018 ), “ Calendar anomaly: unique evidence from the Indian stock market ”, Journal of Advances in Management Research , Vol. 15 No. 1 , pp. 87 - 108 .
Isidore , R. and Christie , P. ( 2017 ), “ Review of the influence of investor personality (The Big-Five model) on investor behavior ”, International Journal of Research in Finance and Marketing , Vol. 7 No. 7 , pp. 23 - 32 .
Jain , D. , Patel , M. , Narsaria , A. and Malik , S. ( 2020 ), “ A study on the efficiency of the Indian stock market ”, available at: https://arxiv.org/pdf/2012.01160.pdf .
Kelikume , I. , Olaniyi , E. and Iyohab , F.A. ( 2020 ), “ Efficient market hypothesis in the presence of market imperfections: evidence from selected stock markets in Africa ”, International Journal of Management, Economics and Social Sciences , Vol. 9 No. 1 , pp. 37 - 57 .
Latham , M. ( 1985 ), “ Defining capital market efficiency ”, Finance Working Paper 150 Institute for Business and Economic Research , University of California , Berkeley .
Lobao , J. , Pacheco , L. and Pereira , C. ( 2017 ), “ The use of the recognition heuristic as an investment strategy in European stock markets ”, Journal of Economics, Finance and Administrative Science , Vol. 22 No. 43 , pp. 207 - 223 .
Lodha , S. and Sora , G. ( 2015 ), “ Seasonal patterns in Indian stock markets: an application of GARCH (1, 1) model ”, American International Journal of Research in Humanities, Arts and Social Sciences , Vol. 9 No. 1 , pp. 33 - 43 .
Malkiel , B.G. ( 2003 ), “ The efficient market hypothesis and its critics ”, Journal of Economic Perspectives , Vol. 17 No. 1 , pp. 59 - 82 .
Miralles-Quirós , M.D.M. , Miralles-Quirós , J.L. and Oliveira , C. ( 2017 ), “ The role of liquidity in asset pricing: the special case of the Portuguese Stock Market ”, Journal of Economics, Finance and Administrative Science , Vol. 22 No. 43 , pp. 191 - 206 .
Parthsarathy , S. ( 2016 ), “ Test of weak form efficiency of the emerging Indian stock market using the non-parametric rank and sign variance ratio test ”, Global Journal of Finance and Management , Vol. 8 No. 1 , pp. 49 - 64 .
Patel , A. , Rajpal , R. and Modi , A. ( 2018 ), “ Testing weak form of market efficiency: a study on Indian stock market ”, International Journal of Management and Business Studies , Vol. 8 No. 4 , pp. 9 - 11 .
Rasool , N. and Ullah , S. ( 2020 ), “ Financial literacy and behavioural biases of individual investors: empirical evidence of Pakistan stock exchange ”, Journal of Economics, Finance and Administrative Science , Vol. 25 No. 50 , pp. 261 - 278 .
Roll , R. ( 1994 ), “ What every CEO should know about scientific progress in economics: what is known and what remains to be resolved ”, Financial Management , Vol. 23 No. 1 , pp. 69 - 75 .
Sadiq , M.N. and Khan , R.A.A. ( 2019 ), “ Impact of personality traits on investment intention: the mediating role of risk behaviour and the moderating role of financial literacy ”, Journal of Finance and Economics Research , Vol. 4 No. 1 , pp. 1 - 18 .
Samsa , G. ( 2021 ), “ The efficient market hypothesis is usually addressed indirectly: what happens if a direct approach is used instead? ”, Archives of Business Research , Vol. 9 No. 6 , pp. 45 - 50 , doi: 10.14738/abr.96.2021 .
Shiller , R. ( 2003 ), “ From efficient markets theory to behavioral finance ”, Journal of Economic Perspectives , Vol. 17 No. 1 , pp. 83 - 104 .
Sitkin , S.B. and Weingart , L.R. ( 1995 ), “ Determinants of risky decision-making behavior: a test of the mediating role of risk perceptions and propensity ”, Academy of Management Journal , Vol. 38 No. 6 , pp. 1573 - 1592 .
Titan , A.G. ( 2015 ), “ The Efficient Market Hypothesis: review of specialized literature and empirical research ”, Procedia Economics and Finance , Vol. 32 , pp. 442 - 449 .
Vidya , A. ( 2018 ), “ An empirical study on weak form efficiency of Indian stock market ”, International Journal of Management Studies , Vol. 5 No. 2 , pp. 94 - 98 .
Acknowledgements
The authors are thankful to the Editor-in-Chief, Professor Nestor U. Salcedo, and the anonymous reviewers for their constructive suggestions in the earlier version of the manuscript.
Corresponding author
Related articles, we’re listening — tell us what you think, something didn’t work….
Report bugs here
All feedback is valuable
Please share your general feedback
Join us on our journey
Platform update page.
Visit emeraldpublishing.com/platformupdate to discover the latest news and updates
Questions & More Information
Answers to the most commonly asked questions here
Study of Impact of Dematerialization of Shares on the Indian Stock Market
- Conference paper
- First Online: 14 July 2022
- Cite this conference paper
- Shreya Bhadra 11 &
- Ashok Chopra ORCID: orcid.org/0000-0002-2863-8730 11
Part of the book series: Lecture Notes in Networks and Systems ((LNNS,volume 487))
Included in the following conference series:
- International Conference on Business and Technology
753 Accesses
In the modern world, the stock market plays a crucial role in the country’s economic development and is amongst the most versatile sectors in the country’s financial system. It provides a platform for investors to trade shares, bonds, and debentures and allows the companies to raise the much-required funds to boost their business. In this way, the stock market plays a crucial role in enhancing the country’s industry and commerce growth. The reason for selecting this topic is that the Indian Share Market has grown unprecedented after the introduction of Dematerialization by the government of India in the mid – 90’s. Hence, to study how the Dematerialization and electronification of shares have shaped the tremendous growth and potential of the Indian Stock Market became an interesting study to explore its various possibilities. The journey traces the growth of the Indian Stock Market from its humble beginning with just a few traders to the globally competitive and colossal stock market of the present day. Currently, the Indian Stock Market boasts of having 5 70 000 traders and a total market capitalization worth 2.27 trillion dollars, leading to becoming the world’s 11th largest stock exchange. This paper explores the multiple benefits that they share trading industry gained as a result of Dematerialization.
This is a preview of subscription content, log in via an institution to check access.
Access this chapter
- Available as PDF
- Read on any device
- Instant download
- Own it forever
- Available as EPUB and PDF
- Compact, lightweight edition
- Dispatched in 3 to 5 business days
- Free shipping worldwide - see info
Tax calculation will be finalised at checkout
Purchases are for personal use only
Institutional subscriptions
Kumar, M., Elahi, Y.: Study of customers’ preference towards investment in mutual funds and equity shares with special reference to Lucknow. Int. J. Manage. Stud. (2018)
Google Scholar
Harshit, S.S., Yadav, S.: Department of Management Studies, Indian Institute of Technology Delhi, Vishwakarma Bhawan, Hauz Khas, New Delhi 110016, India (2021)
Why Would Someone Choose a Mutual Fund Over a Stock? (2020) https://www.investopedia.com/ask/answers/05/062305.asp
Louis, N.: The importance of Diversification Investopedia Investing.docx (2020). https://www.coursehero.com/file/48757160/The-importance-of-Diversification-INVESTOPEDIA-INVESTINGdocx/
Richardson Pete Stock market hit by coronavirus: Reasons for turmoil, what equity investors should do now (2020). https://economictimes.indiatimes.com/wealth/invest/stock-market-hit-by-coronavirus-reasons-for-turmoil-what-equity-investors-should-do-now/articleshow/74623291.cms
Glen Curtis 9 Major Questions Investors Should Ask Management (2020). https://www.investopedia.com/articles/stocks/08/questions-for-management.asp
Vinit, S.: Int. J. Adv. Res. Comput. Sci. Manage. Stud. Res. Article/Paper/Case Stud. (2020) www.ijarcsms.com
Wang: Stock Market Trading Volume, May 2009. https://doi.org/10.1016/B978-0-444-53548-1.50007-6
Karpoff. (2020) http://ijarcsms.com/docs/paper/volume2/issue3/V2I3-0012.pdf
Portnoy, K.: High-Frequency Trading and the Stock Market: A Look at the Effects of Trade Volume on Stock Price Changes Kyle Portnoy 2011 Illinois Wesleyan University (2011). https://core.ac.uk/download/pdf/59222771.pdf
Huddart, S., Lang, M.H., Yetman, M., Yetman, M.: Psychological factors, stock price paths, and trading volume. SSRN Electron. J. (2003). https://doi.org/10.2139/ssrn.353749
Farrukh, S.: Do weather influence investor behavior, stock returns, and volatility? Evidence from the greater China region. Phys. Stat. Mech. Appl 523 , 525–543 (2019). https://doi.org/10.1016/j.physa.2019.02.015
Article Google Scholar
Chopra, A.: Technology disruptions reshaping the market journal of advanced research in dynamical and control systems. (H Index 8 ISSN1943023X SJR {0.11} Ranking of United States Scimago Institutions Ranking), 11 (03), 1704–1710 (2020). Scopus® database (Elsevier B.V.). http://www.jardcs.org/abstract.php?id=975 . 7 Jan 2019
Download references
Author information
Authors and affiliations.
Amity University, Dubai, United Arab Emirates
Shreya Bhadra & Ashok Chopra
You can also search for this author in PubMed Google Scholar
Corresponding author
Correspondence to Ashok Chopra .
Editor information
Editors and affiliations.
Middle East Technical University, Northern Cyprus Campus, Kalkanlı, Güzelyurt, Turkey
Bahaaeddin Alareeni
College of Business of Finance, Ahlia University, Manama, Bahrain
Allam Hamdan
Rights and permissions
Reprints and permissions
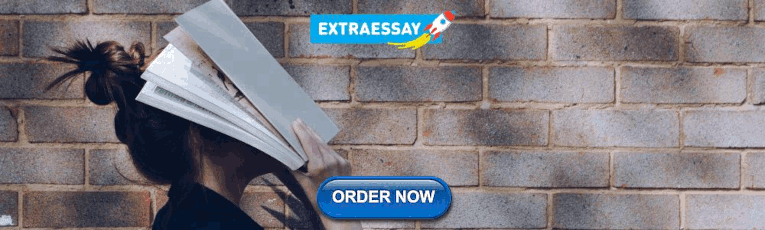
Copyright information
© 2023 The Author(s), under exclusive license to Springer Nature Switzerland AG
About this paper
Cite this paper.
Bhadra, S., Chopra, A. (2023). Study of Impact of Dematerialization of Shares on the Indian Stock Market. In: Alareeni, B., Hamdan, A. (eds) Sustainable Finance, Digitalization and the Role of Technology. ICBT 2021. Lecture Notes in Networks and Systems, vol 487. Springer, Cham. https://doi.org/10.1007/978-3-031-08084-5_25
Download citation
DOI : https://doi.org/10.1007/978-3-031-08084-5_25
Published : 14 July 2022
Publisher Name : Springer, Cham
Print ISBN : 978-3-031-08083-8
Online ISBN : 978-3-031-08084-5
eBook Packages : Engineering Engineering (R0)
Share this paper
Anyone you share the following link with will be able to read this content:
Sorry, a shareable link is not currently available for this article.
Provided by the Springer Nature SharedIt content-sharing initiative
- Publish with us
Policies and ethics
- Find a journal
- Track your research
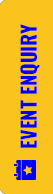
- Number of Visitor : 9895237

- About Research
- Advisory Committee
- Research Paper/ Articles
- Upcoming Conferences
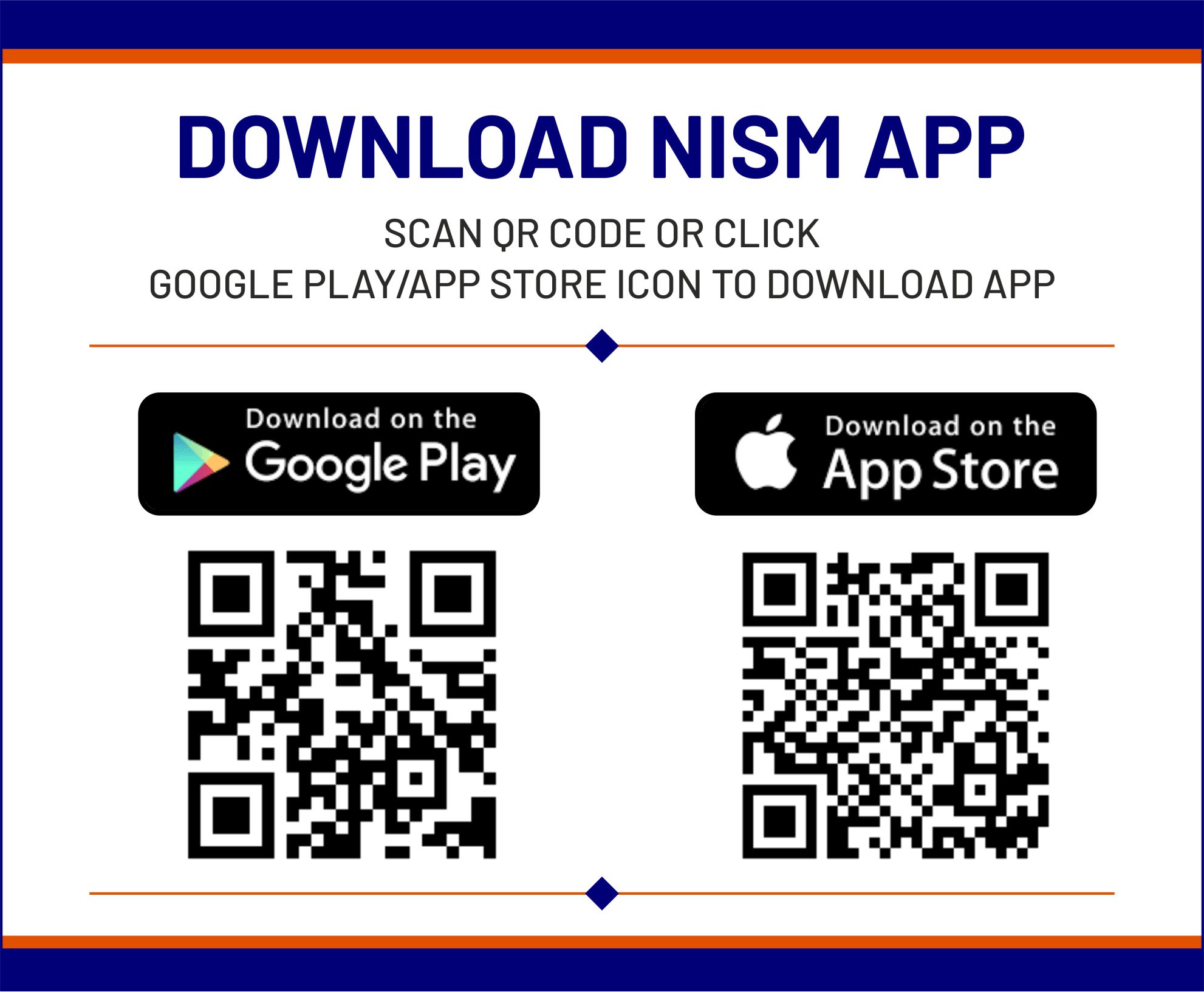
- Certifications
- Long Term Programs
- NISM Newsletters
- Investor Education
- Finance & Insurance ›
Financial Instruments & Investments
Stock market in India - statistics & facts
Impact of liberalization on the stock market, navigating ascent and turbulence: india’s stock market story, key insights.
Detailed statistics
Number of listed companies largest stock exchange operators globally 2023
Age of leading exchanges worldwide 2024
Leading 12 stock exchanges worldwide Q3 2023, by number of IPOs
Editor’s Picks Current statistics on this topic
Current statistics on this topic.
Market capitalization of listed domestic companies India 2005-2022
Investment Funds
Preferred type of stock market investment in India 2023
Related topics
Recommended.
- Stock exchanges
- New York Stock Exchange (NYSE)
- London Stock Exchange (LSE)
- Stock market indices
- Nasdaq Stock Market
Recommended statistics
Global overview.
- Premium Statistic Largest stock exchange operators worldwide 2023, by market capitalization
- Premium Statistic Countries with largest stock markets globally 2023
- Premium Statistic Leading stock exchanges APAC 2023 by domestic market capitalization
- Premium Statistic Leading 12 stock exchanges worldwide Q3 2023, by IPO proceeds
Largest stock exchange operators worldwide 2023, by market capitalization
Largest stock exchange operators worldwide as of December 2023, by market capitalization of listed companies (in trillion U.S. dollars)
Countries with largest stock markets globally 2023
Distribution of countries with largest stock markets worldwide as of January 2023, by share of total world equity market value
Leading stock exchanges APAC 2023 by domestic market capitalization
Leading stock exchanges in the Asia Pacific region as of May 2023, by domestic market capitalization (in billion U.S. dollars)
Leading 12 stock exchanges worldwide Q3 2023, by IPO proceeds
Leading 12 stock exchanges in terms of proceeds of IPO deals worldwide as of 3rd quarter 2023 (in billion U.S. dollars)
Key Indicators
- Premium Statistic Market capitalization to GDP ratio in India FY 2022-2023, by stock exchange
- Premium Statistic Total turnover to GDP ratio in India FY 2023
- Premium Statistic Number of companies listed in NSE and BSE across India FY 2008-2022
- Premium Statistic Number of unique investors on NSE in India 2018-2023
Market capitalization to GDP ratio in India FY 2022-2023, by stock exchange
Market capitalization to GDP ratio in India in the financial year 2022 and 2023, by stock exchange
Total turnover to GDP ratio in India FY 2023
Total turnover to GDP ratio in India in the financial year 2023
Number of companies listed in NSE and BSE across India FY 2008-2022
Number of companies listed in NSE and BSE across India from financial year 2008 to 2022
Number of unique investors on NSE in India 2018-2023
Number of unique investors on National Stock Exchange (NSE) of India from 2018 to 2023 (in millions)
Instruments and ownership
- Premium Statistic Resource mobilization through public and rights issues in India FY 2013-2023
- Premium Statistic Share of equity derivative segment in India FY 2023, by product
- Premium Statistic Share of ownership in companies listed on NSE in India 2023, by category
- Premium Statistic Foreign portfolio investment by instrument in India FY 2023, by instrument
Resource mobilization through public and rights issues in India FY 2013-2023
Resource mobilization through public and rights issues in India from financial year 2013 to 2023 (in billion Indian rupees)
Share of equity derivative segment in India FY 2023, by product
Share of equity derivative segment in India in the financial year 2023, by product
Share of ownership in companies listed on NSE in India 2023, by category
Share of ownership in companies listed on National Stock Exchange (NSE) in India as of December 2023, by category
Foreign portfolio investment by instrument in India FY 2023, by instrument
Foreign portfolio investment by instrument in India as of financial year 2023, by instrument (in billion Indian rupees)
Market Index
- Premium Statistic Annual variation in major indices in India FY 2023
- Premium Statistic Dividend yield of broad market indices listed on NSE in India 2024
- Premium Statistic Annual performance of equity indices listed on BSE in India 2024
- Premium Statistic Annual nifty 50 returns in India 2014-2023
- Premium Statistic Monthly performance of the S&P BSE Sensex Index in India 2017-2024
Annual variation in major indices in India FY 2023
Annual variation in major indices in India in the financial year 2023
Dividend yield of broad market indices listed on NSE in India 2024
Dividend yield of broad market indices listed on National Stock Exchange (NSE) of India as of April 2024
Annual performance of equity indices listed on BSE in India 2024
Annual performance of equity indices listed on Bombay Stock Exchange (BSE) in India as of March 2024
Annual nifty 50 returns in India 2014-2023
Annual nifty 50 returns in India from 2014 to 2023
Monthly performance of the S&P BSE Sensex Index in India 2017-2024
Monthly value of the S&P BSE Sensex Index in India from January 2017 to February 2024
Collective investment schemes
- Premium Statistic Trading volume of ETFs in India FY 2017-2024
- Premium Statistic Number of ETFs in India FY 2017-2024, by type
- Premium Statistic Deployment of mutual funds in India FY 2023, by asset class
- Premium Statistic AUM of equity mutual funds in India 2024, by type
- Premium Statistic AUM of debt mutual funds in India 2024, by type
Trading volume of ETFs in India FY 2017-2024
Trading volume of Exchange Traded Funds (ETFs) in India from financial year 2017 to 2024 (in billion Indian rupees)
Number of ETFs in India FY 2017-2024, by type
Number of Exchange Traded Funds (ETFs) in India from financial year 2017 to 2024, by type
Deployment of mutual funds in India FY 2023, by asset class
Deployment of mutual funds in India in financial year 2023, by asset class (in trillion Indian rupees)
AUM of equity mutual funds in India 2024, by type
Asset under management (AUM) of equity mutual funds in India as of January 31, 2024, by type (in trillion Indian rupees)
AUM of debt mutual funds in India 2024, by type
Asset under management (AUM) of debt mutual funds in India as of January 2024, by type (in billion Indian rupees)
Performance by sector
- Premium Statistic Dividend yield of sectoral market indices listed on NSE in India 2024
- Premium Statistic Leading oil exploration companies in India 2024, by market capitalization
- Premium Statistic Leading IT services and consulting companies in India 2023, by market capitalization
- Premium Statistic Leading FMCG companies in India 2024, by market capitalization
Dividend yield of sectoral market indices listed on NSE in India 2024
Dividend yield of sectoral market indices listed on National Stock Exchange (NSE) of India as of April 2024
Leading oil exploration companies in India 2024, by market capitalization
Leading oil exploration and production companies in India as of March 2024, based on market capitalization (in billion Indian rupees)
Leading IT services and consulting companies in India 2023, by market capitalization
Leading IT services and consulting companies in India as of November 2023, based on market capitalization (in billion Indian rupees)
Leading FMCG companies in India 2024, by market capitalization
Leading FMCG companies in India as of January 2024, by market capitalization (in billion Indian rupees)
Further reports Get the best reports to understand your industry
Get the best reports to understand your industry.
Mon - Fri, 9am - 6pm (EST)
Mon - Fri, 9am - 5pm (SGT)
Mon - Fri, 10:00am - 6:00pm (JST)
Mon - Fri, 9:30am - 5pm (GMT)
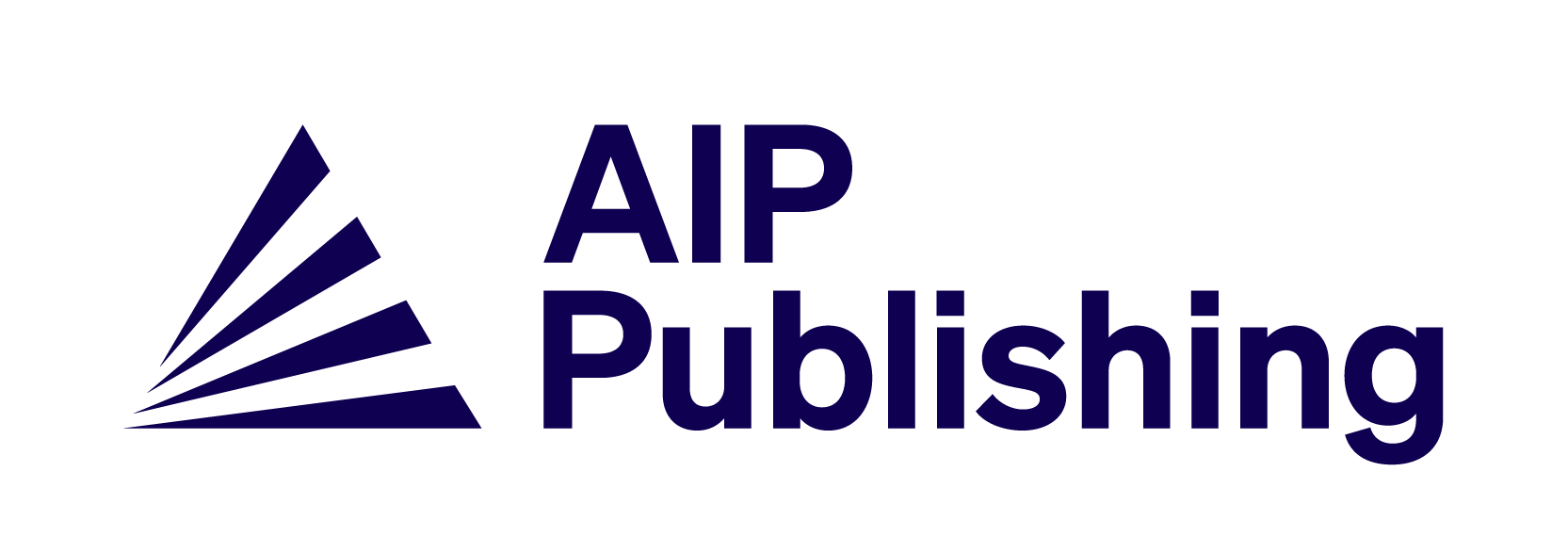
- Previous Article
- Next Article
A comprehensive survey of predicting stock market prices: An analysis of traditional statistical models and machine-learning techniques
- Split-Screen
- Article contents
- Figures & tables
- Supplementary Data
- Peer Review
- Open the PDF for in another window
- Reprints and Permissions
- Cite Icon Cite
- Search Site
Divy Patel , Warish Patel , Hakan Koyuncu; A comprehensive survey of predicting stock market prices: An analysis of traditional statistical models and machine-learning techniques. AIP Conf. Proc. 2 May 2024; 3107 (1): 050025. https://doi.org/10.1063/5.0208904
Download citation file:
- Ris (Zotero)
- Reference Manager
The stock market has witnessed a remarkable surge in popularity in recent years, attracting investors from all walks of life. However, predicting stock values remains a daunting task due to financial markets’ inherent unpredictability and complexity. Despite these challenges, the stock market offers a dynamic and ever-changing platform for traders to invest in shares, with the potential for significant gains and losses. For investors, accurate forecasting of stock prices is crucial as it provides invaluable insights into a company’s financial health and growth prospects. With this information, investors can make informed decisions, mitigate risks, and capitalize on lucrative opportunities in the market. As a result, extensive research has been dedicated to developing effective prediction methods, leveraging various mathematical models and machine-learning techniques. This research paper delves into the realm of stock market prediction, explicitly focusing on evaluating different machine-learning styles. The primary objective is to comprehensively analyze and compare the performance of these techniques in forecasting stock market behavior. By understanding the strengths and limitations of each method, investors, financial analysts, and market participants can gain critical knowledge to optimize their trading strategies and decision-making processes. To achieve this goal, the study explores an array of machine-learning algorithms, ranging from traditional linear regression models to sophisticated deep-learning approaches. These algorithms leverage historical stock market data, macroeconomic indicators, company financials, and sentiment analysis, among other factors, to predict future price movements and market trends. In addition to performance comparison, the research paper examines the impact of various factors that influence the effectiveness of these machine-learning techniques. Factors such as data quality, feature engineering, model selection, hyperparameter tuning, and market conditions play pivotal roles in the accuracy of predictions. Understanding these factors will aid in refining the model-building process and enhancing overall forecasting capabilities. The study encompasses an extensive dataset spanning multiple stock markets and periods, ensuring robustness and reliability in the findings. Performance evaluation metrics, including mean squared error, accuracy, precision, recall, and F1 score, will be employed to assess the predictive power of the machine-learning techniques objectively. Furthermore, the paper investigates the potential of ensemble methods, combining the strengths of multiple models to achieve enhanced prediction accuracy. Ensemble techniques, such as bagging, boosting, and stacking, have proven effective in diverse domains and are expected to demonstrate their value in stock market prediction. By the end of this research, readers will have a comprehensive understanding of the landscape of machine-learning techniques applied to stock market prediction. The findings will offer insights into which methods are most suitable for different market conditions and will aid in establishing best practices for effective and reliable stock market forecasting. In conclusion, this research paper serves as a valuable resource for investors, financial analysts, and researchers, thoroughly assessing machine-learning techniques’ efficacy in predicting stock market behavior. It contributes to the growing body of knowledge in financial technology. It underscores the critical role of data-driven decision-making in navigating the complexities of the modern stock market.
Citing articles via
Publish with us - request a quote.
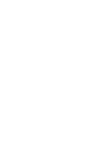
Sign up for alerts
- Online ISSN 1551-7616
- Print ISSN 0094-243X
- For Researchers
- For Librarians
- For Advertisers
- Our Publishing Partners
- Physics Today
- Conference Proceedings
- Special Topics
pubs.aip.org
- Privacy Policy
- Terms of Use
Connect with AIP Publishing
This feature is available to subscribers only.
Sign In or Create an Account
- Tata Steel share price
- 162.35 0.22%
- ITC share price
- 433.20 1.88%
- State Bank Of India share price
- 818.35 -0.16%
- HDFC Bank share price
- 1,437.60 -0.74%
- Tata Motors share price
- 1,046.85 1.62%
- HDFC Bank Share Price
- Reliance Industries Share Price
- TCS Share Price
- Infosys Share Price
- HUL Share Price

Indian stock market: 7 key things that changed for market overnight - Gift Nifty, Japan Services PMI to oil prices
Indian stock market: gift nifty was trading around 22,592 level, a premium of nearly 40 points from the nifty futures’ previous close, indicating a positive start for the indian stock market indices..
Indian stock market: The domestic equity indices, Sensex and Nifty 50, are expected to open higher on Tuesday led by positive global market cues.
Asian markets traded higher, while the US stocks ended in the green overnight amid rising hopes that there was a greater chance of the US Federal Reserve cutting interest rates this year.
Traders are currently pricing in rate cuts worth 46 basis points from the Fed by the end of 2024, with the first cut expected in September or November, according to LSEG’s rate probability app.
On Monday, the Indian stock market benchmark indices ended mixed amid high volatility.
The Sensex gained 17.39 points, or 0.02%, to close at 73,895.54, while the Nifty 50 settled 33.15 points, or 0.15%, lower at 22,442.70.
“The surge in India VIX by over 70% in the last eight trading sessions from around 10 to its 52-week high near 17 level, indicating increasing nervousness among investors due to the ongoing global uncertainties and Lok Sabha election outcome next month. Hence, markets are likely to remain in a broader range with stock-specific action in the near term," said Siddhartha Khemka, Head - Retail Research, Motilal Oswal Financial Services Ltd.
Also Read: Buy or sell: Vaishali Parekh recommends three stocks to buy today — May 7
Here are key global market cues for Sensex today:
Asian Markets
Asian markets traded higher on Tuesday tracking overnight gains on Wall Street.
Japan’s Nikkei 225 rose 0.96%, while the Topix gained 0.61%. South Korea’s Kospi rallied 1.6% and the Kosdaq surged 1.08%. Hong Kong’s Hang Seng index futures indicated a slightly weaker open.
Read here: Asian Stocks Climb After US Gain on Rates Optimism: Markets Wrap
Gift Nifty Today
Gift Nifty was trading around 22,592 level, a premium of nearly 40 points from the Nifty futures’ previous close, indicating a positive start for the Indian stock market indices.
Wall Street
The US stock market ended higher on Monday, extending gains for the third straight session.
The Dow Jones Industrial Average gained 176.59 points, or 0.46%, to 38,852.27, while the S&P 500 rose 52.95 points, or 1.03%, to 5,180.74. The Nasdaq Composite ended 192.92 points, or 1.19%, higher at 16,349.25.
Among stocks, Arm Holdings rallied 5.2%, Micron Technology shares gained 4.7%, Advanced Micro Devices share price rose 3.4% and Super Micro Computer stock price surged 6.1%. Paramount Global share price added 3.1%.
Tyson Foods shares declined 5.7%, while Spirit Airlines shares plunged 9.7%, to a record closing low.
US Federal Reserve
Richmond Fed President Thomas Barkin said the current interest rate level should cool the economy enough to return inflation to the central bank’s 2% target, with the strength of the job market giving officials time to wait, Reuters reported. Barkin, a voter this year on interest rate policy, added that inflation “data whiplash" supported the Fed's deliberative policy towards interest rates.
Federal Reserve Bank of New York President John Williams said while rate cuts would happen, monetary policy was currently in a very good place.
Also Read: Commodity stocks lift Australian shares; RBA decision on tap
Japan Services PMI
Japanese service sector activity grew at the fastest pace in eight months in April, a private survey showed. The final au Jibun Bank Service purchasing managers’ index (PMI) rose to 54.3 last month, the highest level since August 2023, and up slightly from 54.1 in March.
Crude oil prices rose after Israel struck Rafah in Gaza while negotiations for a ceasefire with Hamas continued without resolution, Reuters reported. Brent crude futures rose 0.46% to $83.71 per barrel, while US West Texas Intermediate (WTI) crude futures gained 0.59% to $78.94 a barrel.
The US dollar index, which measures the currency against six major peers, including the yen, sterling and euro, was little changed at 105.13, after dipping as low as 104.52 on Friday.
(With inputs from Reuters)
Disclaimer: The views and recommendations made above are those of individual analysts or broking companies, and not of Mint. We advise investors to check with certified experts before making any investment decisions.
MOTILAL OSWAL FINANCIAL SERVICES
Milestone Alert! Livemint tops charts as the fastest growing news website in the world 🌏 Click here to know more.
Unlock a world of Benefits! From insightful newsletters to real-time stock tracking, breaking news and a personalized newsfeed - it's all here, just a click away! Login Now!

Get the best recommendations on Stocks, Mutual Funds and more based on your Risk profile !
Wait for it…
Log in to our website to save your bookmarks. It'll just take a moment.
You are just one step away from creating your watchlist!
Oops! Looks like you have exceeded the limit to bookmark the image. Remove some to bookmark this image.
Your session has expired, please login again.
Congratulations!
You are now subscribed to our newsletters. In case you can’t find any email from our side, please check the spam folder.

Subscribe to continue
This is a subscriber only feature Subscribe Now to get daily updates on WhatsApp

Open Demat Account and Get Best Offers
Start Investing in Stocks, Mutual Funds, IPOs, and more
- Please enter valid name
- Please enter valid mobile number
- Please enter valid email
- Select Location
I'm interested in opening a Trading and Demat Account and am comfortable with the online account opening process. I'm open to receiving promotional messages through various channels, including calls, emails & SMS.

The team will get in touch with you shortly
Why is Indian stock market falling for five straight sessions? — explained with 5 crucial reasons
Stock market today: The Indian stock market continues to remain under the sell-off heat for the fifth straight session on Thursday. The Nifty 50 index opened lower in the early morning session and touched an intraday low of 22,184, logging more than 450 points in the last five sessions. The BSE Sensex opened lower and hit an intraday low of 72,891, recording over 1700 points loss in five days. The Nifty Bank index has been under selling pressure for the last seven straight sessions, losing 1600 points. According to the astute observations of our esteemed stock market experts, the ongoing Lok Sabha elections, FIIs' selling, bounce back in the US dollar rates, hawkish US Fed fueling treasury yields, unimpressive Q4 results 2024 season and rising India VIX Index are some of the primary reasons that have been dragging the Indian stock market downwards.
Why is the stock market falling?
Speaking on the reasons for the drag on the Indian stock markets, Saurabh Jain, Vice President of research at SMC Global Securities , said, "There are combinations of reasons that are dragging Indian indices together. Ongoing Lok Sabha polls, continuous selling by FIIs, not so impressive Q4 results season, hawkish US Fed and rising VIX India Index can be attributed as one of the major reasons weighing on the Indian stock market these days."
Also Read: Gold price dips ahead of Akshaya Tritiya on hawkish US Fed. Opportunity to buy?
Why share market is falling today? Top 5 reasons
1] Lok Sabha elections: "The Indian stock market has already discounted the victory of BJP-led NDA in the ongoing Lok Sabha polls. Now, the Indian stocks are in overbought condition and hence premature profit booking has triggered on Dalal Street. But, the selling is visible in the frontline large-cap stocks only. We are seeing bottom fishing in the broad market as the small-cap and mid-cap indices shot up on Thursday and outshined the frontline indices," said Avinash Gorakshkar, Head of Research at Profitmart Securities, providing a balanced view of the situation.
2] FIIs' selling: "FIIs are selling heavily this month as they have remained net sellers on all sessions in May 2024. They have sold out Indian shares worth ₹ 15,863 crore in the cash segment till Thursday while the FIIs have sold out shares worth ₹ 5,292 crore in the Future & Option (F&O) segment," said Avinash Gorakshkar of Profitmart Securities.
3] Hawkish US Fed: "Hawkish talks by some US Fed officials recently have put extra pressure on the Indian stocks. After witnessing some profit booking early this month, such statements enabled the US dollar rate to rebound. Rising US dollar prices have fueled the US Treasury yield as well. So, investors are expected to switch money from equity and other assets to currency and treasury markets," said Saurabh Jain of SMC Global Securities.
4] Non-impressive Q4 results 2024: Saurabh Jain added that the current Q4 results for the 2024 season have gone without any surprise. This also failed to trigger extra buying on Dalal Street. "As the market had already discounted the Q4 results 2024 ahead of the quarterly results season, investors are booking profit now as the season is about to end next week," said Saurabh Jain.
5] Rising VIX Index: "The continuous rise in the VIX Index has also put doubt among the fresh buyers, who are shy of pumping money in the current volatile market. As India VIX Index has a history of climbing during Lok Sabha elections and we are just in the middle of general elections 2024, the volatility is expected to pick up further as we come close to the poll results date," said Avinash Gorakshkar of Profitmart Securities.
Disclaimer: The views and recommendations provided in this analysis are those of individual analysts or broking companies, and not Mint. We strongly advise investors to consult with certified experts before making any investment decisions, as market conditions can change rapidly and individual circumstances may vary.
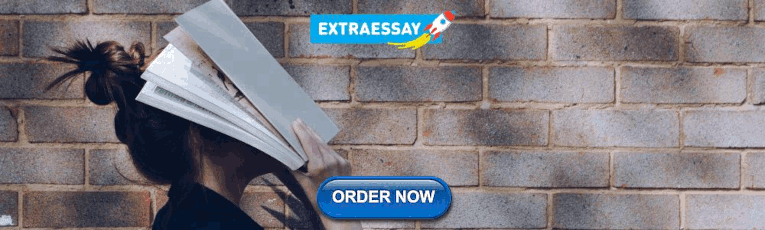
IMAGES
VIDEO
COMMENTS
The present paper is divided into two parts: in the first section, the evolution of international stock markets and the developments in Indian stock markets are briefly reviewed to help us ...
In order to understand the factors that influence the investor's investment decision a study was undertaken in Indian stock market consisting of 10 sectors with 30 companies listed on BSE-30 SENSEX. A research instrument consisting of 14 attributes was designed and sent to 2100 respondents. 467 responses were collected over a period of 6 ...
The results of several studies have shown that the performance of the Indian stock market has a positive bearing on the country's economic growth (Deena, 2013 ... He has published research papers in journals like Journal of Research in Interactive Marketing, Young Consumers, Journal of Internet Commerce, Interactive Technology and Smart ...
International investors will not be able to reduce their portfolio risk by diversifying between India and any other of the 15 countries in the sample because all of them are integrated with Indian stock market. Trade of India with Asian countries has increased in recent years and integration has also increased.
The onset of the COVID-19 pandemic and lockdown announcements by governments have created uncertainty in business operations globally. For the first time, a health shock has impacted the stock markets forcefully. India, one of the major emerging markets, has witnessed a massive fall of around 40% in its major stock indices' value. Therefore, we examined the short-term impact of the pandemic ...
Department of Humanities and Social Sciences, Indian Institute of Technology, Kharagpur, West Bengal, India. Correspondence. Manamani Sahoo, Department of Humanities and Social Sciences, Indian Institute of Technology, Kharagpur, West Bengal 721302, India. Email: [email protected] Search for more papers by this author
The literature relating to the impact of monetary policy on the stock market at a disaggregated sector-specific level is rather limited. An early influential paper by Ehrmann and Fratzcher suggested (1) a tightening of 50 basis points in US monetary policy, on an average, reduces the S&P 500 stock returns by about 3%; (2) individual stocks react in a highly heterogeneous fashion due to ...
Relative momentum strategies yield large and substantial profits in the Indian Stock Market. Nevertheless, relative momentum profits are negatively skewed and prone to occasional severe losses. By taking into consideration 450 stocks listed on the Bombay Stock Exchange, the present study predicts the timing of these huge momentum losses and ...
The findings suggest that all the sectors followed a consistent pattern of being significantly impacted by the pandemic, however, the benchmark index remained resilient in the context of average returns. This study examines the impact of COVID-19 pandemic on the performance of Indian stock market, measured by daily average returns and trading volume. The analysis is aimed at discovering the ...
Abstract. Outbreak and spread of the Covid-19 pandemic have touched to the core of our sentiments. Indian stock market has seen a roller coaster ride so far this year amid the Covid-19 pandemic. Sentiments have turned out to be a significant influence on the movement of the Indian stock market and pandemic has only added more steam.
International Review of Business Research Papers, 3(5), 21-35. Google Scholar. Basu D., Chawla D. (2010). An empirical test of CAPM—The case of Indian stock market. Global Business Review, 11(2), 209-220. Crossref. Google Scholar. Basu D., Chawla D. (2012). An empirical study of Arbitrage Pricing Theory—The case of Indian stock market.
The study aims to examine the market efficiency of the Indian stock market.,For analysis, nine Bombay Stock Exchange (BSE) broad market indices were selected covering the study period from 01 January 2011 to 31 December 2020. The data collected for this study are daily open, high, low and closing prices of selected indices.
IJRAR19K9271 International Journal of Research and Analytical Reviews (IJRAR) www.ijrar.org 34 FACTORS INFLUENCING INVESTMENT DECISIONS IN INDIAN STOCK MARKET: AN ... The stock market has evolved into a vehicle for a thriving economy, and the Indian stock market is booming as a result of millennials and young investors. While the investor ...
The Author's research objective is to study the consequences of the techniques of Dematerialization and electronification of shares introduced in the Indian Stock Exchange in the mid-'90 s on the volumes traded in the Indian Stock Market. The research may also be considered deductive to a certain point.
Also, there is a problem when the statement is too short to understand even by human being. Indian stock market has gained the interest of investors investing in two main stock market named as Bombay Stock Exchange (BSE) and National Stock Exchange (NSE). There is high risk involved for investors because of more complexity of the stock market.
View Article. Dr. V. Shunmugam. India's farm policy needs to focus on creating robust commodity supply chains - Moneycontrol on April 19, 2023. View Article. Mr. Kuldeep Thareja, Ms. Mitu Bhardwaj & Ms. Rasmeet Kohli. It's time to revisit some issues in securities markets - Mint on April 17, 2023. View Article.
The purpose of this study is to identify the key aspects that have been studied in the area of stock market liquidity, accumulate their important findings, and also provide a quantitative categorization of reviewed literature that will facilitate in conducting further research. The study analyzes relevant research papers published after the ...
This research paper focuses on the impact of COVID-19 on Indian Stock Market and shares performance. In other words, the article analyses the market capitalization correlation between the performances of shares and the growth of the share market, using the stock market data of Pre and post COVID-19 status by comparing the data from Jan'20 to Jun'20.
The Indian stock market has undergone a remarkable transformation over its century-long history, emerging as a dynamic and sophisticated financial ecosystem that plays a pivotal role in driving the nation's economic growth. With securities traded on prominent exchanges like the Bombay Stock Exchange (BSE) and the National Stock Exchange (NSE), the market serves as a vital platform for capital ...
being stock market investors. The research paper observes that only 10 % of the respondents intended to stay invested into the stock market for a period of more than 5 years. In other words, the research paper observed that people do not want to stay committed for longer period of time into the stock market despite it giving better returns.
The Indian stock market is one of the oldest in Asia, boasting a rich history dating back to 1875. This was the year the Bombay Stock Exchange (BSE), the country's first stock exchange, was ...
These algorithms leverage historical stock market data, macroeconomic indicators, company financials, and sentiment analysis, among other factors, to predict future price movements and market trends. In addition to performance comparison, the research paper examines the impact of various factors that influence the effectiveness of these machine ...
Additionally, this study intends to discuss the role of RBI in the present context and to examine the relationship between the Indian stock market and US stock markets. This paper employs the data from January 1, 1999 to December 31, 2008 to investigate the impact on Indian stock market and the integration of Indian stock market and US stock ...
Indian stock market: Gift Nifty was trading around 22,592 level, a premium of nearly 40 points from the Nifty futures' previous close, indicating a positive start for the Indian stock market ...
Top 5 reasons. 1] Lok Sabha elections: "The Indian stock market has already discounted the victory of BJP-led NDA in the ongoing Lok Sabha polls. Now, the Indian stocks are in overbought condition ...