Volume 8 Supplement 1
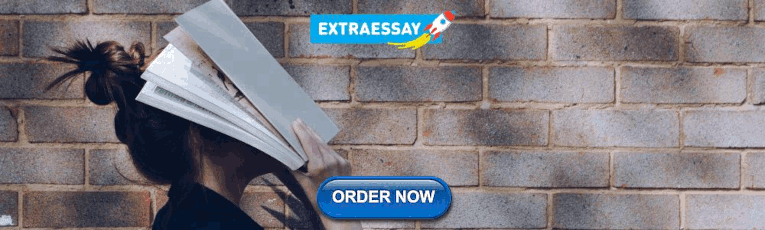
Proceedings of Advancing the Methods in Health Quality Improvement Research 2012 Conference
- Proceedings
- Open access
- Published: 19 April 2013
Understanding and managing variation: three different perspectives
- Michael E Bowen 1 , 2 , 3 &
- Duncan Neuhauser 4
Implementation Science volume 8 , Article number: S1 ( 2013 ) Cite this article
28k Accesses
4 Citations
13 Altmetric
Metrics details
Presentation
Managing variation is essential to quality improvement. Quality improvement is primarily concerned with two types of variation – common-cause variation and special-cause variation. Common-cause variation is random variation present in stable healthcare processes. Special-cause variation is an unpredictable deviation resulting from a cause that is not an intrinsic part of a process. By careful and systematic measurement, it is easier to detect changes that are not random variation.
The approach to managing variation depends on the priorities and perspectives of the improvement leader and the intended generalizability of the results of the improvement effort. Clinical researchers, healthcare managers, and individual patients each have different goals, time horizons, and methodological approaches to managing variation; however, in all cases, the research question should drive study design, data collection, and evaluation. To advance the field of quality improvement, greater understanding of these perspectives and methodologies is needed [ 1 ].
Clinical researcher perspective
The primary goal of traditional randomized controlled trials (RCTs) (ie a comparison of treatment A versus placebo) is to determine treatment or intervention efficacy in a specified population when all else is equal. In this approach, researchers seek to maximize internal validity. Through randomization, researchers seek to balance variation in baseline factors by randomizing patients, clinicians, or organizations to experimental and control groups. Researchers may also increase understanding of variation within a specific study using approaches such as stratification to examine for effect modification. Although the generalizability of outcomes in all research designs is limited by the study population and setting, this can be particularly challenging in traditional RCTs. When inclusion criteria are strict, study populations are not representative of “real world” patients, and the applicability of study findings to clinical practice may be unclear. Traditional RCTs are limited in their ability to evaluate complex processes that are purposefully and continually changing over time because they evaluate interventions in rigorously controlled conditions over fixed time frames [ 2 ]. However, using alternative designs such as hybrid, effectiveness studies discussed in these proceedings or pragmatic RCTs, researchers can rigorously answer a broader range of research questions [ 3 ].
Healthcare manager perspective
Healthcare managers seek to understand and reduce variation in patient populations by monitoring process and outcome measures. They utilize real-time data to learn from and manage variation over time. By comparing past, present, and desired performance, they seek to reduce undesired variation and reinforce desired variation. Additionally, managers often implement best practices and benchmark performance against them. In this process, efficient, time-sensitive evaluations are important. Run charts and Statistical Process Control (SPC) methods leverage the power of repeated measures over time to detect small changes in process stability and increase the statistical power and rapidity with which effects can be detected [ 1 ].
Patient perspective
While the clinical researcher and healthcare manager are interested in understanding and managing variation at a population level, the individual patient wants to know if a particular treatment will allow one to achieve health outcomes similar to those observed in study populations. Although the findings of RCTs help form the foundation of evidence-based practice and managers utilize these findings in population management, they provide less guidance about the likelihood of an individual patient achieving the average benefits observed across a population of patients. Even when RCT findings are statistically significant, many trial participants receive no benefit. In order to understand if group RCT results can be achieved with individual patients, a different methodological approach is needed. “N-of-1 trials” and the longitudinal factorial design of experiments allow patients and providers to systematically evaluate the independent and combined effects of multiple disease management variables on individual health outcomes [ 4 ]. This offers patients and providers the opportunity to collect, analyze, and understand data in real time to improve individual patient outcomes.
Advancing the field of improvement science and increasing our ability to understand and manage variation requires an appreciation of the complementary perspectives held and methodologies utilized by clinical researchers, healthcare managers, and patients. To accomplish this, clinical researchers, healthcare managers, and individual patients each face key challenges.
Recommendations
Clinical researchers are challenged to design studies that yield generalizable outcomes across studies and over time. One potential approach is to anchor research questions in theoretical frameworks to better understand the research problem and relationships among key variables. Additionally, researchers should expand methodological and analytical approaches to leverage the statistical power of multiple observations collected over time. SPC is one such approach. Incorporation of qualitative research and mixed methods can also increase our ability to understand context and the key determinants of variation.
Healthcare managers are challenged to identify best practices and benchmark their processes against them. However, the details of best practices and implementation strategies are rarely described in sufficient detail to allow identification of the key drivers of process improvement and adaption of best practices to local context. By advocating for transparency in process improvement and urging publication of improvement and implementation efforts, healthcare managers can enhance the spread of best practices, facilitate improved benchmarking, and drive continuous healthcare improvement.
Individual patients and providers are challenged to develop the skills needed to understand and manage individual processes and outcomes. As an example, patients with hypertension are often advised to take and titrate medications, modify dietary intake, and increase activity levels in a non-systematic manner. The longitudinal factorial design offers an opportunity to rigorously evaluate the impact of these recommendations, both in isolation and in combination, on disease outcomes [ 1 ]. Patients can utilize paper, smart phone applications, or even electronic health record portals to sequentially record their blood pressures. Patients and providers can then apply simple SPC rules to better understand variation in blood pressure readings and manage their disease [ 5 ].
As clinical researchers, healthcare managers, and individual patients strive to improve healthcare processes and outcomes, each stakeholder brings a different perspective and set of methodological tools to the improvement team. These perspectives and methods are often complementary such that it is not which methodological approach is “best” but rather which approach is best suited to answer the specific research question. By combining these perspectives and developing partnerships with organizational managers, improvement leaders can demonstrate process improvement to key decision makers in the healthcare organization. It is through such partnerships that the future of quality improvement research is likely to find financial support and ultimate sustainability.
Neuhauser D, Provost L, Bergman B: The meaning of variation to healthcare managers, clinical and health-services researchers, and individual patients. BMJ Qual Saf. 2011, 20 (Suppl 1): i36-40. 10.1136/bmjqs.2010.046334.
Article PubMed Central PubMed Google Scholar
Neuhauser D, Diaz M: Quality improvement research: are randomised trials necessary?. Qual Saf Health Care. 2007, 16: 77-80. 10.1136/qshc.2006.021584.
Article PubMed Central CAS PubMed Google Scholar
Eccles M, Grimshaw J, Campbell M, Ramsay C: Research designs for studies evaluating the effectiveness of change and improvement strategies. Quality and Safety in Health Care. 2003, 12: 47-52. 10.1136/qhc.12.1.47.
Olsson J, Terris D, Elg M, Lundberg J, Lindblad S: The one-person randomized controlled trial. Qual Manag Health Care. 2005, 14: 206-216.
Article PubMed Google Scholar
Hebert C, Neuhauser D: Improving hypertension care with patient-generated run charts: physician, patient, and management perspectives. Qual Manag Health Care. 2004, 13: 174-177.
Download references
Author information
Authors and affiliations.
VA National Quality Scholars Fellowship, Tennessee Valley Healthcare System, Nashville, Tennessee, 37212, USA
Michael E Bowen
Division of General Internal Medicine, Department of Medicine, University of Texas Southwestern Medical Center, Dallas, Texas, 75390, USA
Division of Outcomes and Health Services Research, Department of Clinical Sciences, University of Texas Southwestern Medical Center, Dallas, Texas, 75390, USA
Department of Epidemiology and Biostatistics, Case Western Reserve University, Cleveland, Ohio, 44106, USA
Duncan Neuhauser
You can also search for this author in PubMed Google Scholar
Corresponding author
Correspondence to Michael E Bowen .
Rights and permissions
This article is published under license to BioMed Central Ltd. This is an Open Access article distributed under the terms of the Creative Commons Attribution License ( http://creativecommons.org/licenses/by/2.0 ), which permits unrestricted use, distribution, and reproduction in any medium, provided the original work is properly cited.
Reprints and permissions
About this article
Cite this article.
Bowen, M.E., Neuhauser, D. Understanding and managing variation: three different perspectives. Implementation Sci 8 (Suppl 1), S1 (2013). https://doi.org/10.1186/1748-5908-8-S1-S1
Download citation
Published : 19 April 2013
DOI : https://doi.org/10.1186/1748-5908-8-S1-S1
Share this article
Anyone you share the following link with will be able to read this content:
Sorry, a shareable link is not currently available for this article.
Provided by the Springer Nature SharedIt content-sharing initiative
- Statistical Process Control
- Clinical Researcher
- Healthcare Manager
- Healthcare Process
- Quality Improvement Research
Implementation Science
ISSN: 1748-5908
- Submission enquiries: Access here and click Contact Us
- General enquiries: [email protected]
ASSIGNABLE CAUSES OF VARIATIONS
- Reference work entry
- Cite this reference work entry
627 Accesses
1 Citations
Assignable causes of variation are present in most production processes. These causes of variability are also called special causes of variation ( Deming, 1982 ). The sources of assignable variation can usually be identified (assigned to a specific cause) leading to their elimination. Tool wear, equipment that needs adjustment, defective materials, or operator error are typical sources of assignable variation. If assignable causes are present, the process cannot operate at its best. A process that is operating in the presence of assignable causes is said to be “out of statistical control.” Walter A. Shewhart (1931) suggested that assignable causes, or local sources of trouble, must be eliminated before managerial innovations leading to improved productivity can be achieved.
Assignable causes of variability can be detected leading to their correction through the use of control charts.
See Quality: The implications of W. Edwards Deming's approach ; Statistical process control ; Statistical...
This is a preview of subscription content, log in via an institution to check access.
Access this chapter
- Available as PDF
- Read on any device
- Instant download
- Own it forever
Tax calculation will be finalised at checkout
Purchases are for personal use only
Institutional subscriptions
Deming, W. Edwards (1982). Out of the Crisis, Center for Advanced Engineering Study, Massachusetts Institute of Technology, Cambridge, Massachusetts.
Google Scholar
Shewhart, W. A. (1939). Statistical Method from the Viewpoint of Quality Control, Graduate School, Department of Agriculture, Washington.
Download references
Editor information
Rights and permissions.
Reprints and permissions
Copyright information
© 2000 Kluwer Academic Publishers
About this entry
Cite this entry.
(2000). ASSIGNABLE CAUSES OF VARIATIONS . In: Swamidass, P.M. (eds) Encyclopedia of Production and Manufacturing Management. Springer, Boston, MA . https://doi.org/10.1007/1-4020-0612-8_57
Download citation
DOI : https://doi.org/10.1007/1-4020-0612-8_57
Publisher Name : Springer, Boston, MA
Print ISBN : 978-0-7923-8630-8
Online ISBN : 978-1-4020-0612-8
eBook Packages : Springer Book Archive
Share this entry
Anyone you share the following link with will be able to read this content:
Sorry, a shareable link is not currently available for this article.
Provided by the Springer Nature SharedIt content-sharing initiative
- Publish with us
Policies and ethics
- Find a journal
- Track your research
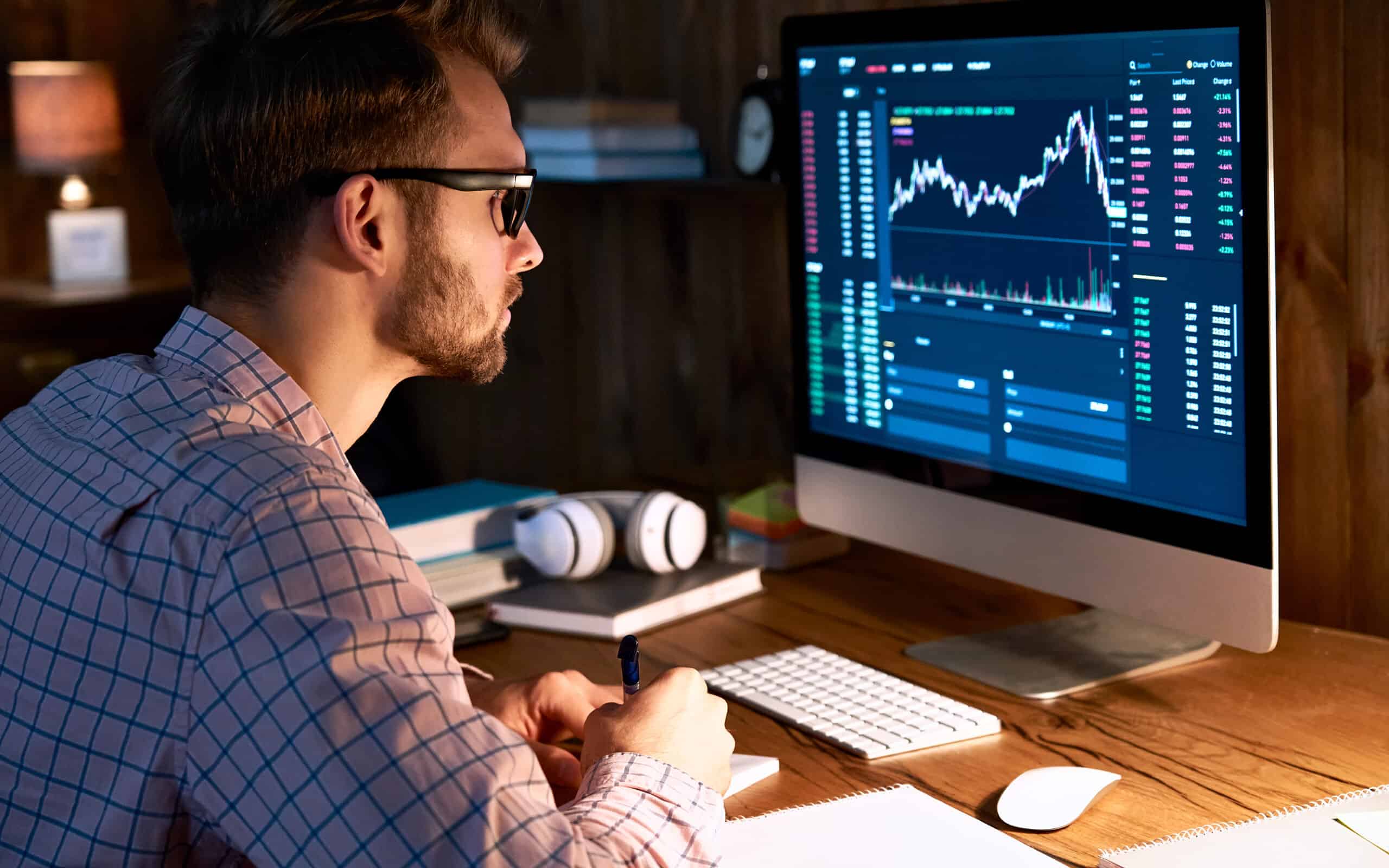
The Power of Special Cause Variation: Learning from Process Changes
Updated: July 28, 2023 by Marilyn Monda
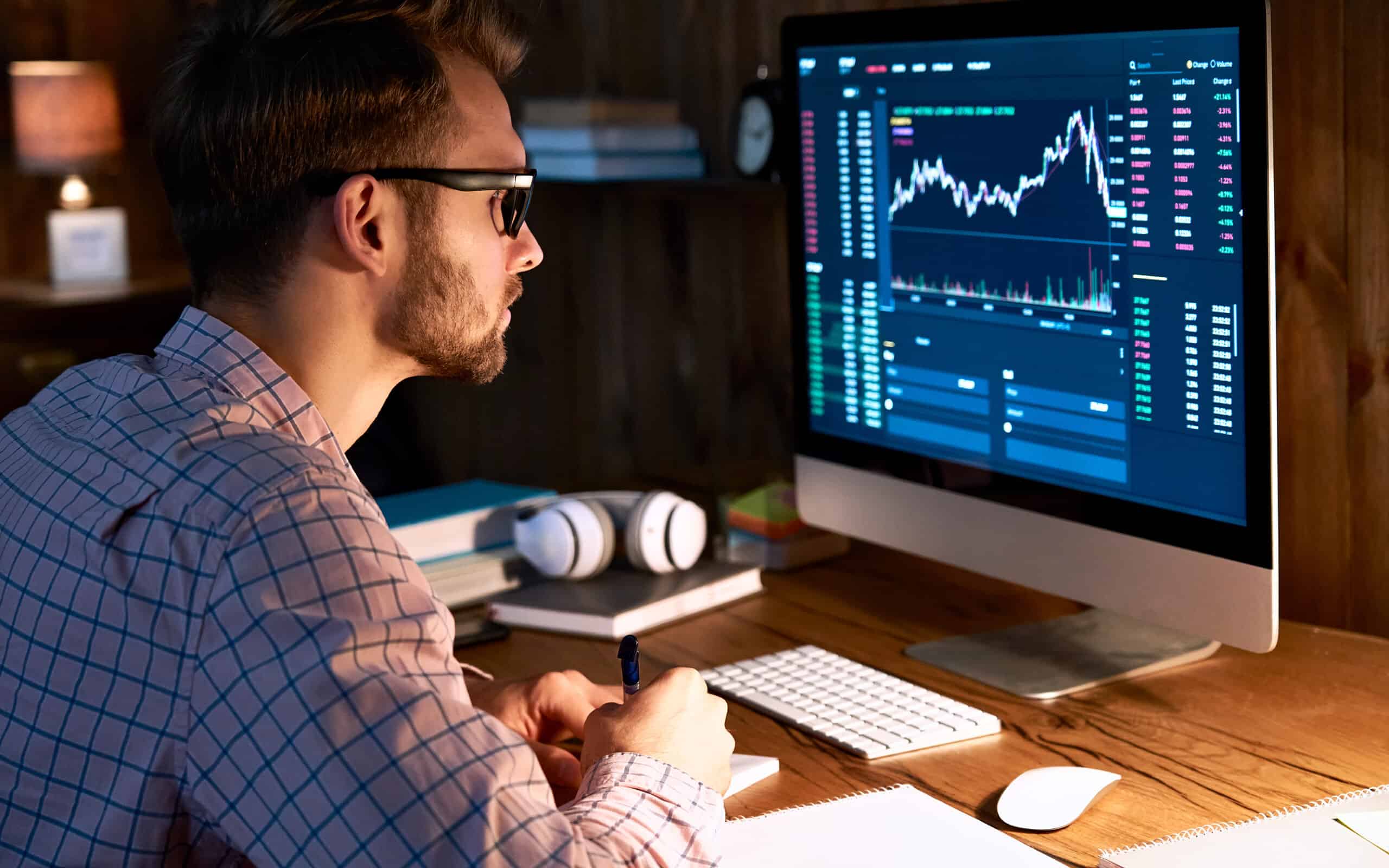
I love to see special cause variation! That’s because I know I’m about to learn something important about my process. A special cause is a signal that the process outcome is changing — and not always for the better.
Overview: What is special cause variation?
A control chart can show two different types of variation: common cause variation (random variation from the various process components) and special cause variation.
Special cause variation is present when the control chart of a process measure shows either plotted point(s) outside the control limits or a non-random pattern of variation.
When a control chart shows special cause variation, a process measure is said to be out-of-control or unstable. Common types of special cause variation signals include:
- A point outside of the upper control limit or lower control limit
- A trend: 6 or 7 points increasing or decreasing
- A cycle or repeating pattern
- A run: 8 or more points on either side of the average
A special cause of variation is assignable to a defect, fault, mistake, delay, breakdown, accident, and/or shortage in the process. When special causes are present, process quality is unpredictable.
Special causes are a signal for you to act to make the process improvements necessary to bring the process measure back into control.
RELATED: COMMON CAUSE VARIATION VS. SPECIAL CAUSE VARIATION
Drawbacks of special cause variation .
The source of a special cause can be difficult to find if you are not plotting the control chart in real time. Unless you have annotated data or a good memory, control charts made from historical data won’t aid your investigation into the source of the special cause.
If a process measure has never been charted, it is almost certain that it will be out of control. When you first start studying a process with a control chart, you will usually see a variety of special causes. To find the sources, begin a study of the status of critical process components.
When a special cause source cannot be found, it will become common to the process. As time goes on, the special causes repeat and cease being special. They then increase the natural or common cause variation in the process.
Why is special cause variation important to understand?
Let’s define quality as minimum variation around an appropriate target. The study of variation using a control chart is one way to tell if the process variation is increasing or if the center is moving away from the desired target over time.
A special cause is assignable to a process component that has changed or is changing. Investigation into the source of a special cause will:
- Let you know when to act to adjust or improve the process.
- Keep you from making the mistake of missing an opportunity to improve a process. If the ignored special cause repeats, you still don’t know how to fix it.
- Provide data to suggest or evaluate a process improvement.
If no special cause variation exists, that is, the process is in control, you should leave the process alone! Making process changes when there is no special cause present is called Tampering and can increase the variation of the process, lowering its quality.
An industry example of special cause variation
In this example, a control chart was used to monitor the number of data entry errors on job applications. Each day a sample of applications was reviewed. The number of errors found were plotted on a control chart.
One day, a point was plotted outside the control limit. Upon investigation, the manager noticed it occurred when a new worker started. It was found the worker wasn’t trained.
The newly trained worker continued data entry. A downward trend of errors followed, indicating the training was a source for the special cause!
The manager issued guidelines for new worker training. Since then, there have been three new workers without the error count spiking.
3 best practices when thinking about special cause variation
Special causes are signals that you need to act to move your process measure back into control.
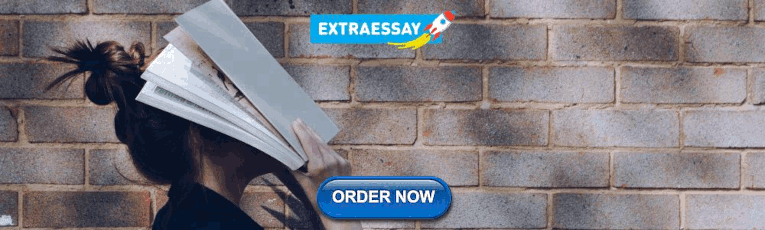
Identify the source
When a special cause of variation exists, make a timely effort to identify its source. A good starting point is to check if any process component changed near to the time the special cause was seen. Also, you could ask process experts to brainstorm why the special cause samples were out of control.
For example, a trend up in screw thickness could be caused by a gage going out of calibration.
Make improvements at the source
Implement improvements to the source of special cause variation. Once you make improvements to the source of the special cause (like re-calibrating that gage), watch what happens as the next thickness samples are plotted. If the plot moves back toward stability, you know you found the issue!
Document everything
As you identify recurring special causes and their sources, document them on a control plan so process operators know what to do if they see the special cause again.
For our gage, the control plan could direct a worker to recalibrate the next time the screw thickness trends up, sending the process back to stability.
Frequently Asked Questions (FAQ) about special cause variation
- Are special causes always bad news?
No. A special cause can indicate either an increase or decrease in the quality of the process measure.
If the special cause shows increased process quality (for example, a decrease in cycle time), then you should make its source common to the process.
- If a process is in control (no special causes) is it also capable?
Not always. Control and capability are two different assessments. Your process measure can be stable (in control) and still not meet the customer specification (capable).
Once a process measure is in control, you can then assess its capability against the customer target and specification limits. If the data is within customer limits and on target, the process is considered both in control and capable.
Final thoughts on special causes
Every process measure will show variation, you will never attain zero variability. Still, it is important to understand the nature of variability so that you can use it to better improve and control your process outcomes.
The special cause variation signal is the key to finding those critical process components that are the sources of variation needing improvement. Use special cause variation to unlock the path to process control.
About the Author
Marilyn Monda

Common Cause and Special Cause

Common Cause and Special Cause in Statistics: Understanding Variability
Statistics is a powerful tool for analyzing data and making informed decisions, but to do so effectively, it’s essential to understand the sources of variability within a process or system.
In the realm of statistical process control , two fundamental concepts come into play: common cause and special cause variation. These concepts help us differentiate between the everyday fluctuations in a process and the exceptional, identifiable factors that can lead to significant deviations from the norm.
Common Cause Variation
Common cause variation, often called random variation or systemic variation, is the inherent variability in any process. It is the everyday, expected variation that occurs when a system is stable and operating under normal conditions.
This type of variation is the result of numerous factors and interactions within a process, and it cannot be traced back to a specific source. Common cause variation is, in a sense, the “background noise” of a process.
Key characteristics of common cause variation include:
- Inherent to the Process: Common cause variation is an inherent part of a process and will always exist to some degree.
- Consistent Patterns: It typically follows consistent, predictable patterns, often resembling a bell-shaped curve (a normal distribution).
- Random and Unpredictable: It is random in nature and cannot be attributed to any specific factor or event. This makes it difficult to control or eliminate entirely.
- Small Fluctuations: Common cause variation results in small, manageable fluctuations around a process’s mean or average value.
Examples of common cause variation can include minor temperature fluctuations in a manufacturing process, small variations in delivery times, or slight variations in the weight of identical products produced on the same assembly line.
Special Cause Variation
Special cause variation, also known as assignable variation or non-random variation, is the opposite of common cause variation. It represents variability in a process that can be traced back to specific, identifiable causes. Unlike common cause variation, which is inherent to the process, special cause variation is due to external factors or events that disrupt the system’s normal functioning.
Key characteristics of special cause variation include:
- Identifiable Causes: Special cause variation can be linked to specific events, actions, or factors that are not part of the usual operation of the process.
- Erratic Patterns: Unlike the consistent patterns of common cause variation, it often exhibits erratic and unpredictable patterns.
- Large Fluctuations: Special cause variation results in significant deviations from a process’s mean or average value.
- Unusual Events: Examples of special cause variation can include equipment breakdowns, power outages, errors in data entry, or major shifts in market demand.
Differentiating Between Common Cause and Special Cause Variation
Distinguishing between common cause and special cause variation is crucial in process improvement and quality control. Understanding the source of variability in a process allows organizations to take appropriate actions.
Here are some guidelines for differentiation:
- Data Analysis: The first step is to collect and analyze data. If the variation observed falls within the expected range of common cause variation, it is likely due to inherent process variability. However, if the data points exhibit patterns or values that deviate significantly from the norm, special cause variation may be present.
- Statistical Tools: Various statistical techniques, such as control charts, can be used to monitor processes and identify abnormal data points that suggest special cause variation. Control charts help in distinguishing between natural process variation and unusual occurrences.
- Root Cause Analysis: When special cause variation is suspected, a thorough root cause analysis is essential. This involves investigating the specific factors that contributed to the variation and taking corrective actions to prevent its recurrence.
- Process Control: Once special cause variation is identified and addressed, process control measures can be put in place to minimize the risk of future occurrences.
How can we Minimize Common Cause Variation?
Minimizing common cause variation is a key goal in statistical process control and quality improvement. While common cause variation is inherent to any process and cannot be completely eliminated, there are several strategies and approaches that can help reduce its impact and maintain greater process stability. Here are some ways to minimize common cause variation:
- A thorough understanding of the process is the first step in minimizing common cause variation. You need to know how the process operates, what factors affect it, and the expected sources of variability.
- Implement process control tools, such as control charts, to continuously monitor the process. Control charts help in distinguishing between common cause and special cause variation. They visually represent the process’s performance over time, making it easier to detect trends or shifts.
- Develop and maintain standardized operating procedures for the process. SOPs ensure that everyone involved follows the same methods and practices, reducing variability in human factors and operational choices.
- Invest in the training and skill development of employees involved in the process. A well-trained workforce is less likely to introduce unnecessary variability through errors or inconsistent practices.
- Regularly maintain and calibrate equipment to minimize common cause variation associated with machinery or tools. Well-maintained equipment is more likely to produce consistent results.
- Use statistical techniques to understand the inherent variability in the process. By analyzing the process’s capability and identifying areas with excessive common cause variation, you can make data-driven decisions to improve it.
- Implement Lean Six Sigma principles to identify and eliminate waste and non-value-added steps in the process. This can help streamline operations and reduce variability.
- Use DOE methodologies to study the impact of various process factors on variability systematically. This approach can help optimize processes and identify which factors have the most significant impact on common cause variation.
- Form cross-functional teams to focus on process improvement. Teams can work together to identify sources of common cause variation, develop solutions, and ensure continuous process optimization.
- Make decisions based on data and evidence rather than intuition. Data-driven decisions allow for a better understanding of the process’s performance and the identification of areas where common cause variation can be reduced.
- Establish feedback loops to ensure that lessons learned from past performance are used to make continuous improvements. Regularly review and update process documentation, procedures, and best practices.
- Compare your process performance to industry benchmarks and best practices. Benchmarking can help identify areas where your process may be underperforming and experiencing excessive common cause variation.
- Encourage employees to provide feedback and suggestions for process improvement. They often have valuable insights into daily operations and can help identify and address common cause variation.
Minimizing common cause variation is an ongoing effort requiring a systematic process improvement approach. Organizations can reduce variability and enhance their processes’ overall quality and performance by consistently monitoring, analyzing, and making data-driven adjustments.
How does Common Cause and Special Cause Apply in Six Sigma Projects
Common cause and special cause variation are fundamental concepts in Six Sigma, a structured and data-driven methodology for process improvement. Understanding these concepts is crucial for identifying, analyzing, and addressing variations within processes to reduce defects and improve overall quality. Here’s how common cause and special cause apply in Six Sigma projects:
- Defining the Problem (Define Phase): In the Define phase of a Six Sigma project, the team identifies the problem that needs to be addressed. At this stage, it’s essential to differentiate between common cause and special cause variation. Common cause variation represents the inherent variability in the process, while special cause variation signifies exceptional factors causing deviations from the norm. This distinction helps in setting realistic improvement goals and understanding the scope of the project.
- Data Collection and Analysis (Measure Phase): The Measure phase involves collecting data to quantify the performance of the process and determine its capability. Six Sigma practitioners use statistical tools and control charts to identify patterns in the data. Control charts help distinguish between common cause and special cause variation. Common cause variation is typically represented by data points within control limits, while data points beyond these limits suggest special cause variation.
- Root Cause Analysis (Analyze Phase): Once special cause variation is identified in the Measure phase, the Analyze phase focuses on determining the specific causes of these exceptional variations. Root cause analysis techniques, such as the “5 Whys,” Fishbone diagrams, or Failure Modes and Effects Analysis (FMEA), are employed to understand the underlying factors responsible for special cause variation. Addressing these root causes is critical for process improvement.
- Improvement Actions (Improve Phase): In the Improve phase , the Six Sigma team devises and implements solutions to eliminate or mitigate the root causes of special cause variation. Improvement actions are carefully planned, tested, and validated to ensure that the process becomes more stable and predictable.
- Monitoring and Control (Control Phase): The Control phase is about sustaining the improvements made during the project. Common cause variation is continuously monitored through control charts, and process performance is measured against the new standards. The control plan, established in this phase, ensures that the process remains in control and that deviations due to common cause variation are promptly identified and addressed.
- Continuous Improvement: Six Sigma is inherently focused on continuous improvement. Common cause variation is always present but can be further reduced and managed through ongoing efforts. Teams conduct periodic reviews and data analysis to detect changes in process performance and address any new sources of special cause variation.
Six Sigma projects involve a structured approach to addressing both common cause and special cause variation. While common cause variation represents the natural variability in a process, special cause variation results from specific, identifiable issues.
A Six Sigma project aims to minimize both types of variation to improve process performance and quality. This requires a combination of data analysis, root cause analysis, process improvement efforts, and ongoing monitoring to ensure that the improvements are sustained over time.
In the world of statistics and quality control, understanding the concepts of common cause and special cause variation is vital for making informed decisions and improving processes. Common cause variation is the inherent, expected variation in a process, while special cause variation represents unusual and identifiable sources of variability. By distinguishing between these two types of variation, organizations can work towards greater process stability, predictability, and overall quality improvement.
Six Sigma Certifications
Six Sigma Resource Center
Common Cause and Special Cause Data (.pdf)
- All Certifications
- Accessibility

Connect With Us
Copyright MSI. All Rights Reserved.
Operations Management: An Integrated Approach, 5th Edition by
Get full access to Operations Management: An Integrated Approach, 5th Edition and 60K+ other titles, with a free 10-day trial of O'Reilly.
There are also live events, courses curated by job role, and more.
SOURCES OF VARIATION: COMMON AND ASSIGNABLE CAUSES
If you look at bottles of a soft drink in a grocery store, you will notice that no two bottles are filled to exactly the same level. Some are filled slightly higher and some slightly lower. Similarly, if you look at blueberry muffins in a bakery, you will notice that some are slightly larger than others and some have more blueberries than others. These types of differences are completely normal. No two products are exactly alike because of slight differences in materials, workers, machines, tools, and other factors. These are called common , or random, causes of variation . Common causes of variation are based on random causes that we cannot identify. These types of variation are unavoidable and are due to slight differences in processing.

Random causes that cannot be identified.
An important task in quality control is to find out the range of natural random variation in a process. For example, if the average bottle of a soft drink called Cocoa Fizz contains 16 ounces of liquid, we may determine that the amount of natural variation is between 15.8 and 16.2 ounces. If this were the case, we would monitor the production process to make sure that the amount stays within this range. If production goes out of this range—bottles are found to contain on average 15.6 ounces—this would lead us to believe that there ...
Get Operations Management: An Integrated Approach, 5th Edition now with the O’Reilly learning platform.
O’Reilly members experience books, live events, courses curated by job role, and more from O’Reilly and nearly 200 top publishers.
Don’t leave empty-handed
Get Mark Richards’s Software Architecture Patterns ebook to better understand how to design components—and how they should interact.
It’s yours, free.
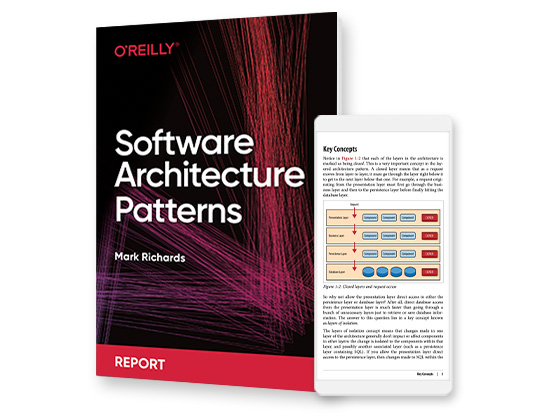
Check it out now on O’Reilly
Dive in for free with a 10-day trial of the O’Reilly learning platform—then explore all the other resources our members count on to build skills and solve problems every day.
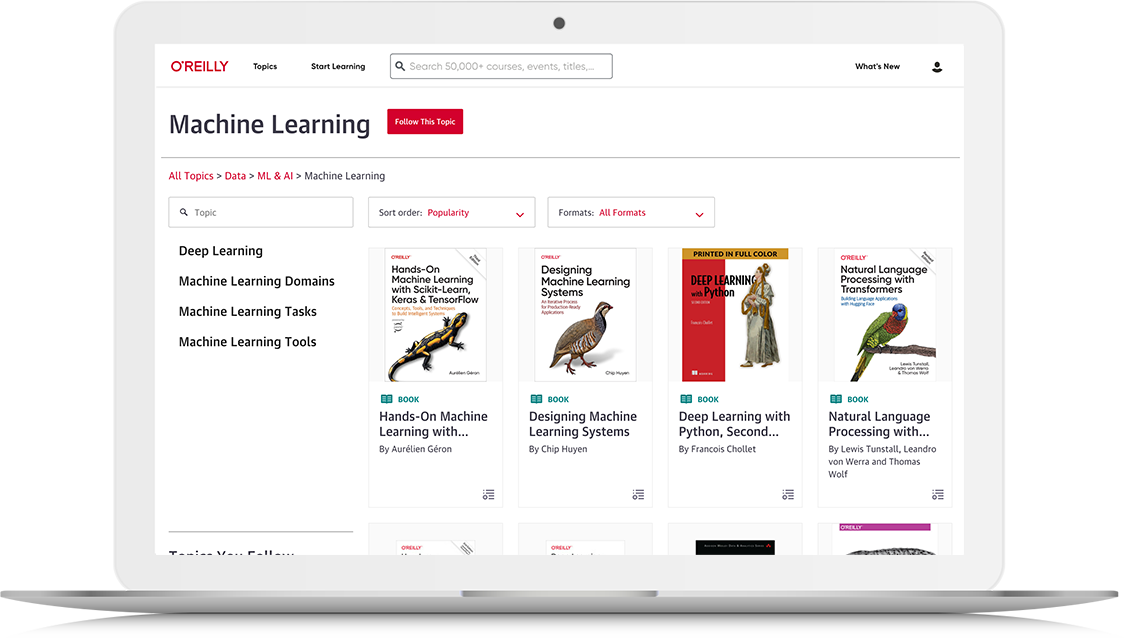
Using control charts to detect common-cause variation and special-cause variation
In this topic, what are common-cause variation and special-cause variation, what special-cause variation looks like on a control chart, using brainstorming to investigate special-cause variation, don't overcorrect your process for common-cause variation.
Some degree of variation will naturally occur in any process. Common-cause variation is the natural or expected variation in a process. Special-cause variation is unexpected variation that results from unusual occurrences. It is important to identify and try to eliminate special-cause variation. Out-of-control points and nonrandom patterns on a control chart indicate the presence of special-cause variation.
Examples of common-cause and special-cause variation
A process is stable if it does not contain any special-cause variation; only common-cause variation is present. Control charts and run charts provide good illustrations of process stability or instability. A process must be stable before its capability is assessed or improvements are initiated.
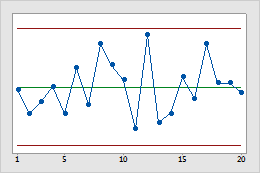
This process is stable because the data appear to be distributed randomly and do not violate any of the 8 control chart tests.
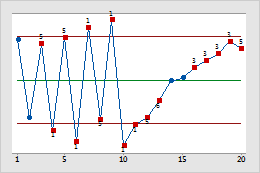
This process is not stable; several of the control chart tests are violated.
A good starting point in investigating special-cause variation is to gather several process experts together. Using the control chart, encourage the process operators, the process engineers, and the quality testers to brainstorm why particular samples were out of control. Depending on your process, you may also want to include the suppliers in this meeting.
- Which samples were out of control?
- Which tests for special causes did the samples fail?
- What does each failed test mean?
- What are all the possible reasons for the failed test?
A common method for brainstorming is to ask questions about why a particular failure occurred to determine the root cause (the 5 why method). You could also use a cause-and-effect diagram (also called fishbone diagram).
While it's important to avoid special-cause variation, trying to eliminate common-cause variation can make matters worse. Consider a bread baking process. Slight drifts in temperature that are caused by the oven's thermostat are part of the natural common-cause variation for the process. If you try to reduce this natural process variation by manually adjusting the temperature setting up and down, you will probably increase variability rather than decrease it. This is called overcorrection.
- Minitab.com
- License Portal
- Cookie Settings
You are now leaving support.minitab.com.
Click Continue to proceed to:
- Skip to primary navigation
- Skip to main content
- Skip to footer
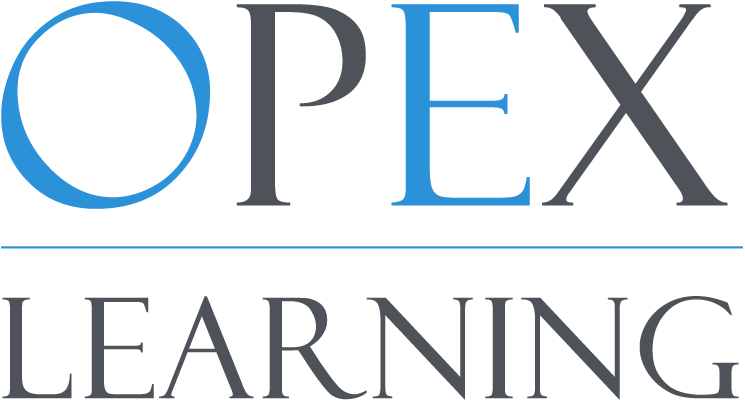
OpEx Learning Resources
Lean Six Sigma Articles, Guides, Insights, and more!
Common Cause vs. Special Cause: The Basics of Process Variation
By OpEx Learning Team , Last Updated April 26, 2018
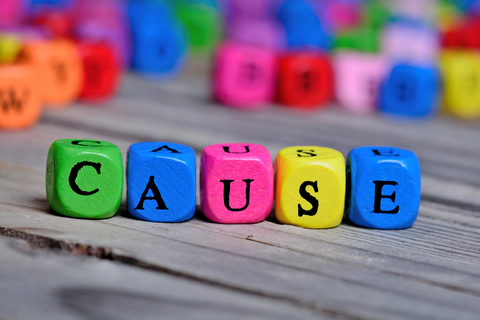
When dealing with process variation, you’ll need to learn to recognize the two general types of it, and the ways they impact the operation of your business. In most cases, some level of variance to a process is inevitable: the question is whether the factors that cause this variation are controlled and predicted, and if you can account for that variation in your output projections. If you’re able to work around it, there’s usually no big issue but things tend to get messier once special cause variation is introduced to the mix.
Common Cause Variation
Common cause variation is variation resulting from factors that may or may not be known, but the final impact they have on your output is predictable and controllable, and you would usually know to expect some variation in specific areas when looking at your final reports. As we said above, some level of variation is inevitable for most types of processes, and this is usually the kind you want to have if you want to be sure that you’re in control of the current situation.
Note that in some cases, common cause variation can still be quite high, and this might cause issues in your production. Take steps to reduce it if you are able to identify the root causes, but don’t stress too much if you can’t get to the bottom of the situation.
Special Cause Variation
On the other hand, special cause variation occurs when something out of the ordinary happens in a process. This might be a one-time occurrence, or it can develop into a repeating effect, in which case it might eventually be categorized as common cause variation depending on the circumstances. It’s important to understand that sometimes you will simply not be able to avoid special cause variation, either because the nature of a process is prone to it, or because of external factors in your environment that are beyond your control.
When you’ve identified special cause variation though, it’s important to take prompt steps to deal with it. It may not be such a problematic factor right now, but if you leave it unattended for a long time, this might be a real problem that can bring down some parts of your operations. There are some tools that can help you figure out where some issues are coming from, but you may need to come up with some solutions of your own in some cases, depending on the complexity of the situation.
Identifying Both with the Appropriate Tools
This brings us back to the most important point you need to be aware of what tools are available in your area of work for dealing with problems like that, and know how to apply them to your current situation in order to figure out where different types of variation are coming from, and develop an adequate solution. Special cause variation is typically easier to uncover as it will deviate from the norm more strongly, but there might be some exceptions. On the other hand, when dealing with common cause variation, you might have access to more historic data that can give you a better idea of what’s going on with the help of some statistical analysis.
Once you’ve learned what common cause and special cause variation both are, and how they relate to your line of work, you should be more prepared to identify and deal with them, and you will find them less problematic in the long run as impact on your operations will be minimized and kept to a controllable state where you can drive things in the right direction.
Become a Lean Six Sigma professional today!
Start your learning journey with Lean Six Sigma White Belt at NO COST
Use coupon code:
Related posts:.
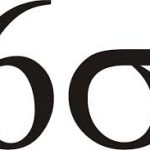
Subscribe to our newsletter
Get the latest posts delivered right to your inbox. We do not spam you and you can always unsubscribe later any time.
Reader Interactions
Leave a reply cancel reply.
Your email address will not be published. Required fields are marked *
Save my name, email, and website in this browser for the next time I comment.
This site uses Akismet to reduce spam. Learn how your comment data is processed .
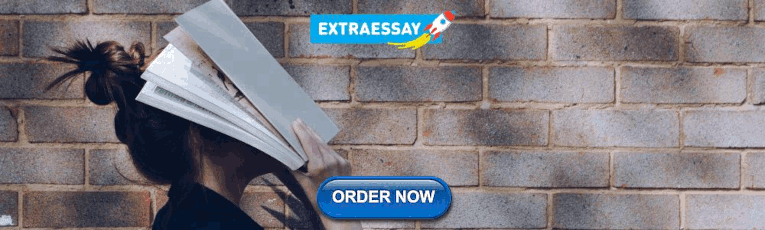
IMAGES
VIDEO
COMMENTS
A cycle or repeating pattern. A run of 8 or more consecutive points on either side of the average or center line. Assignable cause variation can be attributed to a defect, fault, mistake, delay, breakdown, accident, and/or shortage in the process. Or it can be a result of some unique combination of factors coming together to actually improve ...
assignable variation. in process output, a variation whose cause can be identified; non-random variation. attributes. generate data that are counted. ... used to distinguish between random and nonrandom variability. control limits. the dividing lines between random and nonrandom deviations from the mean of the distribution. inspection.
Assignable variation- in process output, a variation whose cause can be identified . Control chart- a time-ordered plot of sample statistics, used to distinguish between random and nonrandom variability . Control limits- the dividing lines between random and nonrandom deviations from the mean of the distribution
cause or assignable cause variations and the random or irregular type of variations. The goal of any process, then, will be to identify the causes and eliminate them to make an unstable process into a stable process. A process with only common cause variation is called stable. 4.1.1 Special Cause Variation
The difference between these two types of variation lies in how common cause variations are normal and expected variations that do not deviate from the natural order of a process. With common cause variations, a process remains stable. With special cause variations, however, a process is dramatically affected and becomes unstable.
Also known as nonrandom or assignable variation. It is important to understand these two types of variation because you should only try to improve a process that reflects random variation. This is because a process demonstrating random variation is stable and therefore predictable. Being stable and predictable, however, does not mean that the ...
The approach to managing variation depends on the priorities and perspectives of the improvement leader and the intended generalizability of the results of the improvement effort. Clinical researchers, healthcare managers, and individual patients each have different goals, time horizons, and methodological approaches to managing variation ...
Assignable causes of variation are present in most production processes. These causes of variability are also called special causes of variation ( Deming, 1982 ). The sources of assignable variation can usually be identified (assigned to a specific cause) leading to their elimination. Tool wear, equipment that needs adjustment, defective ...
A cycle or repeating pattern. A run: 8 or more points on either side of the average. A special cause of variation is assignable to a defect, fault, mistake, delay, breakdown, accident, and/or shortage in the process. When special causes are present, process quality is unpredictable. Special causes are a signal for you to act to make the process ...
Variation inherently unpredictable, even probabilistically; Variation outside the historical experience base; and; Evidence of some inherent change in the system or our knowledge of it. Special-cause variation always arrives as a surprise. It is the signal within a system. Walter A. Shewhart originally used the term assignable cause.
Managing Variation over Time • Statistical Process Control often takes the form of a continuous Hypothesis testing. • The idea is to detect, as quickly as possible, a significant departure from the norm. • A significant change is often attributed to what is known as an assignable cause. •An assignable cause is something that can be
Special cause variation, also known as assignable variation or non-random variation, is the opposite of common cause variation. It represents variability in a process that can be traced back to specific, identifiable causes. Unlike common cause variation, which is inherent to the process, special cause variation is due to external factors or ...
Common causes of variation are based on random causes that we cannot identify. These types of variation are unavoidable and are due to slight differences in processing. Common causes of variation. Random causes that cannot be identified. An important task in quality control is to find out the range of natural random variation in a process.
- Common cause variation or random variation: Natural variations in the output of a process, created by countless minor factors. Random variation is usually left alone. - Special cause or nonrandom variation: A variation whose source can be identified to be nonrandom. When there is special nonrandom variation, we call it 'out of control'.
cause variation. Special causes of variation are a signal that something has changed in the process. Special causes are evidenced by a disrup-tion of the stable, repeating pattern of variation. Special causes of variation result in unpredictable process performance and must therefore be identified and removed before taking other steps to ...
Some degree of variation will naturally occur in any process. Common-cause variation is the natural or expected variation in a process. Special-cause variation is unexpected variation that results from unusual occurrences. It is important to identify and try to eliminate special-cause variation. Out-of-control points and nonrandom patterns on a ...
On the other hand, special cause variation occurs when something out of the ordinary happens in a process. This might be a one-time occurrence, or it can develop into a repeating effect, in which case it might eventually be categorized as common cause variation depending on the circumstances. It's important to understand that sometimes you ...
In answering that question, it becomes crucial to understand the difference between common-cause and special-cause variation (as will be discussed later). Common-cause variation appears as random variation in all measures from healthcare processes. 1 Special-cause variation appears as the effect of causes outside the core processes of the work ...
Should be investigated because an assignable cause of variation might be present. Points outside of the control limits should be investigated as signals of nonrandom variation being present. If a point on a control chart falls outside one of the control limits, this suggests that the process output is nonrandom and should be investigated.
This paper presents a fresh approach to the analysis of Shewhart control chart's performance. We consider two different types of assignable causes of variation. One—called type I—affects only the parameters of a model of the underlying distribution. The other—called type X—impacts the type of the original distribution.
Nonrandom Variation-"Special cause"-The event may be a shift in a process mean or some unexpected occurrence. Random Samples-Randomization is useful because it ensures independence among observations-To randomize means to sample in such a way that every piece of product has an equal chance of being selected for inspection.
C. are intrinsic to the process and will always be there unless the process is changed. D. have everything to do with the underlying process and can only be eliminated by changing the process. o E. are of secondary importance in quality control procedures used to detect and eliminate variation.