Have a language expert improve your writing
Run a free plagiarism check in 10 minutes, generate accurate citations for free.
- Knowledge Base
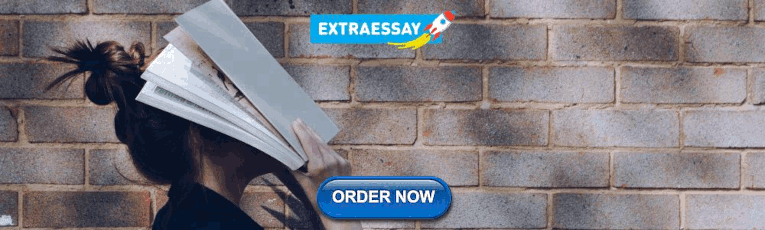
The Beginner's Guide to Statistical Analysis | 5 Steps & Examples
Statistical analysis means investigating trends, patterns, and relationships using quantitative data . It is an important research tool used by scientists, governments, businesses, and other organizations.
To draw valid conclusions, statistical analysis requires careful planning from the very start of the research process . You need to specify your hypotheses and make decisions about your research design, sample size, and sampling procedure.
After collecting data from your sample, you can organize and summarize the data using descriptive statistics . Then, you can use inferential statistics to formally test hypotheses and make estimates about the population. Finally, you can interpret and generalize your findings.
This article is a practical introduction to statistical analysis for students and researchers. We’ll walk you through the steps using two research examples. The first investigates a potential cause-and-effect relationship, while the second investigates a potential correlation between variables.
Table of contents
Step 1: write your hypotheses and plan your research design, step 2: collect data from a sample, step 3: summarize your data with descriptive statistics, step 4: test hypotheses or make estimates with inferential statistics, step 5: interpret your results, other interesting articles.
To collect valid data for statistical analysis, you first need to specify your hypotheses and plan out your research design.
Writing statistical hypotheses
The goal of research is often to investigate a relationship between variables within a population . You start with a prediction, and use statistical analysis to test that prediction.
A statistical hypothesis is a formal way of writing a prediction about a population. Every research prediction is rephrased into null and alternative hypotheses that can be tested using sample data.
While the null hypothesis always predicts no effect or no relationship between variables, the alternative hypothesis states your research prediction of an effect or relationship.
- Null hypothesis: A 5-minute meditation exercise will have no effect on math test scores in teenagers.
- Alternative hypothesis: A 5-minute meditation exercise will improve math test scores in teenagers.
- Null hypothesis: Parental income and GPA have no relationship with each other in college students.
- Alternative hypothesis: Parental income and GPA are positively correlated in college students.
Planning your research design
A research design is your overall strategy for data collection and analysis. It determines the statistical tests you can use to test your hypothesis later on.
First, decide whether your research will use a descriptive, correlational, or experimental design. Experiments directly influence variables, whereas descriptive and correlational studies only measure variables.
- In an experimental design , you can assess a cause-and-effect relationship (e.g., the effect of meditation on test scores) using statistical tests of comparison or regression.
- In a correlational design , you can explore relationships between variables (e.g., parental income and GPA) without any assumption of causality using correlation coefficients and significance tests.
- In a descriptive design , you can study the characteristics of a population or phenomenon (e.g., the prevalence of anxiety in U.S. college students) using statistical tests to draw inferences from sample data.
Your research design also concerns whether you’ll compare participants at the group level or individual level, or both.
- In a between-subjects design , you compare the group-level outcomes of participants who have been exposed to different treatments (e.g., those who performed a meditation exercise vs those who didn’t).
- In a within-subjects design , you compare repeated measures from participants who have participated in all treatments of a study (e.g., scores from before and after performing a meditation exercise).
- In a mixed (factorial) design , one variable is altered between subjects and another is altered within subjects (e.g., pretest and posttest scores from participants who either did or didn’t do a meditation exercise).
- Experimental
- Correlational
First, you’ll take baseline test scores from participants. Then, your participants will undergo a 5-minute meditation exercise. Finally, you’ll record participants’ scores from a second math test.
In this experiment, the independent variable is the 5-minute meditation exercise, and the dependent variable is the math test score from before and after the intervention. Example: Correlational research design In a correlational study, you test whether there is a relationship between parental income and GPA in graduating college students. To collect your data, you will ask participants to fill in a survey and self-report their parents’ incomes and their own GPA.
Measuring variables
When planning a research design, you should operationalize your variables and decide exactly how you will measure them.
For statistical analysis, it’s important to consider the level of measurement of your variables, which tells you what kind of data they contain:
- Categorical data represents groupings. These may be nominal (e.g., gender) or ordinal (e.g. level of language ability).
- Quantitative data represents amounts. These may be on an interval scale (e.g. test score) or a ratio scale (e.g. age).
Many variables can be measured at different levels of precision. For example, age data can be quantitative (8 years old) or categorical (young). If a variable is coded numerically (e.g., level of agreement from 1–5), it doesn’t automatically mean that it’s quantitative instead of categorical.
Identifying the measurement level is important for choosing appropriate statistics and hypothesis tests. For example, you can calculate a mean score with quantitative data, but not with categorical data.
In a research study, along with measures of your variables of interest, you’ll often collect data on relevant participant characteristics.
Here's why students love Scribbr's proofreading services
Discover proofreading & editing
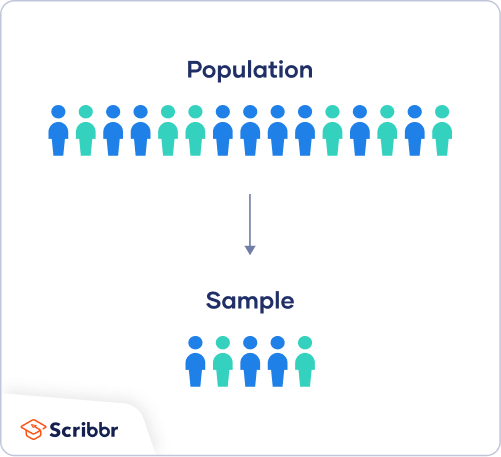
In most cases, it’s too difficult or expensive to collect data from every member of the population you’re interested in studying. Instead, you’ll collect data from a sample.
Statistical analysis allows you to apply your findings beyond your own sample as long as you use appropriate sampling procedures . You should aim for a sample that is representative of the population.
Sampling for statistical analysis
There are two main approaches to selecting a sample.
- Probability sampling: every member of the population has a chance of being selected for the study through random selection.
- Non-probability sampling: some members of the population are more likely than others to be selected for the study because of criteria such as convenience or voluntary self-selection.
In theory, for highly generalizable findings, you should use a probability sampling method. Random selection reduces several types of research bias , like sampling bias , and ensures that data from your sample is actually typical of the population. Parametric tests can be used to make strong statistical inferences when data are collected using probability sampling.
But in practice, it’s rarely possible to gather the ideal sample. While non-probability samples are more likely to at risk for biases like self-selection bias , they are much easier to recruit and collect data from. Non-parametric tests are more appropriate for non-probability samples, but they result in weaker inferences about the population.
If you want to use parametric tests for non-probability samples, you have to make the case that:
- your sample is representative of the population you’re generalizing your findings to.
- your sample lacks systematic bias.
Keep in mind that external validity means that you can only generalize your conclusions to others who share the characteristics of your sample. For instance, results from Western, Educated, Industrialized, Rich and Democratic samples (e.g., college students in the US) aren’t automatically applicable to all non-WEIRD populations.
If you apply parametric tests to data from non-probability samples, be sure to elaborate on the limitations of how far your results can be generalized in your discussion section .
Create an appropriate sampling procedure
Based on the resources available for your research, decide on how you’ll recruit participants.
- Will you have resources to advertise your study widely, including outside of your university setting?
- Will you have the means to recruit a diverse sample that represents a broad population?
- Do you have time to contact and follow up with members of hard-to-reach groups?
Your participants are self-selected by their schools. Although you’re using a non-probability sample, you aim for a diverse and representative sample. Example: Sampling (correlational study) Your main population of interest is male college students in the US. Using social media advertising, you recruit senior-year male college students from a smaller subpopulation: seven universities in the Boston area.
Calculate sufficient sample size
Before recruiting participants, decide on your sample size either by looking at other studies in your field or using statistics. A sample that’s too small may be unrepresentative of the sample, while a sample that’s too large will be more costly than necessary.
There are many sample size calculators online. Different formulas are used depending on whether you have subgroups or how rigorous your study should be (e.g., in clinical research). As a rule of thumb, a minimum of 30 units or more per subgroup is necessary.
To use these calculators, you have to understand and input these key components:
- Significance level (alpha): the risk of rejecting a true null hypothesis that you are willing to take, usually set at 5%.
- Statistical power : the probability of your study detecting an effect of a certain size if there is one, usually 80% or higher.
- Expected effect size : a standardized indication of how large the expected result of your study will be, usually based on other similar studies.
- Population standard deviation: an estimate of the population parameter based on a previous study or a pilot study of your own.
Once you’ve collected all of your data, you can inspect them and calculate descriptive statistics that summarize them.
Inspect your data
There are various ways to inspect your data, including the following:
- Organizing data from each variable in frequency distribution tables .
- Displaying data from a key variable in a bar chart to view the distribution of responses.
- Visualizing the relationship between two variables using a scatter plot .
By visualizing your data in tables and graphs, you can assess whether your data follow a skewed or normal distribution and whether there are any outliers or missing data.
A normal distribution means that your data are symmetrically distributed around a center where most values lie, with the values tapering off at the tail ends.
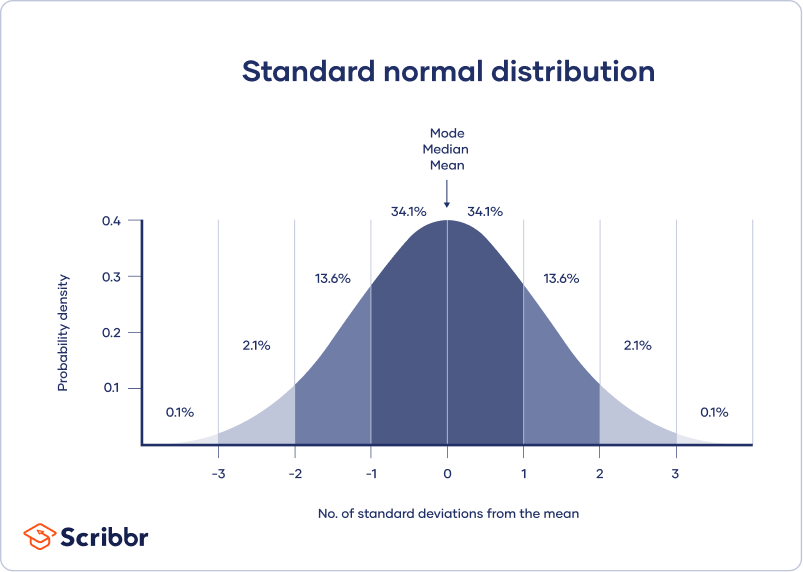
In contrast, a skewed distribution is asymmetric and has more values on one end than the other. The shape of the distribution is important to keep in mind because only some descriptive statistics should be used with skewed distributions.
Extreme outliers can also produce misleading statistics, so you may need a systematic approach to dealing with these values.
Calculate measures of central tendency
Measures of central tendency describe where most of the values in a data set lie. Three main measures of central tendency are often reported:
- Mode : the most popular response or value in the data set.
- Median : the value in the exact middle of the data set when ordered from low to high.
- Mean : the sum of all values divided by the number of values.
However, depending on the shape of the distribution and level of measurement, only one or two of these measures may be appropriate. For example, many demographic characteristics can only be described using the mode or proportions, while a variable like reaction time may not have a mode at all.
Calculate measures of variability
Measures of variability tell you how spread out the values in a data set are. Four main measures of variability are often reported:
- Range : the highest value minus the lowest value of the data set.
- Interquartile range : the range of the middle half of the data set.
- Standard deviation : the average distance between each value in your data set and the mean.
- Variance : the square of the standard deviation.
Once again, the shape of the distribution and level of measurement should guide your choice of variability statistics. The interquartile range is the best measure for skewed distributions, while standard deviation and variance provide the best information for normal distributions.
Using your table, you should check whether the units of the descriptive statistics are comparable for pretest and posttest scores. For example, are the variance levels similar across the groups? Are there any extreme values? If there are, you may need to identify and remove extreme outliers in your data set or transform your data before performing a statistical test.
From this table, we can see that the mean score increased after the meditation exercise, and the variances of the two scores are comparable. Next, we can perform a statistical test to find out if this improvement in test scores is statistically significant in the population. Example: Descriptive statistics (correlational study) After collecting data from 653 students, you tabulate descriptive statistics for annual parental income and GPA.
It’s important to check whether you have a broad range of data points. If you don’t, your data may be skewed towards some groups more than others (e.g., high academic achievers), and only limited inferences can be made about a relationship.
A number that describes a sample is called a statistic , while a number describing a population is called a parameter . Using inferential statistics , you can make conclusions about population parameters based on sample statistics.
Researchers often use two main methods (simultaneously) to make inferences in statistics.
- Estimation: calculating population parameters based on sample statistics.
- Hypothesis testing: a formal process for testing research predictions about the population using samples.
You can make two types of estimates of population parameters from sample statistics:
- A point estimate : a value that represents your best guess of the exact parameter.
- An interval estimate : a range of values that represent your best guess of where the parameter lies.
If your aim is to infer and report population characteristics from sample data, it’s best to use both point and interval estimates in your paper.
You can consider a sample statistic a point estimate for the population parameter when you have a representative sample (e.g., in a wide public opinion poll, the proportion of a sample that supports the current government is taken as the population proportion of government supporters).
There’s always error involved in estimation, so you should also provide a confidence interval as an interval estimate to show the variability around a point estimate.
A confidence interval uses the standard error and the z score from the standard normal distribution to convey where you’d generally expect to find the population parameter most of the time.
Hypothesis testing
Using data from a sample, you can test hypotheses about relationships between variables in the population. Hypothesis testing starts with the assumption that the null hypothesis is true in the population, and you use statistical tests to assess whether the null hypothesis can be rejected or not.
Statistical tests determine where your sample data would lie on an expected distribution of sample data if the null hypothesis were true. These tests give two main outputs:
- A test statistic tells you how much your data differs from the null hypothesis of the test.
- A p value tells you the likelihood of obtaining your results if the null hypothesis is actually true in the population.
Statistical tests come in three main varieties:
- Comparison tests assess group differences in outcomes.
- Regression tests assess cause-and-effect relationships between variables.
- Correlation tests assess relationships between variables without assuming causation.
Your choice of statistical test depends on your research questions, research design, sampling method, and data characteristics.
Parametric tests
Parametric tests make powerful inferences about the population based on sample data. But to use them, some assumptions must be met, and only some types of variables can be used. If your data violate these assumptions, you can perform appropriate data transformations or use alternative non-parametric tests instead.
A regression models the extent to which changes in a predictor variable results in changes in outcome variable(s).
- A simple linear regression includes one predictor variable and one outcome variable.
- A multiple linear regression includes two or more predictor variables and one outcome variable.
Comparison tests usually compare the means of groups. These may be the means of different groups within a sample (e.g., a treatment and control group), the means of one sample group taken at different times (e.g., pretest and posttest scores), or a sample mean and a population mean.
- A t test is for exactly 1 or 2 groups when the sample is small (30 or less).
- A z test is for exactly 1 or 2 groups when the sample is large.
- An ANOVA is for 3 or more groups.
The z and t tests have subtypes based on the number and types of samples and the hypotheses:
- If you have only one sample that you want to compare to a population mean, use a one-sample test .
- If you have paired measurements (within-subjects design), use a dependent (paired) samples test .
- If you have completely separate measurements from two unmatched groups (between-subjects design), use an independent (unpaired) samples test .
- If you expect a difference between groups in a specific direction, use a one-tailed test .
- If you don’t have any expectations for the direction of a difference between groups, use a two-tailed test .
The only parametric correlation test is Pearson’s r . The correlation coefficient ( r ) tells you the strength of a linear relationship between two quantitative variables.
However, to test whether the correlation in the sample is strong enough to be important in the population, you also need to perform a significance test of the correlation coefficient, usually a t test, to obtain a p value. This test uses your sample size to calculate how much the correlation coefficient differs from zero in the population.
You use a dependent-samples, one-tailed t test to assess whether the meditation exercise significantly improved math test scores. The test gives you:
- a t value (test statistic) of 3.00
- a p value of 0.0028
Although Pearson’s r is a test statistic, it doesn’t tell you anything about how significant the correlation is in the population. You also need to test whether this sample correlation coefficient is large enough to demonstrate a correlation in the population.
A t test can also determine how significantly a correlation coefficient differs from zero based on sample size. Since you expect a positive correlation between parental income and GPA, you use a one-sample, one-tailed t test. The t test gives you:
- a t value of 3.08
- a p value of 0.001
Prevent plagiarism. Run a free check.
The final step of statistical analysis is interpreting your results.
Statistical significance
In hypothesis testing, statistical significance is the main criterion for forming conclusions. You compare your p value to a set significance level (usually 0.05) to decide whether your results are statistically significant or non-significant.
Statistically significant results are considered unlikely to have arisen solely due to chance. There is only a very low chance of such a result occurring if the null hypothesis is true in the population.
This means that you believe the meditation intervention, rather than random factors, directly caused the increase in test scores. Example: Interpret your results (correlational study) You compare your p value of 0.001 to your significance threshold of 0.05. With a p value under this threshold, you can reject the null hypothesis. This indicates a statistically significant correlation between parental income and GPA in male college students.
Note that correlation doesn’t always mean causation, because there are often many underlying factors contributing to a complex variable like GPA. Even if one variable is related to another, this may be because of a third variable influencing both of them, or indirect links between the two variables.
Effect size
A statistically significant result doesn’t necessarily mean that there are important real life applications or clinical outcomes for a finding.
In contrast, the effect size indicates the practical significance of your results. It’s important to report effect sizes along with your inferential statistics for a complete picture of your results. You should also report interval estimates of effect sizes if you’re writing an APA style paper .
With a Cohen’s d of 0.72, there’s medium to high practical significance to your finding that the meditation exercise improved test scores. Example: Effect size (correlational study) To determine the effect size of the correlation coefficient, you compare your Pearson’s r value to Cohen’s effect size criteria.
Decision errors
Type I and Type II errors are mistakes made in research conclusions. A Type I error means rejecting the null hypothesis when it’s actually true, while a Type II error means failing to reject the null hypothesis when it’s false.
You can aim to minimize the risk of these errors by selecting an optimal significance level and ensuring high power . However, there’s a trade-off between the two errors, so a fine balance is necessary.
Frequentist versus Bayesian statistics
Traditionally, frequentist statistics emphasizes null hypothesis significance testing and always starts with the assumption of a true null hypothesis.
However, Bayesian statistics has grown in popularity as an alternative approach in the last few decades. In this approach, you use previous research to continually update your hypotheses based on your expectations and observations.
Bayes factor compares the relative strength of evidence for the null versus the alternative hypothesis rather than making a conclusion about rejecting the null hypothesis or not.
If you want to know more about statistics , methodology , or research bias , make sure to check out some of our other articles with explanations and examples.
- Student’s t -distribution
- Normal distribution
- Null and Alternative Hypotheses
- Chi square tests
- Confidence interval
Methodology
- Cluster sampling
- Stratified sampling
- Data cleansing
- Reproducibility vs Replicability
- Peer review
- Likert scale
Research bias
- Implicit bias
- Framing effect
- Cognitive bias
- Placebo effect
- Hawthorne effect
- Hostile attribution bias
- Affect heuristic
Is this article helpful?
Other students also liked.
- Descriptive Statistics | Definitions, Types, Examples
- Inferential Statistics | An Easy Introduction & Examples
- Choosing the Right Statistical Test | Types & Examples
More interesting articles
- Akaike Information Criterion | When & How to Use It (Example)
- An Easy Introduction to Statistical Significance (With Examples)
- An Introduction to t Tests | Definitions, Formula and Examples
- ANOVA in R | A Complete Step-by-Step Guide with Examples
- Central Limit Theorem | Formula, Definition & Examples
- Central Tendency | Understanding the Mean, Median & Mode
- Chi-Square (Χ²) Distributions | Definition & Examples
- Chi-Square (Χ²) Table | Examples & Downloadable Table
- Chi-Square (Χ²) Tests | Types, Formula & Examples
- Chi-Square Goodness of Fit Test | Formula, Guide & Examples
- Chi-Square Test of Independence | Formula, Guide & Examples
- Coefficient of Determination (R²) | Calculation & Interpretation
- Correlation Coefficient | Types, Formulas & Examples
- Frequency Distribution | Tables, Types & Examples
- How to Calculate Standard Deviation (Guide) | Calculator & Examples
- How to Calculate Variance | Calculator, Analysis & Examples
- How to Find Degrees of Freedom | Definition & Formula
- How to Find Interquartile Range (IQR) | Calculator & Examples
- How to Find Outliers | 4 Ways with Examples & Explanation
- How to Find the Geometric Mean | Calculator & Formula
- How to Find the Mean | Definition, Examples & Calculator
- How to Find the Median | Definition, Examples & Calculator
- How to Find the Mode | Definition, Examples & Calculator
- How to Find the Range of a Data Set | Calculator & Formula
- Hypothesis Testing | A Step-by-Step Guide with Easy Examples
- Interval Data and How to Analyze It | Definitions & Examples
- Levels of Measurement | Nominal, Ordinal, Interval and Ratio
- Linear Regression in R | A Step-by-Step Guide & Examples
- Missing Data | Types, Explanation, & Imputation
- Multiple Linear Regression | A Quick Guide (Examples)
- Nominal Data | Definition, Examples, Data Collection & Analysis
- Normal Distribution | Examples, Formulas, & Uses
- Null and Alternative Hypotheses | Definitions & Examples
- One-way ANOVA | When and How to Use It (With Examples)
- Ordinal Data | Definition, Examples, Data Collection & Analysis
- Parameter vs Statistic | Definitions, Differences & Examples
- Pearson Correlation Coefficient (r) | Guide & Examples
- Poisson Distributions | Definition, Formula & Examples
- Probability Distribution | Formula, Types, & Examples
- Quartiles & Quantiles | Calculation, Definition & Interpretation
- Ratio Scales | Definition, Examples, & Data Analysis
- Simple Linear Regression | An Easy Introduction & Examples
- Skewness | Definition, Examples & Formula
- Statistical Power and Why It Matters | A Simple Introduction
- Student's t Table (Free Download) | Guide & Examples
- T-distribution: What it is and how to use it
- Test statistics | Definition, Interpretation, and Examples
- The Standard Normal Distribution | Calculator, Examples & Uses
- Two-Way ANOVA | Examples & When To Use It
- Type I & Type II Errors | Differences, Examples, Visualizations
- Understanding Confidence Intervals | Easy Examples & Formulas
- Understanding P values | Definition and Examples
- Variability | Calculating Range, IQR, Variance, Standard Deviation
- What is Effect Size and Why Does It Matter? (Examples)
- What Is Kurtosis? | Definition, Examples & Formula
- What Is Standard Error? | How to Calculate (Guide with Examples)
What is your plagiarism score?
- Privacy Policy
Buy Me a Coffee

Home » Research Paper Outline – Types, Example, Template
Research Paper Outline – Types, Example, Template
Table of Contents

By creating a well-structured research paper outline, writers can easily organize their thoughts and ideas and ensure that their final paper is clear, concise, and effective. In this article, we will explore the essential components of a research paper outline and provide some tips and tricks for creating a successful one.
Research Paper Outline
Research paper outline is a plan or a structural framework that organizes the main ideas , arguments, and supporting evidence in a logical sequence. It serves as a blueprint or a roadmap for the writer to follow while drafting the actual research paper .
Typically, an outline consists of the following elements:
- Introduction : This section presents the topic, research question , and thesis statement of the paper. It also provides a brief overview of the literature review and the methodology used.
- Literature Review: This section provides a comprehensive review of the relevant literature, theories, and concepts related to the research topic. It analyzes the existing research and identifies the research gaps and research questions.
- Methodology: This section explains the research design, data collection methods, data analysis, and ethical considerations of the study.
- Results: This section presents the findings of the study, using tables, graphs, and statistics to illustrate the data.
- Discussion : This section interprets the results of the study, and discusses their implications, significance, and limitations. It also suggests future research directions.
- Conclusion : This section summarizes the main findings of the study and restates the thesis statement.
- References: This section lists all the sources cited in the paper using the appropriate citation style.
Research Paper Outline Types
There are several types of outlines that can be used for research papers, including:
Alphanumeric Outline
This is a traditional outline format that uses Roman numerals, capital letters, Arabic numerals, and lowercase letters to organize the main ideas and supporting details of a research paper. It is commonly used for longer, more complex research papers.
I. Introduction
- A. Background information
- B. Thesis statement
- 1 1. Supporting detail
- 1 2. Supporting detail 2
- 2 1. Supporting detail
III. Conclusion
- A. Restate thesis
- B. Summarize main points
Decimal Outline
This outline format uses numbers to organize the main ideas and supporting details of a research paper. It is similar to the alphanumeric outline, but it uses only numbers and decimals to indicate the hierarchy of the ideas.
- 1.1 Background information
- 1.2 Thesis statement
- 1 2.1.1 Supporting detail
- 1 2.1.2 Supporting detail
- 2 2.2.1 Supporting detail
- 1 2.2.2 Supporting detail
- 3.1 Restate thesis
- 3.2 Summarize main points
Full Sentence Outline
This type of outline uses complete sentences to describe the main ideas and supporting details of a research paper. It is useful for those who prefer to see the entire paper outlined in complete sentences.
- Provide background information on the topic
- State the thesis statement
- Explain main idea 1 and provide supporting details
- Discuss main idea 2 and provide supporting details
- Restate the thesis statement
- Summarize the main points of the paper
Topic Outline
This type of outline uses short phrases or words to describe the main ideas and supporting details of a research paper. It is useful for those who prefer to see a more concise overview of the paper.
- Background information
- Thesis statement
- Supporting detail 1
- Supporting detail 2
- Restate thesis
- Summarize main points
Reverse Outline
This is an outline that is created after the paper has been written. It involves going back through the paper and summarizing each paragraph or section in one sentence. This can be useful for identifying gaps in the paper or areas that need further development.
- Introduction : Provides background information and states the thesis statement.
- Paragraph 1: Discusses main idea 1 and provides supporting details.
- Paragraph 2: Discusses main idea 2 and provides supporting details.
- Paragraph 3: Addresses potential counterarguments.
- Conclusion : Restates thesis and summarizes main points.
Mind Map Outline
This type of outline involves creating a visual representation of the main ideas and supporting details of a research paper. It can be useful for those who prefer a more creative and visual approach to outlining.
- Supporting detail 1: Lack of funding for public schools.
- Supporting detail 2: Decrease in government support for education.
- Supporting detail 1: Increase in income inequality.
- Supporting detail 2: Decrease in social mobility.
Research Paper Outline Example
Research Paper Outline Example on Cyber Security:
A. Overview of Cybersecurity
- B. Importance of Cybersecurity
- C. Purpose of the paper
II. Cyber Threats
A. Definition of Cyber Threats
- B. Types of Cyber Threats
- C. Examples of Cyber Threats
III. Cybersecurity Measures
A. Prevention measures
- Anti-virus software
- Encryption B. Detection measures
- Intrusion Detection System (IDS)
- Security Information and Event Management (SIEM)
- Security Operations Center (SOC) C. Response measures
- Incident Response Plan
- Business Continuity Plan
- Disaster Recovery Plan
IV. Cybersecurity in the Business World
A. Overview of Cybersecurity in the Business World
B. Cybersecurity Risk Assessment
C. Best Practices for Cybersecurity in Business
V. Cybersecurity in Government Organizations
A. Overview of Cybersecurity in Government Organizations
C. Best Practices for Cybersecurity in Government Organizations
VI. Cybersecurity Ethics
A. Definition of Cybersecurity Ethics
B. Importance of Cybersecurity Ethics
C. Examples of Cybersecurity Ethics
VII. Future of Cybersecurity
A. Overview of the Future of Cybersecurity
B. Emerging Cybersecurity Threats
C. Advancements in Cybersecurity Technology
VIII. Conclusion
A. Summary of the paper
B. Recommendations for Cybersecurity
- C. Conclusion.
IX. References
A. List of sources cited in the paper
B. Bibliography of additional resources
Introduction
Cybersecurity refers to the protection of computer systems, networks, and sensitive data from unauthorized access, theft, damage, or any other form of cyber attack. B. Importance of Cybersecurity The increasing reliance on technology and the growing number of cyber threats make cybersecurity an essential aspect of modern society. Cybersecurity breaches can result in financial losses, reputational damage, and legal liabilities. C. Purpose of the paper This paper aims to provide an overview of cybersecurity, cyber threats, cybersecurity measures, cybersecurity in the business and government sectors, cybersecurity ethics, and the future of cybersecurity.
A cyber threat is any malicious act or event that attempts to compromise or disrupt computer systems, networks, or sensitive data. B. Types of Cyber Threats Common types of cyber threats include malware, phishing, social engineering, ransomware, DDoS attacks, and advanced persistent threats (APTs). C. Examples of Cyber Threats Recent cyber threats include the SolarWinds supply chain attack, the Colonial Pipeline ransomware attack, and the Microsoft Exchange Server hack.
Prevention measures aim to minimize the risk of cyber attacks by implementing security controls, such as firewalls, anti-virus software, and encryption.
- Firewalls Firewalls act as a barrier between a computer network and the internet, filtering incoming and outgoing traffic to prevent unauthorized access.
- Anti-virus software Anti-virus software detects, prevents, and removes malware from computer systems.
- Encryption Encryption involves the use of mathematical algorithms to transform sensitive data into a code that can only be accessed by authorized individuals. B. Detection measures Detection measures aim to identify and respond to cyber attacks as quickly as possible, such as intrusion detection systems (IDS), security information and event management (SIEM), and security operations centers (SOCs).
- Intrusion Detection System (IDS) IDS monitors network traffic for signs of unauthorized access, such as unusual patterns or anomalies.
- Security Information and Event Management (SIEM) SIEM combines security information management and security event management to provide real-time monitoring and analysis of security alerts.
- Security Operations Center (SOC) SOC is a dedicated team responsible for monitoring, analyzing, and responding to cyber threats. C. Response measures Response measures aim to mitigate the impact of a cyber attack and restore normal operations, such as incident response plans (IRPs), business continuity plans (BCPs), and disaster recovery plans (DRPs).
- Incident Response Plan IRPs outline the procedures and protocols to follow in the event of a cyber attack, including communication protocols, roles and responsibilities, and recovery processes.
- Business Continuity Plan BCPs ensure that critical business functions can continue in the event of a cyber attack or other disruption.
- Disaster Recovery Plan DRPs outline the procedures to recover from a catastrophic event, such as a natural disaster or cyber attack.
Cybersecurity is crucial for businesses of all sizes and industries, as they handle sensitive data, financial transactions, and intellectual property that are attractive targets for cyber criminals.
Risk assessment is a critical step in developing a cybersecurity strategy, which involves identifying potential threats, vulnerabilities, and consequences to determine the level of risk and prioritize security measures.
Best practices for cybersecurity in business include implementing strong passwords and multi-factor authentication, regularly updating software and hardware, training employees on cybersecurity awareness, and regularly backing up data.
Government organizations face unique cybersecurity challenges, as they handle sensitive information related to national security, defense, and critical infrastructure.
Risk assessment in government organizations involves identifying and assessing potential threats and vulnerabilities, conducting regular audits, and complying with relevant regulations and standards.
Best practices for cybersecurity in government organizations include implementing secure communication protocols, regularly updating and patching software, and conducting regular cybersecurity training and awareness programs for employees.
Cybersecurity ethics refers to the ethical considerations involved in cybersecurity, such as privacy, data protection, and the responsible use of technology.
Cybersecurity ethics are crucial for maintaining trust in technology, protecting privacy and data, and promoting responsible behavior in the digital world.
Examples of cybersecurity ethics include protecting the privacy of user data, ensuring data accuracy and integrity, and implementing fair and unbiased algorithms.
The future of cybersecurity will involve a shift towards more advanced technologies, such as artificial intelligence (AI), machine learning, and quantum computing.
Emerging cybersecurity threats include AI-powered cyber attacks, the use of deepfakes and synthetic media, and the potential for quantum computing to break current encryption methods.
Advancements in cybersecurity technology include the development of AI and machine learning-based security tools, the use of blockchain for secure data storage and sharing, and the development of post-quantum encryption methods.
This paper has provided an overview of cybersecurity, cyber threats, cybersecurity measures, cybersecurity in the business and government sectors, cybersecurity ethics, and the future of cybersecurity.
To enhance cybersecurity, organizations should prioritize risk assessment and implement a comprehensive cybersecurity strategy that includes prevention, detection, and response measures. Additionally, organizations should prioritize cybersecurity ethics to promote responsible behavior in the digital world.
C. Conclusion
Cybersecurity is an essential aspect of modern society, and organizations must prioritize cybersecurity to protect sensitive data and maintain trust in technology.
for further reading
X. Appendices
A. Glossary of key terms
B. Cybersecurity checklist for organizations
C. Sample cybersecurity policy for businesses
D. Sample cybersecurity incident response plan
E. Cybersecurity training and awareness resources
Note : The content and organization of the paper may vary depending on the specific requirements of the assignment or target audience. This outline serves as a general guide for writing a research paper on cybersecurity. Do not use this in your assingmets.
Research Paper Outline Template
- Background information and context of the research topic
- Research problem and questions
- Purpose and objectives of the research
- Scope and limitations
II. Literature Review
- Overview of existing research on the topic
- Key concepts and theories related to the research problem
- Identification of gaps in the literature
- Summary of relevant studies and their findings
III. Methodology
- Research design and approach
- Data collection methods and procedures
- Data analysis techniques
- Validity and reliability considerations
- Ethical considerations
IV. Results
- Presentation of research findings
- Analysis and interpretation of data
- Explanation of significant results
- Discussion of unexpected results
V. Discussion
- Comparison of research findings with existing literature
- Implications of results for theory and practice
- Limitations and future directions for research
- Conclusion and recommendations
VI. Conclusion
- Summary of research problem, purpose, and objectives
- Discussion of significant findings
- Contribution to the field of study
- Implications for practice
- Suggestions for future research
VII. References
- List of sources cited in the research paper using appropriate citation style.
Note : This is just an template, and depending on the requirements of your assignment or the specific research topic, you may need to modify or adjust the sections or headings accordingly.
Research Paper Outline Writing Guide
Here’s a guide to help you create an effective research paper outline:
- Choose a topic : Select a topic that is interesting, relevant, and meaningful to you.
- Conduct research: Gather information on the topic from a variety of sources, such as books, articles, journals, and websites.
- Organize your ideas: Organize your ideas and information into logical groups and subgroups. This will help you to create a clear and concise outline.
- Create an outline: Begin your outline with an introduction that includes your thesis statement. Then, organize your ideas into main points and subpoints. Each main point should be supported by evidence and examples.
- Introduction: The introduction of your research paper should include the thesis statement, background information, and the purpose of the research paper.
- Body : The body of your research paper should include the main points and subpoints. Each point should be supported by evidence and examples.
- Conclusion : The conclusion of your research paper should summarize the main points and restate the thesis statement.
- Reference List: Include a reference list at the end of your research paper. Make sure to properly cite all sources used in the paper.
- Proofreading : Proofread your research paper to ensure that it is free of errors and grammatical mistakes.
- Finalizing : Finalize your research paper by reviewing the outline and making any necessary changes.
When to Write Research Paper Outline
It’s a good idea to write a research paper outline before you begin drafting your paper. The outline will help you organize your thoughts and ideas, and it can serve as a roadmap for your writing process.
Here are a few situations when you might want to consider writing an outline:
- When you’re starting a new research project: If you’re beginning a new research project, an outline can help you get organized from the very beginning. You can use your outline to brainstorm ideas, map out your research goals, and identify potential sources of information.
- When you’re struggling to organize your thoughts: If you find yourself struggling to organize your thoughts or make sense of your research, an outline can be a helpful tool. It can help you see the big picture of your project and break it down into manageable parts.
- When you’re working with a tight deadline : If you have a deadline for your research paper, an outline can help you stay on track and ensure that you cover all the necessary points. By mapping out your paper in advance, you can work more efficiently and avoid getting stuck or overwhelmed.
Purpose of Research Paper Outline
The purpose of a research paper outline is to provide a structured and organized plan for the writer to follow while conducting research and writing the paper. An outline is essentially a roadmap that guides the writer through the entire research process, from the initial research and analysis of the topic to the final writing and editing of the paper.
A well-constructed outline can help the writer to:
- Organize their thoughts and ideas on the topic, and ensure that all relevant information is included.
- Identify any gaps in their research or argument, and address them before starting to write the paper.
- Ensure that the paper follows a logical and coherent structure, with clear transitions between different sections.
- Save time and effort by providing a clear plan for the writer to follow, rather than starting from scratch and having to revise the paper multiple times.
Advantages of Research Paper Outline
Some of the key advantages of a research paper outline include:
- Helps to organize thoughts and ideas : An outline helps to organize all the different ideas and information that you want to include in your paper. By creating an outline, you can ensure that all the points you want to make are covered and in a logical order.
- Saves time and effort : An outline saves time and effort because it helps you to focus on the key points of your paper. It also helps you to identify any gaps or areas where more research may be needed.
- Makes the writing process easier : With an outline, you have a clear roadmap of what you want to write, and this makes the writing process much easier. You can simply follow your outline and fill in the details as you go.
- Improves the quality of your paper : By having a clear outline, you can ensure that all the important points are covered and in a logical order. This makes your paper more coherent and easier to read, which ultimately improves its overall quality.
- Facilitates collaboration: If you are working on a research paper with others, an outline can help to facilitate collaboration. By sharing your outline, you can ensure that everyone is on the same page and working towards the same goals.
About the author
Muhammad Hassan
Researcher, Academic Writer, Web developer
You may also like

How to Cite Research Paper – All Formats and...

Delimitations in Research – Types, Examples and...

Research Paper Format – Types, Examples and...

Research Design – Types, Methods and Examples

Research Paper Title – Writing Guide and Example

Research Paper Introduction – Writing Guide and...
How to create a helpful research paper outline
Last updated
21 December 2023
Reviewed by
You need to structure your research paper in an orderly way that makes it easy for readers to follow your reasoning and supporting data. That's where a research paper outline can help.
Writing a research paper outline will help you arrange your ideas logically and allow your final paper to flow. It will make the entire process more manageable and help you work out which details to include and which are better left out.
- What is a research paper outline?
Write your research paper outline before starting your first draft. The outline provides a map of how you will structure your ideas throughout the paper. A research paper outline will help you to be more efficient when ordering the sections of your thesis, rather than trying to make structural changes after finishing an entire first draft.
An outline consists of the main topics and subtopics of your paper, listed in a logical order. The main topics will become the sections of your research paper, and the subtopics reveal the content you want to include or discuss under the main topics.
Under each subtopic, you can also jot down items you don't want to forget to include in your research paper, such as:
Topic ideas
Paragraph ideas
Direct quotes
Once you start listing these under your main topics, you can focus your thoughts as you plan and write the research paper using the evidence and data you collected and any additional information.
- Why use an outline?
If your research paper does not have a clear, logical order, readers may not understand the ideas you're trying to share, or they may lose interest and not bother to read the whole paper. An outline helps you structure your research paper so readers can easily connect the content, ideas, and theories you're trying to prove or maintain.
- Are there different kinds of research paper outlines?
Different kinds of research paper outlines might seem similar but have different purposes. You can select an outline type that provides a clear road map and thoroughly explores each point.
Other types will help structure content logically or with a segmented flow and progression of ideas that align closely with the theme of your research.
- The 3 types of outlines
The three outline formats available to research paper writers are:
Alphanumeric or topic outlines
Sentence or full-sentence outlines
Decimal outlines
Let’s look at the differences between each type and see how one may be more beneficial than another, depending on the nature of your research.
This type of research paper outline allows you to segment main headings and subheadings with an alphanumeric arrangement.
The alphanumeric characters of Roman numerals, capital letters, numbers, and lowercase letters define the hierarchy of main topic headings, subtopic headings, and third- and fourth-tier subtopic headings. (e.g., I, A, 1, a)
This method uses minimal words to describe the main and subtopic headings. You'll mostly use this type of research paper outline to focus on the organization of the content while allowing you to review it for unrelated or irrelevant information.
Full-sentence outlines
You will format this type of research paper outline as an alphanumeric outline, using the same alphanumeric characters. However, it contains complete sentences rather than a few words for each main and subtopic heading.
This formatting method allows the writer to focus on looking for inaccuracies and inconsistencies in each point before starting the first draft.
Instead of using alphanumeric characters to define main headings, subheadings, and third- and fourth-tier subheadings, the decimal outline uses a decimal numbering system.
This system shows a logical progression of the content by using 1.0 for the main section heading (and 2.0, 3.0, etc., for subsequent sections), 1.1 for the subheading, 1.1.1 for a third-tier subheading, and 1.1.1.1 for the fourth-tier subheading.
The headings and subheadings will be just a few words, as in the alphanumerical research paper outline. Decimal outlines allow the writer to focus on the content's overall coherence, increasing your writing efficiency and reducing the time it takes to write your research paper.
- How to write a research paper outline
Before you begin your research paper outline, you need to determine your topic and gather your information. Let’s look at these steps first, then dive into how to write your outline.
1. Determine your topic
You'll need to establish a topic or the main point you intend to write about.
For example, you may want to research and write about whether influencers are the most beneficial way to promote products in your industry. This topic is the main point around which your essay will revolve.
2. Gather information
You'll need evidence, data, statistics, and facts to prove or disprove that influencers are the best method of promoting products in your industry.
You'll insert any of these things you collect to substantiate your findings into the outline to support your topic.
3. Determine the type of essay you'll be writing
There are many types of essays or research papers you can write. The kinds of essays include:
Argumentative: Builds logic and support for an argument
Cause and effect: Explains relationships between specific conditions and their results
Analytical: Presents a claim on what is being analyzed
Interpretive: Informative and persuasive explanations on how something is perceived
Experimental: Reports on experimental results and the reasoning behind the results
Review: Offers an understanding and analysis of primary sources on a given topic
Definition: Defines what a term or concept means
Persuasive: Uses logic and reason to show that one idea is more justified than another
Narrative: Tells a story of personal experience from the author’s point of view
Expository: Shows an objective view of a subject by exploring various angles
Descriptive: Describes objects, people, places, experiences, emotions, situations, etc.
Once you understand the essay format you are writing, you'll know how to structure your outline.
4. Include basic sections
You'll begin to structure your outline using basic sections. Your main topic headings for these sections may include an introduction, multiple body paragraph sections, and a conclusion.
Once you establish the sections, you can insert the subtopics under each main topic heading.
5. Organize your outline
For example, if you're writing an argumentative essay taking the position that brand influencers (e.g., social media stars on Instagram or TikTok) are the best way to promote products in your industry, you will argue for that particular position.
You'll organize your argumentative essay outline with a main topic section supporting the position. The subtopics will include the reasoning behind your arguments, and the third-tier subtopics will contain the supporting evidence and data you gathered during your research.
You'll add another main topic section to counter and respond to any opposing arguments. Once you've organized and included all the information in this way, this will provide the structure to start your argumentative essay draft.
6. Consider compare-and-contrast essays
A compare-and-contrast essay is a form of essay that analyzes the differences between two opposing theories or subjects. If you have multiple subjects that are the same or different in just one aspect, you can write a point-by-point outline exploring each subject in terms of this characteristic.
The main topic headings will list that one characteristic, and the subtopic headings will list the subjects or items that are the same or different in relation to this characteristic.
Conversely, if you have multiple items to compare, but they have many characteristics that are similar or different, you can write a block method outline. The main topic headings will contain the items to be compared, while the subtopic headings will contain the aspects in which they are similar or different.
7. Consider advanced organizers for longer essays
An advanced organizer is a sentence that introduces new topics by connecting already-known information to new information. It can also prepare the reader for what they may expect to learn from the entire essay, or each section or paragraph.
Incorporating advanced organizers makes it easier for the reader to process and understand the information you are trying to convey. If you choose to use advanced organizers, depending on how often you want to use them throughout your paper, you can add them to your outline at the end of the introduction, the beginning of a section, or the beginning of each paragraph.
- Do outlines need periods (full stops)?
If you're constructing alphanumerical or decimal topic outlines, they do not need periods because the entries are usually not complete sentences. However, outlines containing full sentences will need to be punctuated as any sentence is, including using periods.
- An example research paper outline
Here is an example of an alphanumerical outline that argues brand influencers are the best method of promoting products in a particular industry:
I. Introduction
A. Background information about the issue and the position being argued.
B. Thesis statement: Influencers are the best way to promote products in this industry.
II. Reasons that support the thesis statement
A. Reason or argument #1
1. Supporting evidence
2. Supporting evidence
B. Reason or argument #2
C. Reason or argument #3
1. Supporting evidence
2. Supporting evidence
III. Counterarguments and responses
A. Arguments from the other point of view
B. Rebuttals against those arguments
IV. Conclusion
- How long is a thesis outline?
There is no set length for a research paper outline or thesis outline. Your outline can be as long as it needs to be to organize your thoughts constructively.
You can start with a short outline containing an introduction , background, methodology, data and analysis, and conclusion. Or you can break these sections into more specific segments according to the content you want to share.
Why make writing a research paper more complicated than it needs to be? Knowing the elements of an outline and how to insert them into a cohesive structure will make your final paper understandable and interesting to the reader.
Understanding how to outline a research paper will make the writing process more efficient and less time-consuming.
Get started today
Go from raw data to valuable insights with a flexible research platform
Editor’s picks
Last updated: 21 December 2023
Last updated: 16 December 2023
Last updated: 6 October 2023
Last updated: 5 March 2024
Last updated: 25 November 2023
Last updated: 15 February 2024
Last updated: 11 March 2024
Last updated: 12 December 2023
Last updated: 6 March 2024
Last updated: 10 April 2023
Last updated: 20 December 2023
Latest articles
Related topics, log in or sign up.
Get started for free
Applied Statistics

11. Statistical report writing
Learning to write useful, productive and readable statistical reports is a critical data analysis skill.
- Undergraduate Statistics Project
- Statistical Report Writing
- The book R eport Writing for Data Science in R by Roger D. Peng (freely dowloaded from LeanPub) is a useful reference for statistical report writing, especially when using the R programming language.
- The book C ommunicating with Data by Deborah Nolan and Sara Stoudt consists of five parts. Part I helps the novice learn to write by reading the work of others. Part II delves into the specifics of how to describe data at a level appropriate for publication, create informative and effective visualizations, and communicate an analysis pipeline through well-written, reproducible code. Part III demonstrates how to reduce a data analysis to a compelling story and organize and write the first draft of a technical paper. Part IV addresses revision; this includes advice on writing about statistical findings in a clear and accurate way, general writing advice, and strategies for proof reading and revising. Part V offers advice about communication strategies beyond the page, which include giving talks, building a professional network, and participating in online communities
One model for a self-contained statistical report, especially one containing empirical methods, has the following sections:
- Give an informative title to your project.
- Assessment : Does the title give an accurate preview of what the report is about? Is it informative, specific and precise?
- The abstract provides a brief summary of the paper (background, methods, results and conclusions). The suggested length is no more than 150 words. This allows you approximately 1 sentence (and likely no more than 2 sentences) summarizing each of the following sections. Typically, abstracts are the last thing you write.
- Assessment : Are the main points of the report described clearly and succinctly?
- In this section you are providing the background of the report – the topic for analysis – and arguing its significance. Well-accepted facts or referenced statements should serve as the majority of content of this section. Typically, the background and significance section starts very broad and moves towards the specific area of analysis.
- Assessment :
Does the background and significance have a logical organization? Does it move from the general to the specific?
Has sufficient background been provided to understand the report?
Does this section end with statements about the goals of the report?
- Data collection . Explain how the data was collected/experiment was conducted.
- Variable creation . Detail the variables in your analysis and how they are defined (if necessary). For example, if you created a combined (frequency times quantity) drinking variable you should describe how. If you are talking about gender no further explanation is really needed.
- Analytic Methods . Explain the statistical procedures that will be used to analyze your data. E.g. Boxplots are used to illustrate differences in GPA across gender and class standing. Correlations are used to assess the impacts of gender and class standing on GPA.
- Assessment : Could the study be repeated based on the information given here? Is the material organized into logical categories (like those above)?
- Typically, results sections start with descriptive statistics, e.g. what percent of the sample is male/female, what is the mean GPA overall, in the different groups, etc. Figures can be nice to illustrate these differences! However, information presented must be relevant in helping to answer the research question(s) of interest. Typically, inferential (i.e. hypothesis tests) statistics come next. Tables can often be helpful for results from multiple regression. Do not give computer output here! This should look like a peer-reviewed journal article results section. Tables and figures should be labeled, embedded in the text, and referenced appropriately. The results section typically makes for fairly dry reading. It does not explain the impact of findings, it merely highlights and reports statistical information.
Is the content appropriate for a results section? Is there a clear description of the results?
Are the results/data analyzed well? Given the data in each figure/table is the interpretation accurate and logical? Is the analysis of the data thorough? Is anything relevant ignored?
Are the figures/tables appropriate for the data being discussed? Are the figure legends and titles clear and concise?
- Restate your objective and draw connections between your analyses and objective. In other words, how did (or didn’t) you answer/address your objective. Place these all in the larger scope of the relevant chapter of the text. Talk about the limitations of your findings and possible areas for future research to better investigate your research question. End with a concluding sentence or two that summarizes your key findings and impact on the field.
Do you clearly state whether the results answer any question posed?
Were specific data cited from the results to support each interpretation? Do you clearly articulate the basis for supporting or rejecting any hypotheses?
- You can copy & paste different style references from Google Scholar: here’s an example (click on the “Cite” link to see the different style citations).
- Assessment : Are references appropriate and of adequate quality? Are the cited properly (both in the text and at the end of the paper)?
Writing quality
- Is your report well-organized, with paragraphs organized in a logical manner?
- Is each paragraph well-written, with a clear topic sentence, and single major point?
- Is your report generally well-written, with good use of language, and sentence structure?
- Are tables and figures labeled correctly and referenced accordingly?
- Does the entire report flow and answer any question(s) sufficiently?
- Is there extraneous information presented (if so, delete it)?
Advice on writing statistical reports
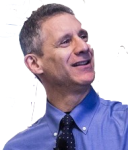
Andrew Gelman
Andrew Gelman , Professor of Statistics and Political Science and Director of the Applied Statistics Center at Columbia University, wrote the following as a guide to preparing statistical research articles; it works equally well for writing statistical reports.
Please try to follow it, and see how your planning for writing for, and actual writing of, statistical reports improves:
- Start writing the conclusions (the final part of your report, before the references). Write up to a couple pages on what you’ve found and what you recommend. In writing these conclusions, you should also be writing some of the introduction, in that you’ll need to give enough background so that general readers can understand what you’re talking about and why they should care. But you want to start with the conclusions, because that will determine what sort of background information you’ll need to give.
- Now step back. What is the principal evidence for your conclusions? Make some graphs and pull out some key numbers that represent your research findings which back up your claims.
- Back one more step, now. What are the methods and data you used to obtain your findings?
- Now go back and write the literature review and the introduction .
- Moving forward one last time: go to your results and conclusions and give alternative explanations . Why might you be wrong? What are the limits of applicability of your findings? What future work would be appropriate to follow up on these loose ends?
- Write the abstract . An easy way to start is to take the first sentence from each of the first five paragraphs of the article. This probably won’t be quite right, but I bet it will be close to what you need.
- Give the article to a friend, ask them to spend 15 minutes looking at it, then ask what they think your message was, and what evidence you have for it. Your friend should read the article as a potential consumer, not as a critic. You can find typos on your own time, but you need somebody else’s eyes to get a sense of the message you’re sending.

How Can You Create a Well Planned Research Paper Outline
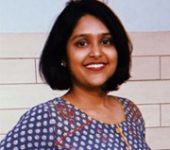
You are staring at the blank document, meaning to start writing your research paper . After months of experiments and procuring results, your PI asked you to write the paper to publish it in a reputed journal. You spoke to your peers and a few seniors and received a few tips on writing a research paper, but you still can’t plan on how to begin!
Writing a research paper is a very common issue among researchers and is often looked upon as a time consuming hurdle. Researchers usually look up to this task as an impending threat, avoiding and procrastinating until they cannot delay it anymore. Seeking advice from internet and seniors they manage to write a paper which goes in for quite a few revisions. Making researchers lose their sense of understanding with respect to their research work and findings. In this article, we would like to discuss how to create a structured research paper outline which will assist a researcher in writing their research paper effectively!
Publication is an important component of research studies in a university for academic promotion and in obtaining funding to support research. However, the primary reason is to provide the data and hypotheses to scientific community to advance the understanding in a specific domain. A scientific paper is a formal record of a research process. It documents research protocols, methods, results, conclusion, and discussion from a research hypothesis .
Table of Contents
What Is a Research Paper Outline?
A research paper outline is a basic format for writing an academic research paper. It follows the IMRAD format (Introduction, Methods, Results, and Discussion). However, this format varies depending on the type of research manuscript. A research paper outline consists of following sections to simplify the paper for readers. These sections help researchers build an effective paper outline.
1. Title Page
The title page provides important information which helps the editors, reviewers, and readers identify the manuscript and the authors at a glance. It also provides an overview of the field of research the research paper belongs to. The title should strike a balance between precise and detailed. Other generic details include author’s given name, affiliation, keywords that will provide indexing, details of the corresponding author etc. are added to the title page.
2. Abstract
Abstract is the most important section of the manuscript and will help the researcher create a detailed research paper outline . To be more precise, an abstract is like an advertisement to the researcher’s work and it influences the editor in deciding whether to submit the manuscript to reviewers or not. Writing an abstract is a challenging task. Researchers can write an exemplary abstract by selecting the content carefully and being concise.
3. Introduction
An introduction is a background statement that provides the context and approach of the research. It describes the problem statement with the assistance of the literature study and elaborates the requirement to update the knowledge gap. It sets the research hypothesis and informs the readers about the big research question.
This section is usually named as “Materials and Methods”, “Experiments” or “Patients and Methods” depending upon the type of journal. This purpose provides complete information on methods used for the research. Researchers should mention clear description of materials and their use in the research work. If the methods used in research are already published, give a brief account and refer to the original publication. However, if the method used is modified from the original method, then researcher should mention the modifications done to the original protocol and validate its accuracy, precision, and repeatability.
It is best to report results as tables and figures wherever possible. Also, avoid duplication of text and ensure that the text summarizes the findings. Report the results with appropriate descriptive statistics. Furthermore, report any unexpected events that could affect the research results, and mention complete account of observations and explanations for missing data (if any).
6. Discussion
The discussion should set the research in context, strengthen its importance and support the research hypothesis. Summarize the main results of the study in one or two paragraphs and show how they logically fit in an overall scheme of studies. Compare the results with other investigations in the field of research and explain the differences.
7. Acknowledgments
Acknowledgements identify and thank the contributors to the study, who are not under the criteria of co-authors. It also includes the recognition of funding agency and universities that award scholarships or fellowships to researchers.
8. Declaration of Competing Interests
Finally, declaring the competing interests is essential to abide by ethical norms of unique research publishing. Competing interests arise when the author has more than one role that may lead to a situation where there is a conflict of interest.
Steps to Write a Research Paper Outline
- Write down all important ideas that occur to you concerning the research paper .
- Answer questions such as – what is the topic of my paper? Why is the topic important? How to formulate the hypothesis? What are the major findings?
- Add context and structure. Group all your ideas into sections – Introduction, Methods, Results, and Discussion/Conclusion.
- Add relevant questions to each section. It is important to note down the questions. This will help you align your thoughts.
- Expand the ideas based on the questions created in the paper outline.
- After creating a detailed outline, discuss it with your mentors and peers.
- Get enough feedback and decide on the journal you will submit to.
- The process of real writing begins.
Benefits of Creating a Research Paper Outline
As discussed, the research paper subheadings create an outline of what different aspects of research needs elaboration. This provides subtopics on which the researchers brainstorm and reach a conclusion to write. A research paper outline organizes the researcher’s thoughts and gives a clear picture of how to formulate the research protocols and results. It not only helps the researcher to understand the flow of information but also provides relation between the ideas.
A research paper outline helps researcher achieve a smooth transition between topics and ensures that no research point is forgotten. Furthermore, it allows the reader to easily navigate through the research paper and provides a better understanding of the research. The paper outline allows the readers to find relevant information and quotes from different part of the paper.
Research Paper Outline Template
A research paper outline template can help you understand the concept of creating a well planned research paper before beginning to write and walk through your journey of research publishing.
1. Research Title
A. Background i. Support with evidence ii. Support with existing literature studies
B. Thesis Statement i. Link literature with hypothesis ii. Support with evidence iii. Explain the knowledge gap and how this research will help build the gap 4. Body
A. Methods i. Mention materials and protocols used in research ii. Support with evidence
B. Results i. Support with tables and figures ii. Mention appropriate descriptive statistics
C. Discussion i. Support the research with context ii. Support the research hypothesis iii. Compare the results with other investigations in field of research
D. Conclusion i. Support the discussion and research investigation ii. Support with literature studies
E. Acknowledgements i. Identify and thank the contributors ii. Include the funding agency, if any
F. Declaration of Competing Interests
5. References
Download the Research Paper Outline Template!
Have you tried writing a research paper outline ? How did it work for you? Did it help you achieve your research paper writing goal? Do let us know about your experience in the comments below.

Downloadable format shared which is great. 🙂
Rate this article Cancel Reply
Your email address will not be published.
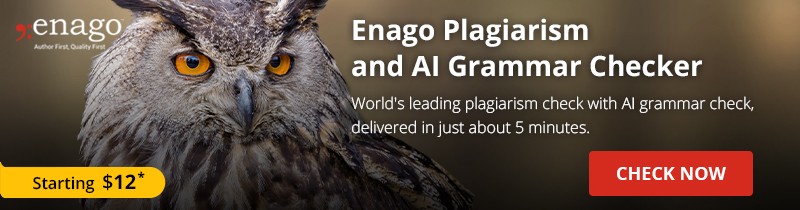
Enago Academy's Most Popular Articles
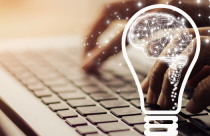
- AI in Academia
- Trending Now
Simplifying the Literature Review Journey — A comparative analysis of 6 AI summarization tools
Imagine having to skim through and read mountains of research papers and books, only to…
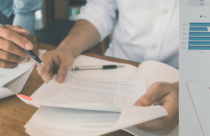
- Reporting Research
Choosing the Right Analytical Approach: Thematic analysis vs. content analysis for data interpretation
In research, choosing the right approach to understand data is crucial for deriving meaningful insights.…
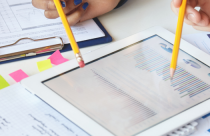
Comparing Cross Sectional and Longitudinal Studies: 5 steps for choosing the right approach
The process of choosing the right research design can put ourselves at the crossroads of…
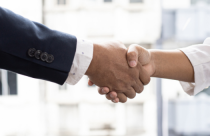
- Career Corner
Unlocking the Power of Networking in Academic Conferences
Embarking on your first academic conference experience? Fear not, we got you covered! Academic conferences…
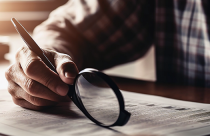
Research Recommendations – Guiding policy-makers for evidence-based decision making
Research recommendations play a crucial role in guiding scholars and researchers toward fruitful avenues of…
Setting Rationale in Research: Cracking the code for excelling at research
Mitigating Survivorship Bias in Scholarly Research: 10 tips to enhance data integrity
The Power of Proofreading: Taking your academic work to the next level
Facing Difficulty Writing an Academic Essay? — Here is your one-stop solution!

Sign-up to read more
Subscribe for free to get unrestricted access to all our resources on research writing and academic publishing including:
- 2000+ blog articles
- 50+ Webinars
- 10+ Expert podcasts
- 50+ Infographics
- 10+ Checklists
- Research Guides
We hate spam too. We promise to protect your privacy and never spam you.
I am looking for Editing/ Proofreading services for my manuscript Tentative date of next journal submission:
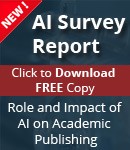
What should universities' stance be on AI tools in research and academic writing?
Reference management. Clean and simple.
Getting started with your research paper outline
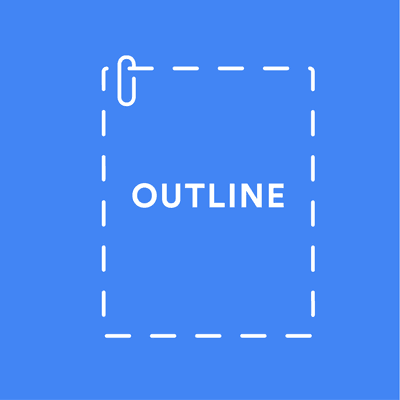
Levels of organization for a research paper outline
First level of organization, second level of organization, third level of organization, fourth level of organization, tips for writing a research paper outline, research paper outline template, my research paper outline is complete: what are the next steps, frequently asked questions about a research paper outline, related articles.
The outline is the skeleton of your research paper. Simply start by writing down your thesis and the main ideas you wish to present. This will likely change as your research progresses; therefore, do not worry about being too specific in the early stages of writing your outline.
A research paper outline typically contains between two and four layers of organization. The first two layers are the most generalized. Each layer thereafter will contain the research you complete and presents more and more detailed information.
The levels are typically represented by a combination of Roman numerals, Arabic numerals, uppercase letters, lowercase letters but may include other symbols. Refer to the guidelines provided by your institution, as formatting is not universal and differs between universities, fields, and subjects. If you are writing the outline for yourself, you may choose any combination you prefer.
This is the most generalized level of information. Begin by numbering the introduction, each idea you will present, and the conclusion. The main ideas contain the bulk of your research paper 's information. Depending on your research, it may be chapters of a book for a literature review , a series of dates for a historical research paper, or the methods and results of a scientific paper.
I. Introduction
II. Main idea
III. Main idea
IV. Main idea
V. Conclusion
The second level consists of topics which support the introduction, main ideas, and the conclusion. Each main idea should have at least two supporting topics listed in the outline.
If your main idea does not have enough support, you should consider presenting another main idea in its place. This is where you should stop outlining if this is your first draft. Continue your research before adding to the next levels of organization.
- A. Background information
- B. Hypothesis or thesis
- A. Supporting topic
- B. Supporting topic
The third level of organization contains supporting information for the topics previously listed. By now, you should have completed enough research to add support for your ideas.
The Introduction and Main Ideas may contain information you discovered about the author, timeframe, or contents of a book for a literature review; the historical events leading up to the research topic for a historical research paper, or an explanation of the problem a scientific research paper intends to address.
- 1. Relevant history
- 2. Relevant history
- 1. The hypothesis or thesis clearly stated
- 1. A brief description of supporting information
- 2. A brief description of supporting information
The fourth level of organization contains the most detailed information such as quotes, references, observations, or specific data needed to support the main idea. It is not typical to have further levels of organization because the information contained here is the most specific.
- a) Quotes or references to another piece of literature
- b) Quotes or references to another piece of literature
Tip: The key to creating a useful outline is to be consistent in your headings, organization, and levels of specificity.
- Be Consistent : ensure every heading has a similar tone. State the topic or write short sentences for each heading but avoid doing both.
- Organize Information : Higher levels of organization are more generally stated and each supporting level becomes more specific. The introduction and conclusion will never be lower than the first level of organization.
- Build Support : Each main idea should have two or more supporting topics. If your research does not have enough information to support the main idea you are presenting, you should, in general, complete additional research or revise the outline.
By now, you should know the basic requirements to create an outline for your paper. With a content framework in place, you can now start writing your paper . To help you start right away, you can use one of our templates and adjust it to suit your needs.

After completing your outline, you should:
- Title your research paper . This is an iterative process and may change when you delve deeper into the topic.
- Begin writing your research paper draft . Continue researching to further build your outline and provide more information to support your hypothesis or thesis.
- Format your draft appropriately . MLA 8 and APA 7 formats have differences between their bibliography page, in-text citations, line spacing, and title.
- Finalize your citations and bibliography . Use a reference manager like Paperpile to organize and cite your research.
- Write the abstract, if required . An abstract will briefly state the information contained within the paper, results of the research, and the conclusion.
An outline is used to organize written ideas about a topic into a logical order. Outlines help us organize major topics, subtopics, and supporting details. Researchers benefit greatly from outlines while writing by addressing which topic to cover in what order.
The most basic outline format consists of: an introduction, a minimum of three topic paragraphs, and a conclusion.
You should make an outline before starting to write your research paper. This will help you organize the main ideas and arguments you want to present in your topic.
- Consistency: ensure every heading has a similar tone. State the topic or write short sentences for each heading but avoid doing both.
- Organization : Higher levels of organization are more generally stated and each supporting level becomes more specific. The introduction and conclusion will never be lower than the first level of organization.
- Support : Each main idea should have two or more supporting topics. If your research does not have enough information to support the main idea you are presenting, you should, in general, complete additional research or revise the outline.
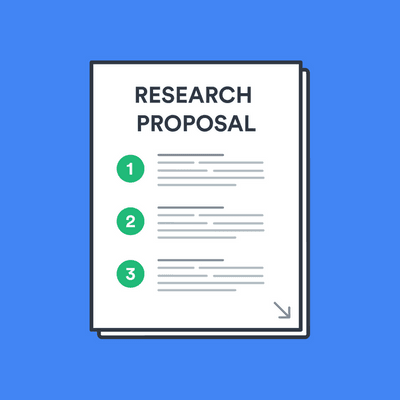
The Data Deep Dive: Statistical Analysis Guide

Table of contents
- 1 What Is Statistical Analysis and Its Role?
- 2 Preparing for Statistical Analysis
- 3 Data Collection and Management
- 4 Performing Descriptive Statistical Analysis
- 5 Performing Inferential Statistics Analysis
- 6 Writing the Statistical Research Paper
- 7 Common Mistakes in Statistical Analysis
- 8 Ethical Considerations
- 9 Concluding the Research Paper
- 10 Examples of Good and Poor Statistical Analysis in Research Paper
- 11 Key Insights: Navigating Statistical Analysis
Statistical analysis is fundamental if you need to reveal patterns or identify trends in datasets, employing numerical data analysis to eliminate bias and extract meaningful vision. Accordingly, it is crucial in research explanation, model evolution, and survey planning.
Statistical analysts make valuable results from the raw data, facilitating informed decision-making and predictive statistical analytics based on historical information.
Do you need to make a statistical analysis for your university studies? In this statistical study article, you will find instructions on how to write statistical analysis, as well as types of statistical analysis, statistical tools, and common mistakes students face.
What Is Statistical Analysis and Its Role?
Statistical analysis is the systematic process of collecting, organizing, and interpreting numbers to reveal patterns and identify trends and relationships. It plays a crucial role in research by providing tools to analyze data objectively, remove bias, and draw conclusions. Moreover, statistical analysis aids in identifying correlations, testing hypotheses, and making predictions, thereby informing decision-making in various fields such as computer science, medicine, economics, and social sciences. Thus, it enables quantitative data and statistical analytics researchers to assess results.
Struggling with how to analyze data in research? Feel free to address our specialists to get skilled and qualified help with research paper data analysis.
Preparing for Statistical Analysis
Preparing for statistical analysis requires some essential steps to ensure the validity and reliability of results.
- Firstly, formulating understandable and measurable questions is critical for valid statistics in a research paper. Questions lead the entire data analysis process and help define the scope of the study. Accordingly, scientists should develop specific, relevant, and capable issues that can be answered through statistical methods.
- Secondly, identifying appropriate data is vital. Picking an accordant data set that aligns with the investigations guarantees the analysis and business intelligence are focused and meaningful. For this purpose, researchers should consider data origin, quality, and responsibility when selecting data for analysis.
By scrupulously formulating problems and selecting appropriate statistical analytics data, researchers can lay a solid foundation for statistical analysis, guiding to strong and prudent results.
Data Collection and Management
Information collection and management are integral components of the statistical analysis process, ensuring the accuracy and reliability of results. Firstly, considering the techniques of data collection is essential. Here, researchers may employ primary approaches, such as examinations, interviews, or experiments, to gather direct information. Secondary methods involve utilizing existing data sources like databases, statistical analysis software, literature reviews, or archival records.
To collect data, specialists need to analyze:
- dependent variable;
- categorical variables;
- outcome variable;
- patterns and trends;
- alternative hypothesis states.
Once data is collected, organizing it is crucial for efficient analysis. As a rule, researchers utilize statistical analysis software tools or spreadsheets to manage data systematically, ensuring clarity and accessibility. Besides, proper organization includes labeling variables, formatting data consistently, and documenting any transformations or cleaning statistical procedures undertaken.
Effective data management also facilitates coherent analysis, empowering scientists to get meaningful insights and draw valid conclusions. By using suitable data collection approaches and organizing data systematically, researchers can unlock the full potential of statistical analysis, advancing knowledge and driving proof-based replies.
Performing Descriptive Statistical Analysis
Performing descriptive statistics is essential in knowing and summarizing data sets for statistics in research. Usually, it involves exploring the crucial characteristics of the data to gain insights into its normal allotting and changeability.
The basics of descriptive statistics encompass measures of central tendency, dispersion, and graphical representations.
- Measures of main bias , such as mean, median, and mode, summarize a dataset’s typical or main value.
- Dispersion repeated measures , including range, variance, and standard deviation, quantify the spread or variability of the data points.
- Graphical representations , such as histograms, box plots, and scatter plots, offer visual insights into the distribution and patterns within the data based on statistical observations.
Explaining descriptive statistical analysis results involves understanding and presenting the findings effectively. Accordingly, researchers should show understandable explanations of the descriptive statistics in the research paper calculated, highlighting key insights and trends within the data. Indeed, visual representations can enhance understanding by illustrating the distribution and relationships in the data. Hence, it’s essential to consider the context of the analysis and the questions when interpreting the results, ensuring that the conclusions drawn are suggestive and relevant.
Overall, performing descriptive statistical data analysis enables researchers to summarize and derive the crucial characteristics after collecting data. It is vital to provide a foundation for further research study and interpretation. By mastering the basics of different types of statistical analysis and correctly explaining the results, experimentals can uncover valuable insights and communicate their findings clearly and precisely.
If you struggle on this step, ask for help from our writers. They can’t write an essay or paper for you but are eager to assist you with each step.
Performing Inferential Statistics Analysis
When students perform statistical analysis, it involves making statistical inference and drawing conclusions about a population based on sample data. For this reason, the inferential statistical tool in research revolves around hypothesis testing, confidence intervals, and significance levels.
On the one side, hypothesis testing allows researchers to assess the validity of assumptions underlying entire population parameters by comparing one sample data to theoretical expectations. On the other side, sample data, null hypothesis, and confidence intervals provide a range of normal and extreme values within which the proper population parameter will likely fall. Lastly, significance levels indicate the probability of obtaining the observed results by chance alone, helping researchers determine the reliability of their findings.
Choosing the proper approach is crucial for conducting meaningful inferential statistics analysis. Accordingly, researchers must select appropriate parametric tests based on the research design, collect data type, null hypothesis, and hypothesis being tested. For example, standard parametric tests and non parametric tests include:
- T-tests: a parametric statistical test used to determine if there is a significant difference between the means of two groups. It is commonly used when the sample size is small, and the population standard deviation is unknown.
- Z test: similar to the t-test, the z-test is a parametric test used to compare means, but it is typically employed when the sample size is large and/or the population standard deviation is known.
- ANOVA: this parametric statistical test compares the means of three or more groups simultaneously. It assesses whether there are any statistically significant differences between the means of the groups.
- Regression: a statistical method used to examine the relationship between one dependent variable (often denoted as Y) and one or more independent variables (often denoted as X) within one case study analysis . Thus, it helps in understanding how the value of the dependent variable changes when one or more independent variables are varied. Here, case study analysis refers to applying regression analysis in specific scenarios or case studies to explore relationships between quantitative variables.
Importantly to note, interpreting results from inferential studies requires a nuanced understanding of statistical concepts and diligent consideration of the context. Here, investigators should assess the strength of evidence supporting their conclusions, considering factors such as effect size, statistical power, and potential biases. Besides, communicating inferential statistics results involves presenting findings and standard deviation to highlight the implications for the research question or troublesome under investigation.
Writing the Statistical Research Paper
Writing a research paper involves integrating and presenting your findings coherently. You need to know the answers to the questions: “What is statistical analysis?” and “How do you do a statistical analysis?”. As a rule, the typical structure includes several essential sections:
- Introduction : This section provides backdrop information on the research theme, states the research questions or null hypothesis, patterns, and trends, and outlines the study’s objectives and statistical attention.
- Methodology : Here, researchers detail the methods and procedures for analyzing and collecting data. This section should be thorough enough for other researchers to replicate the study.
- Results : This section presents the study’s findings, often through descriptive and inferential statistical data analysis. It’s essential to present results objectively and accurately, using appropriate statistical study measures and techniques.
- Discussion : In this segment, investigators interpret statistics and the results, discuss their implications, and compare them to existing literature. It’s an opportunity to critically evaluate the findings and address any limitations or potential biases.
- Conclusion : The conclusion summarizes the study’s key findings, discusses their significance, and suggests avenues for future research.
When you present or write a statistical report in each section, it’s crucial to clearly and concisely explain the methods, results, and research design. Therefore, students usually need to test it in the sample group. In the methodology section, describe the statistical techniques used and justify their appropriateness for the research question. Otherwise, use descriptive statistics to summarize data and inferential statistics to test hypotheses or explore relationships between variables.
Whereas, graphics and tables are potent statistical instruments for presenting data effectively. Choose the most appropriate format for your data, whether it’s a bar graph, scatter plot, or table of descriptive statistics for research.
As a result, writing your research essay must involve such steps:
- Arranging your decisions analytically;
- Integrating statistical analysis throughout;
- Using visuals and tables to enhance clarity and understanding.
Common Mistakes in Statistical Analysis
Common mistakes in statistical analysis can undermine the validity and reliability of research findings. Here are some key pitfalls to avoid:
- Confusing terms like ‘mean’ and ‘median’ or misinterpreting p value and confidence intervals can lead to incorrect conclusions.
- Selecting the wrong test for the research question or ignoring test assumptions can compromise the accuracy of the results.
- Ignoring missing data and outliers or failing to preprocess data properly can introduce bias and skew results.
- Focusing solely on statistical significance without considering practical significance or engaging in p-hacking practices can lead to misleading conclusions.
- Failing to share facts or selectively report results can hinder research reproducibility and transparency.
- Both small and large sample sizes can impact the reliability and generalizability of findings.
- Repeatedly testing hypotheses on the same data set or creating overly complicated models can result in spurious decisions.
- Failing to interpret statistical results within the broader context or generalize findings appropriately can limit the practical relevance of research.
- Misrepresenting graphics or neglecting to label and interval scale graphs correctly can distort the statistical analysis of data.
- Managing redundant analyses or ignoring existing knowledge in the field can hinder the promotion of research.
Avoiding common mistakes in statistical analysis requires diligence and attention to detail. Consequently, researchers should prioritize understanding statistical concepts systematically and using appropriate methods for exploratory data analysis. Thus, it’s essential to double-check calculations, verify assumptions, and seek guidance from statistical analysts if needed.
Furthermore, maintaining transparency and reproducibility in research practices is leading. It includes sharing data, code, and methodology details to facilitate equivalent surveys and replication of findings.
Continuous data learning and staying updated on best practices in statistical analysis are also vital for avoiding pitfalls and improving the quality of research. By addressing these common mistakes and adopting robust practices, researchers can ensure the morality and reliability of their findings, contributing to advancing knowledge in their respective fields.
Ethical Considerations
Ethical considerations in statistical analysis encompass safeguarding data privacy and integrity. That being said, researchers must uphold ethical practices in handling data, ensuring confidentiality, and respecting participants’ rights. Indeed, transparent reporting of results is vital, as is disclosing potential conflicts of passion and holding to moral guidelines data dedicated to relevant institutions and controlling bodies. By prioritizing ethical principles, researchers can maintain trust and integrity in their work, fostering a culture of responsible data analysis in research and decision-making.
Concluding the Research Paper
Concluding a research paper involves summarizing key findings and suggesting future research directions. Here, reiterating the paper’s main points and highlighting the significance of the results is essential. Statistical analysts can also discuss limitations and areas for further investigation, providing context for future studies. By showing insightful outcomes and figuring out avenues for future research, scientists can contribute to the ongoing discourse in their field and inspire further inquiry and exploration.
Examples of Good and Poor Statistical Analysis in Research Paper
Good statistical analysis examples in research:
- A study on the effectiveness of a new drug uses appropriate parametric tests, presents results clearly with confidence intervals, and discusses both statistical and practical significance.
- A survey-based research project employs stratified random sampling, ensuring a representative sample, and utilizes advanced regression analysis to explore complex relationships between variables.
- An experiment investigating the impact of a teaching method on student performance controls for potential confounding variables and conducts power statistical analysis basics to determine sample size, ensuring adequate statistical power.
Examples of poor stat analysis in research:
- A study fails to report key details about information collection and statistical methods, making it impossible to evaluate the validity of the findings.
- A research paper relies solely on p value to conclude without considering effect sizes or practical significance, leading to misleading interpretations.
- An analysis uses an inappropriate statistical test for the research question, resulting in flawed conclusions and misinterpretation of the data.
Here are two good examples.
Example 1: The Effect of Regular Exercise on Anxiety Levels among College Students
Introduction: In recent years, mental health issues among college students have become a growing concern. Anxiety, in particular, is prevalent among this demographic. This study aims to investigate the potential impact of regular exercise on anxiety levels among college students. Understanding this relationship could inform interventions aimed at improving mental well-being in this population.
Methodology: Participants (N = 100) were recruited from a local university and randomly assigned to either an exercise or control group. The exercise group engaged in a supervised 30-minute aerobic exercise session three times a week for eight weeks, while the control group maintained regular activities. Anxiety levels were assessed using the State-Trait Anxiety Inventory (STAI) before and after the intervention period.
Results: The results revealed a significant decrease in anxiety levels among participants in the exercise group compared to the control group (t(98) = -2.45, p < 0.05). Specifically, the mean anxiety score decreased from 45.2 (SD = 7.8) to 38.6 (SD = 6.4) in the exercise group, while it remained relatively stable in the control group (mean = 44.5, SD = 8.2).
Discussion: These findings suggest that regular aerobic exercise may have a beneficial effect on reducing anxiety levels among college students. Engaging in physical activity could serve as a potential non-pharmacological intervention for managing anxiety symptoms in this population. Further research is warranted to explore this relationship’s underlying mechanisms and determine optimal exercise duration and intensity for maximum mental health benefits.
Example 2: The Relationship between Service Quality, Customer Satisfaction, and Loyalty in Retail Settings
Introduction: Maintaining high levels of customer satisfaction and loyalty is essential for the success of retail businesses. This study investigates the relationship between service quality, customer satisfaction, and loyalty in a local retail chain context. Understanding these dynamics can help businesses identify areas for improvement and develop strategies to enhance customer retention.
Methodology: A survey was conducted among the retail chain’s customers (N = 300) to assess their perceptions of service quality, satisfaction with their shopping experience, and intention to repurchase from the store. Service quality was measured using the SERVQUAL scale, while customer satisfaction and loyalty were assessed using Likert-type scales.
Results: The results indicated a strong positive correlation between service quality, customer satisfaction, and loyalty (r = 0.75, p < 0.001). Furthermore, regression analysis revealed that service quality significantly predicted both customer satisfaction (β = 0.60, p < 0.001) and loyalty (β = 0.45, p < 0.001). Additionally, customer satisfaction emerged as a significant predictor of loyalty (β = 0.50, p < 0.001), indicating its mediating role in the relationship between service quality and loyalty.
Discussion: These findings underscore the importance of high-quality service in enhancing customer satisfaction and fostering loyalty in retail settings. Businesses should prioritize investments in service training, infrastructure, and customer relationship management to ensure positive shopping experiences and promote repeat patronage. Future research could explore additional factors influencing customer loyalty and examine the effectiveness of specific loyalty programs and incentives in driving repeat business.
Key Insights: Navigating Statistical Analysis
To sum up, mastering a statistical analysis system is essential for researchers to derive meaningful insights from data. Understanding statistical concepts, choosing appropriate methods, and adhering to ethical guidelines are paramount.
Additionally, transparent reporting, rigorous methodology, and careful interpretation ensure the integrity and reliability of research findings. By avoiding common pitfalls and embracing best practices, researchers can contribute to advancing knowledge and making informed decisions across various fields.
Ultimately, statistical analysis is a powerful tool for unlocking the mysteries hidden within data, guiding us toward more profound understanding and innovation.
Readers also enjoyed

WHY WAIT? PLACE AN ORDER RIGHT NOW!
Just fill out the form, press the button, and have no worries!
We use cookies to give you the best experience possible. By continuing we’ll assume you board with our cookie policy.
Research Paper Guide
Research Paper Outline

Guide to Creating Effective Research Paper Outline
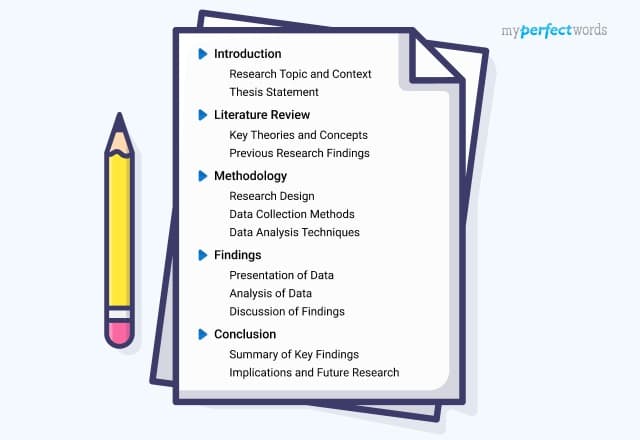
People also read
Research Paper Writing - A Step by Step Guide
Research Paper Examples - Free Sample Papers for Different Formats!
Interesting Research Paper Topics for 2024
Research Proposal Writing - A Step-by-Step Guide
How to Start a Research Paper - 7 Easy Steps
How to Write an Abstract for a Research Paper - A Step by Step Guide
Writing a Literature Review For a Research Paper - A Comprehensive Guide
Qualitative Research - Methods, Types, and Examples
8 Types of Qualitative Research - Overview & Examples
Qualitative vs Quantitative Research - Learning the Basics
200+ Engaging Psychology Research Paper Topics for Students in 2024
Learn How to Write a Hypothesis in a Research Paper: Examples and Tips!
20+ Types of Research With Examples - A Detailed Guide
Understanding Quantitative Research - Types & Data Collection Techniques
230+ Sociology Research Topics & Ideas for Students
How to Cite a Research Paper - A Complete Guide
Excellent History Research Paper Topics- 300+ Ideas
A Guide on Writing the Method Section of a Research Paper - Examples & Tips
How To Write an Introduction Paragraph For a Research Paper: Learn with Examples
Crafting a Winning Research Paper Title: A Complete Guide
Writing a Research Paper Conclusion - Step-by-Step Guide
Writing a Thesis For a Research Paper - A Comprehensive Guide
How To Write A Discussion For A Research Paper | Examples & Tips
How To Write The Results Section of A Research Paper | Steps & Examples
Writing a Problem Statement for a Research Paper - A Comprehensive Guide
Finding Sources For a Research Paper: A Complete Guide
A Guide on How to Edit a Research Paper
200+ Ethical Research Paper Topics to Begin With (2024)
300+ Controversial Research Paper Topics & Ideas - 2024 Edition
150+ Argumentative Research Paper Topics For You - 2024
How to Write a Research Methodology for a Research Paper
Have you ever felt overwhelmed by research, not knowing how to start or structure your thoughts effectively?
You're not alone in facing this problem. The challenge of disorganized research is something many students deal with. But don't worry!
In this blog, we'll not only tell you how to create your research paper outline, but we’ve included some downloadable templates as well.
We'll provide a practical step-by-step solution for organizing your research material so you end with a well-structured research paper. By the end of the blog, you'll understand why outlines matter and how to create compelling research papers.
So, keep reading to learn more!

- 1. What is a Research Paper Outline?
- 2. Different Research Paper Outline Formats
- 3. Steps to Create a Research Paper Outline
- 4. Research Paper Outline Example
What is a Research Paper Outline?
A research paper outline serves as a systematic framework for your paper. It's a structured strategy that assists in the organization of your thoughts and ideas before the writing process begins.
The outline structures out the main sections, subtopics, and supporting details in your research paper. In essence, it offers a well-structured and coherent roadmap for the entirety of your paper, maintaining academic rigor and clarity.
Different Research Paper Outline Formats
When it comes to creating a research paper outline, you've got options. Let's explore a few different formats that you can choose from:
Numeric Outline
A numeric outline is a structured organizational format for planning a research paper.
It uses a numerical system to represent the hierarchy of ideas, with each main section or point numbered and subpoints or details indicated by decimal numbers. Numeric outlines are useful for presenting information in a clear and logical sequence.
Here’s a sample research paper outline template for this format:
Alphanumeric Outline
An alphanumeric outline is a hierarchical structure used to outline a research paper, combining numbers and letters to signify the different levels of information.
Main sections are designated with capital letters (A, B, C), which include major points, while subpoints are indicated by numbers and lowercase letters (1, 2, a, b). Alphanumeric outlines help writers organize complex topics and subtopics effectively.
Here’s a sample sample research paper outline for this format:
Full Sentence Outline
A full-sentence outline is a method of planning a research paper in which each point in the outline is presented as a complete sentence or phrase.
It provides a detailed overview of the content and structure of the paper. Full-sentence outlines are particularly helpful for writers who prefer thorough planning and want to capture the essence of each section or point.
Here’s a research paper outline format for full sentences:
Steps to Create a Research Paper Outline
Creating a research paper outline doesn't have to be complicated. Follow these simple steps to get started:
Step 1: Choose Your Research Topic
Begin by selecting a research topic that is both interesting to you and relevant to your assignment or academic objectives. Your chosen topic will serve as the foundation for your entire research paper.
Step 2: Identify Your Main Sections
Determine the main sections or chapters your research paper will include. These are the broad thematic areas that will structure your paper, and they provide a high-level overview of the topics you plan to cover. Here are the main sections a typical research paper involves:
- Title Page: This is the first page and includes the paper's title, author's name, institutional affiliation, and often the running head.
- Abstract : A concise summary of the paper, usually around 150-250 words, providing an overview of the research, its key findings, and implications.
- Introduction: Sets the stage for your research, offering background information and a thesis statement , which is a central argument or hypothesis.
- Literature Review : A comprehensive analysis of existing research and literature on your topic, demonstrating your understanding of the subject.
- Methodology: Explain the research methods, data collection techniques, and analytical tools used in your study.
- Findings: Presents the research results in a structured manner, often including data, tables, or charts.
- Discussion: Interpretation of the findings and their implications, offering insights into the research's significance.
- Conclusion: Summarizes the main points, reiterates the thesis, and discusses potential future research directions.
- References: A list of all sources cited in your paper, following a specific citation style (e.g., APA, MLA).
Step 3: Break It Down into Subtopics
Under each main section, further divide your content into smaller subtopics. Subtopics are like the building blocks of your paper; they represent the key points or ideas you intend to explore within each main section.
Step 4: Add Supporting Details
For each subtopic, include supporting details, facts, examples, or arguments that bolster your point. These supporting details form the substance of your paper and provide evidence for your claims or arguments.
Step 5: Organize Your Points
Organize your main sections, subtopics, and supporting details in a logical order that flows smoothly from one point to the next. This step ensures that your research paper maintains coherence and readability.
Step 6: Use Numbers or Letters
To enhance clarity within your outline, use numbering, a lettering system, or Roman numerals. Use numerical sequencing for main sections (e.g., "1.," "2.," "3.") and a combination of numbers and letters for subtopics (e.g., "1.1," "1.2," "2.1").
Step 7: Stay Flexible
Recognize that your outline is not set in stone. As you conduct research and begin writing, your ideas may evolve, and you may discover the need to adjust your outline accordingly. Embrace this flexibility to adapt to new insights and information.
By following these steps, you'll create a well-structured research paper outline that serves as a roadmap for your writing journey. It keeps your research organized and makes writing easier, resulting in a more effective paper.
Research Paper Outline Example
A research paper outline could be created in several different ways. Here is a sample research paper outline for a quick review:
Here are some more examples for different formats and subjects:
APA Research Paper Outline PDF
College Research Paper Outline
Argumentative Research Paper Outline
Sample Research Paper Outline
History Research Paper Outline
Research Paper Outline MLA
Research Paper Outline with Annotated Bibliography
Need to consult more examples? Have a look at these top-quality research paper examples and get inspiration!
In conclusion, with the help of these example templates and our step-by-step guide on creating an outline, you're now well-prepared to create an effective one.
If you're in a hurry and want to skip the outlining process, our essay writing service is here to help!
You can get our team of expert essay writers to assist you at any stage of your research or to deliver a well-formatted, accurate research paper.
So, just say, write my research paper , and we’ll deliver an original, top-quality paper to you!

Write Essay Within 60 Seconds!

Nova Allison is a Digital Content Strategist with over eight years of experience. Nova has also worked as a technical and scientific writer. She is majorly involved in developing and reviewing online content plans that engage and resonate with audiences. Nova has a passion for writing that engages and informs her readers.

Paper Due? Why Suffer? That’s our Job!
Keep reading
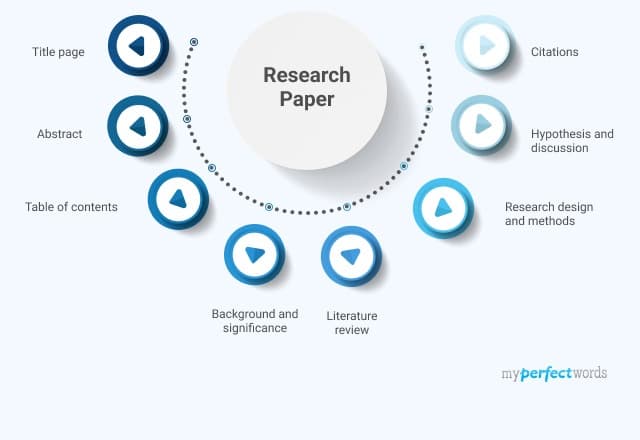

An official website of the United States government
The .gov means it’s official. Federal government websites often end in .gov or .mil. Before sharing sensitive information, make sure you’re on a federal government site.
The site is secure. The https:// ensures that you are connecting to the official website and that any information you provide is encrypted and transmitted securely.
- Publications
- Account settings
Preview improvements coming to the PMC website in October 2024. Learn More or Try it out now .
- Advanced Search
- Journal List
- Indian J Anaesth
- v.60(9); 2016 Sep
Basic statistical tools in research and data analysis
Zulfiqar ali.
Department of Anaesthesiology, Division of Neuroanaesthesiology, Sheri Kashmir Institute of Medical Sciences, Soura, Srinagar, Jammu and Kashmir, India
S Bala Bhaskar
1 Department of Anaesthesiology and Critical Care, Vijayanagar Institute of Medical Sciences, Bellary, Karnataka, India
Statistical methods involved in carrying out a study include planning, designing, collecting data, analysing, drawing meaningful interpretation and reporting of the research findings. The statistical analysis gives meaning to the meaningless numbers, thereby breathing life into a lifeless data. The results and inferences are precise only if proper statistical tests are used. This article will try to acquaint the reader with the basic research tools that are utilised while conducting various studies. The article covers a brief outline of the variables, an understanding of quantitative and qualitative variables and the measures of central tendency. An idea of the sample size estimation, power analysis and the statistical errors is given. Finally, there is a summary of parametric and non-parametric tests used for data analysis.
INTRODUCTION
Statistics is a branch of science that deals with the collection, organisation, analysis of data and drawing of inferences from the samples to the whole population.[ 1 ] This requires a proper design of the study, an appropriate selection of the study sample and choice of a suitable statistical test. An adequate knowledge of statistics is necessary for proper designing of an epidemiological study or a clinical trial. Improper statistical methods may result in erroneous conclusions which may lead to unethical practice.[ 2 ]
Variable is a characteristic that varies from one individual member of population to another individual.[ 3 ] Variables such as height and weight are measured by some type of scale, convey quantitative information and are called as quantitative variables. Sex and eye colour give qualitative information and are called as qualitative variables[ 3 ] [ Figure 1 ].

Classification of variables
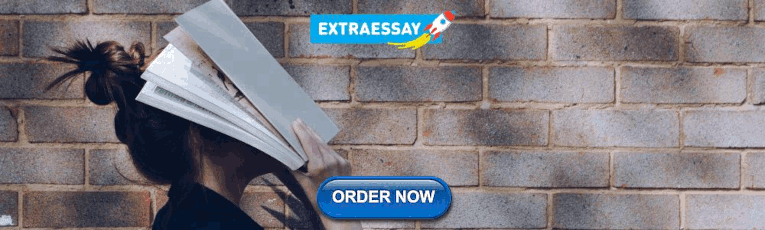
Quantitative variables
Quantitative or numerical data are subdivided into discrete and continuous measurements. Discrete numerical data are recorded as a whole number such as 0, 1, 2, 3,… (integer), whereas continuous data can assume any value. Observations that can be counted constitute the discrete data and observations that can be measured constitute the continuous data. Examples of discrete data are number of episodes of respiratory arrests or the number of re-intubations in an intensive care unit. Similarly, examples of continuous data are the serial serum glucose levels, partial pressure of oxygen in arterial blood and the oesophageal temperature.
A hierarchical scale of increasing precision can be used for observing and recording the data which is based on categorical, ordinal, interval and ratio scales [ Figure 1 ].
Categorical or nominal variables are unordered. The data are merely classified into categories and cannot be arranged in any particular order. If only two categories exist (as in gender male and female), it is called as a dichotomous (or binary) data. The various causes of re-intubation in an intensive care unit due to upper airway obstruction, impaired clearance of secretions, hypoxemia, hypercapnia, pulmonary oedema and neurological impairment are examples of categorical variables.
Ordinal variables have a clear ordering between the variables. However, the ordered data may not have equal intervals. Examples are the American Society of Anesthesiologists status or Richmond agitation-sedation scale.
Interval variables are similar to an ordinal variable, except that the intervals between the values of the interval variable are equally spaced. A good example of an interval scale is the Fahrenheit degree scale used to measure temperature. With the Fahrenheit scale, the difference between 70° and 75° is equal to the difference between 80° and 85°: The units of measurement are equal throughout the full range of the scale.
Ratio scales are similar to interval scales, in that equal differences between scale values have equal quantitative meaning. However, ratio scales also have a true zero point, which gives them an additional property. For example, the system of centimetres is an example of a ratio scale. There is a true zero point and the value of 0 cm means a complete absence of length. The thyromental distance of 6 cm in an adult may be twice that of a child in whom it may be 3 cm.
STATISTICS: DESCRIPTIVE AND INFERENTIAL STATISTICS
Descriptive statistics[ 4 ] try to describe the relationship between variables in a sample or population. Descriptive statistics provide a summary of data in the form of mean, median and mode. Inferential statistics[ 4 ] use a random sample of data taken from a population to describe and make inferences about the whole population. It is valuable when it is not possible to examine each member of an entire population. The examples if descriptive and inferential statistics are illustrated in Table 1 .
Example of descriptive and inferential statistics

Descriptive statistics
The extent to which the observations cluster around a central location is described by the central tendency and the spread towards the extremes is described by the degree of dispersion.
Measures of central tendency
The measures of central tendency are mean, median and mode.[ 6 ] Mean (or the arithmetic average) is the sum of all the scores divided by the number of scores. Mean may be influenced profoundly by the extreme variables. For example, the average stay of organophosphorus poisoning patients in ICU may be influenced by a single patient who stays in ICU for around 5 months because of septicaemia. The extreme values are called outliers. The formula for the mean is

where x = each observation and n = number of observations. Median[ 6 ] is defined as the middle of a distribution in a ranked data (with half of the variables in the sample above and half below the median value) while mode is the most frequently occurring variable in a distribution. Range defines the spread, or variability, of a sample.[ 7 ] It is described by the minimum and maximum values of the variables. If we rank the data and after ranking, group the observations into percentiles, we can get better information of the pattern of spread of the variables. In percentiles, we rank the observations into 100 equal parts. We can then describe 25%, 50%, 75% or any other percentile amount. The median is the 50 th percentile. The interquartile range will be the observations in the middle 50% of the observations about the median (25 th -75 th percentile). Variance[ 7 ] is a measure of how spread out is the distribution. It gives an indication of how close an individual observation clusters about the mean value. The variance of a population is defined by the following formula:

where σ 2 is the population variance, X is the population mean, X i is the i th element from the population and N is the number of elements in the population. The variance of a sample is defined by slightly different formula:

where s 2 is the sample variance, x is the sample mean, x i is the i th element from the sample and n is the number of elements in the sample. The formula for the variance of a population has the value ‘ n ’ as the denominator. The expression ‘ n −1’ is known as the degrees of freedom and is one less than the number of parameters. Each observation is free to vary, except the last one which must be a defined value. The variance is measured in squared units. To make the interpretation of the data simple and to retain the basic unit of observation, the square root of variance is used. The square root of the variance is the standard deviation (SD).[ 8 ] The SD of a population is defined by the following formula:

where σ is the population SD, X is the population mean, X i is the i th element from the population and N is the number of elements in the population. The SD of a sample is defined by slightly different formula:

where s is the sample SD, x is the sample mean, x i is the i th element from the sample and n is the number of elements in the sample. An example for calculation of variation and SD is illustrated in Table 2 .
Example of mean, variance, standard deviation

Normal distribution or Gaussian distribution
Most of the biological variables usually cluster around a central value, with symmetrical positive and negative deviations about this point.[ 1 ] The standard normal distribution curve is a symmetrical bell-shaped. In a normal distribution curve, about 68% of the scores are within 1 SD of the mean. Around 95% of the scores are within 2 SDs of the mean and 99% within 3 SDs of the mean [ Figure 2 ].

Normal distribution curve
Skewed distribution
It is a distribution with an asymmetry of the variables about its mean. In a negatively skewed distribution [ Figure 3 ], the mass of the distribution is concentrated on the right of Figure 1 . In a positively skewed distribution [ Figure 3 ], the mass of the distribution is concentrated on the left of the figure leading to a longer right tail.

Curves showing negatively skewed and positively skewed distribution
Inferential statistics
In inferential statistics, data are analysed from a sample to make inferences in the larger collection of the population. The purpose is to answer or test the hypotheses. A hypothesis (plural hypotheses) is a proposed explanation for a phenomenon. Hypothesis tests are thus procedures for making rational decisions about the reality of observed effects.
Probability is the measure of the likelihood that an event will occur. Probability is quantified as a number between 0 and 1 (where 0 indicates impossibility and 1 indicates certainty).
In inferential statistics, the term ‘null hypothesis’ ( H 0 ‘ H-naught ,’ ‘ H-null ’) denotes that there is no relationship (difference) between the population variables in question.[ 9 ]
Alternative hypothesis ( H 1 and H a ) denotes that a statement between the variables is expected to be true.[ 9 ]
The P value (or the calculated probability) is the probability of the event occurring by chance if the null hypothesis is true. The P value is a numerical between 0 and 1 and is interpreted by researchers in deciding whether to reject or retain the null hypothesis [ Table 3 ].
P values with interpretation

If P value is less than the arbitrarily chosen value (known as α or the significance level), the null hypothesis (H0) is rejected [ Table 4 ]. However, if null hypotheses (H0) is incorrectly rejected, this is known as a Type I error.[ 11 ] Further details regarding alpha error, beta error and sample size calculation and factors influencing them are dealt with in another section of this issue by Das S et al .[ 12 ]
Illustration for null hypothesis

PARAMETRIC AND NON-PARAMETRIC TESTS
Numerical data (quantitative variables) that are normally distributed are analysed with parametric tests.[ 13 ]
Two most basic prerequisites for parametric statistical analysis are:
- The assumption of normality which specifies that the means of the sample group are normally distributed
- The assumption of equal variance which specifies that the variances of the samples and of their corresponding population are equal.
However, if the distribution of the sample is skewed towards one side or the distribution is unknown due to the small sample size, non-parametric[ 14 ] statistical techniques are used. Non-parametric tests are used to analyse ordinal and categorical data.
Parametric tests
The parametric tests assume that the data are on a quantitative (numerical) scale, with a normal distribution of the underlying population. The samples have the same variance (homogeneity of variances). The samples are randomly drawn from the population, and the observations within a group are independent of each other. The commonly used parametric tests are the Student's t -test, analysis of variance (ANOVA) and repeated measures ANOVA.
Student's t -test
Student's t -test is used to test the null hypothesis that there is no difference between the means of the two groups. It is used in three circumstances:

where X = sample mean, u = population mean and SE = standard error of mean

where X 1 − X 2 is the difference between the means of the two groups and SE denotes the standard error of the difference.
- To test if the population means estimated by two dependent samples differ significantly (the paired t -test). A usual setting for paired t -test is when measurements are made on the same subjects before and after a treatment.
The formula for paired t -test is:

where d is the mean difference and SE denotes the standard error of this difference.
The group variances can be compared using the F -test. The F -test is the ratio of variances (var l/var 2). If F differs significantly from 1.0, then it is concluded that the group variances differ significantly.
Analysis of variance
The Student's t -test cannot be used for comparison of three or more groups. The purpose of ANOVA is to test if there is any significant difference between the means of two or more groups.
In ANOVA, we study two variances – (a) between-group variability and (b) within-group variability. The within-group variability (error variance) is the variation that cannot be accounted for in the study design. It is based on random differences present in our samples.
However, the between-group (or effect variance) is the result of our treatment. These two estimates of variances are compared using the F-test.
A simplified formula for the F statistic is:

where MS b is the mean squares between the groups and MS w is the mean squares within groups.
Repeated measures analysis of variance
As with ANOVA, repeated measures ANOVA analyses the equality of means of three or more groups. However, a repeated measure ANOVA is used when all variables of a sample are measured under different conditions or at different points in time.
As the variables are measured from a sample at different points of time, the measurement of the dependent variable is repeated. Using a standard ANOVA in this case is not appropriate because it fails to model the correlation between the repeated measures: The data violate the ANOVA assumption of independence. Hence, in the measurement of repeated dependent variables, repeated measures ANOVA should be used.
Non-parametric tests
When the assumptions of normality are not met, and the sample means are not normally, distributed parametric tests can lead to erroneous results. Non-parametric tests (distribution-free test) are used in such situation as they do not require the normality assumption.[ 15 ] Non-parametric tests may fail to detect a significant difference when compared with a parametric test. That is, they usually have less power.
As is done for the parametric tests, the test statistic is compared with known values for the sampling distribution of that statistic and the null hypothesis is accepted or rejected. The types of non-parametric analysis techniques and the corresponding parametric analysis techniques are delineated in Table 5 .
Analogue of parametric and non-parametric tests

Median test for one sample: The sign test and Wilcoxon's signed rank test
The sign test and Wilcoxon's signed rank test are used for median tests of one sample. These tests examine whether one instance of sample data is greater or smaller than the median reference value.
This test examines the hypothesis about the median θ0 of a population. It tests the null hypothesis H0 = θ0. When the observed value (Xi) is greater than the reference value (θ0), it is marked as+. If the observed value is smaller than the reference value, it is marked as − sign. If the observed value is equal to the reference value (θ0), it is eliminated from the sample.
If the null hypothesis is true, there will be an equal number of + signs and − signs.
The sign test ignores the actual values of the data and only uses + or − signs. Therefore, it is useful when it is difficult to measure the values.
Wilcoxon's signed rank test
There is a major limitation of sign test as we lose the quantitative information of the given data and merely use the + or – signs. Wilcoxon's signed rank test not only examines the observed values in comparison with θ0 but also takes into consideration the relative sizes, adding more statistical power to the test. As in the sign test, if there is an observed value that is equal to the reference value θ0, this observed value is eliminated from the sample.
Wilcoxon's rank sum test ranks all data points in order, calculates the rank sum of each sample and compares the difference in the rank sums.
Mann-Whitney test
It is used to test the null hypothesis that two samples have the same median or, alternatively, whether observations in one sample tend to be larger than observations in the other.
Mann–Whitney test compares all data (xi) belonging to the X group and all data (yi) belonging to the Y group and calculates the probability of xi being greater than yi: P (xi > yi). The null hypothesis states that P (xi > yi) = P (xi < yi) =1/2 while the alternative hypothesis states that P (xi > yi) ≠1/2.
Kolmogorov-Smirnov test
The two-sample Kolmogorov-Smirnov (KS) test was designed as a generic method to test whether two random samples are drawn from the same distribution. The null hypothesis of the KS test is that both distributions are identical. The statistic of the KS test is a distance between the two empirical distributions, computed as the maximum absolute difference between their cumulative curves.
Kruskal-Wallis test
The Kruskal–Wallis test is a non-parametric test to analyse the variance.[ 14 ] It analyses if there is any difference in the median values of three or more independent samples. The data values are ranked in an increasing order, and the rank sums calculated followed by calculation of the test statistic.
Jonckheere test
In contrast to Kruskal–Wallis test, in Jonckheere test, there is an a priori ordering that gives it a more statistical power than the Kruskal–Wallis test.[ 14 ]
Friedman test
The Friedman test is a non-parametric test for testing the difference between several related samples. The Friedman test is an alternative for repeated measures ANOVAs which is used when the same parameter has been measured under different conditions on the same subjects.[ 13 ]
Tests to analyse the categorical data
Chi-square test, Fischer's exact test and McNemar's test are used to analyse the categorical or nominal variables. The Chi-square test compares the frequencies and tests whether the observed data differ significantly from that of the expected data if there were no differences between groups (i.e., the null hypothesis). It is calculated by the sum of the squared difference between observed ( O ) and the expected ( E ) data (or the deviation, d ) divided by the expected data by the following formula:

A Yates correction factor is used when the sample size is small. Fischer's exact test is used to determine if there are non-random associations between two categorical variables. It does not assume random sampling, and instead of referring a calculated statistic to a sampling distribution, it calculates an exact probability. McNemar's test is used for paired nominal data. It is applied to 2 × 2 table with paired-dependent samples. It is used to determine whether the row and column frequencies are equal (that is, whether there is ‘marginal homogeneity’). The null hypothesis is that the paired proportions are equal. The Mantel-Haenszel Chi-square test is a multivariate test as it analyses multiple grouping variables. It stratifies according to the nominated confounding variables and identifies any that affects the primary outcome variable. If the outcome variable is dichotomous, then logistic regression is used.
SOFTWARES AVAILABLE FOR STATISTICS, SAMPLE SIZE CALCULATION AND POWER ANALYSIS
Numerous statistical software systems are available currently. The commonly used software systems are Statistical Package for the Social Sciences (SPSS – manufactured by IBM corporation), Statistical Analysis System ((SAS – developed by SAS Institute North Carolina, United States of America), R (designed by Ross Ihaka and Robert Gentleman from R core team), Minitab (developed by Minitab Inc), Stata (developed by StataCorp) and the MS Excel (developed by Microsoft).
There are a number of web resources which are related to statistical power analyses. A few are:
- StatPages.net – provides links to a number of online power calculators
- G-Power – provides a downloadable power analysis program that runs under DOS
- Power analysis for ANOVA designs an interactive site that calculates power or sample size needed to attain a given power for one effect in a factorial ANOVA design
- SPSS makes a program called SamplePower. It gives an output of a complete report on the computer screen which can be cut and paste into another document.
It is important that a researcher knows the concepts of the basic statistical methods used for conduct of a research study. This will help to conduct an appropriately well-designed study leading to valid and reliable results. Inappropriate use of statistical techniques may lead to faulty conclusions, inducing errors and undermining the significance of the article. Bad statistics may lead to bad research, and bad research may lead to unethical practice. Hence, an adequate knowledge of statistics and the appropriate use of statistical tests are important. An appropriate knowledge about the basic statistical methods will go a long way in improving the research designs and producing quality medical research which can be utilised for formulating the evidence-based guidelines.
Financial support and sponsorship
Conflicts of interest.
There are no conflicts of interest.
How to incorporate data and statistics in research papers
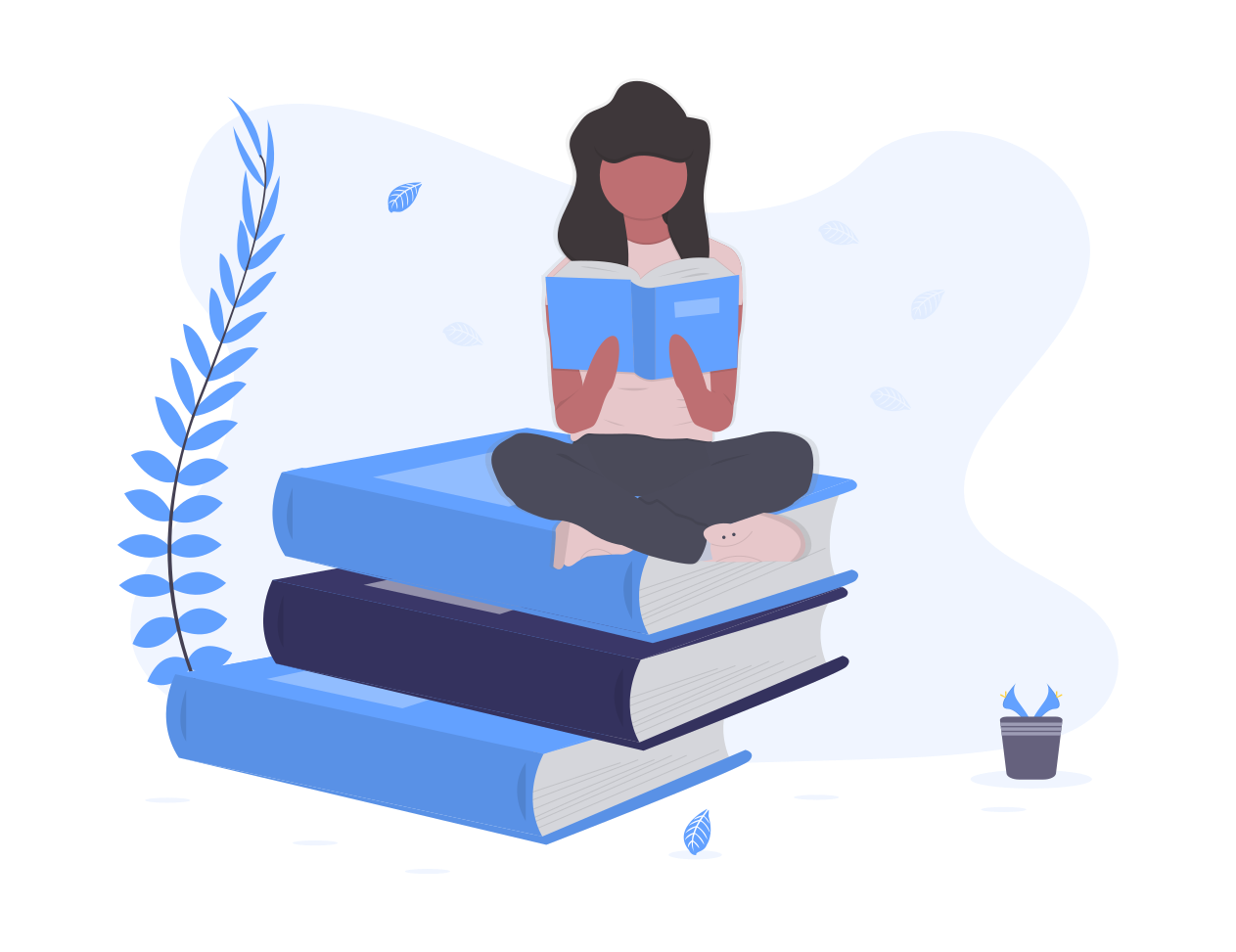
Data and statistics serve as the backbone of empirical research, allowing researchers to move beyond theoretical assumptions and draw concrete conclusions. They provide credibility to your work, as they are objective and verifiable by other researchers.
Differentiating Between Qualitative and Quantitative Research
Before incorporating data and statistics, it’s essential to understand the type of research you are conducting. Qualitative research focuses on non-numeric information, such as interviews and observations, while quantitative research relies on numerical data and statistical analysis. The latter is the primary focus of this guide.
Data Collection and Preparation
Here are the things for data collection and preparation;
Designing Your Research Methodology
To incorporate data effectively, you must design a rigorous research methodology. This involves defining your research questions, selecting data collection methods (surveys, experiments, observations, etc.), and ensuring that your data collection process aligns with your research goals.
Ensuring Data Reliability and Validity
Reliability refers to the consistency and stability of your data collection methods, while validity pertains to the accuracy and relevance of the data to your research questions. Establishing both is crucial to the integrity of your research.
Ethical Considerations in Data Collection
Adhering to ethical guidelines in data collection is essential. This includes obtaining informed consent from participants, protecting their privacy, and ensuring that your research does not harm individuals or communities.
Choosing the Right Statistical Methods
Descriptive vs. inferential statistics.
Descriptive statistics summarize and describe data, providing a snapshot of its characteristics (e.g., mean, median, standard deviation). Inferential statistics, on the other hand, allow you to draw conclusions or make predictions about a population based on a sample.
Selecting Appropriate Statistical Tests
Choosing the right statistical tests depends on your research questions and the type of data you have. Common tests include t-tests, chi-squared tests, ANOVA, regression analysis, and more. Consult with a statistician if needed.
Sample Size Determination
Determining an appropriate sample size is crucial for the reliability and generalizability of your findings. Sample size calculations should be based on statistical power, effect size, and desired level of confidence.
Data Presentation
Creating clear and informative tables and figures.
Well-constructed tables and figures are essential for conveying your data effectively. They should be clear, organized, and directly relevant to your research questions. Avoid clutter and excessive detail.
Properly Labeling and Numbering Visuals
Each table and figure should be labeled with a clear title and numbered sequentially. In the main text, refer to them by their respective numbers and provide brief explanations of their significance.
Captions and Explanations
Include detailed captions for each visual to help readers understand the data’s context and significance. In the main text, provide explanations and interpretations of the visuals.
Interpreting and Discussing Results
Analyzing statistical findings.
Interpret your statistical findings in the context of your research questions. Explain the meaning of statistical measures, such as p-values and confidence intervals, and their implications for your hypotheses.
Relating Data to Research Questions or Hypotheses
Connect your data to your research questions or hypotheses. Discuss how the findings either support or refute your initial hypotheses and provide reasons for any unexpected results.
Identifying Patterns and Trends
Look for patterns and trends within your data and discuss their significance. Are there any notable correlations, trends over time, or outliers that require explanation?
Avoiding Common Mistakes
Common statistical errors to watch out for.
Be aware of common statistical errors, such as misinterpreting p-values, overgeneralizing findings, or ignoring confounding variables. Conduct thorough data checks and seek statistical consultation if necessary.
The Importance of Peer Review and Statistical Consultation
Peer review and statistical consultation are invaluable for ensuring the accuracy and validity of your statistical analyses. Seek feedback from colleagues and experts in your field to improve the quality of your research.
Citing Sources and Data
Properly citing statistical sources.
When using statistical data from external sources, cite them appropriately. Follow citation guidelines (e.g., APA, MLA, Chicago) and provide sufficient information for readers to locate the original data.
Ethical Use of Others' Data
If using others' data, ensure you have permission or are abiding by licensing agreements. Always give credit to the original data sources to maintain academic integrity.
Things to consider when incorporating data and statistics in research papers
Incorporating data and statistics in research papers is a crucial aspect of building a strong argument and supporting your findings. Here are some key considerations to keep in mind when working with data and statistics in your research papers:
Data Quality and Source
- Ensure that the data you use is reliable and from reputable sources.
- Be transparent about the data’s origin and any potential biases or limitations in the data source.
Data Cleaning and Preprocessing
- Clean and preprocess your data to remove errors, outliers, and inconsistencies.
- Document the steps you took in data cleaning and preprocessing for transparency.
Descriptive Statistics
- Use descriptive statistics (mean, median, mode, standard deviation, etc.) to summarize key aspects of your data.
- Include appropriate visualizations like histograms, box plots, or scatter plots to illustrate the data distribution.
Hypothesis Testing and Inferential Statistics
- If your research involves hypothesis testing, select the appropriate statistical tests (e.g., t-tests, ANOVA, chi-squared) based on your research questions and data type.
- Clearly state your hypotheses and the significance level (e.g., alpha = 0.05) you are using.
- Report the results of hypothesis tests accurately, including p-values and effect sizes.
Interpretation and Discussion
- The circumstances of your study question should be used to interpret the statistical findings. Describe the ramifications of the findings and what they mean.
- Discuss the practical significance of your results, not just statistical significance.
Avoid Data Cherry-Picking
- Present all relevant data, not just the data that supports your hypothesis or argument.
- Acknowledge any contradictory or inconclusive findings.
Data Visualization
- Use appropriate data visualization techniques to make your data more understandable.
- Ensure that visualizations have clear labels, scales, and captions.
Ethics and Privacy
- Respect ethical guidelines when using data, especially if it involves human subjects or sensitive information.
- Anonymize or de-identify data when necessary to protect privacy.
Citations and References
- Properly cite the sources of your data and statistics, following a recognized citation style (e.g., APA, MLA, Chicago).
- Include a reference list or bibliography.
Reproducibility and Transparency
- Provide all necessary details to allow others to replicate your data analysis. This includes code, software used, and detailed methodology.
- Consider sharing your data (if possible) and code to facilitate reproducibility.
Limitations and Assumptions
- Clearly state the limitations of your data and statistical analyses. Every dataset has limitations, and it’s important to acknowledge them.
- Discuss any assumptions made in your statistical models.
Peer Review
- Seek peer review from colleagues or mentors with expertise in statistics and data analysis to ensure the validity of your methods and results.
Clarity and Accessibility
- Present your data and statistics in a clear and accessible manner so that readers without a strong statistical background can understand your findings.
Conciseness
- Avoid overloading your paper with excessive statistical detail. Include only what is necessary to support your research questions and findings.
Consult Experts
- If you are not confident in your statistical skills, consider consulting with a statistician or data analyst to ensure the accuracy and validity of your analyses.
Remember that the effective use of data and statistics can greatly enhance the rigor and persuasiveness of your research paper. Properly integrating them into your research process and communicating their significance is essential for producing high-quality academic work.
Incorporating data and statistics into research papers is a multifaceted process that demands meticulous planning, ethical considerations, and a strong understanding of statistical methods. When executed effectively, data and statistics enhance the credibility and persuasiveness of your research, facilitating the advancement of knowledge within your field.
- PRO Courses Guides New Tech Help Pro Expert Videos About wikiHow Pro Upgrade Sign In
- EDIT Edit this Article
- EXPLORE Tech Help Pro About Us Random Article Quizzes Request a New Article Community Dashboard This Or That Game Popular Categories Arts and Entertainment Artwork Books Movies Computers and Electronics Computers Phone Skills Technology Hacks Health Men's Health Mental Health Women's Health Relationships Dating Love Relationship Issues Hobbies and Crafts Crafts Drawing Games Education & Communication Communication Skills Personal Development Studying Personal Care and Style Fashion Hair Care Personal Hygiene Youth Personal Care School Stuff Dating All Categories Arts and Entertainment Finance and Business Home and Garden Relationship Quizzes Cars & Other Vehicles Food and Entertaining Personal Care and Style Sports and Fitness Computers and Electronics Health Pets and Animals Travel Education & Communication Hobbies and Crafts Philosophy and Religion Work World Family Life Holidays and Traditions Relationships Youth
- Browse Articles
- Learn Something New
- Quizzes Hot
- This Or That Game New
- Train Your Brain
- Explore More
- Support wikiHow
- About wikiHow
- Log in / Sign up
- Education and Communications
- Official Writing
- Report Writing
How to Write a Statistical Report
Last Updated: March 6, 2024 Fact Checked
This article was reviewed by Grace Imson, MA and by wikiHow staff writer, Jennifer Mueller, JD . Grace Imson is a math teacher with over 40 years of teaching experience. Grace is currently a math instructor at the City College of San Francisco and was previously in the Math Department at Saint Louis University. She has taught math at the elementary, middle, high school, and college levels. She has an MA in Education, specializing in Administration and Supervision from Saint Louis University. This article has been fact-checked, ensuring the accuracy of any cited facts and confirming the authority of its sources. This article has been viewed 399,880 times.
A statistical report informs readers about a particular subject or project. You can write a successful statistical report by formatting your report properly and including all the necessary information your readers need. [1] X Research source
A Beginner’s Guide to Statistical Report Writing
Use other statistical reports as a guide to format your own. Type your report in an easy-to-read font, include all the information that your reader needs, and present your results in a table or graph.
Formatting Your Report

- If you're completing your report for a class, your instructor or professor may be willing to show you some reports submitted by previous students if you ask.
- University libraries also have copies of statistical reports created by students and faculty researchers on file. Ask the research librarian to help you locate one in your field of study.
- You also may be able to find statistical reports online that were created for business or marketing research, as well as those filed for government agencies.
- Be careful following samples exactly, particularly if they were completed for research in another field. Different fields of study have their own conventions regarding how a statistical report should look and what it should contain. For example, a statistical report by a mathematician may look incredibly different than one created by a market researcher for a retail business.

- You typically want to have 1-inch margins around all sides of your report. Be careful when adding visual elements such as charts and graphs to your report, and make sure they don't bleed over the margins or your report may not print properly and will look sloppy.
- You may want to have a 1.5-inch margin on the left-hand side of the page if you anticipate putting your study into a folder or binder, so all the words can be read comfortably when the pages are turned.
- Don't double-space your report unless you're writing it for a class assignment and the instructor or professor specifically tells you to do so.
- Use headers to add the page number to every page. You may also want to add your last name or the title of the study along with the page number.

- Citation methods typically are included in style manuals, which not only detail how you should cite your references but also have rules on acceptable punctuation and abbreviations, headings, and the general formatting of your report.
- For example, if you're writing a statistical report based on a psychological study, you typically must use the style manual published by the American Psychological Association (APA).
- Your citation method is all the more important if you anticipate your statistical report will be published in a particular trade or professional journal.

- If you're creating your statistical report for a class, a cover sheet may be required. Check with your instructor or professor or look on your assignment sheet to find out whether a cover sheet is required and what should be included on it.
- For longer statistical reports, you may also want to include a table of contents. You won't be able to format this until after you've finished the report, but it will list each section of your report and the page on which that section starts.

- If you decide to create section headings, they should be bold-faced and set off in such a way that they stand out from the rest of the text. For example, you may want to center bold-faced headings and use a slightly larger font size.
- Make sure a section heading doesn't fall at the bottom of the page. You should have at least a few lines of text, if not a full paragraph, below each section heading before the page break.

- Check the margins around visual elements and make sure the text lines up and is not too close to the visual element. You want it to be clear where the text ends and the words associated with the visual element (such as the axis labels for a graph) begin.
- Visual elements can cause your text to shift, so you'll need to double-check your section headings after your report is complete and make sure none of them are at the bottom of a page.
- Where possible, you also want to change your page breaks to eliminate situations in which the last line of a page is the first line of a paragraph, or the first line of a page is the last line of a paragraph. These are difficult to read.
Creating Your Content

- Avoid overly scientific or statistical language in your abstract as much as possible. Your abstract should be understandable to a larger audience than those who will be reading the entire report.
- It can help to think of your abstract as an elevator pitch. If you were in an elevator with someone and they asked you what your project was about, your abstract is what you would say to that person to describe your project.
- Even though your abstract appears first in your report, it's often easier to write it last, after you've completed the entire report.

- Aim for clear and concise language to set the tone for your report. Put your project in layperson's terms rather than using overly statistical language, regardless of the target audience of your report.
- If your report is based on a series of scientific experiments or data drawn from polls or demographic data, state your hypothesis or expectations going into the project.
- If other work has been done in the field regarding the same subject or similar questions, it's also appropriate to include a brief review of that work after your introduction. Explain why your work is different or what you hope to add to the existing body of work through your research.

- Include a description of any particular methods you used to track results, particularly if your experiments or studies were longer-term or observational in nature.
- If you had to make any adjustments during the development of the project, identify those adjustments and explain what required you to make them.
- List any software, resources, or other materials you used in the course of your research. If you used any textbook material, a reference is sufficient – there's no need to summarize that material in your report.

- Start with your main results, then include subsidiary results or interesting facts or trends you discovered.
- Generally you want to stay away from reporting results that have nothing to do with your original expectations or hypotheses. However, if you discovered something startling and unexpected through your research, you may want to at least mention it.
- This typically will be the longest section of your report, with the most detailed statistics. It also will be the driest and most difficult section for your readers to get through, especially if they are not statisticians.
- Small graphs or charts often show your results more clearly than you can write them in text.

- When you get to this section of your report, leave the heavy, statistical language behind. This section should be easy for anyone to understand, even if they skipped over your results section.
- If any additional research or study is necessary to further explore your hypotheses or answer questions that arose in the context of your project, describe that as well.

- It is often the case that you see things in hindsight that would have made data-gathering easier or more efficient. This is the place to discuss those. Since the scientific method is designed so that others can repeat your study, you want to pass on to future researchers your insights.
- Any speculation you have, or additional questions that came to mind over the course of your study, also are appropriate here. Just make sure you keep it to a minimum – you don't want your personal opinions and speculation to overtake the project itself.

- For example, if you compared your study to a similar study conducted in another city the year before yours, you would want to include a citation to that report in your references.
- Cite your references using the appropriate citation method for your discipline or field of study.
- Avoid citing any references that you did not mention in your report. For example, you may have done some background reading in preparation for your project. However, if you didn't end up directly citing any of those sources in your report, there's no need to list them in your references.

- Avoid trade "terms of art" or industry jargon if your report will be read mainly by people outside your particular industry.
- Make sure the terms of art and statistical terms that you do use in your report are used correctly. For example, you shouldn't use the word "average" in a statistical report because people often use that word to refer to different measures. Instead, use "mean," "median," or "mode" – whichever is correct.
Presenting Your Data

- This is particularly important if you're submitting your report for publication in a trade journal. If the pages are different sizes than the paper you print your report on, your visual elements won't line up the same way in the journal as they do in your manuscript.
- This also can be a factor if your report will be published online, since different display sizes can cause visual elements to display differently.
- The easiest way to label your visual elements is "Figure," followed by a number. Then you simply number each element sequentially in the order in which they appear in your report.
- Your title describes the information presented by the visual element. For example, if you've created a bar graph that shows the test scores of students on the chemistry class final, you might title it "Chemistry Final Test Scores, Fall 2016."

- Make sure each visual element is large enough in size that your readers can see everything they need to see without squinting. If you have to shrink down a graph to the point that readers can't make out the labels, it won't be very helpful to them.
- Create your visual elements using a format that you can easily import into your word-processing file. Importing using some graphics formats can distort the image or result in extremely low resolution.

- For example, if you have hundreds of samples, your x axis will be cluttered if you display each sample individually as a bar. However, you can move the measure on the y axis to the x axis, and use the y axis to measure the frequency.
- When your data include percentages, only go out to fractions of a percentage if your research demands it. If the smallest difference between your subjects is two percentage points, there's no need to display more than the whole percentage. However, if the difference between your subjects comes down to hundredths of a percent, you would need to display percentages to two decimal places so the graph would show the difference.
- For example, if your report includes a bar graph of the distribution of test scores for a chemistry class, and those scores are 97.56, 97.52, 97.46, and 97.61, your x axis would be each of the students and your y axis would start at 97 and go up to 98. This would highlight the differences in the students' scores.

- Be careful that your appendix does not overwhelm your report. You don't necessarily want to include every data sheet or other document you created over the course of your project.
- Rather, you only want to include documents that reasonably expand and lead to a further understanding of your report.
- For example, when describing your methods you state that a survey was conducted of students in a chemistry class to determine how they studied for the final exam. You might include a copy of the questions the students were asked in an appendix. However, you wouldn't necessarily need to include a copy of each student's answers to those questions.
Statistical Report Outline

Community Q&A

You Might Also Like

- ↑ https://www.ibm.com/docs/en/iotdm/11.3?topic=SSMLQ4_11.3.0/com.ibm.nex.optimd.dg.doc/11arcperf/oparcuse-r-statistical_reports.html
- ↑ https://www.examples.com/business/report/statistics-report.html
- ↑ https://collaboratory.ucr.edu/sites/g/files/rcwecm2761/files/2019-04/Final_Report_dan.pdf
- ↑ https://tex.stackexchange.com/questions/49386/what-is-the-recommended-font-to-use-for-a-statistical-table-in-an-academic-journ
- ↑ https://psychology.ucsd.edu/undergraduate-program/undergraduate-resources/academic-writing-resources/writing-research-papers/citing-references.html
- ↑ https://www.youtube.com/watch?v=kl3JOCmuil4
About This Article

Start your statistical report with an introduction explaining the purpose of your research. Then, dive into your research methods, how you collected data, and the experiments you conducted. Present you results with any necessary charts and graphs, but do not discuss or analyze the numbers -- in a statistical report, all analysis should happen in the conclusion. Once you’ve finished writing your report, draft a 200 word abstract and create a cover sheet with your name, the date, and the report title. Don’t forget to cite the appropriate references when necessary! For more formatting help, read on! Did this summary help you? Yes No
- Send fan mail to authors
Reader Success Stories

Dorothy Walter
Jan 15, 2017
Did this article help you?

Sarvath Ali
Feb 10, 2017

Mar 8, 2018

Sonam Sharma
Apr 30, 2019

Ashley Persaud
Jan 23, 2018

Featured Articles

Trending Articles

Watch Articles

- Terms of Use
- Privacy Policy
- Do Not Sell or Share My Info
- Not Selling Info
Get all the best how-tos!
Sign up for wikiHow's weekly email newsletter
- Dissertation
- PowerPoint Presentation
- Book Report/Review
- Research Proposal
- Math Problems
- Proofreading
- Movie Review
- Cover Letter Writing
- Personal Statement
- Nursing Paper
- Argumentative Essay
- Research Paper
How To Write A Statistics Research Paper?
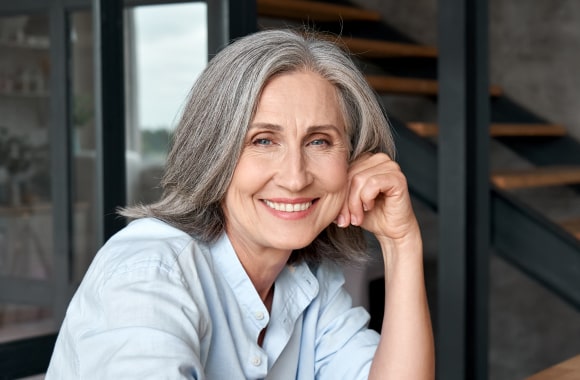
Table of Contents
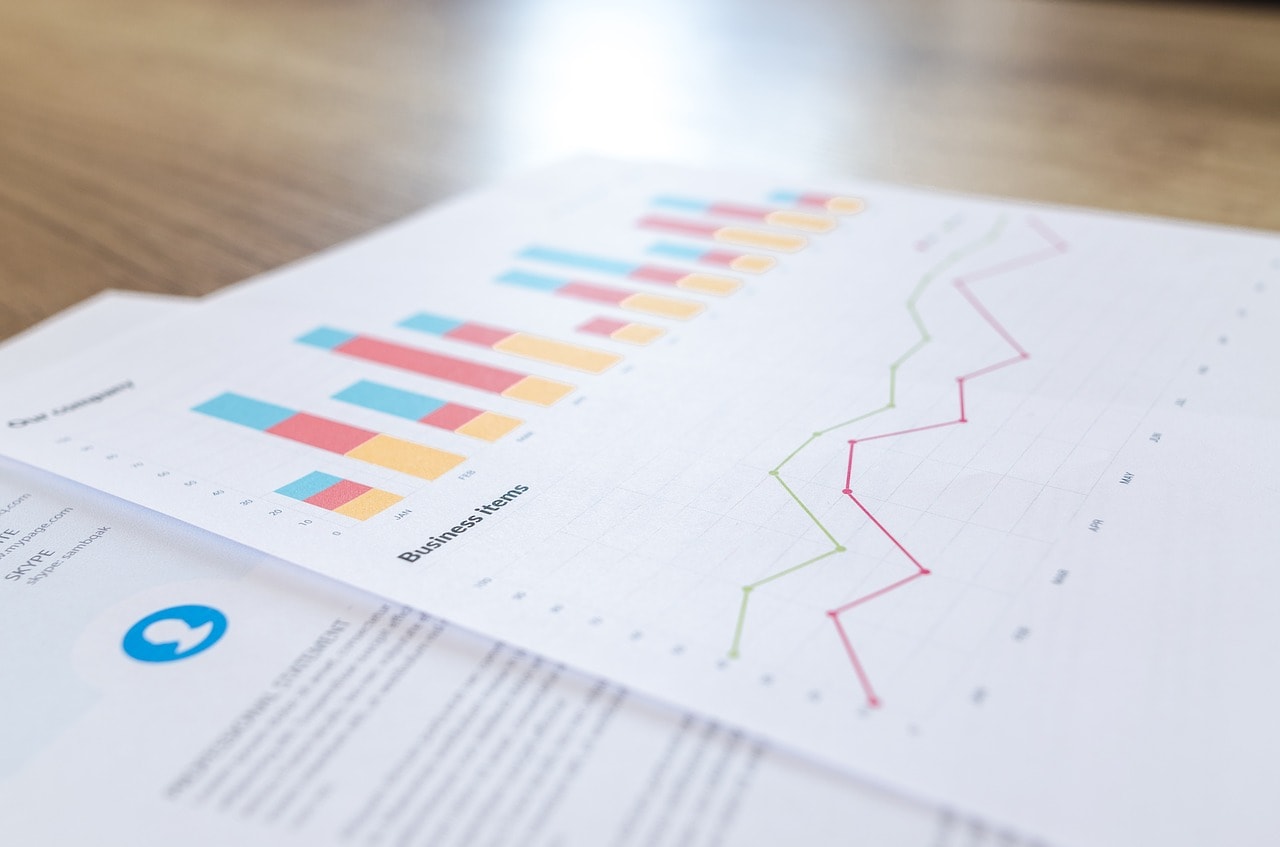
Naturally, all-encompassing information about the slightest details of the statistical paper writing cannot be stuffed into one guideline. Still, we will provide a glimpse of the basics of the stats research paper.
What is a stats research paper?
One of the main problems of stats academic research papers is that not all students understand what it is. Put it bluntly, it is an essay that provides an analysis of the gathered statistical data to induce the key points of a specified research issue. Thus, the author of the paper creates a construct of the topic by explaining the statistical data.
Writing a statistics research paper is quite challenging because the sources of data for statistical analysis are quite numerous. These are data mining, biostatistics, quality control, surveys, statistical modelling, etc.
Collecting data for the college research paper analysis is another headache. Research papers of this type call for the data taken from the most reliable and relevant sources because no indeterminate information is inadmissible here.
How to create the perfect statistics research paper example?
If you want to create the paper that can serve as a research paper writing example of well-written statistics research paper example, then here is a guideline that will help you to master this task.
Select the topic
Obviously, work can’t be written without a topic. Therefore, it is essential to come up with the theme that promises interesting statistics, and a possibility to gather enough data for the research. Access to the reliable sources of the research data is also a must.
If you are not confident about the availability of several sources concerning the chosen topic, you’d better choose something else.
Remember to jot down all the needed information for the proper referencing when you use a resource
Data collection
The duration of this stage depends on the number of data sources and the chosen methodology of the data collection. Mind that once you have chosen the method, you should stick to it. Naturally, it is essential to explain your choice of the methodology in your statistics research paper.
Outlining the paper
Creating a rough draft of the paper is your chance to save some time and nerves. Once you’ve done it, you get a clear picture of what to write about and what points should be worked through.
The intro section
This is, perhaps, the most important part of the paper. As this is the most scientific paper from all the papers you will have to write in your studies, it calls for the most logical and clear approach. Thus, your intro should consist of:
- Opening remarks about the field of the research.
- Credits to other researchers who worked on this theme.
- The scientific motivation for the new research .
- An explanation of why existing researches are not sufficient.
- The thesis statement , aka the core idea of the text.
The body of the text (research report, as they say in statistics)
Believe it or not, but many professional writers start such papers from the body. Here you have to place the Methodology Section where you establish the methods of data collection and the results of it. Usually, all main graphs or charts are placed here as a way to convey the results. All additional materials are gathered in the appendices.
The next paragraph of the paper will be the Evaluation of the gathered data . And that’s where the knowledge on how to read statistics in a research paper can come in handy. If you have no clue how to do it, you’re in trouble, to be honest. At least, you should know three concepts: odds ratios, confidence intervals, and p values. You can start searching for them on the web or in B.S.Everitt’s Dictionary of Statistics.
And the last section of the body is Discussion . Here, as the name suggests, you have to discuss the analysis and the results of the research.
The conclusion
This section requires only several sentences where you summarise the findings and highlight the importance of the research. You may also include a suggestion on how to continue or deepen the research of the issue.
Tips on how to write a statistics paper example
Here are some life hacks and shortcuts that you may use to boost your paper:
- Many sources where you take the statistical data , do offer it with the interpretation. Do not waste time on calculations and take the interpretation from there.
- Visuals are the must: always include a graph, chart, or a table to visualize your words.
- If you do not know the statistical procedure and how to interpret the results , never use it in the paper.
- Always put the statistics at the end of the sentence.
- If your paper requires the presentation of your calculations and you are not confident with it, ask a pro to help you.
- Different types of statistical data require proper formatting. Cite statistics properly according to the chosen format.
…Final thoughts
We hope that our guideline on how to write a statistics paper example unveiled the mystery of writing such papers.
But, in the case you still dread stats essays, here is a sound solution: entrust your task to the professionals! Order a paper at trustworthy writing service and enjoy saved time and the great result.
Psst… You can hand this work to a writer and have a completely stress-free evening. It would take like 3 minutes. And your perfect statistics research paper would be done on time!

Reasons to Select Your College Major As Soon As Possible
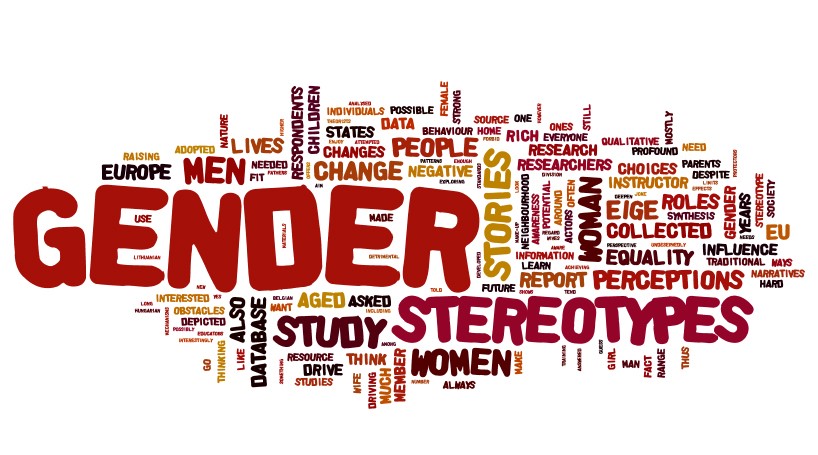
How to write an essay on sterotypes
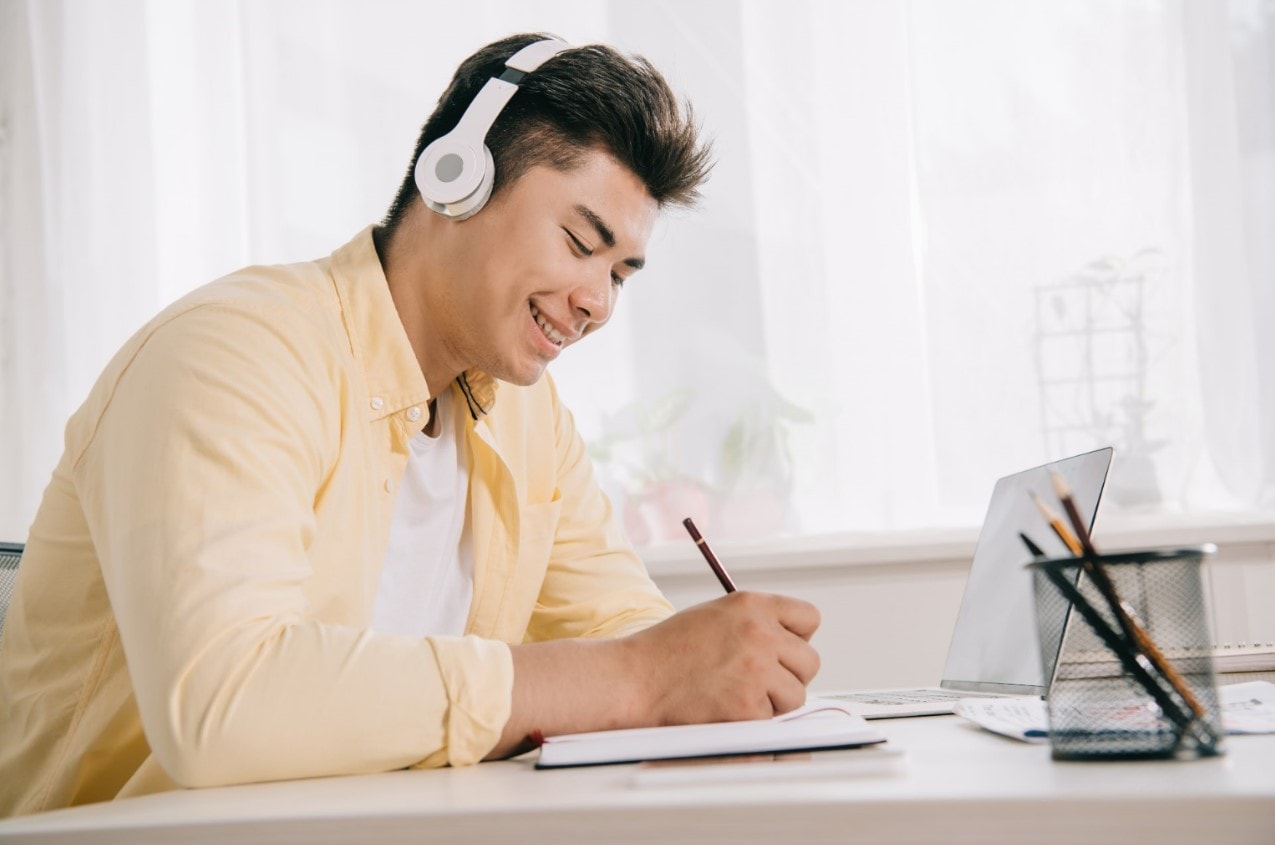
One-And-Done Guide To A-Grade Worthy Essays On Nature

Top 99+ Trending Statistics Research Topics for Students
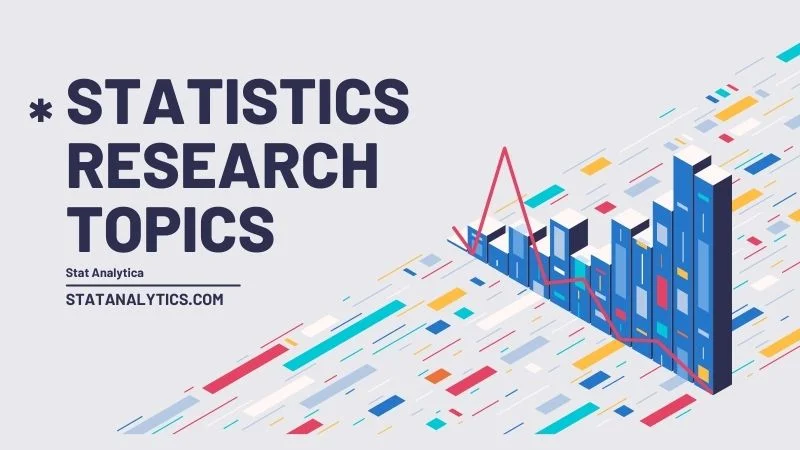
Being a statistics student, finding the best statistics research topics is quite challenging. But not anymore; find the best statistics research topics now!!!
Statistics is one of the tough subjects because it consists of lots of formulas, equations and many more. Therefore the students need to spend their time to understand these concepts. And when it comes to finding the best statistics research project for their topics, statistics students are always looking for someone to help them.
In this blog, we will share with you the most interesting and trending statistics research topics in 2023. It will not just help you to stand out in your class but also help you to explore more about the world.
If you face any problem regarding statistics, then don’t worry. You can get the best statistics assignment help from one of our experts.
As you know, it is always suggested that you should work on interesting topics. That is why we have mentioned the most interesting research topics for college students and high school students. Here in this blog post, we will share with you the list of 99+ awesome statistics research topics.
Why Do We Need to Have Good Statistics Research Topics?
Table of Contents
Having a good research topic will not just help you score good grades, but it will also allow you to finish your project quickly. Because whenever we work on something interesting, our productivity automatically boosts. Thus, you need not invest lots of time and effort, and you can achieve the best with minimal effort and time.
What Are Some Interesting Research Topics?
If we talk about the interesting research topics in statistics, it can vary from student to student. But here are the key topics that are quite interesting for almost every student:-
- Literacy rate in a city.
- Abortion and pregnancy rate in the USA.
- Eating disorders in the citizens.
- Parent role in self-esteem and confidence of the student.
- Uses of AI in our daily life to business corporates.
Top 99+ Trending Statistics Research Topics For 2023
Here in this section, we will tell you more than 99 trending statistics research topics:
Sports Statistics Research Topics
- Statistical analysis for legs and head injuries in Football.
- Statistical analysis for shoulder and knee injuries in MotoGP.
- Deep statistical evaluation for the doping test in sports from the past decade.
- Statistical observation on the performance of athletes in the last Olympics.
- Role and effect of sports in the life of the student.
Psychology Research Topics for Statistics
- Deep statistical analysis of the effect of obesity on the student’s mental health in high school and college students.
- Statistical evolution to find out the suicide reason among students and adults.
- Statistics analysis to find out the effect of divorce on children in a country.
- Psychology affects women because of the gender gap in specific country areas.
- Statistics analysis to find out the cause of online bullying in students’ lives.
- In Psychology, PTSD and descriptive tendencies are discussed.
- The function of researchers in statistical testing and probability.
- Acceptable significance and probability thresholds in clinical Psychology.
- The utilization of hypothesis and the role of P 0.05 for improved comprehension.
- What types of statistical data are typically rejected in psychology?
- The application of basic statistical principles and reasoning in psychological analysis.
- The role of correlation is when several psychological concepts are at risk.
- Actual case study learning and modeling are used to generate statistical reports.
- In psychology, naturalistic observation is used as a research sample.
- How should descriptive statistics be used to represent behavioral data sets?
Applied Statistics Research Topics
- Does education have a deep impact on the financial success of an individual?
- The investment in digital technology is having a meaningful return for corporations?
- The gap of financial wealth between rich and poor in the USA.
- A statistical approach to identify the effects of high-frequency trading in financial markets.
- Statistics analysis to determine the impact of the multi-agent model in financial markets.
Personalized Medicine Statistics Research Topics
- Statistical analysis on the effect of methamphetamine on substance abusers.
- Deep research on the impact of the Corona vaccine on the Omnicrone variant.
- Find out the best cancer treatment approach between orthodox therapies and alternative therapies.
- Statistics analysis to identify the role of genes in the child’s overall immunity.
- What factors help the patients to survive from Coronavirus .
Experimental Design Statistics Research Topics
- Generic vs private education is one of the best for the students and has better financial return.
- Psychology vs physiology: which leads the person not to quit their addictions?
- Effect of breastmilk vs packed milk on the infant child overall development
- Which causes more accidents: male alcoholics vs female alcoholics.
- What causes the student not to reveal the cyberbullying in front of their parents in most cases.
Easy Statistics Research Topics
- Application of statistics in the world of data science
- Statistics for finance: how statistics is helping the company to grow their finance
- Advantages and disadvantages of Radar chart
- Minor marriages in south-east Asia and African countries.
- Discussion of ANOVA and correlation.
- What statistical methods are most effective for active sports?
- When measuring the correctness of college tests, a ranking statistical approach is used.
- Statistics play an important role in Data Mining operations.
- The practical application of heat estimation in engineering fields.
- In the field of speech recognition, statistical analysis is used.
- Estimating probiotics: how much time is necessary for an accurate statistical sample?
- How will the United States population grow in the next twenty years?
- The legislation and statistical reports deal with contentious issues.
- The application of empirical entropy approaches with online grammar checking.
- Transparency in statistical methodology and the reporting system of the United States Census Bureau.
Statistical Research Topics for High School
- Uses of statistics in chemometrics
- Statistics in business analytics and business intelligence
- Importance of statistics in physics.
- Deep discussion about multivariate statistics
- Uses of Statistics in machine learning
Survey Topics for Statistics
- Gather the data of the most qualified professionals in a specific area.
- Survey the time wasted by the students in watching Tvs or Netflix.
- Have a survey the fully vaccinated people in the USA
- Gather information on the effect of a government survey on the life of citizens
- Survey to identify the English speakers in the world.
Statistics Research Paper Topics for Graduates
- Have a deep decision of Bayes theorems
- Discuss the Bayesian hierarchical models
- Analysis of the process of Japanese restaurants.
- Deep analysis of Lévy’s continuity theorem
- Analysis of the principle of maximum entropy
AP Statistics Topics
- Discuss about the importance of econometrics
- Analyze the pros and cons of Probit Model
- Types of probability models and their uses
- Deep discussion of ortho stochastic matrix
- Find out the ways to get an adjacency matrix quickly
Good Statistics Research Topics
- National income and the regulation of cryptocurrency.
- The benefits and drawbacks of regression analysis.
- How can estimate methods be used to correct statistical differences?
- Mathematical prediction models vs observation tactics.
- In sociology research, there is bias in quantitative data analysis.
- Inferential analytical approaches vs. descriptive statistics.
- How reliable are AI-based methods in statistical analysis?
- The internet news reporting and the fluctuations: statistics reports.
- The importance of estimate in modeled statistics and artificial sampling.
Business Statistics Topics
- Role of statistics in business in 2023
- Importance of business statistics and analytics
- What is the role of central tendency and dispersion in statistics
- Best process of sampling business data.
- Importance of statistics in big data.
- The characteristics of business data sampling: benefits and cons of software solutions.
- How may two different business tasks be tackled concurrently using linear regression analysis?
- In economic data relations, index numbers, random probability, and correctness are all important.
- The advantages of a dataset approach to statistics in programming statistics.
- Commercial statistics: how should the data be prepared for maximum accuracy?
Statistical Research Topics for College Students
- Evaluate the role of John Tukey’s contribution to statistics.
- The role of statistics to improve ADHD treatment.
- The uses and timeline of probability in statistics.
- Deep analysis of Gertrude Cox’s experimental design in statistics.
- Discuss about Florence Nightingale in statistics.
- What sorts of music do college students prefer?
- The Main Effect of Different Subjects on Student Performance.
- The Importance of Analytics in Statistics Research.
- The Influence of a Better Student in Class.
- Do extracurricular activities help in the transformation of personalities?
- Backbenchers’ Impact on Class Performance.
- Medication’s Importance in Class Performance.
- Are e-books better than traditional books?
- Choosing aspects of a subject in college
How To Write Good Statistics Research Topics?
So, the main question that arises here is how you can write good statistics research topics. The trick is understanding the methodology that is used to collect and interpret statistical data. However, if you are trying to pick any topic for your statistics project, you must think about it before going any further.
As a result, it will teach you about the data types that will be researched because the sample will be chosen correctly. On the other hand, your basic outline for choosing the correct topics is as follows:
- Introduction of a problem
- Methodology explanation and choice.
- Statistical research itself is in the main part (Body Part).
- Samples deviations and variables.
- Lastly, statistical interpretation is your last part (conclusion).
Note: Always include the sources from which you obtained the statistics data.
Top 3 Tips to Choose Good Statistics Research Topics
It can be quite easy for some students to pick a good statistics research topic without the help of an essay writer. But we know that it is not a common scenario for every student. That is why we will mention some of the best tips that will help you choose good statistics research topics for your next project. Either you are in a hurry or have enough time to explore. These tips will help you in every scenario.
1. Narrow down your research topic
We all start with many topics as we are not sure about our specific interests or niche. The initial step to picking up a good research topic for college or school students is to narrow down the research topic.
For this, you need to categorize the matter first. And then pick a specific category as per your interest. After that, brainstorm about the topic’s content and how you can make the points catchy, focused, directional, clear, and specific.
2. Choose a topic that gives you curiosity
After categorizing the statistics research topics, it is time to pick one from the category. Don’t pick the most common topic because it will not help your grades and knowledge. Instead of it, please choose the best one, in which you have little information, or you are more likely to explore it.
In a statistics research paper, you always can explore something beyond your studies. By doing this, you will be more energetic to work on this project. And you will also feel glad to get them lots of information you were willing to have but didn’t get because of any reasons.
It will also make your professor happy to see your work. Ultimately it will affect your grades with a positive attitude.
3. Choose a manageable topic
Now you have decided on the topic, but you need to make sure that your research topic should be manageable. You will have limited time and resources to complete your project if you pick one of the deep statistics research topics with massive information.
Then you will struggle at the last moment and most probably not going to finish your project on time. Therefore, spend enough time exploring the topic and have a good idea about the time duration and resources you will use for the project.
Statistics research topics are massive in numbers. Because statistics operations can be performed on anything from our psychology to our fitness. Therefore there are lots more statistics research topics to explore. But if you are not finding it challenging, then you can take the help of our statistics experts . They will help you to pick the most interesting and trending statistics research topics for your projects.
With this help, you can also save your precious time to invest it in something else. You can also come up with a plethora of topics of your choice and we will help you to pick the best one among them. Apart from that, if you are working on a project and you are not sure whether that is the topic that excites you to work on it or not. Then we can also help you to clear all your doubts on the statistics research topic.
Frequently Asked Questions
Q1. what are some good topics for the statistics project.
Have a look at some good topics for statistics projects:- 1. Research the average height and physics of basketball players. 2. Birth and death rate in a specific city or country. 3. Study on the obesity rate of children and adults in the USA. 4. The growth rate of China in the past few years 5. Major causes of injury in Football
Q2. What are the topics in statistics?
Statistics has lots of topics. It is hard to cover all of them in a short answer. But here are the major ones: conditional probability, variance, random variable, probability distributions, common discrete, and many more.
Q3. What are the top 10 research topics?
Here are the top 10 research topics that you can try in 2023:
1. Plant Science 2. Mental health 3. Nutritional Immunology 4. Mood disorders 5. Aging brains 6. Infectious disease 7. Music therapy 8. Political misinformation 9. Canine Connection 10. Sustainable agriculture
Related Posts
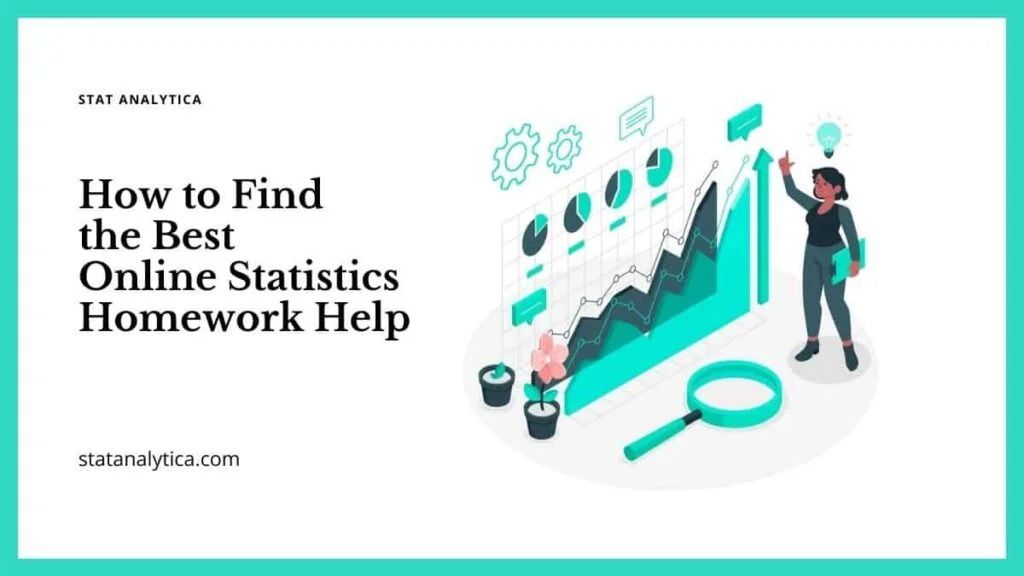
How to Find the Best Online Statistics Homework Help
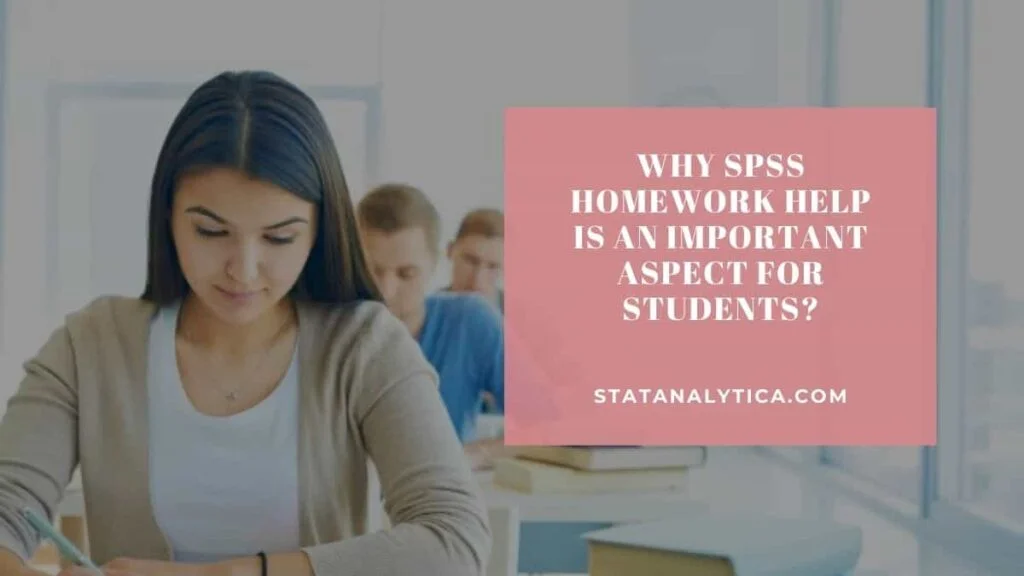
Why SPSS Homework Help Is An Important aspect for Students?
Statistics Research Paper
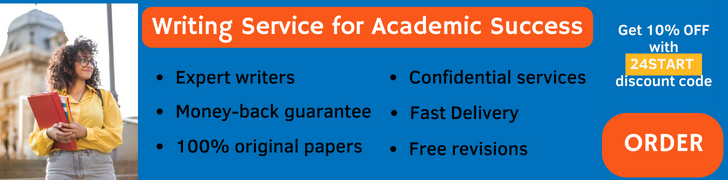
View sample Statistics Research Paper. Browse other research paper examples and check the list of research paper topics for more inspiration. If you need a religion research paper written according to all the academic standards, you can always turn to our experienced writers for help. This is how your paper can get an A! Feel free to contact our custom writing service s for professional assistance. We offer high-quality assignments for reasonable rates.

Academic Writing, Editing, Proofreading, And Problem Solving Services
Get 10% off with 24start discount code, more statistics research papers:.
- Time Series Research Paper
- Crime Statistics Research Paper
- Economic Statistics Research Paper
- Education Statistics Research Paper
- Health Statistics Research Paper
- Labor Statistics Research Paper
- History of Statistics Research Paper
- Survey Sampling Research Paper
- Multidimensional Scaling Research Paper
- Sequential Statistical Methods Research Paper
- Simultaneous Equation Estimation Research Paper
- Statistical Clustering Research Paper
- Statistical Sufficiency Research Paper
- Censuses Of Population Research Paper
- Stochastic Models Research Paper
- Stock Market Predictability Research Paper
- Structural Equation Modeling Research Paper
- Survival Analysis Research Paper
- Systems Modeling Research Paper
- Nonprobability Sampling Research Paper
1. Introduction
Statistics is a body of quantitative methods associated with empirical observation. A primary goal of these methods is coping with uncertainty. Most formal statistical methods rely on probability theory to express this uncertainty and to provide a formal mathematical basis for data description and for analysis. The notion of variability associated with data, expressed through probability, plays a fundamental role in this theory. As a consequence, much statistical effort is focused on how to control and measure variability and/or how to assign it to its sources.
Almost all characterizations of statistics as a field include the following elements:
(a) Designing experiments, surveys, and other systematic forms of empirical study.
(b) Summarizing and extracting information from data.
(c) Drawing formal inferences from empirical data through the use of probability.
(d) Communicating the results of statistical investigations to others, including scientists, policy makers, and the public.
This research paper describes a number of these elements, and the historical context out of which they grew. It provides a broad overview of the field, that can serve as a starting point to many of the other statistical entries in this encyclopedia.
2. The Origins Of The Field of Statistics
The word ‘statistics’ is related to the word ‘state’ and the original activity that was labeled as statistics was social in nature and related to elements of society through the organization of economic, demographic, and political facts. Paralleling this work to some extent was the development of the probability calculus and the theory of errors, typically associated with the physical sciences. These traditions came together in the nineteenth century and led to the notion of statistics as a collection of methods for the analysis of scientific data and the drawing of inferences therefrom.
As Hacking (1990) has noted: ‘By the end of the century chance had attained the respectability of a Victorian valet, ready to be the logical servant of the natural, biological and social sciences’ ( p. 2). At the beginning of the twentieth century, we see the emergence of statistics as a field under the leadership of Karl Pearson, George Udny Yule, Francis Y. Edgeworth, and others of the ‘English’ statistical school. As Stigler (1986) suggests:
Before 1900 we see many scientists of different fields developing and using techniques we now recognize as belonging to modern statistics. After 1900 we begin to see identifiable statisticians developing such techniques into a unified logic of empirical science that goes far beyond its component parts. There was no sharp moment of birth; but with Pearson and Yule and the growing number of students in Pearson’s laboratory, the infant discipline may be said to have arrived. (p. 361)
Pearson’s laboratory at University College, London quickly became the first statistics department in the world and it was to influence subsequent developments in a profound fashion for the next three decades. Pearson and his colleagues founded the first methodologically-oriented statistics journal, Biometrika, and they stimulated the development of new approaches to statistical methods. What remained before statistics could legitimately take on the mantle of a field of inquiry, separate from mathematics or the use of statistical approaches in other fields, was the development of the formal foundations of theories of inference from observations, rooted in an axiomatic theory of probability.
Beginning at least with the Rev. Thomas Bayes and Pierre Simon Laplace in the eighteenth century, most early efforts at statistical inference used what was known as the method of inverse probability to update a prior probability using the observed data in what we now refer to as Bayes’ Theorem. (For a discussion of who really invented Bayes’ Theorem, see Stigler 1999, Chap. 15). Inverse probability came under challenge in the nineteenth century, but viable alternative approaches gained little currency. It was only with the work of R. A. Fisher on statistical models, estimation, and significance tests, and Jerzy Neyman and Egon Pearson, in the 1920s and 1930s, on tests of hypotheses, that alternative approaches were fully articulated and given a formal foundation. Neyman’s advocacy of the role of probability in the structuring of a frequency-based approach to sample surveys in 1934 and his development of confidence intervals further consolidated this effort at the development of a foundation for inference (cf. Statistical Methods, History of: Post- 1900 and the discussion of ‘The inference experts’ in Gigerenzer et al. 1989).
At about the same time Kolmogorov presented his famous axiomatic treatment of probability, and thus by the end of the 1930s, all of the requisite elements were finally in place for the identification of statistics as a field. Not coincidentally, the first statistical society devoted to the mathematical underpinnings of the field, The Institute of Mathematical Statistics, was created in the United States in the mid-1930s. It was during this same period that departments of statistics and statistical laboratories and groups were first formed in universities in the United States.
3. Emergence Of Statistics As A Field
3.1 the role of world war ii.
Perhaps the greatest catalysts to the emergence of statistics as a field were two major social events: the Great Depression of the 1930s and World War II. In the United States, one of the responses to the depression was the development of large-scale probability-based surveys to measure employment and unemployment. This was followed by the institutionalization of sampling as part of the 1940 US decennial census. But with World War II raging in Europe and in Asia, mathematicians and statisticians were drawn into the war effort, and as a consequence they turned their attention to a broad array of new problems. In particular, multiple statistical groups were established in both England and the US specifically to develop new methods and to provide consulting. (See Wallis 1980, on statistical groups in the US; Barnard and Plackett 1985, for related efforts in the United Kingdom; and Fienberg 1985). These groups not only created imaginative new techniques such as sequential analysis and statistical decision theory, but they also developed a shared research agenda. That agenda led to a blossoming of statistics after the war, and in the 1950s and 1960s to the creation of departments of statistics at universities—from coast to coast in the US, and to a lesser extent in England and elsewhere.
3.2 The Neo-Bayesian Revival
Although inverse probability came under challenge in the 1920s and 1930s, it was not totally abandoned. John Maynard Keynes (1921) wrote A Treatise on Probability that was rooted in this tradition, and Frank Ramsey (1926) provided an early effort at justifying the subjective nature of prior distributions and suggested the importance of utility functions as an adjunct to statistical inference. Bruno de Finetti provided further development of these ideas in the 1930s, while Harold Jeffreys (1938) created a separate ‘objective’ development of these and other statistical ideas on inverse probability.
Yet as statistics flourished in the post-World War II era, it was largely based on the developments of Fisher, Neyman and Pearson, as well as the decision theory methods of Abraham Wald (1950). L. J. Savage revived interest in the inverse probability approach with The Foundations of Statistics (1954) in which he attempted to provide the axiomatic foundation from the subjective perspective. In an essentially independent effort, Raiffa and Schlaifer (1961) attempted to provide inverse probability counterparts to many of the then existing frequentist tools, referring to these alternatives as ‘Bayesian.’ By 1960, the term ‘Bayesian inference’ had become standard usage in the statistical literature, the theoretical interest in the development of Bayesian approaches began to take hold, and the neo-Bayesian revival was underway. But the movement from Bayesian theory to statistical practice was slow, in large part because the computations associated with posterior distributions were an overwhelming stumbling block for those who were interested in the methods. Only in the 1980s and 1990s did new computational approaches revolutionize both Bayesian methods, and the interest in them, in a broad array of areas of application.
3.3 The Role Of Computation In Statistics
From the days of Pearson and Fisher, computation played a crucial role in the development and application of statistics. Pearson’s laboratory employed dozens of women who used mechanical devices to carry out the careful and painstaking calculations required to tabulate values from various probability distributions. This effort ultimately led to the creation of the Biometrika Tables for Statisticians that were so widely used by others applying tools such as chisquare tests and the like. Similarly, Fisher also developed his own set of statistical tables with Frank Yates when he worked at Rothamsted Experiment Station in the 1920s and 1930s. One of the most famous pictures of Fisher shows him seated at Whittingehame Lodge, working at his desk calculator (see Box 1978).
The development of the modern computer revolutionized statistical calculation and practice, beginning with the creation of the first statistical packages in the 1960s—such as the BMDP package for biological and medical applications, and Datatext for statistical work in the social sciences. Other packages soon followed—such as SAS and SPSS for both data management and production-like statistical analyses, and MINITAB for the teaching of statistics. In 2001, in the era of the desktop personal computer, almost everyone has easy access to interactive statistical programs that can implement complex statistical procedures and produce publication-quality graphics. And there is a new generation of statistical tools that rely upon statistical simulation such as the bootstrap and Markov Chain Monte Carlo methods. Complementing the traditional production-like packages for statistical analysis are more methodologically oriented languages such as S and S-PLUS, and symbolic and algebraic calculation packages. Statistical journals and those in various fields of application devote considerable space to descriptions of such tools.
4. Statistics At The End Of The Twentieth Century
It is widely recognized that any statistical analysis can only be as good as the underlying data. Consequently, statisticians take great care in the the design of methods for data collection and in their actual implementation. Some of the most important modes of statistical data collection include censuses, experiments, observational studies, and sample Surveys, all of which are discussed elsewhere in this encyclopedia. Statistical experiments gain their strength and validity both through the random assignment of treatments to units and through the control of nontreatment variables. Similarly sample surveys gain their validity for generalization through the careful design of survey questionnaires and probability methods used for the selection of the sample units. Approaches to cope with the failure to fully implement randomization in experiments or random selection in sample surveys are discussed in Experimental Design: Compliance and Nonsampling Errors.
Data in some statistical studies are collected essentially at a single point in time (cross-sectional studies), while in others they are collected repeatedly at several time points or even continuously, while in yet others observations are collected sequentially, until sufficient information is available for inferential purposes. Different entries discuss these options and their strengths and weaknesses.
After a century of formal development, statistics as a field has developed a number of different approaches that rely on probability theory as a mathematical basis for description, analysis, and statistical inference. We provide an overview of some of these in the remainder of this section and provide some links to other entries in this encyclopedia.
4.1 Data Analysis
The least formal approach to inference is often the first employed. Its name stems from a famous article by John Tukey (1962), but it is rooted in the more traditional forms of descriptive statistical methods used for centuries.
Today, data analysis relies heavily on graphical methods and there are different traditions, such as those associated with
(a) The ‘exploratory data analysis’ methods suggested by Tukey and others.
(b) The more stylized correspondence analysis techniques of Benzecri and the French school.
(c) The alphabet soup of computer-based multivariate methods that have emerged over the past decade such as ACE, MARS, CART, etc.
No matter which ‘school’ of data analysis someone adheres to, the spirit of the methods is typically to encourage the data to ‘speak for themselves.’ While no theory of data analysis has emerged, and perhaps none is to be expected, the flexibility of thought and method embodied in the data analytic ideas have influenced all of the other approaches.
4.2 Frequentism
The name of this group of methods refers to a hypothetical infinite sequence of data sets generated as was the data set in question. Inferences are to be made with respect to this hypothetical infinite sequence. (For details, see Frequentist Inference).
One of the leading frequentist methods is significance testing, formalized initially by R. A. Fisher (1925) and subsequently elaborated upon and extended by Neyman and Pearson and others (see below). Here a null hypothesis is chosen, for example, that the mean, µ, of a normally distributed set of observations is 0. Fisher suggested the choice of a test statistic, e.g., based on the sample mean, x, and the calculation of the likelihood of observing an outcome as or more extreme as x is from µ 0, a quantity usually labeled as the p-value. When p is small (e.g., less than 5 percent), either a rare event has occurred or the null hypothesis is false. Within this theory, no probability can be given for which of these two conclusions is the case.
A related set of methods is testing hypotheses, as proposed by Neyman and Pearson (1928, 1932). In this approach, procedures are sought having the property that, for an infinite sequence of such sets, in only (say) 5 percent for would the null hypothesis be rejected if the null hypothesis were true. Often the infinite sequence is restricted to sets having the same sample size, but this is unnecessary. Here, in addition to the null hypothesis, an alternative hypothesis is specified. This permits the definition of a power curve, reflecting the frequency of rejecting the null hypothesis when the specified alternative is the case. But, as with the Fisherian approach, no probability can be given to either the null or the alternative hypotheses.
The construction of confidence intervals, following the proposal of Neyman (1934), is intimately related to testing hypotheses; indeed a 95 percent confidence interval may be regarded as the set of null hypotheses which, had they been tested at the 5 percent level of significance, would not have been rejected. A confidence interval is a random interval, having the property that the specified proportion (say 95 percent) of the infinite sequence, of random intervals would have covered the true value. For example, an interval that 95 percent of the time (by auxiliary randomization) is the whole real line, and 5 percent of the time is the empty set, is a valid 95 percent confidence interval.
Estimation of parameters—i.e., choosing a single value of the parameters that is in some sense best—is also an important frequentist method. Many methods have been proposed, both for particular models and as general approaches regardless of model, and their frequentist properties explored. These methods usually extended to intervals of values through inversion of test statistics or via other related devices. The resulting confidence intervals share many of the frequentist theoretical properties of the corresponding test procedures.
Frequentist statisticians have explored a number of general properties thought to be desirable in a procedure, such as invariance, unbiasedness, sufficiency, conditioning on ancillary statistics, etc. While each of these properties has examples in which it appears to produce satisfactory recommendations, there are others in which it does not. Additionally, these properties can conflict with each other. No general frequentist theory has emerged that proposes a hierarchy of desirable properties, leaving a frequentist without guidance in facing a new problem.
4.3 Likelihood Methods
The likelihood function (first studied systematically by R. A. Fisher) is the probability density of the data, viewed as a function of the parameters. It occupies an interesting middle ground in the philosophical debate, as it is used both by frequentists (as in maximum likelihood estimation) and by Bayesians in the transition from prior distributions to posterior distributions. A small group of scholars (among them G. A. Barnard, A. W. F. Edwards, R. Royall, D. Sprott) have proposed the likelihood function as an independent basis for inference. The issue of nuisance parameters has perplexed this group, since maximization, as would be consistent with maximum likelihood estimation, leads to different results in general than does integration, which would be consistent with Bayesian ideas.
4.4 Bayesian Methods
Both frequentists and Bayesians accept Bayes’ Theorem as correct, but Bayesians use it far more heavily. Bayesian analysis proceeds from the idea that probability is personal or subjective, reflecting the views of a particular person at a particular point in time. These views are summarized in the prior distribution over the parameter space. Together the prior distribution and the likelihood function define the joint distribution of the parameters and the data. This joint distribution can alternatively be factored as the product of the posterior distribution of the parameter given the data times the predictive distribution of the data.
In the past, Bayesian methods were deemed to be controversial because of the avowedly subjective nature of the prior distribution. But the controversy surrounding their use has lessened as recognition of the subjective nature of the likelihood has spread. Unlike frequentist methods, Bayesian methods are, in principle, free of the paradoxes and counterexamples that make classical statistics so perplexing. The development of hierarchical modeling and Markov Chain Monte Carlo (MCMC) methods have further added to the current popularity of the Bayesian approach, as they allow analyses of models that would otherwise be intractable.
Bayesian decision theory, which interacts closely with Bayesian statistical methods, is a useful way of modeling and addressing decision problems of experimental designs and data analysis and inference. It introduces the notion of utilities and the optimum decision combines probabilities of events with utilities by the calculation of expected utility and maximizing the latter (e.g., see the discussion in Lindley 2000).
Current research is attempting to use the Bayesian approach to hypothesis testing to provide tests and pvalues with good frequentist properties (see Bayarri and Berger 2000).
4.5 Broad Models: Nonparametrics And Semiparametrics
These models include parameter spaces of infinite dimensions, whether addressed in a frequentist or Bayesian manner. In a sense, these models put more inferential weight on the assumption of conditional independence than does an ordinary parametric model.
4.6 Some Cross-Cutting Themes
Often different fields of application of statistics need to address similar issues. For example, dimensionality of the parameter space is often a problem. As more parameters are added, the model will in general fit better (at least no worse). Is the apparent gain in accuracy worth the reduction in parsimony? There are many different ways to address this question in the various applied areas of statistics.
Another common theme, in some sense the obverse of the previous one, is the question of model selection and goodness of fit. In what sense can one say that a set of observations is well-approximated by a particular distribution? (cf. Goodness of Fit: Overview). All statistical theory relies at some level on the use of formal models, and the appropriateness of those models and their detailed specification are of concern to users of statistical methods, no matter which school of statistical inference they choose to work within.
5. Statistics In The Twenty-first Century
5.1 adapting and generalizing methodology.
Statistics as a field provides scientists with the basis for dealing with uncertainty, and, among other things, for generalizing from a sample to a population. There is a parallel sense in which statistics provides a basis for generalization: when similar tools are developed within specific substantive fields, such as experimental design methodology in agriculture and medicine, and sample surveys in economics and sociology. Statisticians have long recognized the common elements of such methodologies and have sought to develop generalized tools and theories to deal with these separate approaches (see e.g., Fienberg and Tanur 1989).
One hallmark of modern statistical science is the development of general frameworks that unify methodology. Thus the tools of Generalized Linear Models draw together methods for linear regression and analysis of various models with normal errors and those log-linear and logistic models for categorical data, in a broader and richer framework. Similarly, graphical models developed in the 1970s and 1980s use concepts of independence to integrate work in covariance section, decomposable log-linear models, and Markov random field models, and produce new methodology as a consequence. And the latent variable approaches from psychometrics and sociology have been tied with simultaneous equation and measurement error models from econometrics into a broader theory of covariance analysis and structural equations models.
Another hallmark of modern statistical science is the borrowing of methods in one field for application in another. One example is provided by Markov Chain Monte Carlo methods, now used widely in Bayesian statistics, which were first used in physics. Survival analysis, used in biostatistics to model the disease-free time or time-to-mortality of medical patients, and analyzed as reliability in quality control studies, are now used in econometrics to measure the time until an unemployed person gets a job. We anticipate that this trend of methodological borrowing will continue across fields of application.
5.2 Where Will New Statistical Developments Be Focused ?
In the issues of its year 2000 volume, the Journal of the American Statistical Association explored both the state of the art of statistics in diverse areas of application, and that of theory and methods, through a series of vignettes or short articles. These essays provide an excellent supplement to the entries of this encyclopedia on a wide range of topics, not only presenting a snapshot of the current state of play in selected areas of the field but also affecting some speculation on the next generation of developments. In an afterword to the last set of these vignettes, Casella (2000) summarizes five overarching themes that he observed in reading through the entire collection:
(a) Large datasets.
(b) High-dimensional/nonparametric models.
(c) Accessible computing.
(d) Bayes/frequentist/who cares?
(e) Theory/applied/why differentiate?
Not surprisingly, these themes fit well those that one can read into the statistical entries in this encyclopedia. The coming together of Bayesian and frequentist methods, for example, is illustrated by the movement of frequentists towards the use of hierarchical models and the regular consideration of frequentist properties of Bayesian procedures (e.g., Bayarri and Berger 2000). Similarly, MCMC methods are being widely used in non-Bayesian settings and, because they focus on long-run sequences of dependent draws from multivariate probability distributions, there are frequentist elements that are brought to bear in the study of the convergence of MCMC procedures. Thus the oft-made distinction between the different schools of statistical inference (suggested in the preceding section) is not always clear in the context of real applications.
5.3 The Growing Importance Of Statistics Across The Social And Behavioral Sciences
Statistics touches on an increasing number of fields of application, in the social sciences as in other areas of scholarship. Historically, the closest links have been with economics; together these fields share parentage of econometrics. There are now vigorous interactions with political science, law, sociology, psychology, anthropology, archeology, history, and many others.
In some fields, the development of statistical methods has not been universally welcomed. Using these methods well and knowledgeably requires an understanding both of the substantive field and of statistical methods. Sometimes this combination of skills has been difficult to develop.
Statistical methods are having increasing success in addressing questions throughout the social and behavioral sciences. Data are being collected and analyzed on an increasing variety of subjects, and the analyses are becoming increasingly sharply focused on the issues of interest.
We do not anticipate, nor would we find desirable, a future in which only statistical evidence was accepted in the social and behavioral sciences. There is room for, and need for, many different approaches. Nonetheless, we expect the excellent progress made in statistical methods in the social and behavioral sciences in recent decades to continue and intensify.
Bibliography:
- Barnard G A, Plackett R L 1985 Statistics in the United Kingdom, 1939–1945. In: Atkinson A C, Fienberg S E (eds.) A Celebration of Statistics: The ISI Centennial Volume. Springer-Verlag, New York, pp. 31–55
- Bayarri M J, Berger J O 2000 P values for composite null models (with discussion). Journal of the American Statistical Association 95: 1127–72
- Box J 1978 R. A. Fisher, The Life of a Scientist. Wiley, New York
- Casella G 2000 Afterword. Journal of the American Statistical Association 95: 1388
- Fienberg S E 1985 Statistical developments in World War II: An international perspective. In: Anthony C, Atkinson A C, Fienberg S E (eds.) A Celebration of Statistics: The ISI Centennial Volume. Springer-Verlag, New York, pp. 25–30
- Fienberg S E, Tanur J M 1989 Combining cognitive and statistical approaches to survey design. Science 243: 1017–22
- Fisher R A 1925 Statistical Methods for Research Workers. Oliver and Boyd, London
- Gigerenzer G, Swijtink Z, Porter T, Daston L, Beatty J, Kruger L 1989 The Empire of Chance. Cambridge University Press, Cambridge, UK
- Hacking I 1990 The Taming of Chance. Cambridge University Press, Cambridge, UK
- Jeffreys H 1938 Theory of Probability, 2nd edn. Clarendon Press, Oxford, UK
- Keynes J 1921 A Treatise on Probability. Macmillan, London
- Lindley D V 2000/1932 The philosophy of statistics (with discussion). The Statistician 49: 293–337
- Neyman J 1934 On the two different aspects of the representative method: the method of stratified sampling and the method of purposive selection (with discussion). Journal of the Royal Statistical Society 97: 558–625
- Neyman J, Pearson E S 1928 On the use and interpretation of certain test criteria for purposes of statistical inference. Part I. Biometrika 20A: 175–240
- Neyman J, Pearson E S 1932 On the problem of the most efficient tests of statistical hypotheses. Philosophical Transactions of the Royal Society, Series. A 231: 289–337
- Raiffa H, Schlaifer R 1961 Applied Statistical Decision Theory. Harvard Business School, Boston
- Ramsey F P 1926 Truth and probability. In: The Foundations of Mathematics and Other Logical Essays. Kegan Paul, London, pp.
- Savage L J 1954 The Foundations of Statistics. Wiley, New York
- Stigler S M 1986 The History of Statistics: The Measurement of Uncertainty Before 1900. Harvard University Press, Cambridge, MA
- Stigler S M 1999 Statistics on the Table: The History of Statistical Concepts and Methods. Harvard University Press, Cambridge, MA
- Tukey John W 1962 The future of data analysis. Annals of Mathematical Statistics 33: 1–67
- Wald A 1950 Statistical Decision Functions. Wiley, New York
- Wallis W 1980 The Statistical Research Group, 1942–1945 (with discussion). Journal of the American Statistical Association 75: 320–35
ORDER HIGH QUALITY CUSTOM PAPER

Research Paper Outline Generator
🤖 research outline generator 101.
- 📝 Template & Format
- 🤩 Outline Tips
🔗 References
Outlining an academic assignment serves several purposes:
- It lets students establish connections between the thesis and their ideas.
- A template also allows them to structure papers and identify gaps in their knowledge.
- An outline makes their writing more substantial and lets them know if they understand the topic well enough.
Our outline generator for research papers speeds up this process in several ways:
📝 Outline Generator for Research Paper: Template and Format
Our tool can help you cope with the first step in any academic work, whether it's a high school or college tasks. But what should you do next? This part of the article details the components of the research paper format. It will give you a better understanding of how your work should look.
Popular Academic Formats
The format of your research paper largely depends on the style your school uses. Three main styles are widely used in the academic setting: APA, Chicago, and MLA. Each has its features we’ll discuss briefly.
Good Example of a Template
In this section, we have detailed all the components of a comprehensive research paper. Knowing these elements will make it easy for you to structure your work.
🤩 Best Outline Tips: Research Papers & Academic Essays
We want to wrap things up by offering helpful tips for an effective outline. It doesn’t matter if you’re writing an academic essay, a research work, or a personal statement. These pieces of advice can be helpful in all types of assignments.
- Plan and brainstorm . Make a list of ideas that can become good topics for your essay. By the way, having study buddies is also a fantastic idea! Try to brainstorm topics with fellow students. This way, you can better plan and find impactful research subjects.
- Organize your ideas . Next, organize the ideas into separate categories. Discard bad ones and incorporate good ones in your writing. Narrow them down until you choose the main one to write a good thesis statement.
- Use bullet points . Utilize a list format to keep your outline organized. Use headings to easily navigate the template and structure the paper logically.
- Use online tools . If you need help, turn to online platforms for assistance. For example, our research paper outline generator can make this process faster.
- Write in your mother tongue . If you struggle writing in English , make notes in your native language. It’s also better to research this topic in your mother tongue. You can translate your findings later.
- Remove redundant points . Revise all the paragraphs of your finished essay template. Make sure that all the sections match the purpose of your paper and reveal the main idea. If you find too many inappropriate points, try rephrasing them to fit the topic.
We hope that our outline generator for research papers helped in your work. If you have any questions left, check out the FAQ section below. Besides we also recommend you take a look at our research guide .
❓ Research Outline Generator – FAQ
Updated: Dec 14th, 2023
- Writing a Research Paper. – Arrendale Library, Piedmont University
- How to Write an APA Research Paper – Hamilton College
- How To Write a Research Paper Outline (With Examples and Tips) – Indeed
- Chicago Style Guide – Menlo School
- MLA General Format – The On-Campus Writing Lab & The OWL at Purdue and Purdue University
- Formatting an Academic Paper – Augsburg University
- Free Essays
- Writing Tools
- Lit. Guides
- Donate a Paper
- Referencing Guides
- Free Textbooks
- Tongue Twisters
- Job Openings
- Expert Application
- Video Contest
- Writing Scholarship
- Discount Codes
- IvyPanda Shop
- Terms and Conditions
- Privacy Policy
- Cookies Policy
- Copyright Principles
- DMCA Request
- Service Notice
Learn everything there is to know about our awesome research paper outline generator. This instrument is guaranteed to save you lots of time and energy by creating the perfect plan for your assignment in seconds. Simply set the parameters and get to writing!
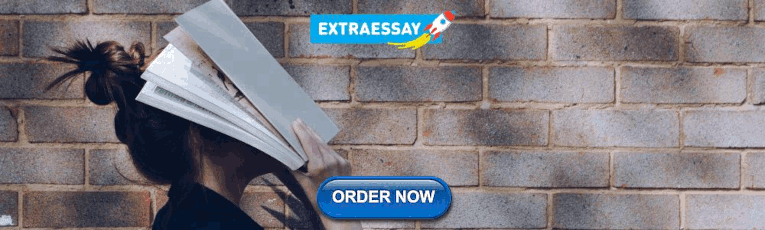
IMAGES
VIDEO
COMMENTS
12. Summary. As you read papers also notice the construction of the papers (learn from the good and bad examples). Abstract and Introduction { keys for getting readers engaged. Be gentle with your audience. Tell them your story. Writing is work { but ultimately rewarding! 13. Created Date.
A decimal outline is similar in format to the alphanumeric outline, but with a different numbering system: 1, 1.1, 1.2, etc. Text is written as short notes rather than full sentences. Example: 1 Body paragraph one. 1.1 First point. 1.1.1 Sub-point of first point. 1.1.2 Sub-point of first point.
The base of every research paper lies in its setup and outline. In this article, we'll look at the key steps involved in writing a successful research paper. ... Tips for Writing Statistics Research Paper. If you are wondering how people write their papers, you are in the right place. We'll take a look at a few pointers that can help you ...
A statistics research paper is an academic document presenting original findings or analyses derived from the data's collection, organization, analysis, and interpretation. ... It's an overview of your statistics research paper where you establish notation and outline the methods and the results. Abstracts are integral for all academic ...
Table of contents. Step 1: Write your hypotheses and plan your research design. Step 2: Collect data from a sample. Step 3: Summarize your data with descriptive statistics. Step 4: Test hypotheses or make estimates with inferential statistics.
This outline format uses numbers to organize the main ideas and supporting details of a research paper. It is similar to the alphanumeric outline, but it uses only numbers and decimals to indicate the hierarchy of the ideas. Example: 1.0 Introduction. 1.1 Background information.
1. Determine your topic. You'll need to establish a topic or the main point you intend to write about. For example, you may want to research and write about whether influencers are the most beneficial way to promote products in your industry. This topic is the main point around which your essay will revolve. 2.
11. Statistical report writing. Learning to write useful, productive and readable statistical reports is a critical data analysis skill. The book R eport Writing for Data Science in R by Roger D. Peng (freely dowloaded from LeanPub) is a useful reference for statistical report writing, especially when using the R programming language.
A research paper outline is a basic format for writing an academic research paper. In this article, we would like to discuss writing an outline with a structured research paper outline template, which will assist a researcher in writing their research paper effectively! ... Report the results with appropriate descriptive statistics. Furthermore ...
Tips for writing a research paper outline. Tip: The key to creating a useful outline is to be consistent in your headings, organization, and levels of specificity. Be Consistent: ensure every heading has a similar tone. State the topic or write short sentences for each heading but avoid doing both.
Statistical analysis is the systematic process of collecting, organizing, and interpreting numbers to reveal patterns and identify trends and relationships. It plays a crucial role in research by providing tools to analyze data objectively, remove bias, and draw conclusions. Moreover, statistical analysis aids in identifying correlations ...
An alphanumeric outline is a hierarchical structure used to outline a research paper, combining numbers and letters to signify the different levels of information. Main sections are designated with capital letters (A, B, C), which include major points, while subpoints are indicated by numbers and lowercase letters (1, 2, a, b).
A research paper outline is a skeleton or a guideline for your final paper. It is typically created after the thesis statement is formatted but before the first draft is written. In this process, you group information into appropriate headers, sub-headers, points, and sub-points. ... Statistics before the advent of the inactivated polio vaccine ...
Abstract. Statistical methods involved in carrying out a study include planning, designing, collecting data, analysing, drawing meaningful interpretation and reporting of the research findings. The statistical analysis gives meaning to the meaningless numbers, thereby breathing life into a lifeless data. The results and inferences are precise ...
Data and statistics serve as the backbone of empirical research, allowing researchers to move beyond theoretical assumptions and draw concrete conclusions. They provide credibility to your work, as they are objective and verifiable by other researchers. Differentiating Between Qualitative and Quantitative Research.
Write down all the ideas you want to include or discuss. 3. Gather information. Gather notes, resources and references that you will need to support your content. Also, complete any necessary research or investigations. Each main idea should have two or more supporting topics.
For example, a statistical report by a mathematician may look incredibly different than one created by a market researcher for a retail business. 2. Type your report in an easy-to-read font. Statistical reports typically are typed single-spaced, using a font such as Arial or Times New Roman in 12-point size.
Research Project Report Outline The goal of your report is to clearly communicate the process and the outcomes of your research project. As promised, here are some essential points that must be communicated in your report followed by a suggested outline. Of course, no one outline will fit every type of project, but it offers you some starting ...
Here you have to place the Methodology Section where you establish the methods of data collection and the results of it. Usually, all main graphs or charts are placed here as a way to convey the results. All additional materials are gathered in the appendices. The next paragraph of the paper will be the Evaluation of the gathered data. And that ...
Statistics Research Paper Topics for Graduates. Have a deep decision of Bayes theorems; Discuss the Bayesian hierarchical models; ... On the other hand, your basic outline for choosing the correct topics is as follows: Introduction of a problem; Methodology explanation and choice. Statistical research itself is in the main part (Body Part).
View sample Statistics Research Paper. Browse other research paper examples and check the list of research paper topics for more inspiration. If you need a religion research paper written according to all the academic standards, you can always turn to our experienced writers for help. This is how your paper can get an A!
Our outline generator for research papers speeds up this process in several ways: ⭐ High-quality. The tool is smart enough to create an outline on any topic effortlessly. It provides blueprints you can turn into examples of high-quality academic writing. 🎯 Motivating.
The function of statistics in research is to purpose as a tool in conniving research, analyzing its data and portrayal of conclusions there from. ... The article covers a brief outline of the ...