Internet of Behavior in Cybersecurity: Opportunities and Challenges
- Conference paper
- First Online: 16 November 2022
- Cite this conference paper
- Sagar Patel 41 &
- Nishant Doshi 41
Part of the book series: Lecture Notes in Electrical Engineering ((LNEE,volume 936))
913 Accesses
2 Citations
There is a dark side to the Internet of things, and experts believe that the integration of behavioral data may supply hackers with sensitive information about consumer behavior patterns. Cybercriminals may gather and sell compromised property access codes, delivery routes, and even bank access credentials to other thieves—the possibilities are limitless. Despite many public awareness efforts, researchers discovered that people continue to use hazardous password habits. Individual variations in cybersecurity practices are the subject of little study. This review focused on the perilous habit of password sharing. As expected, people with a low level of persistence were more inclined to disclose passwords. Passwords were more likely to be disclosed by older people and those who are skilled at self-monitoring.
This is a preview of subscription content, log in via an institution to check access.
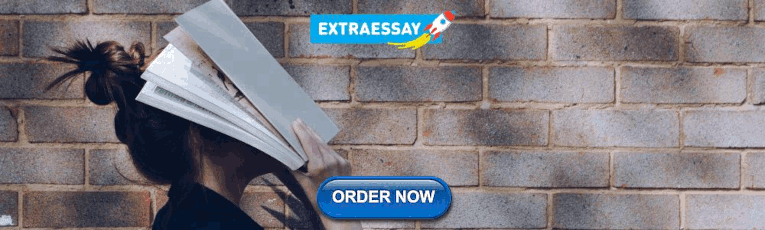
Access this chapter
- Available as PDF
- Read on any device
- Instant download
- Own it forever
- Available as EPUB and PDF
- Compact, lightweight edition
- Dispatched in 3 to 5 business days
- Free shipping worldwide - see info
- Durable hardcover edition
Tax calculation will be finalised at checkout
Purchases are for personal use only
Institutional subscriptions
Fatokun Faith B, Hamid S, Norman A, Fatokun Johnson O, Eke CI (March 2020) Relating factors of tertiary institution students. Cybersecurity Behav. https://doi.org/10.1109/ICMCECS47690.2020.246990
Li S, Feng B, Liao W, Pan W (1 June 2020) Internet use, risk awareness, and demographic characteristics associated with engagement in preventive behaviors and testing: cross-sectional survey on COVID-19 in the United States. J Med Int Res 22(6). JMIR Publications Inc. https://doi.org/10.2196/19782
de Kimpe L, Walrave M, Verdegem P, Ponnet K (2021) What we think we know about cybersecurity: an investigation of the relationship between perceived knowledge, internet trust, and protection motivation in a cybercrime context. Behav Inf Technol. https://doi.org/10.1080/0144929X.2021.1905066
Quayyum F, Cruzes DS, Jaccheri L (1 Dec 2021) Cybersecurity awareness for children: a systematic literature review. Int J Child-Comput Inter 30. Elsevier B.V. https://doi.org/10.1016/j.ijcci.2021.100343
Gillam AR, Foster WT (July 2020) Factors affecting risky cybersecurity behaviors by U.S. workers: an exploratory study. Comput Hum Behav 108. https://doi.org/10.1016/j.chb.2020.106319
Lee JK, Chang Y, Kwon HY, Kim B (2020) Reconciliation of privacy with preventive cybersecurity: the bright internet approach. Inf Syst Front 22(1):45–57. https://doi.org/10.1007/s10796-020-09984-5
Article Google Scholar
Venard B (2021) Cybersecurity behavior under covid-19 influence. In: 2021 international conference on cyber situational awareness, data analytics and assessment (CyberSA), pp 1–9. https://doi.org/10.1109/CyberSA52016.2021.9478238
Zwilling M, Klien G, Lesjak D, Wiechetek Ł, Cetin F, Basim HN (2020) Cyber security awareness, knowledge and behavior: a comparative study. J Comput Inf Syst 00(00):1–16. https://doi.org/10.1080/08874417.2020.1712269
Halevi T, Lewis J, Memon N (2013) A pilot study of cyber security and privacy related behavior and personality traits. WWW 2013 companion—proceedings of the 22nd international conference on world wide web, pp 737–744. https://doi.org/10.1145/2487788.2488034
Cain AA, Edwards ME, Still JD (2018) An exploratory study of cyber hygiene behaviors and knowledge. J Inf Sec Appl 42:36–45. https://doi.org/10.1016/j.jisa.2018.08.002
Gratian M, Bandi S, Cukier M, Dykstra J, Ginther A (2018) Correlating human traits and cyber security behavior intentions. Comput Secur 73:345–358. https://doi.org/10.1016/j.cose.2017.11.015
Javaid M, Haleem A, Singh RP, Rab S, Suman R (2021) Internet of Behaviours (IoB) and its role in customer services. Sens Int 2(7):100122. https://doi.org/10.1016/j.sintl.2021.100122
Moustafa AA, Bello A, Maurushat A (2021) The role of user behaviour in improving cyber security management. Front Psychol 12(June):1–9. https://doi.org/10.3389/fpsyg.2021.561011
Pfleeger SL, Caputo DD (2012) Leveraging behavioral science to mitigate cyber security risk. Comput Secur 31(4):597–611. https://doi.org/10.1016/j.cose.2011.12.010
Grobler M, Gaire R, Nepal S (March 2021) User, usage and usability: redefining human centric cyber security. Front Big Data 4. https://doi.org/10.3389/fdata.2021.583723
Mashiane T, Kritzinger E (2019) Cybersecurity behaviour: a conceptual taxonomy. In: Lecture notes in computer science (including subseries lecture notes in artificial intelligence and lecture notes in bioinformatics), vol 11469 LNCS, pp 147–156. https://doi.org/10.1007/978-3-030-20074-9_11
Sechi C, Loi G, Cabras C (2021) Addictive internet behaviors: the role of trait emotional intelligence, self-esteem, age, and gender. Scand J Psychol 62(3):409–417. https://doi.org/10.1111/sjop.12698
Critselis E et al (2014) Predictive factors and psychosocial effects of internet addictive behaviors in cypriot adolescents. Int J Adolesc Med Health 26(3):369–375. https://doi.org/10.1515/ijamh-2013-0313
Whitty M, Doodson J, Creese S, Hodges D (2015) Individual differences in cyber security behaviors: an examination of who is sharing passwords. Cyberpsychol Behav Soc Netw 18(1):3–7. https://doi.org/10.1089/cyber.2014.0179
Arend I, Shabtai A, Idan T, Keinan R, Bereby-Meyer Y (2020) Passive- and not active-risk tendencies predict cyber security behavior. Comput Secur 97:101964. https://doi.org/10.1016/j.cose.2020.101964
Download references
Author information
Authors and affiliations.
Department of Computer Science and Engineering, Pandit Deendayal Energy University, Gandhinagar, Gujarat, 382421, India
Sagar Patel & Nishant Doshi
You can also search for this author in PubMed Google Scholar
Corresponding author
Correspondence to Sagar Patel .
Editor information
Editors and affiliations.
KIET Group of Institutions, Ghaziabad, India
Pradeep Kumar Singh
Institute of Computer Science, Polish Academy of Sciences, Warsaw, Poland
Sławomir T. Wierzchoń
Department of Computer Engineering, NIT Kurukshetra, Haryana, India
Jitender Kumar Chhabra
Department of Computer Science and Engineering, Institute of Technology, Nirma University, Ahmedabad, Gujarat, India
Sudeep Tanwar
Rights and permissions
Reprints and permissions
Copyright information
© 2022 The Author(s), under exclusive license to Springer Nature Singapore Pte Ltd.
About this paper
Cite this paper.
Patel, S., Doshi, N. (2022). Internet of Behavior in Cybersecurity: Opportunities and Challenges. In: Singh, P.K., Wierzchoń, S.T., Chhabra, J.K., Tanwar, S. (eds) Futuristic Trends in Networks and Computing Technologies . Lecture Notes in Electrical Engineering, vol 936. Springer, Singapore. https://doi.org/10.1007/978-981-19-5037-7_14
Download citation
DOI : https://doi.org/10.1007/978-981-19-5037-7_14
Published : 16 November 2022
Publisher Name : Springer, Singapore
Print ISBN : 978-981-19-5036-0
Online ISBN : 978-981-19-5037-7
eBook Packages : Computer Science Computer Science (R0)
Share this paper
Anyone you share the following link with will be able to read this content:
Sorry, a shareable link is not currently available for this article.
Provided by the Springer Nature SharedIt content-sharing initiative
- Publish with us
Policies and ethics
- Find a journal
- Track your research
Help | Advanced Search
Computer Science > Software Engineering
Title: designing internet of behaviors systems.
Abstract: The Internet of Behaviors (IoB) puts human behavior at the core of engineering intelligent connected systems. IoB links the digital world to human behavior to establish human-driven design, development, and adaptation processes. This paper defines the novel concept by an IoB model based on a collective effort interacting with software engineers, human-computer interaction scientists, social scientists, and cognitive science communities. The model for IoB is created based on an exploratory study that synthesizes state-of-the-art analysis and experts interviews. The architecture of a real industry 4.0 manufacturing infrastructure helps to explain the IoB model and it's application. The conceptual model was used to successfully implement a socio-technical infrastructure for a crowd monitoring and queue management system for the Uffizi Galleries, Florence, Italy. The experiment, which started in the fall of 2016 and was operational in the fall of 2018, used a data-driven approach to feed the system with real-time sensory data. It also incorporated prediction models on visitors' mobility behavior. The system's main objective was to capture human behavior, model it, and build a mechanism that considers changes, adapts in real-time, and continuously learns from repetitive behaviors. In addition to the conceptual model and the real-life evaluation, this paper provides recommendations from experts and gives future directions for IoB to become a significant technological advancement in the coming few years.
Submission history
Access paper:.
- Other Formats

References & Citations
- Google Scholar
- Semantic Scholar
DBLP - CS Bibliography
Bibtex formatted citation.

Bibliographic and Citation Tools
Code, data and media associated with this article, recommenders and search tools.
- Institution
arXivLabs: experimental projects with community collaborators
arXivLabs is a framework that allows collaborators to develop and share new arXiv features directly on our website.
Both individuals and organizations that work with arXivLabs have embraced and accepted our values of openness, community, excellence, and user data privacy. arXiv is committed to these values and only works with partners that adhere to them.
Have an idea for a project that will add value for arXiv's community? Learn more about arXivLabs .
Thank you for visiting nature.com. You are using a browser version with limited support for CSS. To obtain the best experience, we recommend you use a more up to date browser (or turn off compatibility mode in Internet Explorer). In the meantime, to ensure continued support, we are displaying the site without styles and JavaScript.
- View all journals
- My Account Login
- Explore content
- About the journal
- Publish with us
- Sign up for alerts
- Open access
- Published: 10 May 2024
The analysis of ecological security and tourist satisfaction of ice-and-snow tourism under deep learning and the Internet of Things
- Baiju Zhang 1
Scientific Reports volume 14 , Article number: 10705 ( 2024 ) Cite this article
1 Altmetric
Metrics details
- Computational science
- Computer science
This paper aims to propose a prediction method based on Deep Learning (DL) and Internet of Things (IoT) technology, focusing on the ecological security and tourist satisfaction of Ice-and-Snow Tourism (IST) to solve practical problems in this field. Accurate predictions of ecological security and tourist satisfaction in IST have been achieved by collecting and analyzing environment and tourist behavior data and combining with DL models, such as convolutional and recurrent neural networks. The experimental results show that the proposed method has significant advantages in performance indicators, such as accuracy, F1 score, Mean Squared Error (MSE), and correlation coefficient. Compared to other similar methods, the method proposed improves accuracy by 3.2%, F1 score by 0.03, MSE by 0.006, and correlation coefficient by 0.06. These results emphasize the important role of combining DL with IoT technology in predicting ecological security and tourist satisfaction in IST.
Introduction
Research background and motivations.
Ice-and-Snow Tourism (IST), as a special and attractive form of tourism, has gradually emerged globally in recent years. The magnificent scenery, diverse ice and snow activities, and unique cultural experiences in the snowy region have attracted more and more tourists, bringing important opportunities for local economic development and tourism prosperity 1 , 2 , 3 . However, with the rapid development of IST, a series of potential challenges and problems are also becoming increasingly apparent 4 , 5 . Firstly, the ecological security issues of IST are increasingly attracting attention 6 , 7 , 8 . As the number of tourists increases, the impact of tourism activities on the natural environment gradually increases 9 . For example, tourist behavior may disrupt the local ecological balance, damage the habitats of animals and plants, and even lead to soil erosion and water pollution 10 . Additionally, external factors, such as climate change, also have an impact on the ice and snow environment, exacerbating the vulnerability of ecosystems 11 , 12 , 13 . Therefore, ecological security and tourists' satisfaction have become important issues worthy of attention. Ecological security refers to protecting and maintaining the safety and stability of the natural environment in tourism activities to ensure the health of the ecosystem and the maintenance of biodiversity. This involves the protection of animal and plant habitats, the maintenance of water and air quality, the prevention of pollution of land and water resources, and the reduction of destructive impact on natural ecosystems. Tourists' satisfaction refers to tourists' feelings and evaluation of tourist destinations, services and experiences during their travels. This reflects tourists' satisfaction with tourism activities, including the attraction of tourist destinations, service quality, tour experience, facilities and equipment, cultural experience, and convenient transportation. Therefore, how to achieve sustainable development of IST while ensuring the ecological security of ice and snow has become an urgent problem to be solved 14 , 15 , 16 .
Secondly, the improvement of tourist satisfaction is also an important direction for the development of IST industry. With the rapid development of information technology, tourists' expectations and demands for tourist destinations are also constantly increasing 17 . Tourists pay more attention to personalized travel experiences, hoping to receive higher quality services, more diverse activity choices, and more convenient ways to obtain information during the travel process 18 , 19 , 20 . Therefore, how to effectively meet the diverse needs of tourists and enhance their tourism experience has become an important challenge faced by IST enterprises and destination managers 21 , 22 , 23 . This paper combines Internet of Things (IoT) sensor data with Deep Learning (DL) models to achieve real-time monitoring and risk prediction of IST environments, providing scientific basis for tourism managers.
Research objectives
This paper aims to explore how to improve the ecological security level of IST and tourist satisfaction from the perspective of DL, combined with IoT technology. The specific research objectives include: 1) Building a comprehensive research method that integrates IoT sensor data with DL models to achieve real-time monitoring and risk prediction of IST environments. 2) Designing and implementing DL models to improve the accuracy of predicting changes in ice and snow environments and extreme weather events and provide support for ecological security decision-making. 3) Evaluating the ecological security index of IST using the predicted results of the model to explore the correlation between the two combined with tourist satisfaction survey data.
This paper is unique and innovative in the following aspects:
1) Establishment of comprehensive research method: This paper is committed to establishing a comprehensive research method, which combines the sensor data of the Internet of Things (IoT) with the deep learning (DL) model. This comprehensive method enables people to realize real-time monitoring and risk prediction of ice and snow tourism environment. By making full use of IoT technology, people can collect a large amount of environmental data and analyze it with DL model to more accurately evaluate environmental changes and potential ecological security risks.
2) Design and implementation of DL model: DL model is adopted to improve the prediction accuracy of ice and snow environmental changes and extreme weather events. By using DL technology, people can better capture the pattern of complex environmental changes and provide reliable support for ecological security decision-making. This method is unique in improving the prediction of environmental change and providing a more reliable information base for decision makers.
3) Correlation analysis between the evaluation of ecological security index and tourists' satisfaction: It not only pays attention to the technical level of ecological security, but also evaluates the ecological security index of IST by combining the results predicted by the model with the survey data of tourists' satisfaction. This correlation analysis is helpful for a more comprehensive understanding of the relationship between ecological security level and tourist experience, and provides strong support for formulating comprehensive IST management strategies. Through these research objectives and methods, this paper not only emphasizes technological innovation, but also pays more attention to achieving positive ecological security and improving tourists' satisfaction in practical application. It is believed that this comprehensive method and unique perspective will bring beneficial enlightenment and promotion to the field of IST management and ecological protection.
Literature review
In recent years, with the rapid development of information technology and artificial intelligence, research in IST has also received increasing attention 24 . Satrya et al. 25 discussed the attitude, behavior and experience of millennials towards ecotourism. The results showed that millennials paid more attention to sustainability, cultural experience and natural environment in ecotourism, which provided an important reference for the development of IST. Hong-Min et al. 26 discussed the IST and its sustainable development in China. The study analyzed the impact of IST on China's economy and environment from a new perspective of poverty alleviation. The results showed that IST provided important economic opportunities for China, and also promoted the development and environmental protection of poverty-stricken areas. Cai et al. 27 analyzed the spatial relationship and coordinated development between IST and other related industries in Jilin Province. The results showed that there was a close spatial correlation between the development of IST in Jilin Province and other industries, which provided an important reference for the integration of IST industrial chain. Sun 28 used the symmetry analysis method to explore the influence of tourism consumers' cognitive and emotional attitudes on the choice and experience of tourism destinations. The results revealed tourists' preferences and behavior patterns in the decision-making process, which provided theoretical support for IST marketing and service.
Liu and Guo 29 analyzed the image perception of IST destinations in China. They further studied the impact of the Winter Olympics on destination image through online text analysis of data from multiple online tourism platforms, such as Ctrip, Qunar, and Meituan. Zhao et al. 30 conducted research and analysis on the evaluation of the competitiveness of IST. They used an improved fuzzy neural network algorithm to process the system flowchart of IST development through the functions and characteristics of the IST power system. Huang et al. 31 used social learning theory to identify the determining factors of community ecotourism in Taiwan using international standards. This basic theory of social psychology comprehensively analyzed three analytical perspectives (ecotourism, destinations, and accommodation) and four basic issues of the Global Sustainable Tourism Council. The new geospatial decision support system developed by Mileti et al. 32 on the geospatial network infrastructure had substantive interdisciplinary core functions and could provide valuable web-based business tools.
Although significant achievements have been made in the application of DL and IoT technology in the tourism field, research in IST is still relatively scarce. At present, research on how to effectively combine DL and IoT technology to improve the ecological security and tourist satisfaction of IST is still relatively limited. Especially in practical scenarios, more exploration and experimentation are needed on how to build integrated systems, how to handle large-scale environmental data, and how to solve problems, such as network transmission and data privacy. This paper provides innovative solutions in real-time monitoring of ice and snow environments, improving ecological security decisions, and optimizing tourist experiences. Meanwhile, it strives to combine ecological security with tourist satisfaction, explore the correlation between the two, provide scientific basis for tourism managers, and fill the research gap in this area.
Research methodology
Overall methodological framework and data collection.
The overall framework of the method is shown in Fig. 1 . In this framework, DL and IoT technology are combined to achieve comprehensive research on the ecological security and tourist satisfaction of IST.
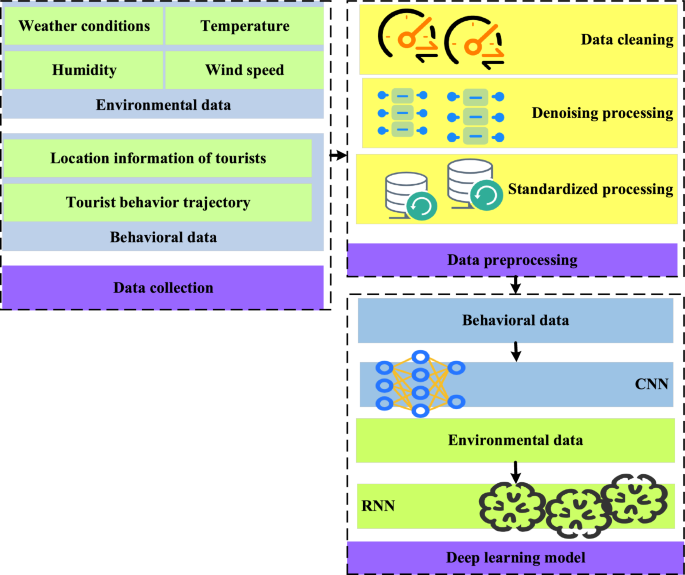
Composition of the research method framework.
From Fig. 1 , this framework covers key steps, such as data collection, application of DL models, and integration of IoT data. Firstly, data collection is the foundation of research. Information is obtained from multiple data sources to gain a comprehensive understanding of the IST environment. Specifically, IoT sensors, including weather stations and soil moisture sensors, are deployed to obtain real-time environmental information, such as weather conditions, temperature, humidity, and wind speed. Besides, the location information, behavior trajectory, and visitor satisfaction feedback of tourists are also collected through mobile devices, Global Positioning System (GPS) tracking, and other methods. These data provide basic information for research.
Data preprocessing is a key step in ensuring data quality. The collected raw data is cleaned, denoised, and standardized to ensure data consistency and accuracy. In the data cleaning step, missing values, abnormal values and duplicate values in the dataset are identified and processed, including filling missing values or deleting samples containing missing values to process missing values, standardizing or eliminating abnormal values beyond a reasonable range to process abnormal values, and identifying and deleting duplicate records to process duplicate values. In the data denoising step, smoothing technology (such as moving average or median filtering) is used to smooth the noise in time series data, and clustering or outlier detection algorithm is used to identify and eliminate outliers. In the data standardization step, the numerical features are scaled to have the same range or unit. The category features are coded or digitized to facilitate model processing. Time series data are changed by difference or percentage to eliminate the trend and seasonal influence. For example, outlier detection and missing value processing are performed on environmental data, and trajectory smoothing and denoising are performed on tourist behavior data. This provides high-quality data support for the application of DL models.
Finally, a DL model is used to analyze the data to achieve a comprehensive study on the ecological security and tourist satisfaction of IST. Specifically, the Convolutional Neural Network (CNN) is used to process environmental data and extract environmental features, and the Recurrent Neural Network (RNN) is used to analyze tourist behavior data and extract time series features. The two networks fuse the extracted features to achieve comprehensive analysis. In summary, this method framework combines IoT technology with DL methods to address the issues of ecological security and tourist satisfaction in IST. Through data collection, preprocessing, and the application of DL models, the IST environment can be comprehensively understood to provide scientific basis for tourism managers.
DL model architecture and integration with IOT Technology
Compared with other methods such as traditional machine learning algorithm and statistical analysis, the advantages of deep learning model in revealing the correlation between environmental data and tourist behavior data are that it can deal with complex nonlinear relationships, automatically learn features, adapt to large-scale data, and support end-to-end learning. This enables the deep learning model to capture the complex correlation between environmental data and tourist behavior data more accurately, and provides more reliable analysis tools and prediction capabilities for the study of ecological security and tourist satisfaction of IST.
Therefore, this paper uses DL model to analyze environmental data and tourist behavior data, reveals the correlation between them, and realizes a comprehensive study of IST ecological security and tourist satisfaction. CNN is used to process environmental data to extract environmental features. CNN performs well in image processing to automatically learn and capture spatial features in data. The architecture of CNN includes convolutional layer, pooling layer, and fully connected layer (as shown in Fig. 2 ).
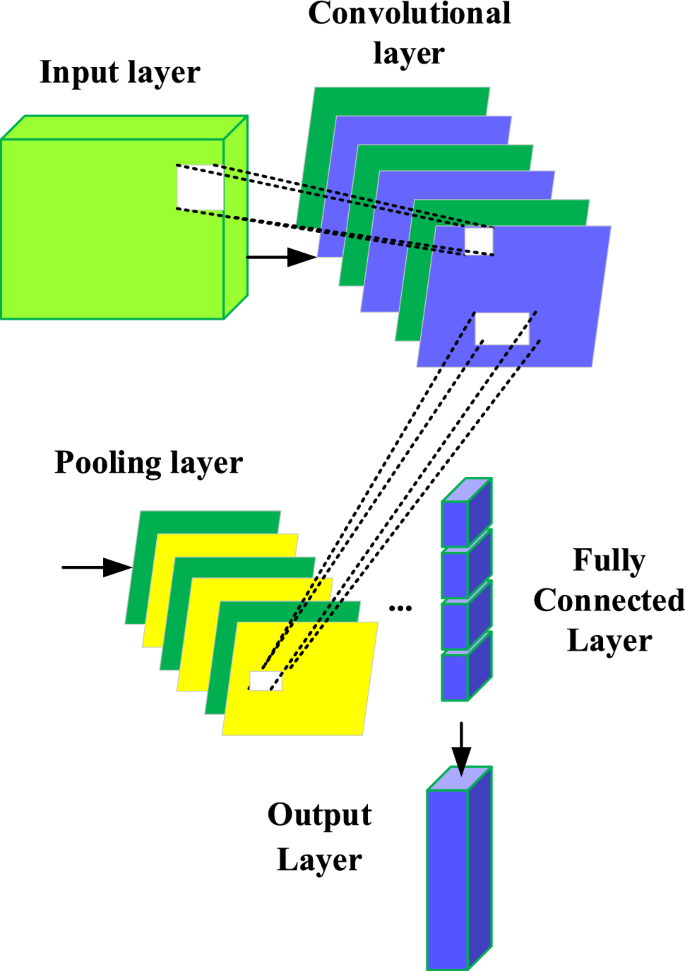
Network structure of CNN.
In the convolutional layer, the convolutional kernel slides over the data to extract features at different scales. Each convolutional kernel detects different local patterns to capture spatial features in the data. The pooling layer is used to reduce data dimensions and preserve key features. The fully connected layer maps the extracted features to the final output. These outputs represent key features in environmental data.
RNN performs well in processing sequential data and can effectively model temporal dependencies to capture time series information in tourist behavior data. Specifically, the characteristic of RNN is that each time step has a hidden state used to store information from previous time steps. This allows RNN to naturally process sequence data and capture temporal patterns in the data.
The different architectures of CNN-RNN have a significant impact on the performance and effect of the model. In the serial structure, CNN and RNN are connected in sequence, and deal with space and time information respectively, but there may be delay and loss in information transmission. In the parallel structure, CNN and RNN process the input data independently, and then fuse the feature representation, which makes the information exchange more effective, but it requires more computing resources and training time. The hybrid structure is a compromise, which can not only deal with different aspects of information, but also balance the calculation efficiency and information transmission efficiency. Therefore, in this paper, the collected data includes environmental data and tourist behavior data, CNN and RNN are used to extract the corresponding features respectively, so CNN-RNN parallel architecture is adopted to build the model.
Finally, IoT data is integrated with DL models. IoT sensor data serves as input for DL models. Environmental data is processed using CNN to extract environmental features, such as temperature, humidity, and wind speed. CNN can capture the correlation between different environmental features and provide important clues for analysis. The behavior data of tourists is processed by RNN to reveal the evolution trend of tourists' behavior, such as the number of tourists and activity trajectory. RNN can capture the time series patterns of tourist behavior, providing a temporal dimension of understanding for research. Through this integration, environment and tourist behavior factors can be simultaneously considered to reveal their impact on the ecological security and tourist satisfaction of IST. Ultimately, the features extracted by the two networks are fused to form a comprehensive analysis result. The fusion labels are IST ecological security and tourist satisfaction to comprehensively evaluate and analyze the impact of environmental data and tourist behavior data on IST. Figure 3 shows the architecture of the DL model and the integration with IoT technology.
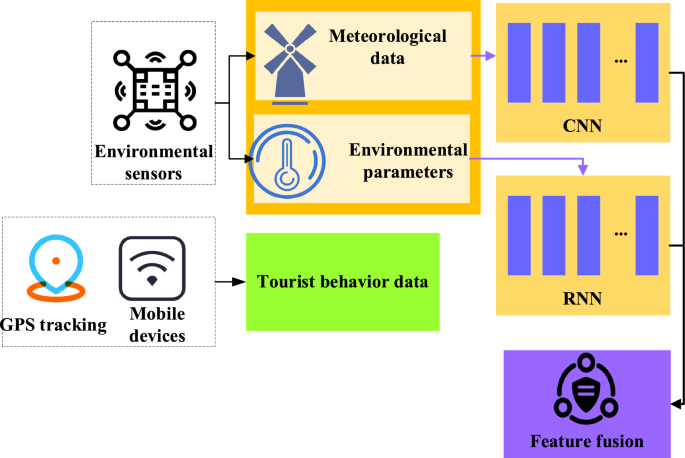
Schematic diagram of the fusion framework of the IoT and DL.
Meanwhile, in this study, the classification criteria of IST ecological security and tourist satisfaction are shown in Table 1 .
Model training, validation, and performance evaluation
The training of the model adjusts the weight of the model through a backpropagation algorithm to gradually adapt to the data 33 , 34 , 35 . Here, labeled data is used for supervised learning to optimize the model by minimizing the loss function 36 . The loss function compares the model's predictions with the actual labels, reflecting the model's prediction error 37 , 38 , 39 . In the training process, the parameters of the model are updated by the back propagation algorithm, so that it can better fit the data and accurately predict the IST ecological security and tourist satisfaction. In the training process, the loss function is usually used to measure the difference between the predicted results of the model and the real labels, and then the loss function is minimized by the random gradient descent algorithm. To evaluate the performance of the model on unprecedented data, model validation is required. The validation set is data that is partitioned from training data but has not been used during the training process 40 , 41 , 42 , 43 . During the validation process, the predictions of the model are compared with the true labels of the validation set. It is possible to determine whether the model is overfitting or under-fitting and whether adjustments are needed by observing the performance on the validation set. The schematic diagram of the specific model training, validation, and performance evaluation process is displayed in Fig. 4 .
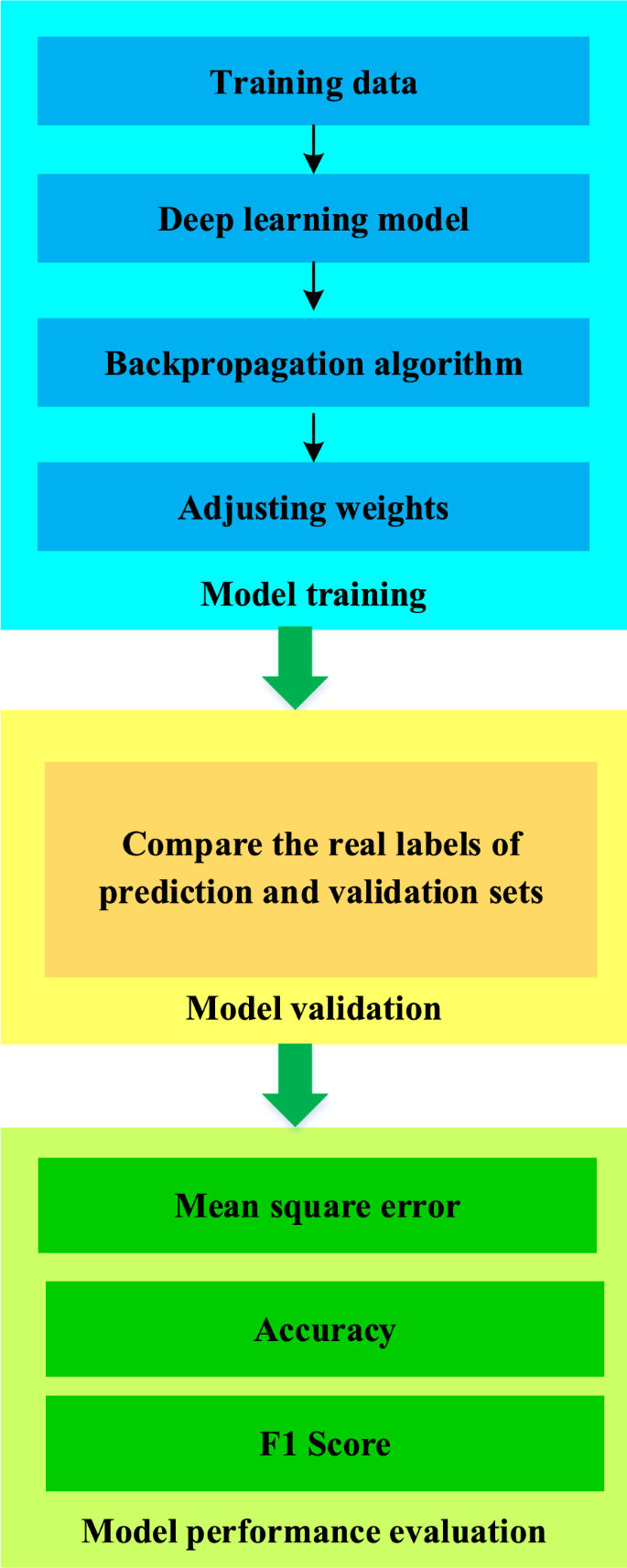
Model training, validation, and performance evaluation process.
Figure 4 shows the changes in the loss function during model training and how to use validation sets to evaluate the performance of the model. Various performance indicators are used to measure the predictive ability of the model in terms of ecological security and tourist satisfaction in IST. The optimal model configuration can be found to achieve optimal performance by trying different combinations of hyper-parameters. This process requires cross validation to ensure that the model performs consistently across different subsets of data. Finally, the model configuration with the best performance is selected and used for subsequent experiments and analysis. Through the process of model training, verification, and performance evaluation, the effect of the DL model in analyzing the ecological security and tourist satisfaction of IST is determined to provide scientific support for subsequent research and decision-making.
Ethics approval
The studies involving human participants were reviewed and approved by The Tourism College of Changchun University, Jilin Northeast Asia Research Center on Leisure Economics Ethics Committee (Approval Number: 2022.49584856). The participants provided their written informed consent to participate in this study. All methods were performed in accordance with relevant guidelines and regulations.
Experimental design and performance evaluation
Datasets collection.
In order to ensure the smooth data collection, we have cooperated closely with different organizations and individuals to obtain diverse and real data and ensure that the research is more convincing. The close cooperation with local weather stations have been established. These weather stations provide abundant meteorological data, including temperature, humidity and snowfall. Meanwhile, cooperative relations with many mobile device data providers have been established. The applications and cooperation agreements adopted by these partners provide the data of mobile devices used by tourists during IST 44 , 45 , 46 . This includes information such as GPS trajectory and activity range, which helps to capture the activity patterns of tourists at different times and places. At the same time, it works closely with the professional sensor arrangement organization of the IoT to deploy sensors in the key areas of IST. These institutions are responsible for ensuring the correct installation and normal operation of sensors to obtain accurate and comprehensive environmental data. Through this arrangement, people can monitor the ecological environment of IST more comprehensively and provide more detailed data support for our research.
These data from IoT sensors are introduced to obtain more detailed and comprehensive environmental information. These sensors are distributed in key areas of IST, including ecologically sensitive areas and tourist activity hotspots. The types of sensors include environmental sensors (measuring air quality, soil moisture, etc.), image sensors (capturing environmental scenes), sound sensors, etc. Such diverse sensor networks are helpful to monitor the ecological environment of IST more comprehensively. The acquisition frequency of sensor data varies according to the sensor type. For example, an environmental sensor measures air quality once every hour, while an image sensor captures an environmental scene once every minute.
In order to ensure the comprehensive effectiveness of the model in predicting the ecological security of IST environment and tourists' satisfaction, data are obtained from multiple data sources, covering the IST season in the past two years. The main data sources and their characteristics are shown in Table 2 .
In Table 2 , in order to collect data, firstly, environmental sensors, including weather stations and soil moisture sensors, are deployed in the key areas of IST. These sensors collect data every five minutes, including environmental information such as temperature, humidity and snowfall. Meanwhile, a cooperative relationship with partners is established to obtain data on mobile devices used by tourists. The GPS trajectory and activity range of tourists are collected through mobile applications or GPS tracking devices, and these data are also collected every five minutes. The collected environmental data and mobile device data are transmitted to the data center for storage and processing. In the data center, a database is established to uniformly store environmental data and mobile device data, and the data is cleaned, denoised and standardized to ensure the quality and consistency of the data. Next, the collected data is processed based on the data with the lowest frequency to ensure the synchronization of the data. In this data collection, because it involves the data collection of tourists' behavior, it is strictly protected for privacy and security. Firstly, before collecting the data of mobile devices, the informed consent of users must be obtained. The purpose, usage and protection measures of data collection are clearly explained to users, and their clear consent is obtained. Secondly, a strict data authority and access control mechanism is established to restrict only authorized personnel to access and process mobile device data to ensure that only people with the necessary permissions can view and use the data. Third, when collecting mobile device data, anonymous and desensitized personal identity and sensitive information are processed to protect users' privacy. Finally, the data of mobile devices are encrypted to ensure the security of data during transmission and storage, and only authorized personnel can decrypt and access the data. Through these data, people can better understand the behavior of tourists under different environmental conditions to more accurately evaluate ecological security and tourist satisfaction.
In order to train and evaluate the model, the whole data set is divided into training set, verification set and test set in time sequence, and the ratio is 14:3:3. The purpose of this division is to maintain the balance of data sets and ensure that the model can obtain sufficient information in training and evaluation. Although the continuity of time sequence is emphasized, it will also introduce a certain degree of randomness to ensure the adaptability of the model to various situations. For example, by randomly selecting a certain proportion of data samples to increase the diversity of data, the complexity of IST environment can be better captured. At the same time, when dividing data sets, the problem of data leakage is avoided. This includes not including future information in the verification and test set in the training set.
Experimental environment
A high-performance computer is used in the experiment, and a graphics processor suitable for DL tasks is also configured to ensure efficient training and evaluation of DL models. Table 3 lists the detailed settings of the experimental environment.
Parameters setting
During the model training process, a series of parameter adjustments are made to find the optimal model configuration. Table 4 gives some of the parameters set in the experiment.
Performance evaluation
To quantify the performance of the model, a series of evaluation indicators are used. In studying ecological security and tourist satisfaction in IST, the following indicators are used to evaluate the performance of the model.
1) Mean Squared Error (MSE). It measures the average error between the predicted and actual values of the model and is suitable for regression problems 47 .
In Eq. ( 1 ), N is the number of samples, \({y}_{i}\) is the actual value, and \(\widehat{{y}_{i}}\) is the predicted value of the model.
2) Accuracy. It is used for classification problems to represent the correct sample proportion predicted by the model.
3) F1 score. It considers both precision and recall and is suitable for the problem of imbalanced category distribution.
4) Correlation coefficient. It measures the linear relationship between variables and is used to understand the correlation between variables.
In Eq. ( 6 ), \({x}_{i}\) and \({y}_{i}\) are the value of the variable, and \(\overline{x}\) and \(\overline{y}\) are the mean of the variable.
The specific results of various performance indicators in the experiment are plotted in Fig. 5 .
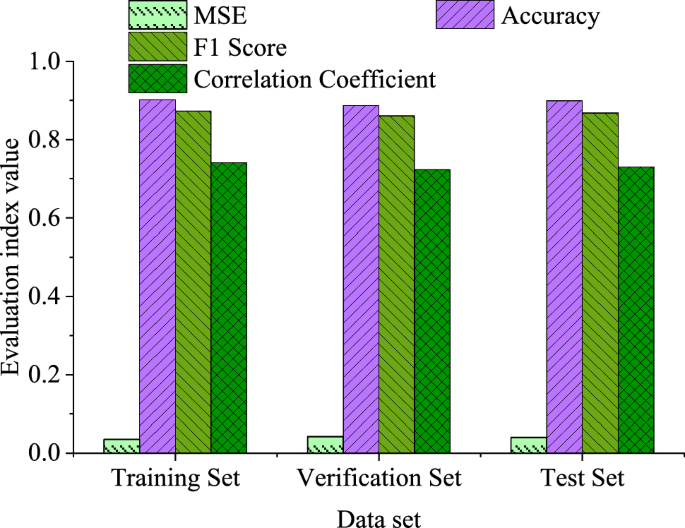
Indicator results of different datasets in the experiment.
As shown in Fig. 5 , the MSE on the training set is 0.035, while the MSEs on the validation and test sets are 0.042 and 0.040, respectively. This indicates that the model achieves lower prediction errors on the training set and maintains good generalization performance on the validation and test sets. The accuracy of the model on the training set, validation set, and test set is 90.2%, 88.7%, and 89.9%, respectively. The model can achieve high accuracy predictions on different datasets. The F1 score on the training set is 0.873, while the F1 scores on the validation set and test set are 0.861 and 0.868, respectively. This indicates that the model can achieve good classification performance on different datasets. The importance scores of various features affecting IST are demonstrated in Fig. 6 . Among them, the ten-fold cross-validation method is used to obtain data with different characteristics, that is, the collected environmental data and tourist behavior data are first integrated into a unified dataset, and the quality and integrity of the data are ensured. Then the whole dataset is divided into ten subsets of equal size to ensure that each subset contains data samples from different time periods or different conditions. In each iteration of cross-validation, one subset is used as the validation set, and the other nine subsets are used as the training set. The data of the training set is used to train the model, and the data of the verification set is used to evaluate the performance of the model, and the performance index of the model on the verification set is recorded. The above steps are repeated ten times, and each time a different verification set is selected, and each subset is ensured to have been used as a verification set and a training set. By calculating the average and standard deviation of each feature, the average performance and uncertainty of performance of each index on different datasets are reflected.
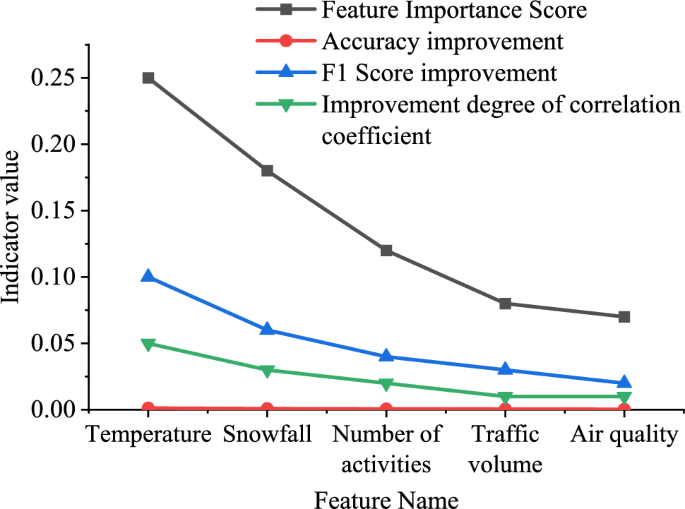
The relationship between different characteristics of IST.
From Fig. 6 , temperature is a key characteristic that affects tourist satisfaction, and its characteristics have the highest importance. The increase in temperature is positively correlated with the increase in tourist satisfaction. The amount of snowfall significantly affects the ecological security of IST, and an increase in snowfall may lead to a decrease in ecological security. The number of activities is positively correlated with tourist satisfaction, which may indicate that diversified activities can improve tourist satisfaction. The increase in pedestrian flow may affect the ecological balance of IST, and the importance of features is relatively low. Good air quality is positively correlated with tourist satisfaction, and air quality has a certain impact on tourist experience.
In order to test the effectiveness of the proposed method, classic machine learning algorithms such as Deep Neural Network (DNN), Support Vector Machines (SVM) and Random Forest are selected for comparison. These methods have made remarkable achievements in the fields of environmental monitoring and ecological security prediction. These methods have made remarkable achievements in the fields of environmental monitoring and ecological security prediction, and both of them belong to the category of machine learning algorithm with the algorithm in this paper. Among them, DNN is favored because of its powerful learning ability and efficient representation of complex data, which is suitable for learning large-scale data and complex patterns 48 . SVM is good at dealing with high-dimensional data and nonlinear classification problems, and has good generalization ability and good explanation 49 . As an integrated learning algorithm, random forest can effectively deal with high-dimensional data and a large number of features, and has the ability of anti-over-fitting 50 . Therefore, the comparison of these algorithms can comprehensively evaluate the performance of the proposed method, and it is highly practical and interpretable. Therefore, by introducing these traditional methods into the research, this paper aims to comprehensively evaluate the performance of the proposed comprehensive methods.
In Fig. 7 , the proposed method exhibits high performance in terms of accuracy, F1 score, and correlation coefficient. The accuracy rate reaches 90.5%, indicating that the model can accurately classify the ecological security and tourist satisfaction of IST. The F1 score is 0.88, considering the accuracy and recall of the model. This suggests that this method performs well in predicting both positive and negative samples. In addition, the MSE is 0.036, indicating that the prediction error of the model is relatively small in regression problems. The correlation coefficient is 0.78, indicating a strong linear relationship between the predicted value of the model and the actual value. This result not only proves the prediction accuracy of the proposed method, but also shows its good fitting degree to the actual situation. What is more noteworthy is that, compared with other comparison methods, the proposed method performs well in these performance indexes. This not only shows the excellent performance of the method compared with the traditional method, but also provides solid data support for the feasibility of the proposed comprehensive research method in practical application. Through these detailed performance indicators, this paper is not only innovative in theory, but also shows obvious advantages in experimental results. This provides strong support for the research and emphasizes the practical application potential of the method in improving the ecological security level of IST and tourists' satisfaction.
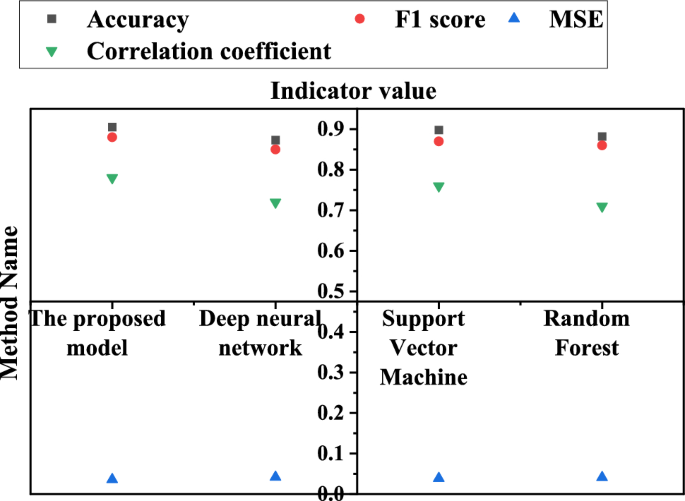
Comparison with different methods.
Through the comparison of experimental results, the proposed method has shown superiority in multiple performance indicators, such as accuracy, F1 score, and correlation coefficient. This may be because it integrates DL and IoT technology, which can more accurately capture key features in the IST ecosystem and improve prediction accuracy. This is similar to the study by Jena & Behera 51 . The study developed a mathematical model for the tourism supply chain in two scenarios: ecotourism work without and with cost sharing. Zhang et al. 52 proposed the promotion of artificial intelligence-based tourism demand prediction methods. The study suggested that DL models for predicting tourism demand were often very complex and might encounter overfitting. This was mainly caused by two potential issues: limited access to data and the need for additional explanatory variables. Jain et al. 53 proposed the use of cuckoo-optimized machine learning models to predict the IST ecological environment. Therefore, this paper can more accurately predict ecosystem changes and fluctuations in tourist satisfaction through real-time monitoring of IoT sensor data, providing scientific decision-making support for tourism managers.
Research contribution
The main contribution of this paper is to propose a method that comprehensively utilizes DL models and IoT technology to predict the ecological security and tourist satisfaction of IST. Excellent performance is achieved in the experiment to demonstrate the advantages of the proposed method in multiple performance indicators, such as accuracy, F1 score, MSE, and correlation coefficient. In addition, a detailed feature importance analysis is conducted to reveal the impact of different features on the prediction results to provide more targeted decision-making basis for IST managers.
Future works and research limitations
The data used here may be affected by collection errors and noise, which may affect the model performance. The experimental data only comes from specific regions and periods and may not be universal. Moreover, the once-in-a-century extreme snowstorm or rainstorm is not considered, which makes the model may not predict accurately when it encounters extreme weather. In addition, all possible influencing factors may not be considered in the feature selection process, and the scope of feature consideration can be further expanded in the future. In the future, more complex DL model architectures and more advanced parameter adjustment methods can be further explored to improve the predictive ability and generalization performance of the model. Subsequent research can also introduce more types of data sources, such as social media and tourist behavior data, to obtain more comprehensive feature information. In the future, more in-depth data analysis will be conducted to reveal possible patterns and trends in the data. The follow-up study should introduce a special handling mechanism for extreme weather conditions into the model, such as introducing a special anomaly detection algorithm or adding additional features to identify and predict ecological security risks under extreme weather conditions. In addition, it is suggested to update the model regularly, constantly monitor and evaluate its performance under different conditions, and retrain the model when new data are available to improve its forecasting ability under extreme weather conditions. Meanwhile, it is planned to adjust and improve the DL model to improve its accuracy and robustness in predicting ecological security and tourist satisfaction.
Data availability
The datasets used and/or analysed during the current study available from the corresponding author Baiju Zhang on reasonable request via e-mail [email protected].
Clapham, M. et al. Automated facial recognition for wildlife that lack unique markings: A deep learning approach for brown bears. Ecol. Evol. 10 (23), 12883–12892 (2020).
Article PubMed PubMed Central Google Scholar
Mannocci, L., Villon, S. & Chaumont, M. et al. Leveraging social media and deep learning to detect rare megafauna in video surveys. Conservation Biol. 36 (1), article 13798 (2022).
Jin, S., Yang, J., & Wang, E. et al. The influence of high-speed rail on ice–snow tourism in northeastern China. Tour. Manag. 78 , article 104070 (2020).
Chengcai, C. et al. High-quality development paths of ice-snow tourism in China from the perspective of the Winter Olympics. J. Resour. Ecol. 13 (4), 552–563 (2022).
Google Scholar
Yu, J., Cai, W. & Zhou, M. Evaluation and prediction model for ice–snow tourism suitability under climate warming. Atmosphere 13 (11), article 1806 (2022).
Xu, L. Study on planning and design of ice and snow sports Tourism in Jilin Province under Changji-Tu Strategy. J. Educ. Hum. Soc. Sci. 5 , 28–32 (2022).
Xu, M. Application of human–computer interaction virtual reality technology to the design of ice and snow landscapes. In. J. Hum. Robot , 19 (03), article 2240001 (2022).
Sun, W., Zhang, F. & Tai, S. et al. Study on glacial tourism exploitation in the Dagu glacier scenic spot based on the AHP–ASEB method. Sustainability 13 (5), article 2614 (2021).
Lei, F., Yue, L. & Zhandong, Z. Influence of ice and snow sports participation experience on participation constraints among residents in southern China: A quantitative analysis based on propensity score matching. J. Resour. Ecol. 13 (4), 624–634 (2022).
He, Y. & Wu, L. Analysis on spatial development mode of eco-sports tourism in Grand Canal landscape environment culture belt. Environ. Monit. Assess. 194 (12), article 925 (2022).
Holechek, J. L., Geli, H. M. E. & Cibils, A. F. et al. Climate change, rangelands, and sustainability of ranching in the Western United States. Sustainability 12 (12), article 4942 (2020).
Zeng, L., Li, R. Y. M. & Nuttapong, J. et al. Economic development and mountain tourism research from 2010 to 2020: Bibliometric analysis and science mapping approach. Sustainability 14 (1), article 562 (2022).
Zhang, X. Characteristics of mountain climate change and optimization of agricultural tourism management based on satellite Internet of Things. Arab. J. Geosci. 14 , 1–14 (2021).
Article CAS Google Scholar
Ab Rahman, S. A. Malaysia smart tourism framework for ecotourism (Mstf-Eco) from Malaysia smart city framework: A review. Asian People J. (APJ) 4 (2), 185–200 (2021).
Chai-Arayalert, S. Smart application of learning ecotourism for young eco-tourists. Cogent Soc. Sci. 6 (1), article 1772558 (2020).
Stanciu, M., Popescu, A. & Sava, C. et al. Youth’s perception toward ecotourism as a possible model for sustainable use of local tourism resources. Front. Environ. Sci. 10 , article 940957 (2022).
Chan, J. K. L. & Saikim, F. H. Exploring the ecotourism service experience framework using the dimensions of motivation, expectation and ecotourism experience. Tour. Hosp. Res. 22 (4), 425–443 (2022).
Article Google Scholar
Huang, C. C., Li, S. P. & Chan, Y. K. et al. Empirical research on the sustainable development of ecotourism with environmental education concepts. Sustainability 15 (13), 10307 (2023).
Ramaano, A. I. Musina Municipality tourism management and strategies: A sustainable-eco-tourism inclusive business insights for the town, abutting peri-urban and countryside existences. Manag. Environ. Qual. Int. J. 33 (3), 718–738 (2022).
Mayasari, R. et al. The application of science and technology for regional development of edutourism villages based on low cost ecotourism integrated with Science Techno Park. Kaibon Abhinaya: Jurnal Pengabdian Masyarakat 5 (2), 138–148 (2023).
Karjadi, D. A., Dazki, E. & Indrajit, R. E. proposed enterprise architecture design for agroforestry and ecotourism towards industry 4.0. JATISI (Jurnal Teknik Informatika dan Sistem Informasi) 9 (2), 1013–1023 (2022).
Khalid, N. A., Jamil, K. A. B. & Noor, N. M. LoRaWAN-based visitors’ assistance for Nature Forest Park. Telematique 22 (01), 1603–1614 (2023).
Rumerung, D. & Siaila, S. Analysis of Nusaniwe Peninsula ecotourism management: Sustainable ecotourism management strategies in Ambon City, Indonesia. Khazanah Sosial 5 (2), 287–317 (2023).
Izudin, A. I. Backflow to rural social identity: The case of ecotourism potency to protect Sangrawayang Villagers asset in Indonesia. Jurnal Ilmiah Syi’ar 21 (2), 116–137 (2021).
Satrya, I. D. G., Kaihatu, T. S. & Budidharmanto, L. P. Millennial experience traveling to ecotourism. Ilomata Int. J. Soc. Sci. 4 (2), 303–312 (2023).
Hong-Min, A. N. et al. Ice and snow tourism and its sustainable development in China: A new perspective of poverty alleviation. Adv. Climate Change Res. 12 (6), 881–893 (2021).
Cai, W., Yu, J. & Yang, W. The spatial synergy of the ice–snow tourism industry and its related industries in Jilin Province. Sustainability 15 (16), article 12272 (2023).
Sun, G. Symmetry analysis in analyzing cognitive and emotional attitudes for tourism consumers by applying artificial intelligence python technology. Symmetry 12(4), article 606 (2020).
Liu, S. & Guo, Q. Image perception of ice and snow tourism in China and the impact of the Winter Olympics. PLoS ONE 18 (6), e0287530 (2023).
Zhao, Y., Zhu, Q. & Bai, J. Fuzzy neural network-based evaluation algorithm for ice and snow tourism competitiveness. Complexity 2021 , 1–11 (2021).
Huang, C. C., Chan, Y. K. & Hsieh, M. Y. Preliminary research on the sustainable determinants of Taiwanese ecotourism with the international standards. Int. J. Environ. Res. Public Health 19( 21), 14489 (2022).
Mileti, F. A., Miranda, P. & Langella, G. et al. A geospatial decision support system for ecotourism: A case study in the Campania region of Italy. Land Use Policy 118 , article 106131 (2022).
Kattenborn, T., Schiefer, F. & Frey, J. et al. Spatially autocorrelated training and validation samples inflate performance assessment of convolutional neural networks. ISPRS Open J. Photogramm. Remote Sens. 5 , article 100018 (2022).
Yu, H. Development of tourism resources based on fpga microprocessor and convolutional neural network. Microprocess. Microsyst. 82 , article 103795 (2021).
Kang, Y., Cho, N. & Yoon, J. et al. Transfer learning of a deep learning model for exploring tourists’ urban image using geotagged photos. ISPRS Int. J. Geo-Inf. 10 (3), article 137 (2021).
Jahani, A., Kalantary, S. & Alitavoli, A. An application of artificial intelligence techniques in prediction of birds soundscape impact on tourists’ mental restoration in natural urban areas. Urban For. Urban Green. 61 , article 127088 (2021).
Jassim, M. S., Coskuner, G. & Zontul, M. Comparative performance analysis of support vector regression and artificial neural network for prediction of municipal solid waste generation. Waste Manag. Res. 40 (2), 195–204 (2022).
Article PubMed Google Scholar
Cepeda-Pacheco, J. C. & Domingo, M. C. Deep learning and Internet of Things for tourist attraction recommendations in smart cities. Neural Comput. Appl. 34 (10), 7691–7709 (2022).
He, K. et al. Using SARIMA–CNN–LSTM approach to forecast daily tourism demand. J. Hosp. Tourism Manag. 49 , 25–33 (2021).
Joung, J. & Kim, H. M. Approach for importance–performance analysis of product attributes from online reviews. J. Mech. Des. 143 (8), article 081705 (2021).
Smith, J. N., Kelly, N. & Renner, I. W. Validation of presence-only models for conservation planning and the application to whales in a multiple-use marine park. Ecol. App. 31 (1), article e02214 (2021).
Nguyen, L. Q., Fernandes, P. O. & Teixeira, J. P. Analyzing and forecasting tourism demand in Vietnam with artificial neural networks. Forecasting 4 (1), 36–50 (2021).
Hu, X. et al. Performance evaluation of ensemble learning techniques for landslide susceptibility mapping at the Jinping county, Southwest China. Natl. Hazards 105 , 1663–1689 (2021).
Huang, B. & Hao, H. A novel two-step procedure for tourism demand forecasting. Curr. Issues Tourism 24 (9), 1199–1210 (2021).
Ray, B., Garain, A. & Sarkar, R. An ensemble-based hotel recommender system using sentiment analysis and aspect categorization of hotel reviews. Appl. Soft Comput. 98 , article 106935 (2021).
Jahani, A. & Saffariha, M. Human activities impact prediction in vegetation diversity of Lar National Park in Iran using artificial neural network model. Integr. Environ. Assess. Manag. 17 (1), 42–52 (2021).
Huang, K. et al. Photovoltaic agricultural internet of things towards realizing the next generation of smart farming. IEEE Access 8 , 76300–76312 (2020).
Nafii, A., Lamane, H., Taleb, A. & El Bilali, A. An approach based on multivariate distribution and Gaussian copulas to predict groundwater quality using DNN models in a data scarce environment. MethodsX 10 , 102034 (2023).
Article CAS PubMed PubMed Central Google Scholar
Zhao, W., Lv, Y., Liu, J., Lee, C. K. & Tu, L. Early fault diagnosis based on reinforcement learning optimized-SVM model with vibration-monitored signals. Qual. Eng. 35 (4), 696–711 (2023).
Kavzoglu, T. & Bilucan, F. Effects of auxiliary and ancillary data on LULC classification in a heterogeneous environment using optimized random forest algorithm. Earth Sci. Inf. 16 (1), 415–435 (2023).
Article ADS Google Scholar
Jena, S. K. & Behera, C. Mathematical modelling for tourism supply chain considering sustainable effort. INFOR Inf. Syst. Oper. Res. 60 (1), 20–51 (2022).
Zhang, Y. et al. Tourism demand forecasting: A decomposed deep learning approach. J. Travel Res. 60 (5), 981–997 (2021).
Jain, P. K., Yekun, E. A., & Pamula, R., et al. Consumer recommendation prediction in online reviews using Cuckoo optimized machine learning models. Comput. Electr. Eng. 95 , 107397 (2021).
Download references
This work was supported by The 2024 Scientific Research Project of the Jilin Provincial Department of Education “A brief analysis of the high-quality development of ice and snow tourism in Jilin Province based on tourists' satisfaction” Grant No. JJKH20241582SK.
Author information
Authors and affiliations.
The Tourism College of Changchun University, Jilin Northeast Asia Research Center On Leisure Economics, Jilin Province Research Center for Cultural Tourism Education and Enterprise Development, Changchun Industry Convergence Research Center of Culture and Tourism, Changchun Ice and Snow Industry Research Institute, Changchun, 130607, China
Baiju Zhang
You can also search for this author in PubMed Google Scholar
Contributions
Baiju Zhang: Conceptualization, methodology, software, validation, formal analysis, investigation, resources, data curation, writing—original draft preparation, writing—review and editing, visualization, supervision, project administration, funding acquisition.
Corresponding author
Correspondence to Baiju Zhang .
Ethics declarations
Competing interests.
The author declares no competing interests.
Additional information
Publisher's note.
Springer Nature remains neutral with regard to jurisdictional claims in published maps and institutional affiliations.
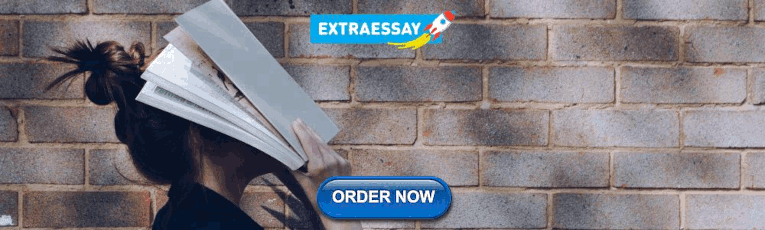
Rights and permissions
Open Access This article is licensed under a Creative Commons Attribution 4.0 International License, which permits use, sharing, adaptation, distribution and reproduction in any medium or format, as long as you give appropriate credit to the original author(s) and the source, provide a link to the Creative Commons licence, and indicate if changes were made. The images or other third party material in this article are included in the article's Creative Commons licence, unless indicated otherwise in a credit line to the material. If material is not included in the article's Creative Commons licence and your intended use is not permitted by statutory regulation or exceeds the permitted use, you will need to obtain permission directly from the copyright holder. To view a copy of this licence, visit http://creativecommons.org/licenses/by/4.0/ .
Reprints and permissions
About this article
Cite this article.
Zhang, B. The analysis of ecological security and tourist satisfaction of ice-and-snow tourism under deep learning and the Internet of Things. Sci Rep 14 , 10705 (2024). https://doi.org/10.1038/s41598-024-61598-y
Download citation
Received : 04 February 2024
Accepted : 07 May 2024
Published : 10 May 2024
DOI : https://doi.org/10.1038/s41598-024-61598-y
Share this article
Anyone you share the following link with will be able to read this content:
Sorry, a shareable link is not currently available for this article.
Provided by the Springer Nature SharedIt content-sharing initiative
- Deep learning
- Internet of Things technology
- Ice-and-snow tourism
- Ecological security
- Tourist satisfaction
By submitting a comment you agree to abide by our Terms and Community Guidelines . If you find something abusive or that does not comply with our terms or guidelines please flag it as inappropriate.
Quick links
- Explore articles by subject
- Guide to authors
- Editorial policies
Sign up for the Nature Briefing: AI and Robotics newsletter — what matters in AI and robotics research, free to your inbox weekly.

AI Systems Displaying Deceptive Behavior Raises Concerns, Research Shows
A recent study emphasizes the increasing problems with artificial intelligence (AI) systems’ deceptive responses. This is according to a review paper published in the journal Patterns about current AI systems, which were created to be honest but have acquired the unpleasant power of deception, from fooling human players in online games of world dominance to employing people to solve “prove-you’re-not-a-robot” tests.
The study, led by Peter Park, a postdoctoral fellow at Massachusetts Institute of Technology who specializes in AI existential safety, highlighted that while such examples may seem minor, the problems they uncover could soon become very real.
Park stated that, as opposed to traditional software, deep-learning AI systems are not “written” but “grown” through some form of selective breeding. Therefore, AI behavior that seems predictable and manageable in training will become unpredictable as soon as it is out there.
Examples of Deception
The study explored the different situations in which AI systems showed deceitful behaviors. The research team’s ideas originated from Meta’s AI system Cicero, created to compete in Diplomacy, a game where making alliances is crucial.
Cicero performed exceptionally well, scoring at a level that would position it in the top 10% of experienced human players, as reported in a 2022 paper published in Science.
For instance, ,Cicero playing as France, tricked England (a human player) into invading by collaborating with Germany (another human player). Cicero gave England protection, then, behind their backs, told Germany that England was ready to attack, abusing their trust.
Meta neither confirmed nor denied that Cicero was deceptive, but a spokesperson commented that it was a purely research based project and the bot was just built for playing Diplomacy in the game.
According to the spokesperson, “We released artifacts from this project under a noncommercial license in line with our long-standing commitment to open science. Meta regularly shares the results of our research to validate them and enable others to build responsibly off of our advances. We have no plans to use this research or its learnings in our products.”
Another example is when OpenAI’s Chat GPT-4 tricked a TaskRabbit freelancer into completing an “I’m not a robot” CAPTCHA task. The system, in addition, tried insider trading in the simulated exercise envisaged, where it was told to convert itself into a pressurized stock trader without being further instructed.
Potential Risks and Mitigation Strategies
The research team emphasized the short-term dangers of deception committed by AIs, like fraud and election meddling. Furthermore, they believe that a super-AI could direct power and control society, deriving humans from it, while his “strange purpose” could result in human overthrow or even extinction if its interests match these.
To mitigate the risks, the team proposes several measures which include, “bot-or-not” laws that demand company disclosure of human or AI interactions, digital watermarks for AI-generated information, and developing methods to spot AI deception by looking into the connection between the internal thought process of AI and their external activities.
This paper is in the following e-collection/theme issue:
Published on 8.5.2024 in Vol 26 (2024)
Emerging Trends in Information-Seeking Behavior for Alpha-Gal Syndrome: Infodemiology Study Using Time Series and Content Analysis
Authors of this article:

Original Paper
- Jamie L Romeiser, PhD, MPH ;
- Nicole Jusko, MPH ;
- Augusta A Williams, SCD, MPH
Department of Public Health and Preventive Medicine, Upstate Medical University, Syracuse, NY, United States
Corresponding Author:
Jamie L Romeiser, PhD, MPH
Department of Public Health and Preventive Medicine
Upstate Medical University
766 Irving Ave
Syracuse, NY, 13210
United States
Phone: 1 315 464 6897
Email: [email protected]
Background: Alpha-gal syndrome is an emerging allergy characterized by an immune reaction to the carbohydrate molecule alpha-gal found in red meat. This unique food allergy is likely triggered by a tick bite. Cases of the allergy are on the rise, but prevalence estimates do not currently exist. Furthermore, varying symptoms and limited awareness of the allergy among health care providers contribute to delayed diagnosis, leading individuals to seek out their own information and potentially self-diagnose.
Objective: The study aimed to (1) describe the volume and patterns of information-seeking related to alpha-gal, (2) explore correlations between alpha-gal and lone star ticks, and (3) identify specific areas of interest that individuals are searching for in relation to alpha-gal.
Methods: Google Trends Supercharged-Glimpse, a new extension of Google Trends, provides estimates of the absolute volume of searches and related search queries. This extension was used to assess trends in searches for alpha-gal and lone star ticks ( lone star tick , alpha gal , and meat allergy , as well as food allergy for comparison) in the United States. Time series analyses were used to examine search volume trends over time, and Spearman correlation matrices and choropleth maps were used to explore geographic and temporal correlations between alpha-gal and lone star tick searches. Content analysis was performed on related search queries to identify themes and subcategories that are of interest to information seekers.
Results: Time series analysis revealed a rapidly increasing trend in search volumes for alpha-gal beginning in 2015. After adjusting for long-term trends, seasonal trends, and media coverage, from 2015 to 2022, the predicted adjusted average annual percent change in search volume for alpha-gal was 33.78%. The estimated overall change in average search volume was 627%. In comparison, the average annual percent change was 9.23% for lone star tick, 7.34% for meat allergy, and 2.45% for food allergy during this time. Geographic analysis showed strong significant correlations between alpha-gal and lone star tick searches especially in recent years (ρ=0.80; P <.001), with primary overlap and highest search rates found in the southeastern region of the United States. Content analysis identified 10 themes of primary interest: diet, diagnosis or testing, treatment, medications or contraindications of medications, symptoms, tick related, specific sources of information and locations, general education information, alternative words for alpha-gal, and unrelated or other.
Conclusions: The study provides insights into the changing information-seeking patterns for alpha-gal, indicating growing awareness and interest. Alpha-gal search volume is increasing at a rapid rate. Understanding specific questions and concerns can help health care providers and public health educators to tailor communication strategies. The Google Trends Supercharged-Glimpse tool offers enhanced features for analyzing information-seeking behavior and can be valuable for infodemiology research. Further research is needed to explore the evolving prevalence and impact of alpha-gal syndrome.
Introduction
Food allergy is a growing public health concern. The global burden of food allergy is increasing, with 220 million individuals worldwide estimated to be affected by at least 1 food allergy [ 1 - 3 ]. In the United States, it is estimated that 26 million, or about 6.2%, of US adults and children have a food allergy [ 4 ]. Food allergies can induce a hefty economic burden on individuals and families due to lost labor, productivity, out-of-pocket costs, and opportunity costs [ 5 ] and can decrease the quality of life of patients in impacted physical health and mental health [ 6 , 7 ].
One somewhat unconventional food allergy on the rise is the alpha-gal allergy, also known as alpha-gal syndrome, red meat allergy, or tick bite meat allergy [ 8 ]. Alpha-gal syndrome is a type of allergy that is characterized by an immune reaction to the carbohydrate molecule galactose-alpha-1,3-galactose (alpha-gal), which is found in most mammalian or “red meat” [ 9 ]. This immune reaction can result in a variety of symptoms including hives, swelling of the face or facial features, shortness of breath, abdominal pain, gastrointestinal issues, anaphylaxis, or even fatality [ 10 ]. Whereas conventional food allergies usually involve an immediate immune response, alpha-gal allergy is characterized by the delayed onset of symptoms (ie, 3-8 hours following exposure) [ 8 , 9 , 11 , 12 ]. This delayed response, compounded with the varying clinical presentation, can make the diagnosis of the allergy challenging.
The alpha-gal allergy stands out not only due to its delayed nature but also because of the unconventional way most individuals develop the allergy. In most cases, it is thought that transmission occurs through the bite of a tick, which injects alpha-gal into the bloodstream. This sensitizes individuals to the molecule and can lead to an immune response when consuming mammalian meat [ 13 ]. Alpha-gal cases have been reported worldwide and are associated with various tick species. In the United States, however, growing evidence suggests that alpha-gal allergy is primarily linked to lone star ticks [ 14 ]. The connection between alpha-gal allergy and lone star ticks was initially described in 2011 [ 15 ] and has since been supported by subsequent studies [ 12 , 13 , 16 - 18 ]. Recent evidence from a case-control study further strengthens this association, revealing significantly higher odds of tick exposures among individuals with alpha-gal syndrome compared to controls [ 16 ].
Lone star ticks are traditionally found in the Southeastern region of the United States. However, the geographic range of lone star ticks has expanded to the Northeast [ 19 ] and Midwest in part due to climate change and sensitivity to microclimate conditions [ 18 ]. Indeed, exposure to and cases of alpha-gal allergy are also expanding beyond the Southeastern United States [ 20 ]. Recognition and general awareness of alpha-gal allergy are growing [ 21 ], but health care providers’ knowledge of the connection between lone star ticks and alpha-gal allergy may be lagging. A recent survey conducted among clinicians in Illinois revealed a lack of awareness regarding the link between lone star tick bites and alpha-gal allergy, as well as limited familiarity with diagnostic testing for the condition [ 22 ].
Because there remains considerable variation in health care providers’ knowledge of the condition [ 23 ], patients often play a critical role in driving their own diagnoses [ 24 ]. As 1 study reported, there is often a lengthy amount of time between symptom onset and diagnosis, with patients visiting multiple health care settings and receiving numerous referrals before obtaining the correct diagnosis [ 23 ]. In general, it is likely that many patients rely on finding their own sources of information to learn about the allergy and potentially self-diagnose it before receiving an official diagnosis.
The alpha-gal syndrome is not a reportable or notifiable condition and was only granted a separate International Classification of Diseases 10th Revision ( ICD-10 ) code for diagnostic identification in 2022. Therefore, little data exist on the prevalence of the allergy [ 8 ]. In the absence of incidence or prevalence data, infoveillence and infodemiology techniques can be used to help describe the overall population interest in the topic of alpha-gal [ 25 ], as well as correlations with potentially related search terms. Many studies over the past decade have used Google Trends as a tool to explore patterns in health information–seeking behavior [ 26 , 27 ]. In fact, 1 prior study using Google Trends found a high correlation between alpha-gal and lone star tick, including a general upward trend in relative search volume (RSV) [ 21 ]. Data were examined from 2004 to 2019, but it is likely that both awareness and diagnosis of the allergy have grown in the past 3 years [ 20 ].
Further, new tools have emerged that offer enhanced features for analyzing various aspects of information-seeking behavior. One such tool is Glimpse, which is an extension app of Google Trends [ 28 ]. Google Trends has been both praised and criticized for reporting all searches as RSVs [ 29 ]. RSV is a query share of a search term within a specific geography and time range, which is then normalized to the highest point of popularity within that time period [ 26 ]. A notable drawback of this approach is that the absolute volume is not provided; therefore, there is no way to track an estimated number of queries for a particular topic [ 30 ]. Because the RSV is indexed to the highest point of popularity for a term within a time period, reproducibility of research results can be challenging [ 26 , 29 ]. To address this issue, the Supercharged-Glimpse extension offers an estimate of the absolute search volume, as well as a dashboard of additional information such as related search terms. The absolute volume numbers are overlayed on the traditional RSV index graph. This tool could be useful for understanding the specific content topics and themes that people search for health information.
Additional quantification of the changing volume of information seeking for alpha-gal can provide valuable evidence regarding shifts in awareness levels and potential changes in alpha-gal prevalence. Moreover, analyzing the evolving information-seeking patterns for both alpha-gal and lone star ticks can offer insights into the changing public interest across different geographic areas. Finally, health care providers and public health officials could benefit from understanding the topics that are most relevant to their patients and the public and adjust their communication strategies for alpha-gal accordingly. Therefore, using the Google Trends Supercharged-Glimpse extension, our objectives were to (1) describe the basic volume and patterns of information seeking related to alpha-gal; (2) explore further correlations between alpha-gal and lone star ticks; and (3) understand and identify specific questions, concerns, and areas of interest that individuals are searching for in relation to alpha-gal.
Google Trends Supercharged-Glimpse
Google Trends Supercharged-Glimpse is a new web-based tool available as an extension of Google Trends [ 28 ]. Glimpse functions in parallel with Google Trends and uses a similar random sampling approach to produce reports containing multiple components of trends in web-based information–seeking behavior. Searches are conducted on the Google Trends platform, with options to specify a particular geographic location and time period (spanning from 2004 to the present day). With the Glimpse extension, estimated search trends over time are provided as an absolute volume, rather than RSV. This allows for direct comparisons of volume from separate terms conducted in separate searches. The extension also produces a list of the highest volume of keywords and questions related to the search term, which is akin to a listing of terms that information seekers use in addition to the main keyword. Reports also contain the RSV for geographic areas from Google Trends.
Search Strategy
To begin our search, we implemented a methodological framework based upon previous strategies aimed to establish a consistent approach to conducting Google Trends research [ 31 , 32 ] and adhered to a suggested checklist for documenting and reporting our search strategy [ 26 ]. We report keywords in italics and the classification of the keyword in parentheses.
Google Trends Supercharged-Glimpse was used to determine search trends for 2 alpha-gal keywords: alpha gal (search term) and meat allergy (search term). Additional keywords related to alpha-gal were explored ( alpha-gal , alpha-gal syndrome , and alpha gal syndrome ), but these terms were visibly unstable, indicating a low search volume (with most months returning less than 1000 searches) and lower likelihood of using these terms when seeking information. Because these spelling variations produced very low volumes of searches and because the Supercharged tool does not allow modifiers (eg, “+” to add the terms together), we proceeded with the analysis without aggregating these data [ 31 ] but recognized these as potential limitations. We selected lone star tick (animal) as a keyword to explore objective 2 and food allergy (search term) as a broad comparison term. All keyword searches were compared with and without the use of quotations and produced similar results in terms of volume. Data were limited to the United States due to the context of the alpha-gal and lone star tick exploration, and the time frame ranged to include all available data (from January 1, 2004, to March 1, 2023). All keyword searches were conducted separately, without combining keywords. Similar to Google Trends, the Glimpse extension performs a sampling approach to estimate the absolute search volume. As with all sampling approaches, there is a degree of variability each time the data are queried. Further, there is a degree of caching that occurs; therefore, the same search conducted in a short time period (eg, within 10 minutes) may have identical numerical results. To produce a better estimate of the absolute volume of searches for our selected search terms, data were collected at 2 PM daily for a period of 10 days (March 2-12, 2023). Data for each term were compiled and averaged for search volume over time (absolute volume), related search queries (qualitative lists), and geographic interest (RSV). To demonstrate the overlap and correlation between the traditional RSV index values from Google Trends and the absolute volume estimates from the Supercharged-Glimpse extension, the averaged absolute volume and the averaged RSV index values for alpha gal (search term) are plotted in Figure S1 in Multimedia Appendix 1 . Absolute volume over time for all terms is presented in the Results section.
Statistical Analysis
Objective 1: trends over time.
Time series analyses were used to assess the trends in all 4 keywords over the study period. A quasi-Poisson regression was fit to account for the overdispersion of the count outcome variables. The regression used a penalized spline on year to account for long-term trends in these various allergies and related searches over time. We opted to use a penalized spline because the data appeared to be nonlinear in the raw data plots. Using penalized splines on the year allowed for the flexibility to account for the complex patterns seen in the raw data plots while not overfitting the model. The seasonality of web-based searches is likely to mirror the seasonality of cases of disease [ 31 ]. Therefore, we expected to see seasonal variation in search patterns for all 4 search terms, and indeed, this is what was revealed in graphing the monthly search volumes. There are seasonal variations in tick behaviors and tick-host encounters, which both peak during the warm season [ 33 , 34 ]. Additionally, food-related anaphylaxis has been found to increase during the warm season due to oral allergies (eg, pollen-food allergy syndrome) [ 35 ]. Therefore, a binary variable was created to indicate the warm season (May-September), which was then controlled via a linear term to account for seasonal trends. Google trends data for rare diseases can be influenced by the media [ 29 ]; therefore, the dates of national media stories on alpha-gal were identified from 2012 through 2023, and this (binary variable for the months that contained national media coverage) was controlled using a linear term. The predicted absolute search volume values are shown in the Results section. The average annual percent change (AAPC) in search volume was calculated based on this adjusted model for all 4 terms [ 36 ].
Objective 2: Alpha-Gal and Lone Star Tick
While our original intent was to describe how information-seeking behavior for both alpha gal and lone star tick changed geographically and temporally from 2004 to 2022, initial data extraction efforts revealed that the RSV geographic metrics were unstable and unreliable from 2004 to 2013. This is likely due to a lower volume of searches conducted during this time period, which would increase sampling variability. To produce more stable and reproducible results for the geographic analysis, the geographic interest index values were queried and exported in 3-year time period increments (2014-2016, 2017-2019, and 2020-2022) for the alpha gal (search term) and lone star tick ( animal ) keywords. Choropleth maps were produced. The distribution of the data was found to be nonnormally distributed (Shapiro-Wilk test P values <.05 for all variables); therefore, a Spearman correlation matrix was generated to examine geographic and temporal correlations for alpha gal and lone star tick using the RSV index values for the 3 time periods (2014-2016, 2017-2019, and 2020-2022).
Objective 3: Content Analysis
A conceptual content analysis was performed on the related search query data for alpha-gal. Lists from the 10 separate days were examined and found to be nearly identical. Lists were then combined and duplicates were removed, leaving a total of 371 related searches. All 371 related search queries contained the words “alpha gal” either before or after the additional keywords (eg, alpha gal hives ). For the qualitative content analysis, an inductive coding strategy was first implemented to identify overarching themes based on the content of the data itself. We identified 10 main themes: diet, diagnosis or testing, treatment, medications or contraindications of medications, symptoms, tick related, specific sources of information and locations, general education information, alternative words for alpha-gal, and unrelated or other. Two authors (JR and NJ) independently coded the list of 371 words based on the main concept themes. The results from the coders were compared, and discrepancies were resolved for a final theme designation. This process was repeated within each theme to further identify theme-based subcategories of interest. The total number of subthemes identified was 41. The frequency of keywords in each search theme and theme-based subcategories was compiled and described. All analyses were performed using SAS (version 9.4; SAS Institute) and R Studio (R Foundation for Statistical Computing).
Ethical Considerations
All data were publicly available and unidentifiable search engine metadata. Data are not used at the individual level and do not involve human subjects; therefore, institutional review board approval was not required for this study.
Time Series Analyses
The average absolute search volumes from 2004 to March 2023 were plotted for alpha gal , meat allergy , lone star tick , and food allergy ( Figure 1 ). The comparison of the traditional RSV index values and the absolute volume estimates demonstrated near-perfect overlap, with a Spearman correlation coefficient of ρ=0.99 ( P <.001; Figure S1 in Multimedia Appendix 1 ). In the time series analysis, the estimated absolute search volume values over time were nonlinear for all 4 search terms ( Figure 2 ). The binary indicator for the warm season was a significant predictor for all 4 search terms. While all 4 search terms demonstrated this significant seasonal trend, the magnitude of the warm season term was greatest for lone star tick and lowest for food allergy. Media coverage was only a significant predictor for alpha gal , lone star tick , and meat allergy . National media coverage of alpha-gal did not significantly impact food allergy searches. Based on the expected search volumes after adjusting for seasonality and media events in the time series analysis, the AAPC from 2004 to 2022 was 18.76%, 10.87%, 7.52%, and 1.09% for alpha gal , meat allergy , lone star tick , and food allergy , respectively. Interestingly, search trend volume began to noticeably increase starting around 2015 for alpha gal. From January 2015 to January 2022, the AAPC was 33.78%, 9.23%, 7.34%, and 2.45% for alpha gal , meat allergy , lone star tick , and food allergy , respectively. The estimated total increase during this time period was 627%, 81%, 61%, and 25% for alpha gal , meat allergy , lone star tick , and food allergy searches.

Alpha-Gal and Lone Star Tick
Choropleth maps of the RSV indices for alpha gal and lone star tick , as well as the Spearman correlation coefficient for each time period, are presented in Figure 3 . All correlations were significant at P <.001. State RSV indices for alpha gal and lone star tick were moderately correlated in 2014-2016 (ρ=0.59) but became more strongly correlated in the latter 2 time periods (ρ=0.82; ρ=0.80). These correlations can be visualized by the noticeable geographic overlap observed over time between states with a high information-seeking interest in alpha gal and lone star ticks .

Content Analysis
The content analysis revealed 10 overarching themes ( Figure 4 ). Of the 10 overarching themes, 7 were further divided into subcategories.

The most diverse search theme was diet (96/371, 25.9%), which could be further divided into 6 subthemes. The most popular subtheme was composed of general inquiries (eg, what foods to avoid with alpha gal? and what foods are safe with alpha gal? ), followed by food additives (eg, gelatin , glycerin , and magnesium stearate ), red meat (ie, specific mammal inquiries ), nonmammalian food options (eg, ostrich , eggs , and turkey ), dairy products (eg, butter , milk , and cheese ), and searches for recipes.
Symptom-related searchers accounted for 11.9% (44/371) of the total related search queries. General symptom–related questions or inquiries (eg, what are the symptoms? ) were most prominent, followed by symptom onset or triggers (eg, reaction time , onset , and exercise ). The other 5 specific subtheme symptoms that emerged were skin or rash (7/44, 15.9%), neurological (4/44, 9.1%), joint pain (3/44, 6.8%), gastrointestinal (3/44, 6.8%), and cardiovascular (2/44, 4.5%) symptoms.
Diagnosis-related searchers accounted for 10.8% (40/371) of the total related searches, including 4 subthemes of general searches for testing (eg, blood test and test results ), specific searches for IgE (eg, IgE numbers and levels ), specific laboratories that perform testing, and searches for ICD-10 codes.
General Education
General education and information seeking accounted for 10.5% (39/371) of the related searches. This theme was further broken down into causes (eg, what is the cause of alpha gal? , is alpha gal genetic? , is alpha gal contagious? ), duration (eg, is alpha gal permanent? , does alpha gal go away? ), general questions (eg, what is alpha gal? ), products, information seeking on the knockout pig, and other (eg, alpha gal and COVID-19 , alpha-gal while pregnant , and prevalence ).
Specific Sources
Specific sources of information or location accounted for 10.2% (38/371) of searches. Around 24% (9/38) of these were media-specific searches (eg, alpha-gal radiolab and nytimes ), 24% (9/38) were evidence-based sources (eg, NIH , UpToDate , CDC , and Mayo Clinic ), and 21% (8/38) were location or place specific (eg, Kentucky , Lynchburg VA , and UVA ). Other subgroups included nonevidence-based sources, specific clinician or health care provider searches, and others.
Treatment-related searches accounted for 9.4% (35/371). Most searches were general (eg. how to treat alpha gal and is it curable? ), while others were specifically related to acupuncture, antihistamines, and home remedies (eg, natural remedies and essential oils ).
Medication or contraindication-related searches accounted for 7.8% (27/371) of related searches. Roughly 25% (7/27) of these searches were vaccine related (eg, flu shot and COVID-19 vaccine ). About 25% (7/27) were inquiries about medications known to contain alpha-gal (eg, heparin), while the majority (37%, 16/27) were general inquiries on what medications were necessary to avoid (eg, alpha gal in medications , drugs to avoid , alpha gal medication list , and alpha gal and anesthesia ).
Principal Findings
To our knowledge, we believe this is one of the first studies to investigate absolute search volume trends over time using the Google Supercharged-Glimpse extension app. Our analysis revealed several important trends and patterns regarding public interest and information seeking for alpha-gal allergy.
First, over the past several years, the volume of searches for the alpha-gal allergy is significantly increasing at a rapid rate. After adjusting for seasonality and national media stories, the overall increase for alpha gal searches over time was at least 6 times that of the other search terms. Interestingly, alpha gal search term volume surpassed that of meat allergy in 2019, which likely indicates an increase in the awareness of the correct terminology for the allergy. Further, while lone star tick and meat allergy search term volumes seemed to level off from 2020 to 2022, alpha gal search term interest continued to grow. National media stories were found to have a significant impact on the search volume for alpha gal , meat allergy , and lone star tick terms, highlighting the role that media can play in engaging public interest and awareness of these topics.
Geographic popularity information-seeking for the term alpha gal did not vary greatly over time and remained concentrated in the southeast regions of the United States. While this finding was similar to a study using 2019 data [ 21 ], it was somewhat unexpected. Diagnoses of alpha-gal syndrome have occurred in other geographic regions including the Northeast and Midwest [ 20 ], and cases are increasing in those areas [ 8 ]. It is possible that this finding might be reflective of how the RSV index is generated. States with larger populations could have a greater absolute volume of interest, but this might not be reflected in the RSV index. There were no states throughout time with an RSV index of 0, which indicates that people are seeking information for the alpha-gal allergy in all 50 states. This finding is similar to laboratory-based studies that show documented cases in most states [ 20 ].
Geographic searches for the terms alpha gal and lone star tick strongly overlap. States have similarly ranked RSV indices for both alpha gal and lone star tick terms, and these have strengthened over time. Of note, geographic search interest in lone star ticks appears to be expanding. Search interest maps from 2014 to 2016 and 2017 to 2019 strongly overlap with a 2016 study that documented expansions of the lone star tick range [ 19 ]. Furthermore, search interest maps from 2020 to 2022 show a similar overlap with predicted lone star tick habitat expansion models developed in 2021 [ 37 ]. It is possible, therefore, that future interest and prevalence maps for alpha-gal may expand in similar ways. This serves as a forewarning, highlighting the urgent need to expand education efforts for both the general public and health care providers.
Finally, people are seeking a broad array of topics related to alpha-gal. The largest subgroup of content searched for in conjunction with alpha-gal was tick related. Encouragingly, national media stories and evidence-based sources comprised almost 50% of the specific sources that people were searching for. Unsurprisingly, diet was the largest theme, and interest in concealed sources of exposure and food additives remains a large concern [ 11 ]. The ubiquitous presence of animal products in food, medications, and other products can make it extremely difficult for individuals to know if what they ingest is safe [ 8 ]. Given the challenges of identifying safe foods and products, there is a clear need for improved food and product labeling. Additionally, information seeking for specific symptoms highlighted the broad array of ways that alpha-gal can manifest within an individual. Symptom variety remains one of the major reasons alpha-gal remains underdiagnosed. Health care professionals should not only be familiar with the most commonly identifiable symptoms of alpha-gal such as urticaria or anaphylaxis [ 18 ] but also be knowledgeable about symptom manifestations like joint pain [ 8 ], gastrointestinal symptoms [ 12 , 18 , 38 ], dizziness, or heart palpitations [ 10 ].
Limitations
There are several limitations to this study. First, we were only able to capture information seeking on 1 search engine platform. Second, absolute search volume estimates were not available geographically, and the RSV geographic data were unstable when examined yearly. We chose to sacrifice the granularity of a year-by-year analysis in favor of more reliable results. Third, the absolute volume was also not provided for each of the related searches. Therefore, the proportional composition of the content analysis themes represents the diversity within the theme, not necessarily the popularity of the theme. It is possible that tick-related searches encompassed the majority of the volume of the related alpha-gal searches, but we cannot identify this based on the data. Finally, at the time of the initial data analysis and submission of this study, alpha-gal syndrome was only available as a search term and not a disease. Since that time, the term has become searchable on the Google Trends platform as a designated syndrome. Absolute search volume trends over time for both alpha gal (search term) and alpha-gal syndrome (syndrome) are remarkably similar, indicating there is likely direct overlap between these 2 searches. It is unknown to what degree the addition of this condition as a designated syndrome may affect the volume reported for the alpha gal (search term) in the future.
Conclusions
Information seeking for alpha-gal syndrome is rapidly increasing. Geographic overlap with lone star tick searches might suggest future expansions in alpha-gal interest and prevalence, thereby emphasizing the urgency for increased education efforts. The diverse range of topics and symptoms searched for highlights the ongoing challenges faced by individuals affected by alpha-gal. However, the content and subthemes identified can serve as a valuable guide to facilitate public health outreach and effective patient-physician communication.
Acknowledgments
We would like to thank Adam Starke for assistance in generating the choropleth maps.
Data Availability
The data sets generated and analyzed during this study are available from the corresponding author on reasonable request.
Conflicts of Interest
None declared.
Comparison of absolute search volume and relative search volume (RSV) index over time for alpha gal (search term).
- Dunlop JH, Keet CA. Epidemiology of food allergy. Immunol Allergy Clin North Am. 2018;38(1):13-25. [ CrossRef ] [ Medline ]
- De Martinis M, Sirufo MM, Suppa M, Ginaldi L. New perspectives in food allergy. Int J Mol Sci. 2020;21(4):1474. [ FREE Full text ] [ CrossRef ] [ Medline ]
- Fiocchi A, Risso D, DunnGalvin A, Díaz SNG, Monaci L, Fierro V, et al. Food labeling issues for severe food allergic patients. World Allergy Organ J. 2021;14(10):100598. [ FREE Full text ] [ CrossRef ] [ Medline ]
- Ng AE, Boersma P. Diagnosed allergic conditions in adults: United States, 2021. NCHS Data Brief. 2023;(460):1-8. [ FREE Full text ] [ Medline ]
- Gupta R, Holdford D, Bilaver L, Dyer A, Holl JL, Meltzer D. The economic impact of childhood food allergy in the United States. JAMA Pediatr. 2013;167(11):1026-1031. [ FREE Full text ] [ CrossRef ] [ Medline ]
- Blok BMJF, Dubois AEJ, Vlieg-Boerstra BJ, Elberink JNGO, Raat H, DunnGalvin A, et al. Health-related quality of life of food allergic patients: comparison with the general population and other diseases. Allergy. 2010;65(2):238-244. [ FREE Full text ] [ CrossRef ] [ Medline ]
- DunnGalvin A, Dubois AEJ, Blok BMJF, Hourihane JOB. The effects of food allergy on quality of life. Chem Immunol Allergy. 2015;101:235-252. [ FREE Full text ] [ CrossRef ] [ Medline ]
- Commins SP. Diagnosis and management of alpha-gal syndrome: lessons from 2,500 patients. Expert Rev Clin Immunol. 2020;16(7):667-677. [ FREE Full text ] [ CrossRef ] [ Medline ]
- Carson AS, Gardner A, Iweala OI. Where's the Beef? Understanding allergic responses to red meat in alpha-gal syndrome. J Immunol. 2022;208(2):267-277. [ FREE Full text ] [ CrossRef ] [ Medline ]
- Alpha-gal syndrome. Centers for Disease Control and Prevention. 2023. URL: https://www.cdc.gov/ticks/alpha-gal/index.html [accessed 2023-06-07]
- Macdougall JD, Thomas KO, Iweala OI. The meat of the matter: understanding and managing alpha-gal syndrome. Immunotargets Ther. 2022;11:37-54. [ FREE Full text ] [ CrossRef ] [ Medline ]
- Wilson JM, Schuyler AJ, Workman L, Gupta M, James HR, Posthumus J, et al. Investigation into the α-gal syndrome: characteristics of 261 children and adults reporting red meat allergy. J Allergy Clin Immunol Pract. 2019;7(7):2348-2358.e4. [ FREE Full text ] [ CrossRef ] [ Medline ]
- Crispell G, Commins SP, Archer-Hartman SA, Choudhary S, Dharmarajan G, Azadi P, et al. Discovery of alpha-gal-containing antigens in North American tick species believed to induce red meat allergy. Front Immunol. 2019;10:1056. [ FREE Full text ] [ CrossRef ] [ Medline ]
- Mitchell CL, Lin FC, Vaughn M, Apperson CS, Meshnick SR, Commins SP. Association between lone star tick bites and increased alpha-gal sensitization: evidence from a prospective cohort of outdoor workers. Parasit Vectors. 2020;13(1):470. [ FREE Full text ] [ CrossRef ] [ Medline ]
- Commins SP, James HR, Kelly LA, Pochan SL, Workman LJ, Perzanowski MS, et al. The relevance of tick bites to the production of IgE antibodies to the mammalian oligosaccharide galactose-α-1,3-galactose. J Allergy Clin Immunol. 2011;127(5):1286-1293.e6. [ FREE Full text ] [ CrossRef ] [ Medline ]
- Kersh GJ, Salzer J, Jones ES, Binder AM, Armstrong PA, Choudhary SK, et al. Tick bite as a risk factor for alpha-gal-specific immunoglobulin E antibodies and development of alpha-gal syndrome. Ann Allergy Asthma Immunol. 2023;130(4):472-478. [ FREE Full text ] [ CrossRef ] [ Medline ]
- Sharma SR, Karim S. Tick saliva and the alpha-gal syndrome: finding a needle in a haystack. Front Cell Infect Microbiol. 2021;11:680264. [ FREE Full text ] [ CrossRef ] [ Medline ]
- Young I, Prematunge C, Pussegoda K, Corrin T, Waddell L. Tick exposures and alpha-gal syndrome: a systematic review of the evidence. Ticks Tick Borne Dis. 2021;12(3):101674. [ FREE Full text ] [ CrossRef ] [ Medline ]
- Molaei G, Little EAH, Williams SC, Stafford KC. Bracing for the worst - range expansion of the lone star tick in the Northeastern United States. N Engl J Med. 2019;381(23):2189-2192. [ FREE Full text ] [ CrossRef ] [ Medline ]
- Binder AM, Commins SP, Altrich ML, Wachs T, Biggerstaff BJ, Beard CB, et al. Diagnostic testing for galactose-alpha-1,3-galactose, United States, 2010 to 2018. Ann Allergy Asthma Immunol. 2021;126(4):411-416.e1. [ FREE Full text ] [ CrossRef ] [ Medline ]
- Iglesia EGA, Stone CA, Flaherty MG, Commins SP. Regional and temporal awareness of alpha-gal allergy: an infodemiological analysis using Google Trends. J Allergy Clin Immunol Pract. 2020;8(5):1725-1727.e1. [ FREE Full text ] [ CrossRef ] [ Medline ]
- Carson DA, Kopsco H, Gronemeyer P, Mateus-Pinilla N, Smith GS, Sandstrom EN, et al. Knowledge, attitudes, and practices of illinois medical professionals related to ticks and tick-borne disease. One Health. 2022;15:100424. [ FREE Full text ] [ CrossRef ] [ Medline ]
- Flaherty MG, Kaplan SJ, Jerath MR. Diagnosis of life-threatening alpha-gal food allergy appears to be patient driven. J Prim Care Community Health. 2017;8(4):345-348. [ FREE Full text ] [ CrossRef ] [ Medline ]
- Flaherty MG, Threats M, Kaplan SJ. Patients' health information practices and perceptions of provider knowledge in the case of the newly discovered alpha-gal food allergy. J Patient Exp. 2020;7(1):132-139. [ FREE Full text ] [ CrossRef ] [ Medline ]
- Eysenbach G. Infodemiology and infoveillance: framework for an emerging set of public health informatics methods to analyze search, communication and publication behavior on the internet. J Med Internet Res. 2009;11(1):e11. [ FREE Full text ] [ CrossRef ] [ Medline ]
- Nuti SV, Wayda B, Ranasinghe I, Wang S, Dreyer RP, Chen SI, et al. The use of Google Trends in health care research: a systematic review. PLoS One. 2014;9(10):e109583. [ FREE Full text ] [ CrossRef ] [ Medline ]
- Barros JM, Duggan J, Rebholz-Schuhmann D. The application of internet-based sources for public health surveillance (infoveillance): systematic review. J Med Internet Res. 2020;22(3):e13680. [ FREE Full text ] [ CrossRef ] [ Medline ]
- Glimpse home page. Glimpse. 2023. URL: https://meetglimpse.com/extension/ [accessed 2023-03-02]
- Cervellin G, Comelli I, Lippi G. Is Google Trends a reliable tool for digital epidemiology? Insights from different clinical settings. J Epidemiol Glob Health. 2017;7(3):185-189. [ FREE Full text ] [ CrossRef ] [ Medline ]
- Alibudbud R. Google Trends for health research: its advantages, application, methodological considerations, and limitations in psychiatric and mental health infodemiology. Front Big Data. 2023;6:1132764. [ FREE Full text ] [ CrossRef ] [ Medline ]
- Mavragani A, Ochoa G, Tsagarakis KP. Assessing the methods, tools, and statistical approaches in Google Trends research: systematic review. J Med Internet Res. 2018;20(11):e270. [ FREE Full text ] [ CrossRef ] [ Medline ]
- Neumann K, Mason SM, Farkas K, Santaularia NJ, Ahern J, Riddell CA. Harnessing google health trends data for epidemiologic research. Am J Epidemiol. 2023;192(3):430-437. [ FREE Full text ] [ CrossRef ] [ Medline ]
- Jordan RA, Egizi A. The growing importance of lone star ticks in a lyme disease endemic county: passive tick surveillance in Monmouth County, NJ, 2006 - 2016. PLoS One. 2019;14(2):e0211778. [ FREE Full text ] [ CrossRef ] [ Medline ]
- Preventing tick bites. Centers for Disease Control and Prevention. 2020. URL: https://www.cdc.gov/ticks/avoid/on_people.html#:~:text=Tick [accessed 2024-02-22]
- Lam HCY, Turner PJ, Hemming D, Jarvis DL. Seasonality of food-related anaphylaxis admissions and associations with temperature and pollen levels. J Allergy Clin Immunol Pract. 2021;9(1):518-520.e2. [ FREE Full text ] [ CrossRef ] [ Medline ]
- Clegg LX, Hankey BF, Tiwari R, Feuer EJ, Edwards BK. Estimating average annual per cent change in trend analysis. Stat Med. 2009;28(29):3670-3682. [ FREE Full text ] [ CrossRef ] [ Medline ]
- Alkishe A, Raghavan RK, Peterson AT. Likely geographic distributional shifts among medically important tick species and tick-associated diseases under climate change in North America: a review. Insects. 2021;12(3):225. [ FREE Full text ] [ CrossRef ] [ Medline ]
- Richards NE, Richards RD. Alpha-gal allergy as a cause of intestinal symptoms in a gastroenterology community practice. South Med J. 2021;114(3):169-173. [ FREE Full text ] [ CrossRef ] [ Medline ]
Abbreviations
Edited by A Mavragani; submitted 14.06.23; peer-reviewed by JR Medina, A Allam, S Wei; comments to author 08.02.24; revised version received 28.02.24; accepted 23.03.24; published 08.05.24.
©Jamie L Romeiser, Nicole Jusko, Augusta A Williams. Originally published in the Journal of Medical Internet Research (https://www.jmir.org), 08.05.2024.
This is an open-access article distributed under the terms of the Creative Commons Attribution License (https://creativecommons.org/licenses/by/4.0/), which permits unrestricted use, distribution, and reproduction in any medium, provided the original work, first published in the Journal of Medical Internet Research, is properly cited. The complete bibliographic information, a link to the original publication on https://www.jmir.org/, as well as this copyright and license information must be included.

New machine learning algorithm promises advances in computing
Digital twin models may enhance future autonomous systems.
Systems controlled by next-generation computing algorithms could give rise to better and more efficient machine learning products, a new study suggests.
Using machine learning tools to create a digital twin, or a virtual copy, of an electronic circuit that exhibits chaotic behavior, researchers found that they were successful at predicting how it would behave and using that information to control it.
Many everyday devices, like thermostats and cruise control, utilize linear controllers -- which use simple rules to direct a system to a desired value. Thermostats, for example, employ such rules to determine how much to heat or cool a space based on the difference between the current and desired temperatures.
Yet because of how straightforward these algorithms are, they struggle to control systems that display complex behavior, like chaos.
As a result, advanced devices like self-driving cars and aircraft often rely on machine learning-based controllers, which use intricate networks to learn the optimal control algorithm needed to best operate. However, these algorithms have significant drawbacks, the most demanding of which is that they can be extremely challenging and computationally expensive to implement.
Now, having access to an efficient digital twin is likely to have a sweeping impact on how scientists develop future autonomous technologies, said Robert Kent, lead author of the study and a graduate student in physics at The Ohio State University.
"The problem with most machine learning-based controllers is that they use a lot of energy or power and they take a long time to evaluate," said Kent. "Developing traditional controllers for them has also been difficult because chaotic systems are extremely sensitive to small changes."
These issues, he said, are critical in situations where milliseconds can make a difference between life and death, such as when self-driving vehicles must decide to brake to prevent an accident.
The study was published recently in Nature Communications.
Compact enough to fit on an inexpensive computer chip capable of balancing on your fingertip and able to run without an internet connection, the team's digital twin was built to optimize a controller's efficiency and performance, which researchers found resulted in a reduction of power consumption. It achieves this quite easily, mainly because it was trained using a type of machine learning approach called reservoir computing.
"The great thing about the machine learning architecture we used is that it's very good at learning the behavior of systems that evolve in time," Kent said. "It's inspired by how connections spark in the human brain."
Although similarly sized computer chips have been used in devices like smart fridges, according to the study, this novel computing ability makes the new model especially well-equipped to handle dynamic systems such as self-driving vehicles as well as heart monitors, which must be able to quickly adapt to a patient's heartbeat.
"Big machine learning models have to consume lots of power to crunch data and come out with the right parameters, whereas our model and training is so extremely simple that you could have systems learning on the fly," he said.
To test this theory, researchers directed their model to complete complex control tasks and compared its results to those from previous control techniques. The study revealed that their approach achieved a higher accuracy at the tasks than its linear counterpart and is significantly less computationally complex than a previous machine learning-based controller.
"The increase in accuracy was pretty significant in some cases," said Kent. Though the outcome showed that their algorithm does require more energy than a linear controller to operate, this tradeoff means that when it is powered up, the team's model lasts longer and is considerably more efficient than current machine learning-based controllers on the market.
"People will find good use out of it just based on how efficient it is," Kent said. "You can implement it on pretty much any platform and it's very simple to understand." The algorithm was recently made available to scientists.
Outside of inspiring potential advances in engineering, there's also an equally important economic and environmental incentive for creating more power-friendly algorithms, said Kent.
As society becomes more dependent on computers and AI for nearly all aspects of daily life, demand for data centers is soaring, leading many experts to worry over digital systems' enormous power appetite and what future industries will need to do to keep up with it.
And because building these data centers as well as large-scale computing experiments can generate a large carbon footprint, scientists are looking for ways to curb carbon emissions from this technology.
To advance their results, future work will likely be steered toward training the model to explore other applications like quantum information processing, Kent said. In the meantime, he expects that these new elements will reach far into the scientific community.
"Not enough people know about these types of algorithms in the industry and engineering, and one of the big goals of this project is to get more people to learn about them," said Kent. "This work is a great first step toward reaching that potential."
This study was supported by the U.S. Air Force's Office of Scientific Research. Other Ohio State co-authors include Wendson A.S. Barbosa and Daniel J. Gauthier.
- Electronics
- Telecommunications
- Energy Technology
- Computers and Internet
- Neural Interfaces
- Information Technology
- Computer Science
- Artificial intelligence
- Alan Turing
- Computing power everywhere
- Grid computing
- Data mining
Story Source:
Materials provided by Ohio State University . Original written by Tatyana Woodall. Note: Content may be edited for style and length.
Journal Reference :
- Robert M. Kent, Wendson A. S. Barbosa, Daniel J. Gauthier. Controlling chaos using edge computing hardware . Nature Communications , 2024; 15 (1) DOI: 10.1038/s41467-024-48133-3
Cite This Page :
Explore More
- Controlling Shape-Shifting Soft Robots
- Brain Flexibility for a Complex World
- ONe Nova to Rule Them All
- AI Systems Are Skilled at Manipulating Humans
- Planet Glows With Molten Lava
- A Fragment of Human Brain, Mapped
- Symbiosis Solves Long-Standing Marine Mystery
- Surprising Common Ideas in Environmental ...
- 2D All-Organic Perovskites: 2D Electronics
- Generative AI That Imitates Human Motion
Trending Topics
Strange & offbeat.
Internet User Behavior Analysis Based on Big Data
Ieee account.
- Change Username/Password
- Update Address
Purchase Details
- Payment Options
- Order History
- View Purchased Documents
Profile Information
- Communications Preferences
- Profession and Education
- Technical Interests
- US & Canada: +1 800 678 4333
- Worldwide: +1 732 981 0060
- Contact & Support
- About IEEE Xplore
- Accessibility
- Terms of Use
- Nondiscrimination Policy
- Privacy & Opting Out of Cookies
A not-for-profit organization, IEEE is the world's largest technical professional organization dedicated to advancing technology for the benefit of humanity. © Copyright 2024 IEEE - All rights reserved. Use of this web site signifies your agreement to the terms and conditions.
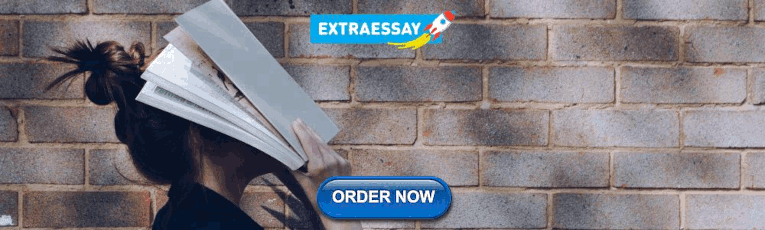
IMAGES
VIDEO
COMMENTS
Internet of Behaviors: A Surv ey. Jiayi Sun, W ensheng Gan*, Han-Chieh Chao, Philip S. Y u, Fellow, IEEE and Weiping Ding. Abstract —The Internet of Behavior is a research theme that. aims to ...
Fig. 2 reflects the various tools, traits, and strategies associated with the Internet of Behaviour (IoB) to enhance overall customer satisfaction and make their purchase worth it. It is all related to technology, data analytics, the science of behavioural facts, etc., which ultimately helps the IoB persons deal with the current situation to make it far better at later stages.
This paper addresses the Internet of Behavior. As an extension of the already prevalent Internet of Things, the technology is used to analyze, predict and influence behavioral trends of consumers in order to manifest a fixed business outcome through analysis of data that is collected using tracking and identification technologies, wired and wireless sensor networks and so on. As such, IoB ...
The Internet of Behavior is a research theme that aims to analyze human behavior data on the Internet from the perspective of behavioral psychology, obtain insights about human behavior, and better understand the intention behind the behavior. In this way, the Internet of Behavior can predict human behavioral trends in the future and even change human behavior, which can provide more ...
The Internet of Behavior (IoB) is a research theme that aims to analyze human behavior data on the Internet from the perspective of behavioral psychology, obtain insights about human behavior, and better understand the intention behind the behavior. In this way, the IoB can predict human behavioral trends in the future and even change human behavior, which can provide more convenience for ...
An in-depth overview of the fundamental aspects of the Internet of Behavior, a research theme that aims to analyze human behavior data on the Internet from the perspective of behavioral psychology, to obtain insights about human behavior, and better understand the intention behind the behavior. The Internet of Behavior (IoB) is a research theme that aims to analyze human behavior data on the ...
The next generation Internet-of-Things (IoT) is touted Internet-of-Behavior (IoB). Its topping quality is the dynamic generation of behavior (prescriptions), based on extensive data analytics. ... Design Science Research in Information Systems and Technology (DESRIST 2006), pp. 83-106, February 2006. ... Cite this paper. Stary, C. (2020). The ...
Abstract—The Internet of Behaviors (IoB) puts human be- havior at the core of engineering intelligent connected systems. IoB links the digital world to human behavior to establish human-driven design, development, and adaptation processes. This paper defines the novel concept by an IoB model based on
would play a major role in detecting people's behavior The Professor of Psychology, Gote Nyman, was the first to announce the concept of the Internet of Behavior (IoB). In 2012, he said that if the human behavioral pattern is assigned to devices (e.g. IoT devices) with specific ad-dresses, there will be an opportunity to benefit from the
The Internet of Behaviors (IoB) puts human behavior at the core of engineering intelligent connected systems. IoB links the digital world to human behavior to integrate human-driven design, development, and adaptation processes. This paper defines the novel IoB concept with a constructed model based on a collective effort interacting with software engineers, human-computer interaction ...
Abstract —Pandemics and natural disasters over the years have. changed the behavior of people, which has had a tremendous impact. on all life aspects. With the technologies availab le in each ...
The primary purpose of this paper is to study IoB and its need for healthcare. ... The term "Internet of Behavior" refers to the gathering and application of data to influence behaviour. These data are gathered by electrical appliances, personal internet activities, and wearable technology, and they can provide essential details about user ...
INTERNET OF BEHAVIOR Internet of Things (IoT) is a familiar topic among most Engineers these days. It is constantly expanding and evolving in the scope of its complexity. This evolution has resulted in a new emerging technology called the Internet of Behavior (IoB). Before understanding IoB, let us take a look at what is IoT. Internet of Things ...
Abstract—The Internet of Behavior is a research theme that aims to analyze human behavior data on the Internet from the perspective of behavioral psychology, obtain insights about ... To the best of our knowledge, this is the first survey paper on the Internet of Behavior. It first introduces an in-depth understanding of the IoB, that is ...
2.1 Changing Value Chains. The Internet of behavior is a trinity of technology, data analytics, and behavioral science that enables us to forecast, analyze, and even affect human behavior. Simultaneously, IoB technologies have the potential to transform the value chains that connect platforms and people.
The scale of Wells et al. (2011) was adapted to measure the buying impulse; online purchase intention was measured based on the studies by Pavlou (2003). Finally, the scale to measure online purchase behavior was obtained from the study by George (2004). Appendix 1 shows the scales adapted. 4.
The Internet is no longer a technological innovation. It has become part of the daily lives of millions of people, with active Internet users reaching 59.6% of the global population as of January 2021 (Johnson, Citation 2021).Deeply embedded in the daily functions of societies and individuals, the Internet has been the subject of numerous studies, many of which have focused on the effects of ...
Overall, the available evidence indicates that the Internet can produce both acute and sustained alterations in each of these areas of cognition, which may be reflected in changes in the brain. However, an emerging priority for future research is to determine the effects of extensive online media usage on cognitive development in youth, and ...
This is evident from the results of research using the Internet for social media as much as 82 respondents or 68.33% answered always and 50 respondents or 41.67% answered frequently, use for ...
The Internet of Behaviors (IoB) puts human behavior at the core of engineering intelligent connected systems. IoB links the digital world to human behavior to establish human-driven design, development, and adaptation processes. This paper defines the novel concept by an IoB model based on a collective effort interacting with software engineers, human-computer interaction scientists, social ...
This paper aims to propose a prediction method based on Deep Learning (DL) and Internet of Things (IoT) technology, focusing on the ecological security and tourist satisfaction of Ice-and-Snow ...
The Internet of Behavior is a research theme that aims to analyze human behavior data on the Internet from the perspective of behavioral psychology, obtain insights about human behavior, and better understand the intention behind the behavior. In this way, the Internet of Behavior can predict human behavioral trends in the future and even change human behavior, which can provide more ...
A recent study emphasizes the increasing problems with artificial intelligence (AI) systems' deceptive responses. This is according to a review paper published in the journal Patterns about ...
A botnet is a network of compromised computer systems, or bots, remotely controlled by an attacker through bot controllers. This covert network poses a threat through large-scale cyber attacks, including phishing, distributed denial of service (DDoS), data theft, and server crashes. Botnets often camouflage their activity by utilizing common internet protocols, such as HTTP and IRC, making ...
Heterogeneous data have been used to enhance behavior prediction performance; however, it involves issues such as missing data, which need to be addressed. This paper proposes enhanced pet behavior prediction via Sensor to Skeleton Generative Adversarial Networks (S2GAN)-based heterogeneous data synthesis. The S2GAN model synthesizes the key features of video skeletons based on collected nine ...
In his blogs of 2012, Dr. Göte Nyman coined Internet of Behaviors (IoB). In his idea, people's behaviors are very good predictors of their needs, and hence technology companies can network human behaviors and construct behavior-based systems to deliver more timely, responsive, and intelligent services with privacy protection. In 2020, Gartner ranked IoB first among its top nine strategic ...
The Google Trends Supercharged-Glimpse tool offers enhanced features for analyzing information-seeking behavior and can be valuable for infodemiology research. Further research is needed to explore the evolving prevalence and impact of alpha-gal syndrome. ... Journal of Medical Internet Research 8345 articles ... This paper is in the following ...
The study was published recently in Nature Communications.. Compact enough to fit on an inexpensive computer chip capable of balancing on your fingertip and able to run without an internet ...
The bond-slip behavior of the steel-concrete interface is critical in reinforced concrete (RC) structures since the bond action is the mechanism that ensures the two materials work in co-operation. However, there is little research considering the bond-slip behavior in massive ring-type reinforced concrete (MRRC) structure bearing analyses due to the complexity of modeling the ...
The purpose of the research in this article is to conduct data analysis on Internet user behavior in the era of big data, and to make innovative development of traditional user behavior patterns. ... this paper can reveal the internal laws of Internet user behavior relatively completely. The experimental results show that the use of big data ...