data mining Recently Published Documents
Total documents.
- Latest Documents
- Most Cited Documents
- Contributed Authors
- Related Sources
- Related Keywords
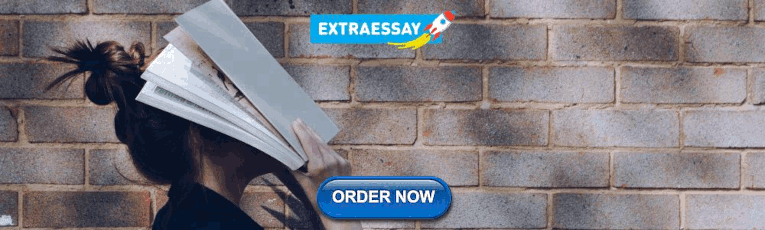
Distance Based Pattern Driven Mining for Outlier Detection in High Dimensional Big Dataset
Detection of outliers or anomalies is one of the vital issues in pattern-driven data mining. Outlier detection detects the inconsistent behavior of individual objects. It is an important sector in the data mining field with several different applications such as detecting credit card fraud, hacking discovery and discovering criminal activities. It is necessary to develop tools used to uncover the critical information established in the extensive data. This paper investigated a novel method for detecting cluster outliers in a multidimensional dataset, capable of identifying the clusters and outliers for datasets containing noise. The proposed method can detect the groups and outliers left by the clustering process, like instant irregular sets of clusters (C) and outliers (O), to boost the results. The results obtained after applying the algorithm to the dataset improved in terms of several parameters. For the comparative analysis, the accurate average value and the recall value parameters are computed. The accurate average value is 74.05% of the existing COID algorithm, and our proposed algorithm has 77.21%. The average recall value is 81.19% and 89.51% of the existing and proposed algorithm, which shows that the proposed work efficiency is better than the existing COID algorithm.
Implementation of Data Mining Technology in Bonded Warehouse Inbound and Outbound Goods Trade
For the taxed goods, the actual freight is generally determined by multiplying the allocated freight for each KG and actual outgoing weight based on the outgoing order number on the outgoing bill. Considering the conventional logistics is insufficient to cope with the rapid response of e-commerce orders to logistics requirements, this work discussed the implementation of data mining technology in bonded warehouse inbound and outbound goods trade. Specifically, a bonded warehouse decision-making system with data warehouse, conceptual model, online analytical processing system, human-computer interaction module and WEB data sharing platform was developed. The statistical query module can be used to perform statistics and queries on warehousing operations. After the optimization of the whole warehousing business process, it only takes 19.1 hours to get the actual freight, which is nearly one third less than the time before optimization. This study could create a better environment for the development of China's processing trade.
Multi-objective economic load dispatch method based on data mining technology for large coal-fired power plants
User activity classification and domain-wise ranking through social interactions.
Twitter has gained a significant prevalence among the users across the numerous domains, in the majority of the countries, and among different age groups. It servers a real-time micro-blogging service for communication and opinion sharing. Twitter is sharing its data for research and study purposes by exposing open APIs that make it the most suitable source of data for social media analytics. Applying data mining and machine learning techniques on tweets is gaining more and more interest. The most prominent enigma in social media analytics is to automatically identify and rank influencers. This research is aimed to detect the user's topics of interest in social media and rank them based on specific topics, domains, etc. Few hybrid parameters are also distinguished in this research based on the post's content, post’s metadata, user’s profile, and user's network feature to capture different aspects of being influential and used in the ranking algorithm. Results concluded that the proposed approach is well effective in both the classification and ranking of individuals in a cluster.
A data mining analysis of COVID-19 cases in states of United States of America
Epidemic diseases can be extremely dangerous with its hazarding influences. They may have negative effects on economies, businesses, environment, humans, and workforce. In this paper, some of the factors that are interrelated with COVID-19 pandemic have been examined using data mining methodologies and approaches. As a result of the analysis some rules and insights have been discovered and performances of the data mining algorithms have been evaluated. According to the analysis results, JRip algorithmic technique had the most correct classification rate and the lowest root mean squared error (RMSE). Considering classification rate and RMSE measure, JRip can be considered as an effective method in understanding factors that are related with corona virus caused deaths.
Exploring distributed energy generation for sustainable development: A data mining approach
A comprehensive guideline for bengali sentiment annotation.
Sentiment Analysis (SA) is a Natural Language Processing (NLP) and an Information Extraction (IE) task that primarily aims to obtain the writer’s feelings expressed in positive or negative by analyzing a large number of documents. SA is also widely studied in the fields of data mining, web mining, text mining, and information retrieval. The fundamental task in sentiment analysis is to classify the polarity of a given content as Positive, Negative, or Neutral . Although extensive research has been conducted in this area of computational linguistics, most of the research work has been carried out in the context of English language. However, Bengali sentiment expression has varying degree of sentiment labels, which can be plausibly distinct from English language. Therefore, sentiment assessment of Bengali language is undeniably important to be developed and executed properly. In sentiment analysis, the prediction potential of an automatic modeling is completely dependent on the quality of dataset annotation. Bengali sentiment annotation is a challenging task due to diversified structures (syntax) of the language and its different degrees of innate sentiments (i.e., weakly and strongly positive/negative sentiments). Thus, in this article, we propose a novel and precise guideline for the researchers, linguistic experts, and referees to annotate Bengali sentences immaculately with a view to building effective datasets for automatic sentiment prediction efficiently.
Capturing Dynamics of Information Diffusion in SNS: A Survey of Methodology and Techniques
Studying information diffusion in SNS (Social Networks Service) has remarkable significance in both academia and industry. Theoretically, it boosts the development of other subjects such as statistics, sociology, and data mining. Practically, diffusion modeling provides fundamental support for many downstream applications (e.g., public opinion monitoring, rumor source identification, and viral marketing). Tremendous efforts have been devoted to this area to understand and quantify information diffusion dynamics. This survey investigates and summarizes the emerging distinguished works in diffusion modeling. We first put forward a unified information diffusion concept in terms of three components: information, user decision, and social vectors, followed by a detailed introduction of the methodologies for diffusion modeling. And then, a new taxonomy adopting hybrid philosophy (i.e., granularity and techniques) is proposed, and we made a series of comparative studies on elementary diffusion models under our taxonomy from the aspects of assumptions, methods, and pros and cons. We further summarized representative diffusion modeling in special scenarios and significant downstream tasks based on these elementary models. Finally, open issues in this field following the methodology of diffusion modeling are discussed.
The Influence of E-book Teaching on the Motivation and Effectiveness of Learning Law by Using Data Mining Analysis
This paper studies the motivation of learning law, compares the teaching effectiveness of two different teaching methods, e-book teaching and traditional teaching, and analyses the influence of e-book teaching on the effectiveness of law by using big data analysis. From the perspective of law student psychology, e-book teaching can attract students' attention, stimulate students' interest in learning, deepen knowledge impression while learning, expand knowledge, and ultimately improve the performance of practical assessment. With a small sample size, there may be some deficiencies in the research results' representativeness. To stimulate the learning motivation of law as well as some other theoretical disciplines in colleges and universities has particular referential significance and provides ideas for the reform of teaching mode at colleges and universities. This paper uses a decision tree algorithm in data mining for the analysis and finds out the influencing factors of law students' learning motivation and effectiveness in the learning process from students' perspective.
Intelligent Data Mining based Method for Efficient English Teaching and Cultural Analysis
The emergence of online education helps improving the traditional English teaching quality greatly. However, it only moves the teaching process from offline to online, which does not really change the essence of traditional English teaching. In this work, we mainly study an intelligent English teaching method to further improve the quality of English teaching. Specifically, the random forest is firstly used to analyze and excavate the grammatical and syntactic features of the English text. Then, the decision tree based method is proposed to make a prediction about the English text in terms of its grammar or syntax issues. The evaluation results indicate that the proposed method can effectively improve the accuracy of English grammar or syntax recognition.
Export Citation Format
Share document.
Data Mining: Data Mining Concepts and Techniques
Ieee account.
- Change Username/Password
- Update Address
Purchase Details
- Payment Options
- Order History
- View Purchased Documents
Profile Information
- Communications Preferences
- Profession and Education
- Technical Interests
- US & Canada: +1 800 678 4333
- Worldwide: +1 732 981 0060
- Contact & Support
- About IEEE Xplore
- Accessibility
- Terms of Use
- Nondiscrimination Policy
- Privacy & Opting Out of Cookies
A not-for-profit organization, IEEE is the world's largest technical professional organization dedicated to advancing technology for the benefit of humanity. © Copyright 2024 IEEE - All rights reserved. Use of this web site signifies your agreement to the terms and conditions.
Privacy-preserving data (stream) mining techniques and their impact on data mining accuracy: a systematic literature review
- Open access
- Published: 22 February 2023
- Volume 56 , pages 10427–10464, ( 2023 )
Cite this article
You have full access to this open access article
- U. H. W. A. Hewage ORCID: orcid.org/0000-0003-1293-9560 1 ,
- R. Sinha 1 &
- M. Asif Naeem 2
4923 Accesses
7 Citations
Explore all metrics
This study investigates existing input privacy-preserving data mining (PPDM) methods and privacy-preserving data stream mining methods (PPDSM), including their strengths and weaknesses. A further analysis was carried out to determine to what extent existing PPDM/PPDSM methods address the trade-off between data mining accuracy and data privacy which is a significant concern in the area. The systematic literature review was conducted using data extracted from 104 primary studies from 5 reputed databases. The scope of the study was defined using three research questions and adequate inclusion and exclusion criteria. According to the results of our study, we divided existing PPDM methods into four categories: perturbation, non-perturbation, secure multi-party computation, and combinations of PPDM methods. These methods have different strengths and weaknesses concerning the accuracy, privacy, time consumption, and more. Data stream mining must face additional challenges such as high volume, high speed, and computational complexity. The techniques proposed for PPDSM are less in number than the PPDM. We categorized PPDSM techniques into three categories (perturbation, non-perturbation, and other). Most PPDM methods can be applied to classification, followed by clustering and association rule mining. It was observed that numerous studies have identified and discussed the accuracy-privacy trade-off. However, there is a lack of studies providing solutions to the issue, especially in PPDSM.
Similar content being viewed by others
Privacy Preserving Data Mining: A Parametric Analysis
Privacy-Preserving Data Mining Techniques: Survey and Challenges
An Analysis of Privacy Preservation Techniques in Data Mining
Avoid common mistakes on your manuscript.
1 Introduction
Data Mining and machine learning involve extracting knowledge from data, which significantly impacts organizations’ growth. Organizations use their past and current data to make decisions to improve their performance or services, where data mining comes to play (Kiran and Vasumathi 2018 ). This process consists of mining helpful information from raw data and making predictions that support the decision-making (Dutta and Guppta 2016 ; Dhanalakshmi and Siva Sankari 2014 ). There are two main approaches for data mining and machine learning: supervised learning and unsupervised learning. These include techniques such as classification, clustering, and association rules (Kiran and Vasumathi 2018 ; Narwaria and Arya 2016 ). These methods identify data patterns and produce useful information and predictions that benefit organizations.
The success of the data mining process is measured using the accuracy of data mining results (Paul et al. 2021 ; Putri and Hira 2017 ). Accuracy depicts the percentage of patterns learned by the data mining process. For instance, in a classification task, accuracy can be computed as the percentage of correctly classified unknown data records over the total number of records (Nayahi and Kavitha 2017 ). Higher accuracy leads to improved decision-making. Some studies represent accuracy as “utility” (Feyisetan et al. 2020 ; Nayahi and Kavitha 2017 ; Denham et al. 2020 ; Tsai et al. 2016 ). Henceforth, we use the term accuracy for consistency.
One of the significant challenges stakeholders have to face in data mining is to protect the individual’s privacy in data while using those for data mining (Patel and Kotecha 2017 ). Datasets may contain some data that data owners do not want to reveal to the outside world (Bhandari and Pahwa 2019 ). This data is called sensitive data (Qi and Zong 2012 ). For example, patients’ medical history details from a hospital database or customers’ bank balance details from a banking database can be considered sensitive data. Sensitive data needs to be protected so that the privacy of the individuals can be preserved in the data mining process.
Defining privacy is not straightforward as accuracy. Privacy depends on the techniques and environment used to measure privacy. A more generic definition for privacy is proposed by Aggarwal and Yu ( 2008b ), which is "the degree of uncertainty according to which original private data can be inferred." Most currently using privacy-measuring metrics assume that some background knowledge of the original data is known to the attacker. The most commonly used method of measuring privacy is by performing attacks on perturbed data to recover original records. Breach probability is another commonly used measure of privacy that compares the error/difference between the original and recovered records with a threshold value. If the error is less than the threshold, it is identified as a breach of privacy (Giannella et al. 2013 ; Denham et al. 2020 ).
Privacy-Preserving Data Mining (PPDM) (Malik et al. 2012 ; Md Siraj et al. 2019 ; Carvalho and Moniz 2021 ) has been introduced as a solution to privacy concerns in data mining and has become a prominent area of data mining in the past few decades. PPDM methods should protect the privacy of the data while allowing the data mining process to carry out its duty as usual (Bhandari and Pahwa 2019 ; Carvalho and Moniz 2021 ). This means PPDM methods should not cause a considerable impact on the output of the data mining (Malik et al. 2012 ; Carvalho and Moniz 2021 ). Two broader categories of PPDM methods, called input PPDM and output PPDM, can be seen in the literature (Kotecha and Garg 2017 ; Peng et al. 2010 ). Input PPDM modifies original data before data mining to preserve privacy, while output PPDM deals with modifying the data mining output to preserve privacy. Our work focuses only on input PPDM methods as output PPDM methods mainly involve modifying data mining techniques (classifier or clustering algorithm) and need to be discussed separately. Different input privacy-preserving methods such as perturbation, anonymization, and encryption have been proposed and practiced in the data mining community. These methods positively and negatively impact both privacy and data mining tasks. However, the ultimate expectation of PPDM methods is to protect data privacy so that unauthorized parties cannot identify the individuals using data. And maintain the statistical properties of the data so that it does not degrade the performance of data mining (Denham et al. 2020 ; Lin et al. 2016 ; Chen and Liu 2011 ; Kabir et al. 2007a ). So that the transformed and protected dataset can be used for data mining without accessing the original dataset.
Data stream mining involves methods and algorithms to extract knowledge from volatile streaming data (Krempl et al. 2014 ). Combining privacy-preserving techniques in data stream mining is called Privacy-Preserving Data Stream Mining (PPDSM) Lin et al. ( 2016 ), Denham et al. ( 2020 ). Mining helpful information and making predictions from data streams have additional concerns due to the behaviour of data streams. Unlike static datasets, streaming data is continuous, transient, and unbounded that needs faster processing (Cao et al. 2011 ; Chamikara et al. 2018 ; Martínez Rodríguez et al. 2017 ). PPDSM methods must cater to the specific behaviour of data streams (Kotecha and Garg 2017 ; Chamikara et al. 2019 ), and therefore, privacy preservation in data streams needs to be addressed differently (Cuzzocrea 2017 ; Tayal and Srivastava 2019 ).
Most of the PPDM/PPDSM methods proposed have succeeded in preserving the privacy of data but negatively affect the data mining results (Chamikara et al. 2019 ; Kaur 2017 ). PPDM methods transform original data values into another form that makes them unrecognizable by outsiders. This process can destroy the statistical properties of the data useful in mining. Therefore, there is a trade-off between data privacy and data mining accuracy (Chen and Liu 2011 ). Increasing data privacy can decrease the data mining accuracy and vice versa (Paul et al. 2021 ). Current researches have identified this inherent trade-off between data privacy and data mining accuracy (use as accuracy-privacy trade-off hereafter) and have proposed different PPDM methods to address the issue (Kaur 2017 ; Wang and Zhang 2007 ; Soria-Comas et al. 2016 ; Babu and Jena 2011 ). Nevertheless, no perfect method has been found to optimize the accuracy-privacy trade-off, and the issue is still open to discussion.
Several existing works study PPDM and PPDSM, but we could not find secondary studies that discuss the accuracy-privacy trade-off in PPDM/PPDSM in detail. This study investigates existing PPDM methods, their strengths/weaknesses and applicable data mining tasks. Subsequently, we consider the unique challenges facing PPDSM and compare current techniques. This leads to a discussion on the accuracy-privacy trade-off and an assessment of how well current PPDM/PPDSM methods address this vital metric.
This systematic literature review makes the following contributions to the area of PPDM/PPDSM.
Indepth analysis of PPDM (Sect. 3.1 ) and PPDSM (Sect. 3.2.2 ) methods including techniques, strengths and weaknesses.
Analyzing the challenging nature of data streams and the impact on privacy preservation (Sect. 3.2.1 ).
Analyzing different accuracy and privacy evaluation metrics used in PPDM (Sect. 3.3.1 ) and PPDSM (Sect. 3.3.2 ) techniques.
Highlighting the importance of optimizing accuracy-privacy trade-off while investigating existing techniques used for the optimization (Sect. 3.3 ).
The remainder of this paper has been organized as follows. Section 2 describes the method we followed in carrying out this systematic literature review. We discuss the results and findings of the study in Sect. 3 . Finally, we discuss and conclude the knowledge gained from this study in Sects. 4 and 5 .
2 SLR protocol
This study has been carried out as a Systematic Literature Review (SLR), and the study’s primary goal is to evaluate methods and techniques proposed in the areas of PPDM and PPDSM. PPDM is a broad area that can be evaluated in many branches. We focus on evaluating PPDM’s applicability to data streams and its effect on the accuracy-privacy trade-off. The rest of this section explains the steps followed in conducting the SLR (Kitchenham et al. 2009 ).
2.1 Problem identification
Existing literature consists of a plethora of secondary studies in PPDM. Most of these studies (Dutta and Guppta 2016 ; Sharma and Ahuja 2019 ; Dhanalakshmi and Siva Sankari 2014 ; Md Siraj et al. 2019 ) summarize and evaluate the existing PPDM techniques while other studies talk about challenges and possible improvements for enhancing PPDM methods (Patel and Kotecha 2017 ; Abdul et al. 2015 ; Vishwakarma et al. 2016 ; Malik et al. 2012 ). Studies such as Kiran and Vasumathi ( 2018 ), Nasiri and Keyvanpour ( 2020 ), Dutta and Guppta ( 2016 ) present frameworks and categorizations of existing PPDM methods to provide the overall picture of the PPDM methods.
Though there are numerous studies on PPDM, only a few are focused on the application of PPDM in data stream mining. Research work such as Tayal and Srivastava ( 2019 ), Gomes et al. ( 2019 ), Cuzzocrea ( 2017 ), Krempl et al. ( 2014 ) discuss the challenges, opportunities, and possible future directions in privacy-preserving data stream mining. However, to the best of our knowledge, we could locate only one study (Sakpere and Kayem 2014 ) that discusses existing PPDM methods specifically for data streams. A few studies (Tran and Hu 2019 ; Sangeetha and Sadasivam 2019 ) discuss PPDM methods that can be used in big data in general and have the potential of being used in data streams as it is a category of big data. Therefore, proper evaluation of PPDM methods for data streams is necessary.
The well-known accuracy-privacy trade-off is a concern that still needs to more attention, and a considerable number of studies (Qi and Zong 2012 ; Narwaria and Arya 2016 ; Patel and Kotecha 2017 ; Jain et al. 2016 ) have identified this issue. But very few (Malik et al. 2012 ; Vishwakarma et al. 2016 ; Shanthi and Karthikeyan 2012 ) have discussed this in detail with respect to different PPDM methods. We find the accuracy-privacy trade-off as an aspect that needs to be discussed, considering both static datasets and data streams.
By analyzing the existing secondary studies, we could confirm a lack of studies that discuss the accuracy-privacy trade-off in PPDM and the existing PPDM techniques specifically for data stream mining. The motivation for our research work arises from this gap, and we try to address these issues in this comprehensive study.
2.2 Research questions
After identifying gaps in existing secondary studies related to PPDM, we started our SLR by formulating three Research Questions (RQ) to address those gaps.
RQ1: What are the existing privacy-preserving data mining methods?
RQ1.1: What are the strengths and weaknesses of the investigated methods?
RQ1.2: What data mining tasks can these methods be used for?
RQ2: What is the nature of privacy preservation in data stream mining?
RQ2.1: What challenges can be identified when applying PPDM methods for data stream mining?
RQ2.2: What are the PPDM methods that have been proposed for data stream mining?
RQ3: To what extent do the privacy-preserving data mining approaches identified in answering RQ1 and RQ2 address the trade-off between data privacy and classification accuracy, and what methods have been proposed to optimize the accuracy-privacy trade-off?
Concerning RQ1, we summarize the most common PPDM methods used in the data mining community. To provide a broader insight into existing PPDM methods, we discuss the merits and demerits of each method, along with the data mining tasks they can be used for under the sub-questions of RQ1.
Under RQ2, we discuss the applicability of PPDM methods identified in RQ1 in data streams and the different PPDSM methods proposed specifically for data stream mining. Here we also try to identify the challenges in applying PPDM methods in data stream mining as data streams behave differently than static datasets.
By answering RQ3, we aim to determine whether the accuracy-privacy trade-off has received the attention it deserves, as it is a severe concern in the area. We investigate whether the authors have identified or discussed the above issue and the possible steps they have proposed or implemented to reduce the trade-off.
2.3 Search process
A systematic manual search was conducted to find out the potential research work. First, we identified search keywords to initiate our search. The search was conducted using three sets of keywords to reduce the complexity of the searching process.
Set 1: (PPDM OR accuracy-privacy trade*)
Set 2: (PPDM AND utility)
Set 3: (data stream) AND (privacy OR PPDM)
Set 1 focuses on selecting articles on privacy-preserving data mining, which may or may not include a discussion about the accuracy-privacy trade-off. We considered the articles with the term “utility” together with PPDM in Set 2, as some researchers prefer using “utility” instead of “accuracy,” and those terms are being used interchangeably in the literature. Set 3 selects data stream mining articles about PPDM or privacy.
Five major databases were selected to search: Scopus, IEEE, Science Direct, Springer, and ACM. The initial search was carried out to filter out the potential research work using the search strings mentioned above and the studies using the inclusion and exclusion criteria mentioned in the next section.
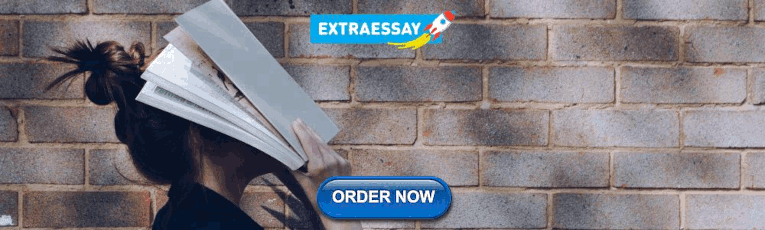
2.4 Inclusion Criteria (IC) and Exclusion Criteria (EC)
Running the three sets of keywords through five databases resulted in 3923 studies. The following IC and EC were used to select the relevant studies manually to address the formulated research questions.
Inclusion Criteria (IC)
IC1- Studies propose PPDM techniques for general data mining tasks.
IC2- Studies that discuss the challenges of applying PPDM in data streams.
IC3- Studies that only focus on the privacy aspect of PPDM.
IC4- Studies propose PPDM techniques specifically for data stream mining.
IC5- Studies that mainly focus on privacy-accuracy trade-off (though it is only for a specific application)
Exclusion Criteria (EC)
EC1- Studies do not have a proper evaluation.
EC2- Studies that lack full details of the implementation and context of the proposed methods.
EC3- Studies that do not carry out with relevant experimentation.
EC4- Studies that propose frameworks/conceptual models using existing PPDM methods.
EC5- Studies that only focus on a specific application/area/ or studies with limited usage.
EC6- Survey articles/secondary studies.
EC7- Studies that discuss the impact of PPDM on different industries.
EC8- Studies that only discuss/propose privacy breaching methods.
EC9- Duplicate research articles.
EC10- Studies that have new/improved versions.
Using this process, we made sure that all the relevant studies were included and irrelevant studies were excluded to increase the effectiveness of the SLR.
2.5 Search execution
Figure 1 illustrates the process of selecting the primary studies for SLR. The above-mentioned IC and EC were applied in different steps to filter out the articles that were out of scope considering the defined research questions. This process was carried out manually. For example, after selecting the potential articles from the initial search as the first step, IC1, IC3, EC5, EC6, EC7, and EC8 were applied as the second step. After this, the remaining number of articles could be reduced to 1930. The process was repeated until the most relevant articles remained, which turned out to be 114. We only considered the studies published in the last 20 years (2002–2022), as most studies related to PPDM were published after 2001.
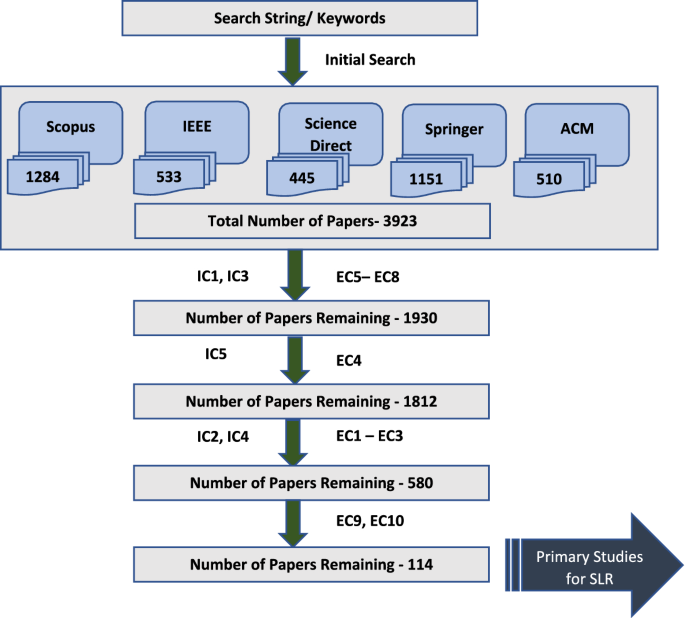
Search execution process, demonstrating all the steps followed to filter out the research articles for SLR
2.6 Data extraction and analysis
Data was extracted from each selected article by thoroughly reading the abstract and conclusion and skimming through the rest of the text. The following data was collected.
Title of the study
Year of publication
PPDM technique/method proposed
Strengths and weaknesses of the proposed method/technique
Accuracy and privacy evaluation metrics
Applicable data mining tasks
Applicability to data streams
Challenges identified on applying PPDM to data streams
Discussion on accuracy-privacy trade-off
Collected data were stored and analyzed using MS. Excel to answer the formulated research questions. Figure 2 shows the distribution of the studies selected according to the year of publication. We can observe that many related studies have been published from 2010 to 2021 than between 2000 and 2010. The number of studies published yearly has steadily risen in the last decade.
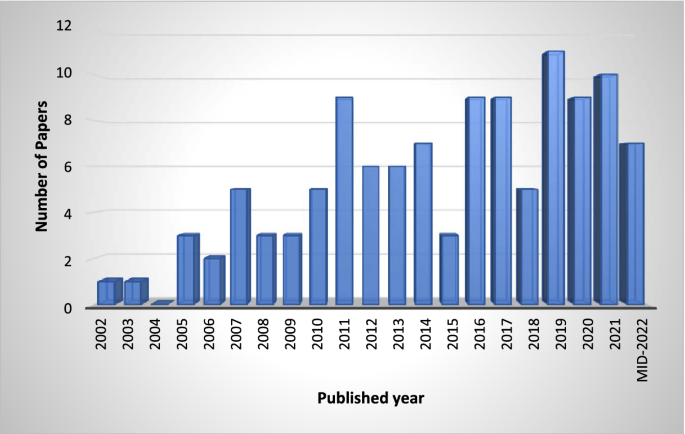
Distribution of the selected studies according to the year of publication
This section summarizes the results and findings of our SLR for each research question.
3.1 Addressing RQ1—Generic PPDM methods
All the different PPDM techniques and methods found in the final set of articles were studied to answer RQ1 and its sub-questions. There are many categorizations of PPDM proposed in the literature. Authors of Arumugam and Sulekha ( 2016 ), Rajalakshmi and Mala ( 2013 ) categorized PPDM techniques into two main categories, called Secure Multi-Party Computation and perturbation . In Kaur ( 2017 ), PPDM has been divided into five categories namely, Anonymization, Perturbation, Randomization, Cryptography and Condensation .
According to the analysis of extracted data, we agree with the categorization proposed in Tran and Hu ( 2019 ) as we believe it is more generic and justifiable. Therefore, we divide existing input PPDM techniques into four main categories: Secure Multi-Party Computation, perturbation methods, non-perturbation methods, and combinations of the above techniques by extending the categorization provided by Tran and Hu ( 2019 ). This section discusses all the techniques included in these four categories in detail.
3.1.1 Secure Multiparty Computation (SMC)
The Secure Multi-Party Computation (SMC) methods are being used for collaborative data mining and use cryptographic tools to protect data (Rajalakshmi and Mala 2013 ). It allows different parties to jointly compute a certain functionality without revealing personal data (Tran and Hu 2019 ). Therefore, cryptographic methods can be used for distributed privacy and information sharing. This became popular as it provides a well-defined privacy model and the methods for proving and quantifying (Sachan et al. 2013 ). However, there is a concern that the cryptographic techniques do not protect the output privacy; instead, they stop the leakage of sensitive data in the computation process (Sachan et al. 2013 ). The data mining community prefers perturbation techniques over SMC techniques because of their lower computational complexity (Chamikara et al. 2021 , 2019 ). Cryptographic methods use encryption schemes that are challenging in scalability and implementation efficiency (Tran and Hu 2019 ). However, some improved encryption methods such as Park et al. ( 2022 ) and Dhinakaran and Prathap ( 2022b ) have been implemented recently with less computational complexity and execution time.
3.1.2 Perturbation methods
This section discusses data perturbation methods that distort data values in specific ways to hide sensitive information while maintaining data properties important for data mining (Chen and Liu 2011 ). Data perturbation is the most commonly used privacy-preserving technique in data mining because of its simplicity and computational efficiency. In Rajalakshmi and Mala ( 2013 ), perturbation has been identified as altering data using statistical methodologies. However, Data perturbation methods have to pay special attention to the accuracy of data mining, as distorting data can highly affect the data mining process. Perturbation can be divided into the value alternation approach and the probability distribution approach (Chidambaram and Srinivasagan 2014 ). This section discusses the techniques that can be considered data perturbation methods.
Using noise to distort the data is one of the earliest data perturbation methods (Denham et al. 2020 ). Additive and multiplicative noise are the two main usages of noise in the PPDM context (Chidambaram and Srinivasagan 2014 ). Random values with zero mean and a specified variance are generated from a given distribution, such as Gaussian or Uniform distribution. Generated noise values are added to each record in additive noise environment, while each record is multiplied with the noise values in multiplicative environment (Denham et al. 2020 ; Chidambaram and Srinivasagan 2014 ; Kim and Winkler 2003 ). The original data values are distorted, while the underlying data distribution can be reconstructed (Kim et al. 2012 ). If the variance of added noise is high, then a high level of privacy can be expected, but it also causes a high information loss. Later, a combined version of additive and multiplicative noise was proposed in Chidambaram and Srinivasagan ( 2014 ). This combined approach guarantees more privacy than individual approaches.
Keke and Ling (Chen and Liu 2005 ) first proposed a geometric transformation method named random rotation for PPDM-based classification. The original dataset with m attributes is multiplied using a (m x m) random orthogonal matrix (Denham et al. 2020 ) perturbing all the attributes together (Chen and Liu 2005 ). A rotation-based approach that only transforms sensitive attributes is proposed in Ketel and Homaifar ( 2005 ). Perturbation using rotation transformation is vulnerable to rotation center attacks (Ketel and Homaifar 2005 ; Chen and Liu 2005 ), as data closer to the origin is less perturbed than the other data records (Denham et al. 2020 ). Recently, more improved versions of random rotation, such as 3-D rotation transformation (Upadhyay et al. 2018 ) and 4-D rotation transformation (Javid and Gupta 2020 ), have been proposed, and these methods assure high data mining accuracy.
Other geometric data perturbation methods that combine random rotation, translation, and noise addition have been proposed to minimize the vulnerabilities accompanied by rotation transformation (Chen and Liu 2011 ; Chen et al. 2007 ). These methods became robust to rotation centre attacks by adding a translation and to distance inference attacks by adding noise. However, it can still be vulnerable to background knowledge-related attacks (Chen et al. 2007 ).
Differential privacy (Dwork 2008 ) is a high privacy guaranteed algorithm that works by adding Laplace noise to statistical databases. It ensures that an outsider cannot determine if a data item has been altered. According to Dwork ( 2008 ), the result of the dataset is insensitive to the change of a record. Hence makes it difficult for an attacker to gain knowledge about data. Research work such as Mivule et al. ( 2012 ), Tang et al. ( 2019 ) discuss research work using differential privacy as the PPDM technique.
Tables 1 and 2 summarize different PPDM techniques in noise injection, rotation, differential privacy and other geometric transformations, along with the strengths and weaknesses.
Random Projection (RP) based multiplicative data perturbation was proposed in Liu et al. ( 2006 ). RP projects a given dataset from a higher-dimensional space to a lower-dimensional subspace. This method is based on the Johnson–Lindenstrauss Lemma, and pair-wise distances of any two data points can be maintained within a small range (Liu et al. 2006 ; Denham et al. 2020 ). So, it can be considered an approximate distance preserving method. The authors of Liu et al. ( 2006 ) have stated that RP can be more powerful when used with geometric transformation techniques such as scaling, rotation, and translation. Recently, a random projection-based noise addition method was proposed in Denham et al. ( 2020 ). This method experimentally proved high accuracy and privacy levels by combining RP, translation, and noise addition.
Condensation can also be considered as a perturbation PPDM method. It condenses data records into groups of pre-defined size k while maintaining statistical properties within the group (Aggarwal and Yu 2004 ). It is not possible to distinguish one record from another within the group. Then pseudo data is generated instead of original data using the statistical information within the group. Condensation maintains inter-attribute correlations that guarantee a high accuracy level (Aggarwal and Yu 2004 , 2008a ).
Few works such as Meghanathan et al. ( 2014 ), Jahan et al. ( 2016 ), Cano et al. ( 2010 ) have considered using fuzzy logic-based techniques for data perturbation. A fuzzy logic-based perturbation method with less processing time has been proposed in Meghanathan et al. ( 2014 ). Though the method’s accuracy is similar to the accuracy of the original dataset, privacy needs to be evaluated. A multiplication perturbation method using fuzzy logic has been implemented in Jahan et al. ( 2016 ). This method has achieved better accuracy and privacy levels for classification and clustering. Another work that uses fuzzy models for synthetic data generation as a perturbation method can be found in Cano et al. ( 2010 ).
Table 3 gives an overall idea about different techniques in random projection, condensation, fuzzy logic and some other distortion methods in PPDM.
A considerable number of PPDM methods combine different transformations and data distortion methods to achieve a better performance, considering both privacy and accuracy. Some research work (Peng et al. 2010 ; Nethravathi et al. 2016 ; Li and Wang 2011 ; Putri and Hira 2017 ; Wang and Zhang 2007 ; Xu et al. 2006 ; Hasan et al. 2019 ; Li and Xue 2018 ) use transformation techniques such as Singular Value Decomposition (SVD), Non-negative Matrix Factorization (NMF) and Discrete Wavelet Transformation (DWT) to perturb data. In Gokulnath et al. ( 2015 ), authors have used Principal Component Analysis (PCA) while PCA, together with noise addition, has been used in Mukherjee et al. ( 2008 ) for data perturbation. These methods can be summarised in Table 4 .
3.1.3 Non-perturbation methods
Non-perturbation methods sanitize the identifiable information to preserve privacy (Tran and Hu 2019 ), and different anonymization techniques are included in this category. Non-perturbation methods modify or remove only a portion of data (Vijayarani and Tamilarasi 2013 ), whereas perturbation methods distort each data value. This process uses techniques to make a single record indistinguishable from another set of a specified number of records so that individual records cannot be identified and privacy is preserved. We discuss a set of non-perturbation-based PPDM methods in this section.
Anonymization is the most used non-perturbation technique that involves identifying different parts of a data record, such as Identifiers, Quasi-identifiers, and Sensitive and Non-sensitive attributes. Then it removes identifiers and modifies quasi-identifiers by performing techniques such as generalization and suppression, making a record indistinguishable from a set of other records (Tran and Hu 2019 ). Different anonymization methods can be seen in the literature, such as k -anonymity (Sweeney 2002 ), l -diversity (Machanavajjhala et al. 2007 ) and t -closeness (Li and Venkatasubramanian 2007 ).
The basic method of anonymization, k -anonymity, ensures that a single data record cannot be distinguished from at least k-1 records (Sweeney 2002 ; Tsai et al. 2016 ). Identifying different parts of a data record is essential here, and then applying generalization and suppression techniques to achieve k -anonymized set of data. This method reduces the risk of a re-identification attack caused by the direct linkage of shared attributes (Tsai et al. 2016 ). The main weakness of the method is that it assumes that no two tuples contain data of the same person, which may not always be true (Sweeney 2002 ).
Another weakness of k -anonymity is that it can be vulnerable to background knowledge-based attacks such as complementary release attacks and Temporal inference attacks. As a solution to this, an improved anonymization model called l -diversity was introduced (Machanavajjhala et al. 2007 ). A table is called l -diverse if there are l well-represented values for the sensitive attribute (Wang et al. 2009 ). The method provides privacy even when the data owner does not know what kind of knowledge the attacker has. However, it is difficult to implement for multiple sensitive attributes (Machanavajjhala et al. 2007 ) and vulnerable to attacks such as similarity attacks (Wang et al. 2009 ).
Another anonymization method named t -closeness was proposed in Li and Venkatasubramanian ( 2007 ). The requirement to achieve t -closeness is maintaining the distribution of a sensitive attribute in an equivalence closer to the distribution of the same attribute in the overall table. If the distance between two distributions less than the threshold t , it has achieved the t -closeness (Li and Venkatasubramanian 2007 ; Soria-Comas et al. 2016 ). This overcomes the skewness and similarity attacks but cannot deal with identity disclosure attacks and multiple sensitive attributes (Li and Venkatasubramanian 2007 ). There are several more variations of anonymization such as p-sensitive, t -closeness (Sowmyarani et al. 2013 ) have been proposed in addition to these main methods as solutions to privacy issues of the existing methods.
The main issue with all these anonymization methods is that there is no specific computational approach to determine what data should be anonymized. This entirely depends on the expertise knowledge (Sowmyarani et al. 2013 ). Different anonymization techniques, along with their strengths and weaknesses, can be found in Table 5 .
3.1.4 Methods combining cryptographic, perturbation and non-perturbation techniques
Privacy-Preserving Data Mining methods that use different combinations of the above-discussed techniques are being used to preserve privacy. The reason for proposing these combing methods is to use the benefits of each method by reducing or eliminating the weaknesses.
In Kaur ( 2017 ), authors have proposed a hybrid PPDM method by combining perturbation and anonymization. This method uses additive noise and suppression techniques to achieve a minimum loss by avoiding the generalization involved in the anonymization. An improved PPDM method was proposed in Poovammal and Ponnavaikko ( 2009 ) to implement privacy separately according to the data owners’ willingness. This method combines transformation and anonymization. The hybrid approach implemented in Lohiya and Ragha ( 2012 ) uses randomization and generalization techniques to achieve better accuracy. Randomization and generalization are involved with the perturbation and non-perturbation methods, respectively. Authors of Deivanai et al. ( 2011 ) propose a PPDM method by combining suppression and perturbation techniques. This method performs suppression only on specific attributes, leading to a minimum loss of information. A hybrid multi-group approach proposed in Teng and Du ( 2009 ) uses randomization and SMC techniques together to achieve high accuracy and efficiency.
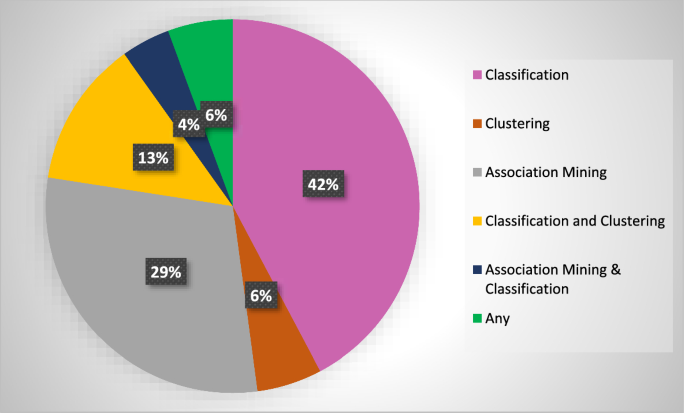
Distribution of generic PPDM methods according to the applicability of different data mining tasks (These percentages are derived according to the number of studies covering generic PPDM methods from the total number of papers reviewed)
The existing PPDM methods consist of techniques that can be used with most supervised (Classification and Regression) and unsupervised (Clustering and Association Rule Mining) learning algorithms. Methods in Chen et al. ( 2007 ), Chen and Liu ( 2005 ), Kim et al. ( 2012 ), Sun et al. ( 2014 ), Kumar and Premalatha ( 2021 ) can be applied to clustering methods such as Decision Tree (DT), Naive Bayes (NB), K-Nearest Neighbor (KNN), Multi-Layer Perceptron (MLP) and Support Vector Machines (SVM). PPDM methods such as Cano et al. ( 2010 ), Vijayarani and Tamilarasi ( 2011 ), Gokulnath et al. ( 2015 ), Upadhayay et al. ( 2009 ) can be used for clustering, while methods like Cheng et al. ( 2014 ), Xiaoping et al. ( 2020 ), Suma and Shobha ( 2021 ) are suitable for Association Rule Mining. There are improved methods (Aggarwal and Yu 2004 , 2008a ; Li and Xue 2018 ; Meghanathan et al. 2014 ) that can be used for more than one data mining task. Table 6 briefs the PPDM methods that combine perturbation, non-perturbation and cryptographic techniques.
The distribution of PPDM methods among data mining tasks can be seen in Fig. 3 . Most PPDM methods can be applied to classification, followed by association rule mining. While comparably fewer methods have been proposed specifically for clustering algorithms, a considerably high number of PPDM methods can be used for more than one data mining task (classification and clustering, classification and association rule mining). Moreover, some PPDM methods can be used with any data mining algorithm.
For PPDM methods, we have reviewed their strengths and weaknesses. Most methods are vulnerable to attacks related to background knowledge, and the researchers have identified this problem. Though we cannot provide a simplified categorization of strengths and weaknesses, we have pointed out the strengths and weaknesses of the reviewed methods in Tables 1 , 2 , 3 , 4 , 5 and 6 . These tables provide a comprehensive answer to the sub-questions in RQ1 by summarizing all the different PPDM techniques we found and the strengths, weaknesses, and challenges.
3.2 Addressing RQ2—Privacy-Preserving Data Stream Mining (PPDSM)
This section discusses the PPDM methods that can be applied to data streams and the challenges we have to overcome when successfully applying PPDM methods to data streams.
3.2.1 Challenges in data stream mining
Most generic PPDM methods discussed in Sect. 3.1 cannot be directly applied to data streams due to the challenging behavior. There are three principal challenges in mining data streams named volume, velocity, and volatility (Krempl et al. 2014 ; Tran and Hu 2019 ). Data streams have numerous challenges to consider, such as data preprocessing, analyzing complex data, dealing with delayed data, and handling concept drift (Krempl et al. 2014 ; Gomes et al. 2019 ). Privacy is only one concern that data stream mining has to focus on.
Data streams are continuous, transient, and usually unbounded (Wang et al. 2007 , 2018 ; Martínez Rodríguez et al. 2017 ) in nature. Mining data streams is a continuous process, and it cannot be redone as done for the static datasets because it is not possible to access the full set of data at once Khavkin and Last ( 2019 ). Data may reach a high speed, and therefore fast execution is needed (Chamikara et al. 2018 ; Lin et al. 2016 ). Privacy preservation needs to be performed quickly, and incoming data should be released with a minimum delay. Due to the unbounded nature of the data streams, PPDM methods should be able to cope with a massive volume of data with a fast execution time (Denham et al. 2020 ). Computer memory is too small relative to the vast data volume, and all data cannot be stored (Wang et al. 2007 ). Another challenge of data streams mining is the concept-drift (Cuzzocrea 2017 ; Gomes et al. 2019 ; Tayal and Srivastava 2019 ) and it affects the PPDM process. Underlying data distribution can change with time, and data mining models should be able to adapt to the concept drift to achieve a good accuracy level (Zhang and Li 2019 ; Khavkin and Last 2019 ). Privacy preservation methods should be able to cope with the effects of the concept drift.
Considering all these facts, data stream mining and privacy preservation are two conflicting tasks (Kotecha and Garg 2017 ). The data stream mining should quickly cope with the memory restrictions, while generic privacy preservation methods require multiple scans over the data, which is time and memory-consuming.
3.2.2 PPDM methods for data stream mining
The possibility of applying proposed methods to data streams or difficulties of adapting the methods to data streams have not been discussed in generic PPDM methods except in a few. Authors of Kadampur and Somayajulu ( 2008 ) have mentioned that the proposed field rotation and binning method cannot be applied to data streams. All data should be presented to the binning process, which cannot be done for data streams because of their incremental behavior. The combined noise perturbation method proposed in Chidambaram and Srinivasagan ( 2014 ) is also challenging to adapt to data streams. According to the authors, the concept of multi-level trust used in this combined perturbation method is challenging to implement for data streams. The condensation-based PPDM method proposed in Aggarwal and Yu ( 2008a ) is the only method we could find from the selected articles that discuss the possibility of applying the method for data streams. It is suitable for both static data and dynamic data streams. However, for infinite data streams, there is a need for a mechanism to store a fixed number of condensed groups (Aggarwal and Yu 2008a ).
PPDSM methods implemented specifically for data streams and modified versions of generic PPDM methods for use in data streams can be seen in the literature. These PPDSM methods have been designed to overcome the above-discussed common challenges of the data streams. We divided these methods into Perturbation, Non-perturbation (Anonymization), and others, based on the main techniques they used. Most methods are based on anonymization-based non-perturbation methods followed by perturbation methods. A small proportion of PPDSM methods use different other distortion techniques such as differential privacy, fuzzy logic, and PCA. Though we roughly categorize these methods into these categories, we observed that there is no clear boundary to define this. Most of these methods are combinations of different categories.
Anonymization-based non-perturbation methods are among the most used PPDSM techniques for data stream mining. An anonymization method called FAST was presented in Mohammadian et al. ( 2014 ) for the fast execution of privacy preservation in data streams with less information loss. This method uses a multithreading technique through k -anonymization and can be used for clustering. Another PPDM method for clustering using k -anonymization for data streams has been introduced in Mohamed et al. ( 2017 ). This method is scalable and can be used with less communication cost and less information loss for distributed data streams. A continuously anonymizing method called “CASTLE” has been implemented using k -anonymity and l -diversity in Cao et al. ( 2011 ). CASTLE can manage outliers and release data with a minimum delay but can be vulnerable to inference-related attacks. Microaggregation-based differential private anonymization has been proposed for classification in Khavkin and Last ( 2019 ). This method deals with concept drift by applying Kolmogorov–Smirnov statistical test and minimizes the information loss and possible disclosure risks. The privacy preservation method discussed in Rajalakshmi and Mala ( 2013 ) uses a frequency discretization technique similar to anonymization. Moreover, sliding window-based anonymization methods were discussed in Wang et al. ( 2018 , 2007 ), Navarro-Arribas and Torra ( 2014 ). The fast anonymization method proposed in Wang et al. ( 2018 ) can be used for associate rule mining, and the method in Wang et al. ( 2007 ) facilitates high-speed data processing with small memory requirements. Anonymization based on rank swapping in a sliding window discussed in Navarro-Arribas and Torra ( 2014 ) can reduce information loss by swapping selected tuples from the sliding window but can be impractical for infinite data streams.
Perturbation based privacy preservation methods proposed for data stream mining are Chamikara et al. ( 2018 ), Martínez Rodríguez et al. ( 2017 ), Virupaksha and Dondeti ( 2021 ), Denham et al. ( 2020 ), Rajalakshmi and Mala ( 2013 ). In Chamikara et al. ( 2018 ), “P2RoCAl”, a combination of Condensation, rotation, and random swapping has been proposed for data stream classification. P2RoCAl offers a better accuracy level than similar methods and is robust to data reconstruction attacks, but condensed group size can affect the performance. It can be used in static environments as well. Statistical Conflict of interest Control (SDC) with different filters such as noise addition, micro-aggregation, rank swapping, and differential privacy has been used in Martínez Rodríguez et al. ( 2017 ). However, the noise addition filter has the risk of disclosure. An anonymization method based on noise addition for privacy preservation has been proposed in Virupaksha and Dondeti ( 2021 ) for clustering. This method chooses random noise within the subspace limits of the dense and non-dense subspaces to reduce information loss and enhance cluster identification. Random projection-based cumulative noise addition implemented in Denham et al. ( 2020 ) combines three perturbation techniques, random projection, translation, and noise addition, to achieve good accuracy and privacy. In addition to traditional independent noise addition, authors of Denham et al. ( 2020 ) have introduced a novel noise addition method that seems promising in performance. A random projection-based encryption method discussed in Rajalakshmi and Mala ( 2013 ) provides a low computational cost with a good privacy level.
Other privacy-preserving methods proposed for data stream mining use different techniques such as fuzzy logic and PCA (Rajesh et al. 2012 ), differential privacy (Chamikara et al. 2019 ; Katsomallos et al. 2022 ; Gondara et al. 2022 ), sliding window (Lin et al. 2016 ), and hashing (Nyati et al. 2018 ). These methods try to overcome the challenges in data stream mining by using different techniques. We observed that there are numerous methods proposed for PPDSM that fall under the category of output PPDSM (Kotecha and Garg 2017 ; Zhang and Li 2019 ), which is out of our scope.
Figure 4 provides an idea of how data stream-based PPDM methods are spread over different data mining tasks. Like the generic PPDM methods, most PPDSM can be applied to classification. The second-highest applicability was achieved by clustering. A few PPDSM methods have been proposed specifically for association rule mining. Some PPDSM methods can be applied to more than one data mining algorithm, which is a good sign.
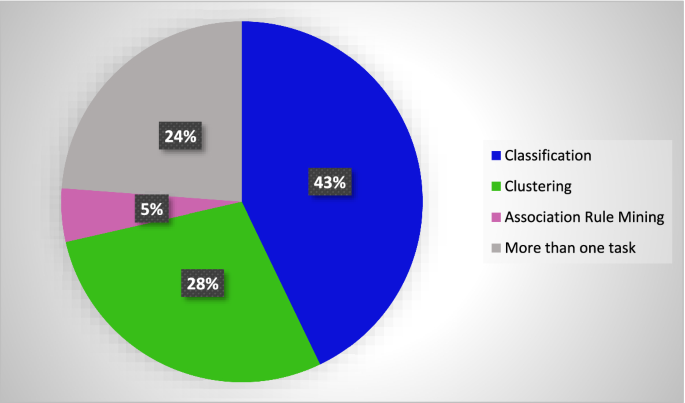
Distribution of PPDSM methods according to the applicability on different data mining tasks (These percentages are derived according to the number of studies covering PPDSM methods from the total number of papers reviewed)
3.3 Addressing RQ3—accuracy-privacy trade-off
The accuracy-privacy trade-off is the most common issue in PPDM/PPDSM and should be addressed appropriately to get maximum performance. If not, the objective of PPDM methods, which is effectively protecting private data while maintaining the knowledge in original data (Lin et al. 2016 ; Denham et al. 2020 ), can be violated. It was observed that lots of researchers have identified and discussed this trade-off, while some studies try to provide possible solutions. In this section, by answering RQ3, we discuss to what extent the accuracy-privacy trade-off has been addressed.
3.3.1 Accuracy-privacy trade-off in generic PPDM methods
Metrics of accuracy and privacy are helpful when understanding the trade-off between those two properties. Tables 7 and 8 compile different evaluation metrics and measures used to calculate privacy and accuracy for generic PPDM methods. The most commonly used measure of accuracy is the error/accuracy of the data mining task. A few privacy preservation methods, such as anonymization and rule hiding, use different techniques to measure accuracy. Differential privacy and privacy after various attacks are the most used methods. Moreover, some methods have used metrics such as VD, RP, RK, CP and CK \(^{*}\) to measure privacy. However, we can see that measuring privacy and accuracy are mostly specific to data mining and privacy preservation techniques.
Regarding the accuracy-privacy trade-off discussion, We first look at the generic PPDM methods discussed in Sect. 3.1 .
Research work such as (Putri and Hira 2017 ; Vijayarani and Tamilarasi 2011 ; Lohiya and Ragha 2012 ; Alotaibi et al. 2012 ; Upadhyay et al. 2018 ; Chamikara et al. 2020 ; Kiran and Vasumathi 2020 ; Tsiafoulis et al. 2012 ; Arumugam and Sulekha 2016 ) have identified the existing accuracy-privacy trade-off while (Zaman et al. 2016 ; Peng et al. 2010 ; Nethravathi et al. 2016 ; Chidambaram and Srinivasagan 2014 ; Javid and Gupta 2020 ; Liu et al. 2019 ; Sowmyarani et al. 2013 ; Xiaoping et al. 2020 ; Sun et al. 2014 ; Aggarwal and Yu 2008a ; Upadhayay et al. 2009 ) discuss the matter in detail. Accuracy-privacy trade-off w.r.t. rotation perturbation has been discussed with extensive experimental results in Chen and Liu ( 2005 ). Authors of Giannella et al. ( 2013 ) have discussed the nature of this trade-off in the distance preserving PPDM methods with examples. Accuracy-privacy behavior of p -sensitive, t -closeness was discussed in Sowmyarani et al. ( 2013 ). In this method, When p decreases, utility also decreases, but good in high t values. Accuracy-privacy trade-off of additive multiplicative perturbation has been discussed in Teng and Du ( 2009 ). This method shows that the error and privacy increase when the trust level increases, which denotes a trade-off between accuracy and privacy.
Numerous research work has proposed different solutions to optimize or reduce the accuracy-privacy trade-off. Combining suppression and perturbation to minimize the loss caused by generalization in anonymization has been proposed in Kaur ( 2017 ). Performing the perturbation only on sensitive attributes to achieve a high accuracy level while maintaining good privacy using NMF and SVD has been discussed in Wang and Zhang ( 2007 ). The t -closeness anonymization proposed in Li and Venkatasubramanian ( 2007 ) discuss how the t parameter can be tuned to achieve a good trade-off, while (Soria-Comas et al. 2016 ) tries to achieve a better trade-off by applying t -closeness through micro-aggregation. Anonymization-based clustering methods proposed in (Nayahi and Kavitha 2017 ; Babu and Jena 2011 ) shows that the number of clusters formed determines the trade-off as the number of clusters increases, accuracy increases, and privacy decreases. Authors of Mukherjee et al. ( 2008 ) try to achieve a better accuracy-privacy trade-off by combining PCA and additive noise, but privacy increases while accuracy decreases when more noise is added. The differential privacy-based approach proposed in Mivule et al. ( 2012 ) uses an ensemble classifier to calculate the error, which is repeated until a pre-defined threshold is achieved. The Laplace noise added for the differential privacy is re-adjusted if it cannot be achieved.
A rotational transformation was combined with a translation implemented in Singh and Batten ( 2013 ) to get a good privacy level with a low accuracy loss. Authors of Teng and Du ( 2009 ) try to balance the trade-off between accuracy and privacy using a multi-group approach. The perturbation method “NRoReM” (Paul et al. 2021 ) has been implemented to optimize the accuracy-privacy trade-off by combining normalization, geometric rotation, linear regression, and scalar multiplication. In addition to the above discussed methods, research work such as Feyisetan et al. ( 2020 ); Hasan et al. ( 2019 ); Kabir et al. ( 2007a ); Li and Xue ( 2018 ); Kim et al. ( 2012 ) and Chamikara et al. ( 2021 ) also implemented different techniques to optimize the accuracy-privacy trade-off.
Some research work have proposed interesting PPDM techniques but have not paid much attention to the accuracy-privacy trade-off (Tsai et al. 2016 ; Tang et al. 2019 ; Ashok and Mukkamala 2011 ; Hong et al. 2010 ; Meghanathan et al. 2014 ; Li and Wang 2011 ; Kaur and Bansal 2016 ; Gokulnath et al. 2015 ; Oishi 2017 ; Lin et al. 2015 ; Miyaji and Rahman 2011 ; Ketel and Homaifar 2005 ; Hong et al. 2011 ).
3.3.2 Accuracy-privacy trade-off in data stream mining
Table 9 presents evaluation metrics used to measure accuracy and privacy in data streaming environments. Information loss and classification accuracy are the most frequently used accuracy evaluation metrics. Differential privacy and calculating breach probability by performing attacks can be identified as the most commonly used privacy measures in data stream mining environments.
Some PPDM methods proposed for data streams have also attempted to optimize the accuracy-privacy trade-off. According to the challenges identified in 3.2.1 , it is clear that handling the trade-off issue in the streaming environment is rather complex. However, some methods have discussed this issue (Gitanjali et al. 2010 ; Lin et al. 2016 ; Cao et al. 2011 ) while some have tried to address it using different techniques (Zhang and Li 2019 ; Khavkin and Last 2019 ; Chamikara et al. 2019 , 2020 ; Denham et al. 2020 ).
Sequential Backward Selection (SBS) of the greedy algorithm and k-fold cross-validation to select the optimal mode in NB classification has been used in Zhang and Li ( 2019 ) to achieve a balanced accuracy-privacy trade-off. Micro-aggregation based differential private stream anonymization has been proposed in Khavkin and Last ( 2019 ), and the trade-off has been evaluated using disclosure risk and Area Under the Curve (AUC) of the classifier. The differential privacy-based PPDM method “SEALdou” Chamikara et al. ( 2019 ) has been proposed as a solution to the accuracy-privacy trade-off in data stream mining. To optimize the trade-off, it provides flexibility to select privacy parameters according to the domain and dataset to improve privacy and maintain the shape of the original data distribution after noise addition to improve accuracy. P2RoCAl (Chamikara et al. 2020 ) tries to achieve the same goal by combining condensation and rotation. Random projection-based cumulative noise addition (Denham et al. 2020 ) tries to add noise with a small variance cumulatively to minimize the effect to accuracy while maintaining good privacy. This method has experimentally proven to achieve a better trade-off. Authors of Hewage et al. ( 2022 ) have proposed a novel random projection-based noise addition method using (Denham et al. 2020 ) as the base technique. It uses the effect of logistic function to control the noise level but still adds it cumulatively to achieve a high accuracy level. Meanwhile, some interesting PPDM methods in data stream mining do not include any discussion about accuracy-privacy trade-off (Mohammadian et al. 2014 ; Rajesh et al. 2012 ; Mohamed et al. 2017 ; Martínez Rodríguez et al. 2017 ; Nyati et al. 2018 ; Rajalakshmi and Mala 2013 ; Navarro-Arribas and Torra 2014 ; Virupaksha and Dondeti 2021 ; Wang et al. 2018 , 2007 ).
Figure 5 gives an overall idea about to what extent the PPDM research community has paid attention to the accuracy-privacy trade-off. It can be seen that there is a lack of attention to the accuracy-privacy trade-off in data stream-based PPDM methods relative to the generic PPDM methods. However, in both areas, some research work tries to solve this issue (30.26% in generic PPDM and 23.81% in Stream-based PPDM).
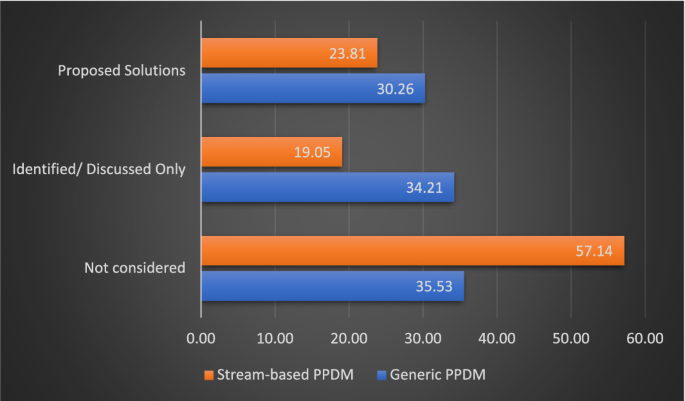
Consideration of accuracy-privacy trade-off in existing PPDM research (These percentages are derived according to the number of studies covering accuracy-privacy trade-off in PPDM/PPDSM from the total number of papers reviewed)
4 Discussion
In this section, we discuss how we addressed the gaps in the existing secondary studies by answering the formulated research questions.
In RQ1 and its subsections, we tried to identify the generic PPDM methods, the strengths and weaknesses of the identified methods, and the applicable data mining tasks. There are two broad categories of PPDM methods called input and output PPDM. We considered input PPDM methods as output PPDM methods focus on changing data mining output, which is a different scenario. After reviewing selected primary studies, it was found that a plethora of techniques has been proposed for privacy preservation in data mining. We divided them into four main categories: Secure Multiparty Computation, perturbation methods, non-perturbation methods, and combinations of the above categories. These methods cover most supervised and unsupervised learning techniques (Classification, Clustering, Association Rule Mining) in data mining. Most of the PPDM methods can be used for classification, and a considerable number of PPDM methods can apply to more than one data mining algorithm (Refer Fig. 3 ). Also, we observed that several studies lack standard accuracy and privacy evaluations after applying a data mining algorithm. This is a section where PPDM studies can be improved. Applying new techniques to a data mining algorithm using real or synthetic datasets provides validity and clarity and helps identify the sections needing improvement.
These generic PPDM methods have different strengths and weaknesses considering privacy, accuracy, time consumption, and more. The main reason for this is the nature of the techniques used to preserve privacy. Different techniques affect different characteristics differently. Because of this variety of advantages and weaknesses, when selecting a PPDM method, several factors such as the size of the dataset, domain, and contained sensitive data should be considered. There are no pre-defined criteria to decide on the appropriate PPDM methods for a specific dataset. Properties of the dataset and the characteristics of the privacy preservation technique should be considered when making this decision. All the facts found from the review have been summarized in Table 1 to 6 .
A categorization model of all the input PPDM methods was created by analyzing the extracted data and considering the existing categorizations. This model helps to grasp the overall picture of existing PPDM methods. Figure 6 illustrates the categorization model created, summarizing all the generic PPDM techniques.
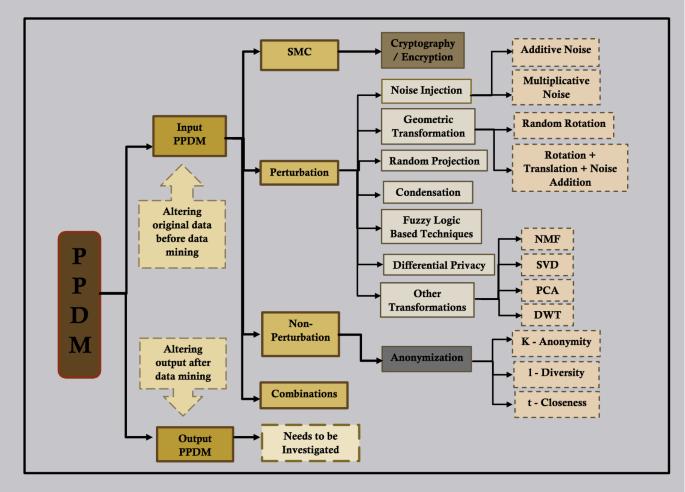
Categorization model of generic PPDM methods
For RQ2, we investigated the applicability of PPDM techniques for data stream mining. It was observed that most of the generic PPDM methods could not be used directly for data stream mining because of the challenging nature of data streams. This includes incremental nature, high speed, vast or infinite data, and possible concept drifts. Therefore, generic PPDM methods need improvements and amendments to be successfully used in PPDSM. It was observed that most of the generic PPDM methods do not discuss its applicability to data streams, which we suggest is something to be considered. If there is such discussion, it would be helpful for future development. Numerous PPDSM methods proposed for data stream mining improve existing generic PPDM methods or combinations of different techniques. Most of these methods use anonymization techniques to preserve privacy in data streams. Perturbation methods such as noise addition are also applicable because noise can be added independently to a single record at a time. While these methods could overcome most of the challenges in data stream mining, they still have some concerns, such as concept drift handling and time and computational complexity, that need to be improved. We also looked into the applicability of PPDSM methods in different data mining techniques. It was found that the majority of proposed PPDSM methods can be used for classification, followed by clustering. Interestingly, several PPDSM methods are available for more than one data mining task, which shows the generalizability of PPSM methods in data stream mining (Refer Fig. 4 ).
The well-known trade-off between data mining accuracy and data privacy was investigated in answering RQ3 to determine how it has been addressed. A majority of research work has identified and discussed the accuracy-privacy trade-off, but little effort has been made to propose techniques to address the issue. The conflicting nature of accuracy and privacy is the main reason for this. Though some methods have been proposed to optimize the accuracy-privacy trade-off, it is impossible to simultaneously achieve ideal values for both measures. Some methods have achieved this to some extent, but there is still considerable room for improvement. The techniques proposed to optimize the trade-off in data stream mining are less in number compared to generic PPDM methods (Refer Fig. 5 ). The proposed methods to optimize the trade-off include different techniques and methods. That includes preserving more statistical information, making changes only to sensitive attributes, parameter optimization, and considering users’ privacy requirements. We believe optimizing the accuracy-privacy trade-off has not received the attention it deserves, especially in PPDSM.
5 Conclusions and future directions
A significantly higher number of works address privacy in generic data mining as compared to techniques that apply to stream-based data mining. A positive remark is that these proposed PPDM methods can be used in different data mining algorithms in both supervised and unsupervised learning. However, all these methods have strengths and weaknesses due to the techniques used to preserve privacy. Though the PPDM research community has identified the trade-off between data mining accuracy and data privacy, there is a lack of research that tries to implement techniques with extensive experimentation to optimize this trade-off. Especially, PPDSM has a great need of techniques to optimize the accuracy-privacy trade-off in data stream mining. All our findings from this study can be listed as follows;
A plethora of studies propose different privacy-preserving techniques for PPDM.
The existing generic PPDM methods can be divided into four categories, namely SMC, perturbation, non-perturbation , and combinations of the above .
These PPDM methods can be used for different data mining algorithms, including classification, clustering, and association rule mining. Numerous methods work well on more than one data mining algorithm.
The existing PPDM methods have different strengths and weaknesses in several areas, including accuracy, privacy, and time complexity. These are caused by the techniques used to preserve privacy.
Different studies have been implemented to preserve privacy in data stream mining
Data streams behave differently than static datasets due to characteristics such as high volume, high speed, and concept drift. Therefore, privacy preservation in data stream mining is rather challenging.
Most of the generic PPDM methods cannot be used for PPDSM and need improvements to adapt to the behavior of data streams.
The trade-off between data mining accuracy and data privacy is one of the main issues in PPDM that needs more attention.
Evaluating accuracy is straightforward. However, privacy evaluation is a complicated task. Generally, this depends on the data mining technique and privacy preservation technique.
The most used accuracy evaluation metric is data mining accuracy, while privacy is measured by performing attacks or using other metrics such as differential privacy.
Many studies have identified and discussed the accuracy-privacy trade-off in PPDM.
Numerous studies have proposed and improved advanced PPDM techniques to optimize this trade-off in generic PPDM.
There are only a few studies that focus on optimizing the accuracy-privacy trade-off in PPDSM.
We only considered input PPDM methods in this study. Therefore, as a future direction, we would like to suggest an investigation on output PPDM methods and how they can optimize the accuracy-privacy trade-off as it often is used in data stream mining.
Abdul Y, Aldeen AS, Salleh M et al (2015) A comprehensive review on privacy preserving data mining. SpringerPlus. https://doi.org/10.1186/s40064-015-1481-x
Article Google Scholar
Aggarwal CC, Yu PS (2004) A condensation approach to privacy preserving data mining. Advances in database technology–EDBT 2004. Springer, Berlin, pp 183–199. https://doi.org/10.1007/978-3-540-24741-8_12
Chapter Google Scholar
Aggarwal CC, Yu PS (2008) On static and dynamic methods for condensation-based privacy-preserving data mining. ACM Trans Database Syst 33(1):1–40. https://doi.org/10.1145/1331904.1331906
Aggarwal CC, Yu PS (2008) Privacy-preserving data mining-models and algorithms. Springer, Berlin. https://doi.org/10.1007/978-0-387-70992-5
Book Google Scholar
Agrawal S, Haritsa JR (2005) A framework for high-accuracy privacy-preserving mining. In: Proceedings of the 21st International Conference on Data Engineering, ICDE
Ah-Fat P, Huth M (2019) Optimal accuracy-privacy trade-off for secure computations. IEEE Trans Inf Theory 65(5):3165–3182. https://doi.org/10.1109/TIT.2018.2886458
Article MathSciNet MATH Google Scholar
Alotaibi K, Rayward-Smith VJ, Wang W, et al. (2012) Non-linear dimensionality reduction for privacy-preserving data classification. In: Proceedings—2012 ASE/IEEE International Conference on Privacy, Security, Risk and Trust and 2012 ASE/IEEE International Conference on Social Computing, SocialCom/PASSAT 2012. IEEE, pp 694–701, https://doi.org/10.1109/SocialCom-PASSAT.2012.76
Arumugam G, Sulekha V (2016) IMR based anonymization for privacy preservation in data mining. In: ACM International Conference Proceeding Series, https://doi.org/10.1145/2925995.2926005
Ashok V, Mukkamala R (2011) Data mining without data: A novel approach to privacy-preserving collaborative distributed data mining. In: Proceedings of the ACM Conference on Computer and Communications Security, pp 159–164, https://doi.org/10.1145/2046556.2046578
Babu KS, Jena SK (2011) Balancing between utility and privacy for k-anonymity. Communications in Computer and Information Science 191 CCIS(PART 2):1–8. https://doi.org/10.1007/978-3-642-22714-1_1
Bhandari N, Pahwa P (2019) Comparative analysis of privacy-preserving data mining techniques. In: International Conference on Innovative Computing and Communications. Springer Singapore, pp 535–541, https://doi.org/10.1007/978-981-13-2354-6 , https://doi.org/10.1007/978-981-13-2354-6_54
Bhuyan HK, Ravi V, Yadav MS (2022) Multi-objective optimization-based privacy in data mining. Cluster Comput. https://doi.org/10.1007/s10586-022-03667-3
Cano I, Ladra S, Torra V, (2010) Evaluation of information loss for privacy preserving data mining through comparison of fuzzy partitions. In, (2010) IEEE World Congress on Computational Intelligence, WCCI 2010. IEEE. https://doi.org/10.1109/FUZZY.2010.5584186
Cao J, Carminati B, Ferrari E et al (2011) CASTLE: continuously anonymizing data streams. IEEE Trans Dependable Secure Comput 8(3):337–352. https://doi.org/10.1109/TDSC.2009.47
Carvalho T, Moniz N (2021) The compromise of data privacy in predictive performance. In: International Symposium on Intelligent Data Analysis, pp 426–438, https://doi.org/10.1007/978-3-030-74251-5
Chamikara MA, Bertok P, Liu D et al (2018) Efficient data perturbation for privacy preserving and accurate data stream mining. Pervasive Mobile Comput 48:1–19. https://doi.org/10.1016/j.pmcj.2018.05.003
Chamikara MA, Bertok P, Liu D et al (2019) An efficient and scalable privacy preserving algorithm for big data and data streams. Comput Secur 87(101):570. https://doi.org/10.1016/j.cose.2019.101570
Chamikara MA, Bertok P, Liu D et al (2020) Efficient privacy preservation of big data for accurate data mining. Inform Sci 527:420–443. https://doi.org/10.1016/j.ins.2019.05.053
Chamikara MA, Bertok P, Khalil I et al (2021) PPaaS: Privacy Preservation as a Service. Comput Commun 173:192–205. https://doi.org/10.1016/j.comcom.2021.04.006
Chen K, Liu L (2005) A random rotation perturbation approach to privacy preserving data classification. In: International Conference on Data Mining
Chen K, Liu L (2011) Geometric data perturbation for privacy preserving outsourced data mining. Knowl Inf Syst 29(3):657–695. https://doi.org/10.1007/s10115-010-0362-4
Chen K, Sun G, Liu L (2007) Towards Attack-Resilient Geometric Data Perturbation. In: SIAM International Conference on Data Mining, pp 78–89, https://doi.org/10.1137/1.9781611972771.8
Cheng P, Chu SC, Lin CW et al (2014) Distortion-based heuristic sensitive rule hiding method—The greedy way. Lecture Notes in Artificial Intelligence (Subseries of Lecture Notes in Computer Science) 8481:77–86. https://doi.org/10.1007/978-3-319-07455-9_9
Chidambaram S, Srinivasagan KG (2014) A combined random noise perturbation approach for multi level privacy preservation in data mining. In: 2014 International Conference on Recent Trends in Information Technology, ICRTIT 2014. IEEE, pp 1–6, https://doi.org/10.1109/ICRTIT.2014.6996194
Cuzzocrea A (2017) Privacy-preserving big data stream mining: Opportunities, challenges, directions. In: IEEE International Conference on Data Mining Workshops, ICDMW, pp 992–994, https://doi.org/10.1109/ICDMW.2017.140
Deivanai P, Nayahi JJV, Kavitha V (2011) A hybrid data anonymization integrated with suppression for preserving privacy in mining multi party data. In: International Conference on Recent Trends in Information Technology, ICRTIT 2011. IEEE, pp 732–736, https://doi.org/10.1109/ICRTIT.2011.5972462
Denham B, Pears R, Naeem MA (2020) Enhancing random projection with independent and cumulative additive noise for privacy-preserving data stream mining. Expert Systems with Applications 152. https://doi.org/10.1016/j.eswa.2020.113380
Dhanalakshmi M, Siva Sankari E (2014) Privacy Preserving Data Mining Techniques-Survey. In: International Conference on Information Communication and Embedded Systems (ICICES2014). IEEE, pp 1–6, https://doi.org/10.1109/ICICES.2014.7033869.
Dhinakaran D, Prathap PM (2022) Protection of data privacy from vulnerability using two-fish technique with Apriori algorithm in data mining. J Supercomputing. https://doi.org/10.1007/s11227-022-04517-0
Dhinakaran D, Prathap PMJ (2022) Preserving data confidentiality in association rule mining using data share allocator algorithm. Intell Auto Soft Computing 33:1877–1892. https://doi.org/10.32604/iasc.2022.024509
Dutta S, Guppta AK (2016) Privacy in data mining—a review. In: International Conference on Computing for Sustainable Global Development (INDIACom), pp 556–559
Dwork C (2008) Differential privacy: a survey of results. Lecture Notes in Computer Science (including subseries Lecture Notes in Artificial Intelligence and Lecture Notes in Bioinformatics) 4978 LNCS:1–19. https://doi.org/10.1007/978-3-540-79228-4-1
Feyisetan O, Balle B, Drake T, et al. (2020) Privacy- and utility-preserving textual analysis via calibrated perturbations. In: CEUR Workshop Proceedings, pp 41–42
Giannella CR, Liu K, Kargupta H (2013) Breaching Euclidean distance-preserving data perturbation using few known inputs. Data Knowl Eng 83:93–110. https://doi.org/10.1016/j.datak.2012.10.004
Gitanjali J, Indumathi J, Sriman NC, et al. (2010) A Pristine clean cabalistic foruity strategize based approach for incremental data stream. In: IEEE 2nd International Advance Computing Conference. IEEE, pp 410–415
Gokulnath C, Priyan MK, Balan EV, et al. (2015) Preservation of privacy in data mining by using PCA based perturbation technique. In: 2015 International Conference on Smart Technologies and Management for Computing, Communication, Controls, Energy and Materials, ICSTM 2015 - Proceedings. IEEE, May, pp 202–206, https://doi.org/10.1109/ICSTM.2015.7225414
Gomes HM, Read J, Bifet A et al (2019) Machine learning for streaming data: state of the art, challenges, and opportunities. SIGKDD Explor Newsl 21(2):6–22. https://doi.org/10.1145/3373464.3373470
Gondara L, Wang K, Carvalho RS (2022) Differentially private ensemble classifiers for data streams. Association for Computing Machinery, Inc, pp 325–333, https://doi.org/10.1145/3488560.3498498
Hasan MM, Hossain S, Paul MK, et al. (2019) A new hybrid approach for privacy preserving data mining using matrix decomposition technique. In: 2019 4th International Conference on Electrical Information and Communication Technology, EICT 2019. IEEE, December, pp 20–22, https://doi.org/10.1109/EICT48899.2019.9068789
Hewage U, Pears R, Naeem MA (2022) Optimizing the trade-off between classification accuracy and data privacy in the area of data stream mining. Int J Artif Intell 1(1):147–167
Google Scholar
Hong Tp, Yang Kt, Lin Cw, et al. (2010) Evolutionary privacy-preserving data mining. In: World Automation Congress. IEEE, pp 2–8
Hong TP, Lin CW, Yang KT, et al. (2011) A heuristic data-sanitization approach based on TF-IDF. Lecture Notes in Computer Science (including subseries Lecture Notes in Artificial Intelligence and Lecture Notes in Bioinformatics) 6703 LNAI(PART 1):156–164. https://doi.org/10.1007/978-3-642-21822-4_17
Jahan T, Narsimha G, Guru Rao CV (2016) Multiplicative data perturbation using fuzzy logic in preserving privacy. In: ACM International Conference Proceeding Series, https://doi.org/10.1145/2905055.2905096
Jain P, Gyanchandani M, Khare N (2016) Big data privacy: a technological perspective and review. J Big Data. https://doi.org/10.1186/s40537-016-0059-y
Javid T, Gupta MK (2020) Privacy preserving classification using 4-dimensional rotation transformation. In: Proceedings of the 2019 8th International Conference on System Modeling and Advancement in Research Trends, SMART 2019, pp 279–284, https://doi.org/10.1109/SMART46866.2019.9117391
Kabir SM, Youssef AM, Elhakeem AK (2007a) On data distortion for privacy preserving data mining. In: Canadian Conference on Electrical and Computer Engineering, pp 308–311, https://doi.org/10.1109/CCECE.2007.83
Kabir SM, Youssef AM, Elhakeem AK (2007b) On data distortion for privacy preserving data mining. In: Canadian Conference on Electrical and Computer Engineering, pp 308–311, https://doi.org/10.1109/CCECE.2007.83
Kadampur MA, Somayajulu DV (2008) A data perturbation method by field rotation and binning by averages strategy for privacy preservation. Lecture Notes in Computer Science (including subseries Lecture Notes in Artificial Intelligence and Lecture Notes in Bioinformatics) 5326 LNCS:250–257. https://doi.org/10.1007/978-3-540-88906-9_32
Katsomallos M, Tzompanaki K, Kotzinos D (2022) Landmark privacy: configurable differential privacy protection for time series. Association for Computing Machinery, Inc, pp 179–190, https://doi.org/10.1145/3508398.3511501
Kaur A (2017) A hybrid approach of privacy preserving data mining using suppression and perturbation techniques. In: IEEE International Conference on Innovative Mechanisms for Industry Applications, ICIMIA 2017—Proceedings. IEEE, Icimia, pp 306–311, https://doi.org/10.1109/ICIMIA.2017.7975625
Kaur R, Bansal M (2016) Transformation approach for Boolean attributes in privacy preserving data mining. In: Proceedings on 2015 1st International Conference on Next Generation Computing Technologies, NGCT 2015, September, pp 644–648, https://doi.org/10.1109/NGCT.2015.7375200
Ketel M, Homaifar A (2005) Privacy-preserving mining by rotational data transformation. In: Proceedings of the Annual Southeast Conference, pp 1233–1236, https://doi.org/10.1145/1167350.1167419
Khavkin M, Last M (2019) Preserving differential privacy and utility of non-stationary data streams. In: IEEE International Conference on Data Mining Workshops, ICDMW, vol 2018-Novem. IEEE, pp 29–34, https://doi.org/10.1109/ICDMW.2018.00012
Kim D, Chen Z, Gangopadhyay A (2012) Optimizing privacy-accuracy tradeoff for privacy preserving distance-based classification. Int J Inf Secur Privacy 6(2):16–33. https://doi.org/10.4018/jisp.2012040102
Kim JJ, Winkler WE (2003) Multiplicative noise for masking continuous data. Tech. rep., Statistical Research Division U.S. Bureau of the Census, Washington
Kiran A, Vasumathi D (2018) A comprehensive survey on privacy preservation algorithms in data mining. In: 2017 IEEE International Conference on Computational Intelligence and Computing Research, ICCIC 2017. IEEE, https://doi.org/10.1109/ICCIC.2017.8524294
Kiran A, Vasumathi D (2020) Data mining: min-max normalization based data perturbation technique for privacy preservation, vol 1090. Springer, Singapore. https://doi.org/10.1007/978-981-15-1480-7_66
Kitchenham B, Pearl Brereton O, Budgen D et al (2009) Systematic literature reviews in software engineering—a systematic literature review. Inf Softw Technol 51(1):7–15. https://doi.org/10.1016/j.infsof.2008.09.009
Kotecha R, Garg S (2017) Preserving output-privacy in data stream classification. Prog Artif Intell 6(2):87–104. https://doi.org/10.1007/s13748-017-0114-8
Krempl G, Žliobaite I, Brzeziński D et al (2014) Open challenges for data stream mining research. ACM SIGKDD Explorations Newsletter 16(1):1–10. https://doi.org/10.1145/2674026.2674028
Kumar GS, Premalatha K (2021) Securing private information by data perturbation using statistical transformation with three dimensional shearing. Appl Soft Comput 112(107):819. https://doi.org/10.1016/j.asoc.2021.107819
Li G, Wang Y (2011) Privacy-preserving data mining based on sample selection and singular value decomposition. In: Proceedings—2011 International Conference on Internet Computing and Information Services, ICICIS 2011. IEEE, pp 298–301, https://doi.org/10.1109/ICICIS.2011.79
Li G, Xue R (2018) A new privacy-preserving data mining method using non-negative matrix factorization and singular value decomposition. Wireless Personal Commun 102(2):1799–1808. https://doi.org/10.1007/s11277-017-5237-5
Li TNinghui Li, Venkatasubramanian S (2007) t-Closeness: Privacy Beyond k-Anonymity and l-DiversityT. In: IEEE 23rd International Conference on Data Engineering, 2, pp 106–115
Lin CY, Kao YH, Lee WB et al (2016) An efficient reversible privacy-preserving data mining technology over data streams. SpringerPlus 5(1):1–11. https://doi.org/10.1186/s40064-016-3095-3
Lin KP, Chang YW, Chen MS (2015) Secure support vector machines outsourcing with random linear transformation. Knowl Inf Syst 44(1):147–176. https://doi.org/10.1007/s10115-014-0751-1
Liu C, Chen S, Zhou S et al (2019) A novel privacy preserving method for data publication. Inf Sci 501:421–435. https://doi.org/10.1016/j.ins.2019.06.022
Article MathSciNet Google Scholar
Liu K, Kargupta H, Ryan J (2006) Random projection-based multiplicative data perturbation for privacy preserving distributed data mining. IEEE Trans Knowl Data Eng 18(1):92–106. https://doi.org/10.1109/TKDE.2006.14
Lohiya S, Ragha L (2012) Privacy preserving in data mining using hybrid approach. In: Proceedings—4th International Conference on Computational Intelligence and Communication Networks, CICN 2012. IEEE, pp 743–746, https://doi.org/10.1109/CICN.2012.166
Machanavajjhala A, Kifer D, Gehrke J et al (2007) l-diversity: privacy beyond k-anonymity. ACM Trans Knowl Discov Data. https://doi.org/10.1145/1217299.1217302
Malik MB, Ghazi MA, Ali R (2012) Privacy preserving data mining techniques: current scenario and future prospects. In: Proceedings of the 2012 3rd International Conference on Computer and Communication Technology, ICCCT 2012. IEEE, pp 26–32, https://doi.org/10.1109/ICCCT.2012.15
Martínez Rodríguez D, Nin J, Nuñez-del Prado M (2017) Towards the adaptation of SDC methods to stream mining. Computers and Security 70(2017):702–722. https://doi.org/10.1016/j.cose.2017.08.011
Md Siraj M, Rahmat NA, Din MM (2019) A survey on privacy preserving data mining approaches and techniques. In: ACM International Conference Proceeding Series, pp 65–69, https://doi.org/10.1145/3316615.3316632
Meghanathan N, Nagamalai D, Rajasekaran S (2014) A comparative study of data perturbation using fuzzy logic to preserve privacy. Lecture Notes in Electrical Engineering 284 LNEE:161–170. https://doi.org/10.1007/978-3-319-03692-2
Mivule K, Turner C, Ji SY (2012) Towards a differential privacy and utility preserving machine learning classifier. Proc Computer Sci 12:176–181. https://doi.org/10.1016/j.procs.2012.09.050
Miyaji A, Rahman MS (2011) Privacy-preserving data mining : a game-theoretic approach. Data and Applications Security and Privacy XXV pp 186–200
Modi CN, Rao UP, Patel DR (2010) Maintaining privacy and data quality in privacy preserving association rule mining. In: 2010 2nd International Conference on Computing, Communication and Networking Technologies, ICCCNT 2010. IEEE, pp 7–12, https://doi.org/10.1109/ICCCNT.2010.5592589
Mohamed MA, Nagi MH, Ghanem SM (2017) A clustering approach for anonymizing distributed data streams. Proceedings of 2016 11th International Conference on Computer Engineering and Systems, ICCES 2016 pp 9–16. https://doi.org/10.1109/ICCES.2016.7821968
Mohammadian E, Noferesti M, Jalili R (2014) FAST: Fast anonymization of big data streams. In: ACM International Conference Proceeding Series, https://doi.org/10.1145/2640087.2644149
Mukherjee S, Banerjee M, Chen Z et al (2008) A privacy preserving technique for distance-based classification with worst case privacy guarantees. Data Knowl Eng 66(2):264–288. https://doi.org/10.1016/j.datak.2008.03.004
Narwaria M, Arya S (2016) Privacy preserving data mining:"A state of the art". In: 2016 International Conference on Computing for Sustainable Global Development (INDIACom). Bharati Vidyapeeth, New Delhi as the Organizer of INDIACom - 2016, pp 1–15, https://doi.org/10.1007/978-981-13-0761-4_1
Nasiri N, Keyvanpour M (2020) Classification and evaluation of Privercy preserving data mining methods. In: 11th International Conference on Information and Knowledge Discovery (IKT), pp 17–22
Navarro-Arribas G, Torra V (2014) Rank swapping for stream data. Lecture Notes in Computer Science (including subseries Lecture Notes in Artificial Intelligence and Lecture Notes in Bioinformatics) 8825:217–226. https://doi.org/10.1007/978-3-319-12054-6_19
Nayahi JJV, Kavitha V (2017) Privacy and utility preserving data clustering for data anonymization and distribution on Hadoop. Future Gener Computer Syst 74:393–408. https://doi.org/10.1016/j.future.2016.10.022
Nethravathi NP, Rao PG, Shenoy PD, et al. (2016) CBTS: Correlation based transformation strategy for privacy preserving data mining. In: 2015 IEEE International WIE Conference on Electrical and Computer Engineering, WIECON-ECE 2015. IEEE, pp 190–194, https://doi.org/10.1109/WIECON-ECE.2015.7443894
Nyati A, Dargar SK, Sharda S (2018) Design and implementation of a new model for privacy preserving classification of data streams, vol 906. Springer, Singapore. https://doi.org/10.1007/978-981-13-1813-9_45
Oishi K (2017) Proposal of l -diversity algorithm considering distance between sensitive attribute values. In: 2017 IEEE Symposium Series on Computational Intelligence (SSCI), pp 1–8, https://doi.org/10.1109/SSCI.2017.8280973
Park S, Byun J, Lee J (2022) Privacy-preserving fair learning of support vector machine with homomorphic encryption. Association for Computing Machinery, Inc, pp 3572–3583, https://doi.org/10.1145/3485447.3512252
Patel D, Kotecha R (2017) Privacy preserving data mining: A parametric analysis. Adv Intell Syst Comput 516:139–149. https://doi.org/10.1007/978-981-10-3156-4_14
Paul MK, Islam MR, Sattar AS (2021) An efficient perturbation approach for multivariate data in sensitive and reliable data mining. J Info Secur Appl 62(102):954. https://doi.org/10.1016/j.jisa.2021.102954
Peng B, Geng X, Zhang J (2010) Combined data distortion strategies for privacy-preserving data mining. In: ICACTE 2010 - 2010 3rd International Conference on Advanced Computer Theory and Engineering, Proceedings, pp 572–576, https://doi.org/10.1109/ICACTE.2010.5578952
Poovammal E, Ponnavaikko M (2009) An improved method for privacy preserving data mining. In: 2009 IEEE International Advance Computing Conference, IACC 2009, March, pp 1453–1458, https://doi.org/10.1109/IADCC.2009.4809231
Putri AW, Hira L (2017) Hybrid transformation in privacy-preserving data mining. In: Proceedings of 2016 International Conference on Data and Software Engineering, ICoDSE 2016, pp 0–5, https://doi.org/10.1109/ICODSE.2016.7936114
Qi X, Zong M (2012) An overview of privacy preserving data mining. Procedia Environmental Sciences 12(Icese 2011):1341–1347. https://doi.org/10.1016/j.proenv.2012.01.432
Rajalakshmi V, Mala GS (2013) An intensified approach for privacy preservation in incremental data mining. Adv Intell Syst Computing 178:347–355. https://doi.org/10.1007/978-3-642-31600-5_34
Rajesh P, Narisimha G, Rupa C (2012) Fuzzy based privacy preserving classification of data streams. In: ACM International Conference Proceeding Series, pp 784–788, https://doi.org/10.1145/2381716.2381865
Sachan A, Roy D, Arun PV (2013) An analysis of privacy preservation techniques in data mining. Adv Intell Syst Comput 178:119–128. https://doi.org/10.1007/978-3-642-31600-5_12
Sakpere AB, Kayem AV (2014) A state-of-the-art review of data stream anonymization schemes. Information Security in Diverse Computing Environments pp 24–50. https://doi.org/10.4018/978-1-4666-6158-5.ch003
Sangeetha S, Sadasivam GS (2019) Privacy of big data : a review. In: Handbook of Big Data and IoT Security. Springer Nature Switzerland AG
Shanthi SA, Karthikeyan M (2012) A review on privacy preserving data mining. In: IEEE International Conference on Computational Intelligence and Computing Research, vol 4. IEEE, pp 1–36, https://doi.org/10.1186/s40064-015-1481-x
Sharma S, Ahuja S (2019) Privacy preserving data mining: a review of the state of the art BT-harmony search and nature inspired optimization algorithms. Springer, Singapore. https://doi.org/10.1007/978-981-13-0761-4_1
Singh K, Batten L (2013) An attack-resistant hybrid data-privatization method with low information loss. IFIP Adv Inf Commun Technol 401:263–271. https://doi.org/10.1007/978-3-642-38323-6_21
Soria-Comas J, Domingo-Ferrer J, Sanchez D, et al. (2016) T-closeness through microaggregation: strict privacy with enhanced utility preservation. In: 2016 IEEE 32nd International Conference on Data Engineering, ICDE 2016, pp 1464–1465, https://doi.org/10.1109/ICDE.2016.7498376
Sowmyarani CN, Srinivasan GN, Sukanya K (2013) A new privacy preserving measure: p-sensitive, t-closeness. Adv Intell Syst Comput 174 AISC:57–62. https://doi.org/10.1007/978-81-322-0740-5_7
Suma B, Shobha G (2021) Privacy preserving association rule hiding using border based approach. Indones J Electric Eng Comput Sci 23(2):1137–1145. https://doi.org/10.11591/ijeecs.v23.i2.pp1137-1145
Sun C, Gao H, Zhou J, et al. (2014) A new hybrid approach for privacy preserving distributed data mining. IEICE Transactions on Information and Systems E97-D(4):876–883. https://doi.org/10.1587/transinf.E97.D.876
Sweeney L (2002) k-Anonymity: A model for protecting privacy. IEEE Security And Privacy 10(5):1–14
MathSciNet MATH Google Scholar
Tang W, Zhou Y, Wu Z, et al. (2019) Naive bayes classification based on differential privacy. In: ACM International Conference Proceeding Series, https://doi.org/10.1145/3358331.3358396
Tayal V, Srivastava R (2019) Challenges in mining big data streams, vol 847. Springer, Singapore. https://doi.org/10.1007/978-981-13-2254-9_15
Teng Z, Du W (2009) A hybrid multi-group approach for privacy-preserving data mining. Knowl Inf Syst 19(2):133–157. https://doi.org/10.1007/s10115-008-0158-y
Tran Hy HuJ (2019) Privacy-preserving big data analytics—a comprehensive survey. J Parallel Distributed Computing 134:207–218. https://doi.org/10.1016/j.jpdc.2019.08.007
Tran NH, Le-Khac NA, Kechadi MT (2020) Lightweight privacy-Preserving data classification. Computers and Security 97(101):835. https://doi.org/10.1016/j.cose.2020.101835
Tsai YC, Wang SL, Song CY, et al. (2016) Privacy and utility effects of k-anonymity on association rule hiding. In: ACM International Conference Proceeding Series, pp 0–5, https://doi.org/10.1145/2955129.2955169
Tsiafoulis SG, Zorkadis VC, Pimenidis E (2012) Maximum entropy oriented anonymization algorithm. Social Inform Telecommun Eng 2012:9–16
Upadhayay AK, Agarwal A, Masand R, et al. (2009) Privacy preserving data mining: a new methodology for data transformation. In: Proceedings of the First International Conference on Intelligent Human Computer Interaction, pp 372–390, https://doi.org/10.1007/978-81-8489-203-1_36
Upadhyay S, Sharma C, Sharma P et al (2018) Privacy preserving data mining with 3-D rotation transformation. J King Saud Univ Comput Inform Sci 30(4):524–530. https://doi.org/10.1016/j.jksuci.2016.11.009
Vijayarani S, Tamilarasi A (2011) An efficient masking technique for sensitive data protection. In: International Conference on Recent Trends in Information Technology, ICRTIT 2011. IEEE, pp 1245–1249, https://doi.org/10.1109/ICRTIT.2011.5972275
Vijayarani S, Tamilarasi A (2013) Data transformation and data transitive techniques for protecting sensitive data in privacy preserving data mining. In: Sobh T, Elleithy K (eds) Emerging trends in computing, informatics, systems sciences, and engineering. Springer, New York, pp 345–355
Virupaksha S, Dondeti V (2021) Anonymized noise addition in subspaces for privacy preserved data mining in high dimensional continuous data. Peer-to-Peer Networking and Applications 14(3):1608–1628. https://doi.org/10.1007/s12083-021-01080-y
Vishwakarma B, Gupta H, Manoria M (2016) A survey on privacy preserving mining implementing techniques. In: 2016 Symposium on Colossal Data Analysis and Networking, CDAN 2016. IEEE, pp 7–11, https://doi.org/10.1109/CDAN.2016.7570874
Wang J, Chan WKV (2021) A Design for Private Data Protection Combining with Data Perturbation and Data Reconstruction. In: ACM International Conference Proceeding Series, pp 545–550, https://doi.org/10.1145/3459104.3459193
Wang J, Zhang J (2007) Addressing accuracy issues in privacy preserving data mining through matrix factorization. ISI 2007: 2007 IEEE Intelligence and Security Informatics pp 217–220. https://doi.org/10.1109/isi.2007.379474
Wang J, Luo Y, Jiang S, et al. (2009) A survey on anonymity-based privacy preserving. In: 2009 International Conference on E-Business and Information System Security, EBISS 2009. IEEE, pp 7–10, https://doi.org/10.1109/EBISS.2009.5137908
Wang J, Deng C, Li X (2018) Two Privacy-Preserving Approaches for Publishing Transactional Data Streams. IEEE Access 6:23,648–23,658. https://doi.org/10.1109/ACCESS.2018.2814622
Wang W, Li J, Ai C, et al. (2007) Privacy protection on sliding window of data streams. In: Proceedings of the 3rd International Conference on Collaborative Computing: Networking, Applications and Worksharing, CollaborateCom 2007, pp 213–221, https://doi.org/10.1109/COLCOM.2007.4553832
Xiaoping L, Jianfeng L, Haina S (2020) Research on privacy preserving data mining based on randomized response. In: ACM International Conference Proceeding Series, pp 129–132, https://doi.org/10.1145/3407703.3407727
Xu S, Zhang J, Han D et al (2006) Singular value decomposition based data distortion strategy for privacy protection. Knowledge and Information Systems 10(3):383–397. https://doi.org/10.1007/s10115-006-0001-2
Yang F, Liao X (2022) An optimized sanitization approach for minable data publication. Big Data Mining and Analytics 5:257–269. https://doi.org/10.26599/bdma.2022.9020007
Zaman AN, Obimbo C, Dara RA (2016) A novel differential privacy approach that enhances classification accuracy. In: ACM International Conference Proceeding Series, pp 79–84, https://doi.org/10.1145/2948992.2949027
Zhang G, Li S (2019) Research on Differentially Private Bayesian Classification Algorithm for Data Streams. In: 2019 4th IEEE International Conference on Big Data Analytics, ICBDA 2019. IEEE, pp 14–20, https://doi.org/10.1109/ICBDA.2019.8713253
Download references
Open Access funding enabled and organized by CAUL and its Member Institutions.
Author information
Authors and affiliations.
School of Engineering Computer and Mathematical Sciences, Auckland University of Technology, Auckland, 1010, New Zealand
U. H. W. A. Hewage & R. Sinha
Department of Computer Science, National University of Computer and Emerging Sciences, Islamabad, Pakistan
M. Asif Naeem
You can also search for this author in PubMed Google Scholar
Corresponding author
Correspondence to U. H. W. A. Hewage .
Ethics declarations
Conflict of interest.
The authors have no conflicts of interest to declare that are relevant to the content of this article.
Additional information
Publisher's note.
Springer Nature remains neutral with regard to jurisdictional claims in published maps and institutional affiliations.
Rights and permissions
Open Access This article is licensed under a Creative Commons Attribution 4.0 International License, which permits use, sharing, adaptation, distribution and reproduction in any medium or format, as long as you give appropriate credit to the original author(s) and the source, provide a link to the Creative Commons licence, and indicate if changes were made. The images or other third party material in this article are included in the article's Creative Commons licence, unless indicated otherwise in a credit line to the material. If material is not included in the article's Creative Commons licence and your intended use is not permitted by statutory regulation or exceeds the permitted use, you will need to obtain permission directly from the copyright holder. To view a copy of this licence, visit http://creativecommons.org/licenses/by/4.0/ .
Reprints and permissions
About this article
Hewage, U.H.W.A., Sinha, R. & Naeem, M.A. Privacy-preserving data (stream) mining techniques and their impact on data mining accuracy: a systematic literature review. Artif Intell Rev 56 , 10427–10464 (2023). https://doi.org/10.1007/s10462-023-10425-3
Download citation
Published : 22 February 2023
Issue Date : September 2023
DOI : https://doi.org/10.1007/s10462-023-10425-3
Share this article
Anyone you share the following link with will be able to read this content:
Sorry, a shareable link is not currently available for this article.
Provided by the Springer Nature SharedIt content-sharing initiative
- Privacy-preserving data mining
- Data streams
- Accuracy-privacy trade-off
- Data privacy
- Find a journal
- Publish with us
- Track your research
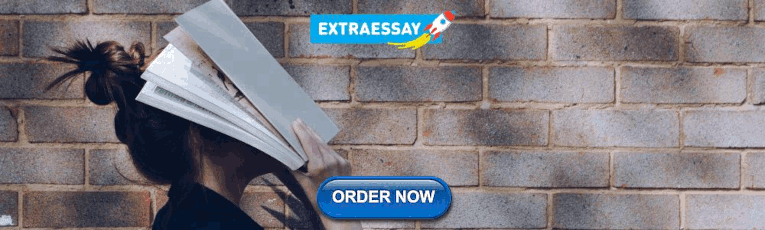
IMAGES
VIDEO
COMMENTS
Data mining is a process which finds useful patterns from large amount of data. The paper discusses few of the data mining techniques, algorithms and some of the organizations which have adapted ...
The information gain, gain ratio, gini decrease, chi-square, and relieff are used to rank the features. This work comprises the introduction, literature review, and proposed methodology parts. In this research paper, a new method of analyzing skin disease has been proposed in which six different data mining techniques are used to develop an ...
Twitter is sharing its data for research and study purposes by exposing open APIs that make it the most suitable source of data for social media analytics. Applying data mining and machine learning techniques on tweets is gaining more and more interest. ... This paper uses a decision tree algorithm in data mining for the analysis and finds out ...
Data mining plays an important role in various human activities because it extracts the unknown useful patterns (or knowledge). Due to its capabilities, data mining become an essential task in large number of application domains such as banking, retail, medical, insurance, bioinformatics, etc. To take a holistic view of the research trends in the area of data mining, a comprehensive survey is ...
This paper reviews data mining techniques and its applications such as educational data mining (EDM), finance, commerce, life sciences and medical etc. We group existing approaches to determine how the data mining can be used in different fields. Our categorization specifically focuses on the research that has been published over the period ...
Motivated by this, in this paper, we attempt to make a comprehensive survey of the important recent developments of data mining research. This survey focuses on knowledge view, utilized techniques view, and application view of data mining. ... City incident information management system can integrate data mining methods to provide a ...
To take a holistic view of the research trends in the area of data mining, a comprehensive survey is presented in this paper. This paper presents a systematic and comprehensive survey of various data mining tasks and techniques. Further, various real-life applications of data mining are presented in this paper.
Data Mining and Knowledge Discovery is a leading technical journal focusing on the extraction of information from vast databases. Publishes original research papers and practice in data mining and knowledge discovery. Provides surveys and tutorials of important areas and techniques. Offers detailed descriptions of significant applications.
Data mining is a field of intersection of computer science and statistics used to discover patterns in the information bank. The main aim of the data mining process is to extract the useful information from the dossier of data and mold it into an understandable structure for future use. There are different process and techniques used to carry out data mining successfully.
Finally, there are studies that surveyed data mining techniques and applications across domains, yet, they focus on data mining process artifacts and outcomes ... In this section, we address the research questions of the paper. Initially, as part of RQ1, we present overview of data mining methodologies 'as-is' and adaptation trends. In ...
A broad range of data mining techniques can be utilized for big data in education, which Baker and ... We did not consider papers that solely report on methodological improvements or conceptual papers. Also, the research needed to be situated in a formal or informal educational context. For instance, research studies that focused on students ...
Abstract. Data mining is the process of extracting hidden and useful patterns and information from data. Data mining is a new technology that helps businesses to predict future trends and behaviors, allowing them to make proactive, knowledge driven decisions. The aim of this paper is to show the process of data mining and how it can help ...
Furthermore, in the thematic network structure the themes were then manually classified between data mining techniques and medical research concepts. 2.1.4. Performance Analysis ... its fading could provide a first insight into the overall evolution of the data mining papers applied to healthcare. The occurrence of the cluster knowledge ...
Data mining research has been significantly motivated by and benefited from real-world applications in novel domains. This special issue was proposed and edited to draw attention to domain-driven data mining and disseminate research in foundations, frameworks, and applications for data-driven and actionable knowledge discovery. Along with this special issue, we also organized a related ...
Data mining techniques are capable of handling the three dominant research issues with SM data which are size, noise and dynamism. This paper reviews data mining techniques currently in use on analysing SM and looked at other data mining techniques that can be considered in the field. Keywords: Social Media, Social Media Analysis, Data Mining.
In this paper, we propose a diabetes prediction model using data mining techniques. We apply four data mining techniques such as Random Forest, Support Vector Machine (SVM), Logistic Regression, and Naive Bayes. The proposed mechanism is trained using Python and analysed with a real dataset, which is collected from Kaggle.
In order to identify the strategic topics and the thematic evolution structure of data mining applied to healthcare, in this paper, a bibliometric performance and network analysis (BPNA) was conducted. For this purpose, 6138 articles were sourced from the Web of Science covering the period from 1995 to July 2020 and the SciMAT software was used. Our results present a strategic diagram composed ...
The research objective is to review and examine the latest data mining techniques related to financial fraud and classifying them based on their types of fraud. The research scope is to select the range of papers that includes data mining techniques used in financial domains that are published between 2009 and 2019.
The purpose of this research is to present a sample study analyzing data gathered from an educational study using data mining techniques appropriate for processing these data. In order to achieve this aim, a "Computer Self-efficiency Scale" used in educational sciences was selected and this scale was applied in a study group.
This study investigates existing input privacy-preserving data mining (PPDM) methods and privacy-preserving data stream mining methods (PPDSM), including their strengths and weaknesses. A further analysis was carried out to determine to what extent existing PPDM/PPDSM methods address the trade-off between data mining accuracy and data privacy which is a significant concern in the area. The ...