If you're seeing this message, it means we're having trouble loading external resources on our website.
If you're behind a web filter, please make sure that the domains *.kastatic.org and *.kasandbox.org are unblocked.
To log in and use all the features of Khan Academy, please enable JavaScript in your browser.
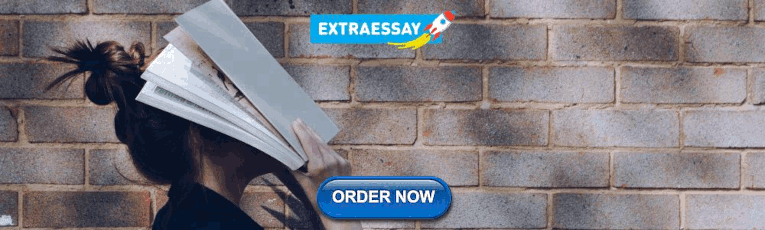
AP®︎/College Statistics
Course: ap®︎/college statistics > unit 6.
- Statistical significance of experiment
Random sampling vs. random assignment (scope of inference)
- Conclusions in observational studies versus experiments
- Finding errors in study conclusions
- (Choice A) Just the residents involved in Hilary's study. A Just the residents involved in Hilary's study.
- (Choice B) All residents in Hilary's town. B All residents in Hilary's town.
- (Choice C) All residents in Hilary's country. C All residents in Hilary's country.
- (Choice A) Yes A Yes
- (Choice B) No B No
- (Choice A) Just the residents in Hilary's study. A Just the residents in Hilary's study.
Want to join the conversation?
- Upvote Button navigates to signup page
- Downvote Button navigates to signup page
- Flag Button navigates to signup page

Scientific Research and Methodology
7.3 random allocation vs random sampling.
Random sampling and random allocation are two different concepts (Fig. 7.4 ), that serve two different purposes, but are often confused:
- Random sampling allows results to be generalised to a larger population, and impacts external validity. It concerns how the sample is found to study.
- Random allocation tries to eliminate confounding issues, by evening-out possible confounders across treatment groups. Random allocation of treatments helps establish cause-and-effect, and impacts internal validity. It concerns how the members of the chosen sample get the treatments .

FIGURE 7.4: Comparing random allocation and random sampling

Statistics Made Easy
Randomization in Statistics: Definition & Example
In the field of statistics, randomization refers to the act of randomly assigning subjects in a study to different treatment groups.
For example, suppose researchers recruit 100 subjects to participate in a study in which they hope to understand whether or not two different pills have different effects on blood pressure.
They may decide to use a random number generator to randomly assign each subject to use either pill #1 or pill #2.

Benefits of Randomization
The point of randomization is to control for lurking variables – variables that are not directly included in an analysis, yet impact the analysis in some way.
For example, if researchers are studying the effects of two different pills on blood pressure then the following lurking variables could affect the analysis:
- Smoking habits
By randomly assigning subjects to treatment groups, we maximize the chances that the lurking variables will affect both treatment groups equally.
This means any differences in blood pressure can be attributed to the type of pill, rather than the effect of a lurking variable.
Block Randomization
An extension of randomization is known as block randomization . This is the process of first separating subjects into blocks, then using randomization to assign subjects within blocks to different treatments.
For example, if researchers want to know whether or not two different pills affect blood pressure differently then they may first separate all subjects into one of two blocks based on gender: Male or Female.
Then, within each block they can use randomization to randomly assign subjects to use either Pill #1 or Pill #2.

The benefit of this approach is that researchers can directly control for any effect that gender may have on blood pressure since we know that males and females are likely to respond to each pill differently.
By using gender as a block, we’re able to eliminate this variable as a potential source of variation. If there are differences in blood pressure between the two pills then we can know that gender is not the underlying cause of these differences.
Additional Resources
Blocking in Statistics: Definition & Example Permuted Block Randomization: Definition & Example Lurking Variables: Definition & Examples
Featured Posts

Hey there. My name is Zach Bobbitt. I have a Masters of Science degree in Applied Statistics and I’ve worked on machine learning algorithms for professional businesses in both healthcare and retail. I’m passionate about statistics, machine learning, and data visualization and I created Statology to be a resource for both students and teachers alike. My goal with this site is to help you learn statistics through using simple terms, plenty of real-world examples, and helpful illustrations.
Leave a Reply Cancel reply
Your email address will not be published. Required fields are marked *
Join the Statology Community
Sign up to receive Statology's exclusive study resource: 100 practice problems with step-by-step solutions. Plus, get our latest insights, tutorials, and data analysis tips straight to your inbox!
By subscribing you accept Statology's Privacy Policy.
- Search Menu
- Advance Articles
- Author Guidelines
- Open Access Policy
- Self-Archiving Policy
- About Significance
- About The Royal Statistical Society
- Editorial Board
- Advertising & Corporate Services
- Journals on Oxford Academic
- Books on Oxford Academic

Article Contents
What is randomisation, why do we randomise, choosing a randomisation method, implementing the chosen randomisation method.
- < Previous
Randomisation: What, Why and How?
- Article contents
- Figures & tables
- Supplementary Data
Zoë Hoare, Randomisation: What, Why and How?, Significance , Volume 7, Issue 3, September 2010, Pages 136–138, https://doi.org/10.1111/j.1740-9713.2010.00443.x
- Permissions Icon Permissions
Randomisation is a fundamental aspect of randomised controlled trials, but how many researchers fully understand what randomisation entails or what needs to be taken into consideration to implement it effectively and correctly? Here, for students or for those about to embark on setting up a trial, Zoë Hoare gives a basic introduction to help approach randomisation from a more informed direction.
Most trials of new medical treatments, and most other trials for that matter, now implement some form of randomisation. The idea sounds so simple that defining it becomes almost a joke: randomisation is “putting participants into the treatment groups randomly”. If only it were that simple. Randomisation can be a minefield, and not everyone understands what exactly it is or why they are doing it.
A key feature of a randomised controlled trial is that it is genuinely not known whether the new treatment is better than what is currently offered. The researchers should be in a state of equipoise; although they may hope that the new treatment is better, there is no definitive evidence to back this hypothesis up. This evidence is what the trial is trying to provide.
You will have, at its simplest, two groups: patients who are getting the new treatment, and those getting the control or placebo. You do not hand-select which patient goes into which group, because that would introduce selection bias. Instead you allocate your patients randomly. In its simplest form this can be done by the tossing of a fair coin: heads, the patient gets the trial treatment; tails, he gets the control. Simple randomisation is a fair way of ensuring that any differences that occur between the treatment groups arise completely by chance. But – and this is the first but of many here – simple randomisation can lead to unbalanced groups, that is, groups of unequal size. This is particularly true if the trial is only small. For example, tossing a fair coin 10 times will only result in five heads and five tails about 25% of the time. We would have a 66% chance of getting 6 heads and 4 tails, 5 and 5, or 4 and 6; 33% of the time we would get an even larger imbalance, with 7, 8, 9 or even all 10 patients in one group and the other group correspondingly undersized.
The impact of an imbalance like this is far greater for a small trial than for a larger trial. Tossing a fair coin 100 times will result in imbalance larger than 60–40 less than 1% of the time. One important part of the trial design process is the statement of intention of using randomisation; then we need to establish which method to use, when it will be used, and whether or not it is in fact random.
Randomisation needs to be controlled: You would not want all the males under 30 to be in one trial group and all the women over 70 in the other
It is partly true to say that we do it because we have to. The Consolidated Standards of Reporting Trials (CONSORT) 1 , to which we should all adhere, tells us: “Ideally, participants should be assigned to comparison groups in the trial on the basis of a chance (random) process characterized by unpredictability.” The requirement is there for a reason. Randomisation of the participants is crucial because it allows the principles of statistical theory to stand and as such allows a thorough analysis of the trial data without bias. The exact method of randomisation can have an impact on the trial analyses, and this needs to be taken into account when writing the statistical analysis plan.
Ideally, simple randomisation would always be the preferred option. However, in practice there often needs to be some control of the allocations to avoid severe imbalances within treatments or within categories of patient. You would not want, for example, all the males under 30 to be in one group and all the females over 70 in the other. This is where restricted or stratified randomisation comes in.
Restricted randomisation relates to using any method to control the split of allocations to each of the treatment groups based on certain criteria. This can be as simple as generating a random list, such as AAABBBABABAABB …, and allocating each participant as they arrive to the next treatment on the list. At certain points within the allocations we know that the groups will be balanced in numbers – here at the sixth, eighth, tenth and 14th participants – and we can control the maximum imbalance at any one time.
Stratified randomisation sets out to control the balance in certain baseline characteristics of the participants – such as sex or age. This can be thought of as producing an individual randomisation list for each of the characteristics concerned.

© iStockphoto.com/dra_schwartz
Stratification variables are the baseline characteristics that you think might influence the outcome your trial is trying to measure. For example, if you thought gender was going to have an effect on the efficacy of the treatment then you would use it as one of your stratification variables. A stratified randomisation procedure would aim to ensure a balance of the two gender groups between the two treatment groups.
If you also thought age would be affecting the treatment then you could also stratify by age (young/old) with some sensible limits on what old and young are. Once you start stratifying by age and by gender, you have to start taking care. You will need to use a stratified randomisation process that balances at the stratum level (i.e. at the level of those characteristics) to ensure that all four strata (male/young, male/old, female/young and female/old) have equivalent numbers of each of the treatment groups represented.
“Great”, you might think. “I'll just stratify by all my baseline characteristics!” Better not. Stop and consider what this would mean. As the number of stratification variables increases linearly, the number of strata increases exponentially. This reduces the number of participants that would appear in each stratum. In our example above, with our two stratification variables of age and sex we had four strata; if we added, say “blue-eyed” and “overweight” to our criteria to give four stratification variables each with just two levels we would get 16 represented strata. How likely is it that each of those strata will be represented in the population targeted by the trial? In other words, will we be sure of finding a blue-eyed young male who is also overweight among our patients? And would one such overweight possible Adonis be statistically enough? It becomes evident that implementing pre-generated lists within each stratification level or stratum and maintaining an overall balance of group sizes becomes much more complicated with many stratification variables and the uncertainty of what type of participant will walk through the door next.
Does it matter? There are a wide variety of methods for randomisation, and which one you choose does actually matter. It needs to be able to do everything that is required of it. Ask yourself these questions, and others:
Can the method accommodate enough treatment groups? Some methods are limited to two treatment groups; many trials involve three or more.
What type of randomness, if any, is injected into the method? The level of randomness dictates how predictable a method is.
A deterministic method has no randomness, meaning that with all the previous information you can tell in advance which group the next patient to appear will be allocated to. Allocating alternate participants to the two treatments using ABABABABAB … would be an example.
A static random element means that each allocation is made with a pre-defined probability. The coin-toss method does this.
With a dynamic element the probability of allocation is always changing in relation to the information received, meaning that the probability of allocation can only be worked out with knowledge of the algorithm together with all its settings. A biased coin toss does this where the bias is recalculated for each participant.
Can the method accommodate stratification variables, and if so how many? Not all of them can. And can it cope with continuous stratification variables? Most variables are divided into mutually exclusive categories (e.g. male or female), but sometimes it may be necessary (or preferable) to use a continuous scale of the variable – such as weight, or body mass index.
Can the method use an unequal allocation ratio? Not all trials require equal-sized treatment groups. There are many reasons why it might be wise to have more patients receiving treatment A than treatment B 2 . However, an allocation ratio being something other than 1:1 does impact on the study design and on the calculation of the sample size, so is not something to be changing mid-trial. Not all allocation methods can cope with this inequality.
Is thresholding used in the method? Thresholding handles imbalances in allocation. A threshold is set and if the imbalance becomes greater than the threshold then the allocation becomes deterministic to reduce the imbalance back below the threshold.
Can the method be implemented sequentially? In other words, does it require that the total number of participants be known at the beginning of the allocations? Some methods generate lists requiring exactly N participants to be recruited in order to be effective – and recruiting participants is often one of the more problematic parts of a trial.
Is the method complex? If so, then its practical implementation becomes an issue for the day-to-day running of the trial.
Is the method suitable to apply to a cluster randomisation? Cluster randomisations are used when randomising groups of individuals to a treatment rather than the individuals themselves. This can be due to the nature of the treatment, such as a new teaching method for schools or a dietary intervention for families. Using clusters is a big part of the trial design and the randomisation needs to be handled slightly differently.
Should a response-adaptive method be considered? If there is some evidence that one treatment is better than another, then a response-adaptive method works by taking into account the outcomes of previous allocations and works to minimise the number of participants on the “wrong” treatment.
For multi-centred trials, how to handle the randomisations across the centres should be considered at this point. Do all centres need to be completely balanced? Are all centres the same size? Considering the various centres as stratification variables is one way of dealing with more than one centre.
Once the method of randomisation has been established the next important step is to consider how to implement it. The recommended way is to enlist the services of a central randomisation office that can offer robust, validated techniques with the security and back-up needed to implement many of the methods proposed today. How the method is implemented must be as clearly reported as the method chosen. As part of the implementation it is important to keep the allocations concealed, both those already done and any future ones, from as many people as possible. This helps prevent selection bias: a clinician may withhold a participant if he believes that based on previous allocations the next allocations would not be the “preferred” ones – see the section below on subversion.
Part of the trial design will be to note exactly who should know what about how each participant has been allocated. Researchers and participants may be equally blinded, but that is not always the case.
For example, in a blinded trial there may be researchers who do not know which group the participants have been allocated to. This enables them to conduct the assessments without any bias for the allocation. They may, however, start to guess, on the basis of the results they see. A measure of blinding may be incorporated for the researchers to indicate whether they have remained blind to the treatment allocated. This can be in the form of a simple scale tool for the researcher to indicate how confident they are in knowing which allocated group the participant is in by the end of an assessment. With psychosocial interventions it is often impossible to hide from the participants, let alone the clinicians, which treatment group they have been allocated to.
In a drug trial where a placebo can be prescribed a coded system can ensure that neither patients nor researchers know which group is which until after the analysis stage.
With any level of blinding there may be a requirement to unblind participants or clinicians at any point in the trial, and there should be a documented procedure drawn up on how to unblind a particular participant without risking the unblinding of a trial. For drug trials in particular, the methods for unblinding a participant must be stated in the trial protocol. Wherever possible the data analysts and statisticians should remain blind to the allocation until after the main analysis has taken place.
Blinding should not be confused with allocation concealment. Blinding prevents performance and ascertainment bias within a trial, while allocation concealment prevents selection bias. Bias introduced by poor allocation concealment may be thought of as a predictive bias, trying to influence the results from the outset, while the biases introduced by non-blinding can be thought of as a reactive bias, creating causal links in outcomes because of being in possession of information about the treatment group.
In the literature on randomisation there are numerous tales of how allocation schemes have been subverted by clinicians trying to do the best for the trial or for their patient or both. This includes anecdotal tales of clinicians holding sealed envelopes containing the allocations up to X-ray lights and confessing to breaking into locked filing cabinets to get at the codes 3 . This type of behaviour has many explanations and reasons, but does raise the question whether these clinicians were in a state of equipoise with regard to the trial, and whether therefore they should really have been involved with the trial. Randomisation schemes and their implications must be signed up to by the whole team and are not something that only the participants need to consent to.
Clinicians have been known to X-ray sealed allocation envelopes to try to get their patients into the preferred group in a trial
The 2010 CONSORT statement can be found at http://www.consort-statement.org/consort-statement/ .
Dumville , J. C. , Hahn , S. , Miles , J. N. V. and Torgerson , D. J. ( 2006 ) The use of unequal randomisation ratios in clinical trials: A review . Contemporary Clinical Trials , 27 , 1 – 12 .
Google Scholar
Shulz , K. F. ( 1995 ) Subverting randomisation in controlled trials . Journal of the American Medical Association , 274 , 1456 – 1458 .
Email alerts
Citing articles via.
- Recommend to Your Librarian
- Advertising & Corporate Services
- Journals Career Network
Affiliations
- Online ISSN 1740-9713
- Print ISSN 1740-9705
- Copyright © 2024 Royal Statistical Society
- About Oxford Academic
- Publish journals with us
- University press partners
- What we publish
- New features
- Open access
- Institutional account management
- Rights and permissions
- Get help with access
- Accessibility
- Advertising
- Media enquiries
- Oxford University Press
- Oxford Languages
- University of Oxford
Oxford University Press is a department of the University of Oxford. It furthers the University's objective of excellence in research, scholarship, and education by publishing worldwide
- Copyright © 2024 Oxford University Press
- Cookie settings
- Cookie policy
- Privacy policy
- Legal notice
This Feature Is Available To Subscribers Only
Sign In or Create an Account
This PDF is available to Subscribers Only
For full access to this pdf, sign in to an existing account, or purchase an annual subscription.
Experimental Design: Types, Examples & Methods
Saul Mcleod, PhD
Editor-in-Chief for Simply Psychology
BSc (Hons) Psychology, MRes, PhD, University of Manchester
Saul Mcleod, PhD., is a qualified psychology teacher with over 18 years of experience in further and higher education. He has been published in peer-reviewed journals, including the Journal of Clinical Psychology.
Learn about our Editorial Process
Olivia Guy-Evans, MSc
Associate Editor for Simply Psychology
BSc (Hons) Psychology, MSc Psychology of Education
Olivia Guy-Evans is a writer and associate editor for Simply Psychology. She has previously worked in healthcare and educational sectors.
On This Page:
Experimental design refers to how participants are allocated to different groups in an experiment. Types of design include repeated measures, independent groups, and matched pairs designs.
Probably the most common way to design an experiment in psychology is to divide the participants into two groups, the experimental group and the control group, and then introduce a change to the experimental group, not the control group.
The researcher must decide how he/she will allocate their sample to the different experimental groups. For example, if there are 10 participants, will all 10 participants participate in both groups (e.g., repeated measures), or will the participants be split in half and take part in only one group each?
Three types of experimental designs are commonly used:
1. Independent Measures
Independent measures design, also known as between-groups , is an experimental design where different participants are used in each condition of the independent variable. This means that each condition of the experiment includes a different group of participants.
This should be done by random allocation, ensuring that each participant has an equal chance of being assigned to one group.
Independent measures involve using two separate groups of participants, one in each condition. For example:
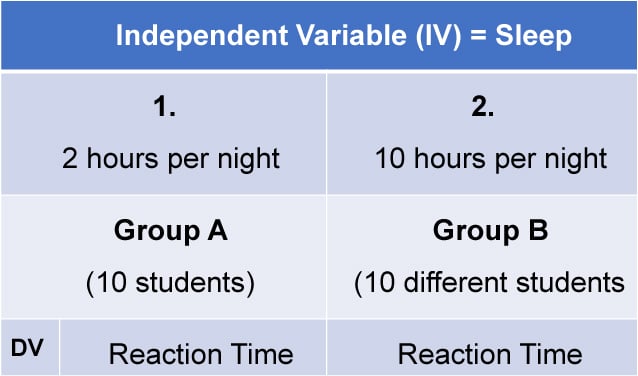
- Con : More people are needed than with the repeated measures design (i.e., more time-consuming).
- Pro : Avoids order effects (such as practice or fatigue) as people participate in one condition only. If a person is involved in several conditions, they may become bored, tired, and fed up by the time they come to the second condition or become wise to the requirements of the experiment!
- Con : Differences between participants in the groups may affect results, for example, variations in age, gender, or social background. These differences are known as participant variables (i.e., a type of extraneous variable ).
- Control : After the participants have been recruited, they should be randomly assigned to their groups. This should ensure the groups are similar, on average (reducing participant variables).
2. Repeated Measures Design
Repeated Measures design is an experimental design where the same participants participate in each independent variable condition. This means that each experiment condition includes the same group of participants.
Repeated Measures design is also known as within-groups or within-subjects design .
- Pro : As the same participants are used in each condition, participant variables (i.e., individual differences) are reduced.
- Con : There may be order effects. Order effects refer to the order of the conditions affecting the participants’ behavior. Performance in the second condition may be better because the participants know what to do (i.e., practice effect). Or their performance might be worse in the second condition because they are tired (i.e., fatigue effect). This limitation can be controlled using counterbalancing.
- Pro : Fewer people are needed as they participate in all conditions (i.e., saves time).
- Control : To combat order effects, the researcher counter-balances the order of the conditions for the participants. Alternating the order in which participants perform in different conditions of an experiment.
Counterbalancing
Suppose we used a repeated measures design in which all of the participants first learned words in “loud noise” and then learned them in “no noise.”
We expect the participants to learn better in “no noise” because of order effects, such as practice. However, a researcher can control for order effects using counterbalancing.
The sample would be split into two groups: experimental (A) and control (B). For example, group 1 does ‘A’ then ‘B,’ and group 2 does ‘B’ then ‘A.’ This is to eliminate order effects.
Although order effects occur for each participant, they balance each other out in the results because they occur equally in both groups.
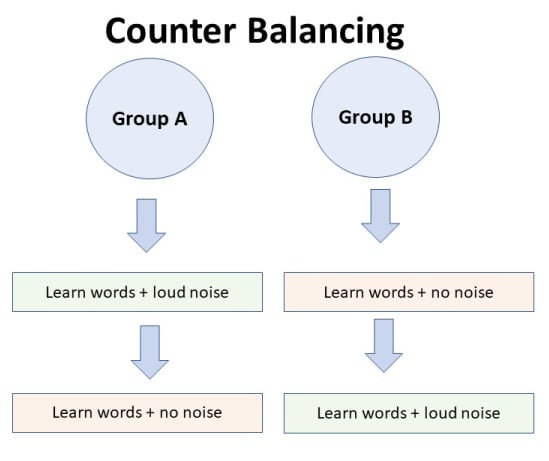
3. Matched Pairs Design
A matched pairs design is an experimental design where pairs of participants are matched in terms of key variables, such as age or socioeconomic status. One member of each pair is then placed into the experimental group and the other member into the control group .
One member of each matched pair must be randomly assigned to the experimental group and the other to the control group.
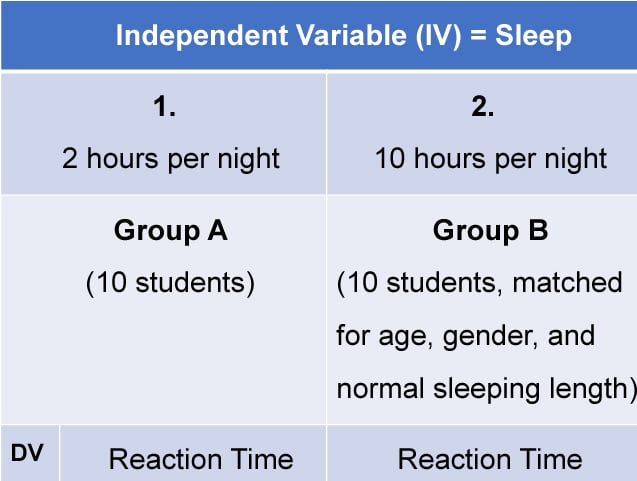
- Con : If one participant drops out, you lose 2 PPs’ data.
- Pro : Reduces participant variables because the researcher has tried to pair up the participants so that each condition has people with similar abilities and characteristics.
- Con : Very time-consuming trying to find closely matched pairs.
- Pro : It avoids order effects, so counterbalancing is not necessary.
- Con : Impossible to match people exactly unless they are identical twins!
- Control : Members of each pair should be randomly assigned to conditions. However, this does not solve all these problems.
Experimental design refers to how participants are allocated to an experiment’s different conditions (or IV levels). There are three types:
1. Independent measures / between-groups : Different participants are used in each condition of the independent variable.
2. Repeated measures /within groups : The same participants take part in each condition of the independent variable.
3. Matched pairs : Each condition uses different participants, but they are matched in terms of important characteristics, e.g., gender, age, intelligence, etc.
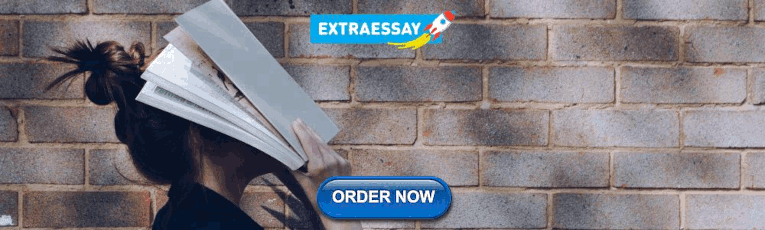
Learning Check
Read about each of the experiments below. For each experiment, identify (1) which experimental design was used; and (2) why the researcher might have used that design.
1 . To compare the effectiveness of two different types of therapy for depression, depressed patients were assigned to receive either cognitive therapy or behavior therapy for a 12-week period.
The researchers attempted to ensure that the patients in the two groups had similar severity of depressed symptoms by administering a standardized test of depression to each participant, then pairing them according to the severity of their symptoms.
2 . To assess the difference in reading comprehension between 7 and 9-year-olds, a researcher recruited each group from a local primary school. They were given the same passage of text to read and then asked a series of questions to assess their understanding.
3 . To assess the effectiveness of two different ways of teaching reading, a group of 5-year-olds was recruited from a primary school. Their level of reading ability was assessed, and then they were taught using scheme one for 20 weeks.
At the end of this period, their reading was reassessed, and a reading improvement score was calculated. They were then taught using scheme two for a further 20 weeks, and another reading improvement score for this period was calculated. The reading improvement scores for each child were then compared.
4 . To assess the effect of the organization on recall, a researcher randomly assigned student volunteers to two conditions.
Condition one attempted to recall a list of words that were organized into meaningful categories; condition two attempted to recall the same words, randomly grouped on the page.
Experiment Terminology
Ecological validity.
The degree to which an investigation represents real-life experiences.
Experimenter effects
These are the ways that the experimenter can accidentally influence the participant through their appearance or behavior.
Demand characteristics
The clues in an experiment lead the participants to think they know what the researcher is looking for (e.g., the experimenter’s body language).
Independent variable (IV)
The variable the experimenter manipulates (i.e., changes) is assumed to have a direct effect on the dependent variable.
Dependent variable (DV)
Variable the experimenter measures. This is the outcome (i.e., the result) of a study.
Extraneous variables (EV)
All variables which are not independent variables but could affect the results (DV) of the experiment. Extraneous variables should be controlled where possible.
Confounding variables
Variable(s) that have affected the results (DV), apart from the IV. A confounding variable could be an extraneous variable that has not been controlled.
Random Allocation
Randomly allocating participants to independent variable conditions means that all participants should have an equal chance of taking part in each condition.
The principle of random allocation is to avoid bias in how the experiment is carried out and limit the effects of participant variables.
Order effects
Changes in participants’ performance due to their repeating the same or similar test more than once. Examples of order effects include:
(i) practice effect: an improvement in performance on a task due to repetition, for example, because of familiarity with the task;
(ii) fatigue effect: a decrease in performance of a task due to repetition, for example, because of boredom or tiredness.

Related Articles
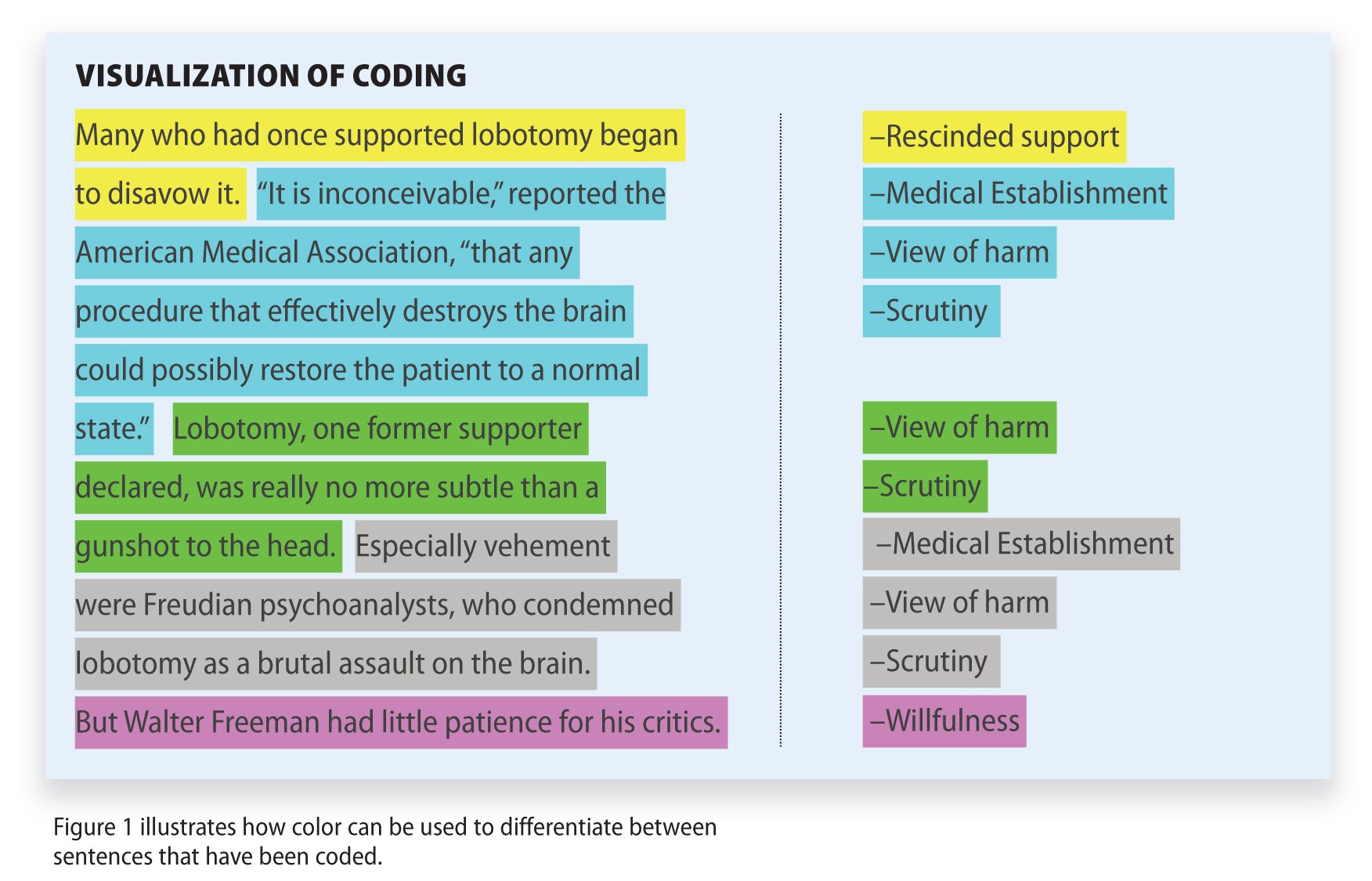
Research Methodology
Qualitative Data Coding
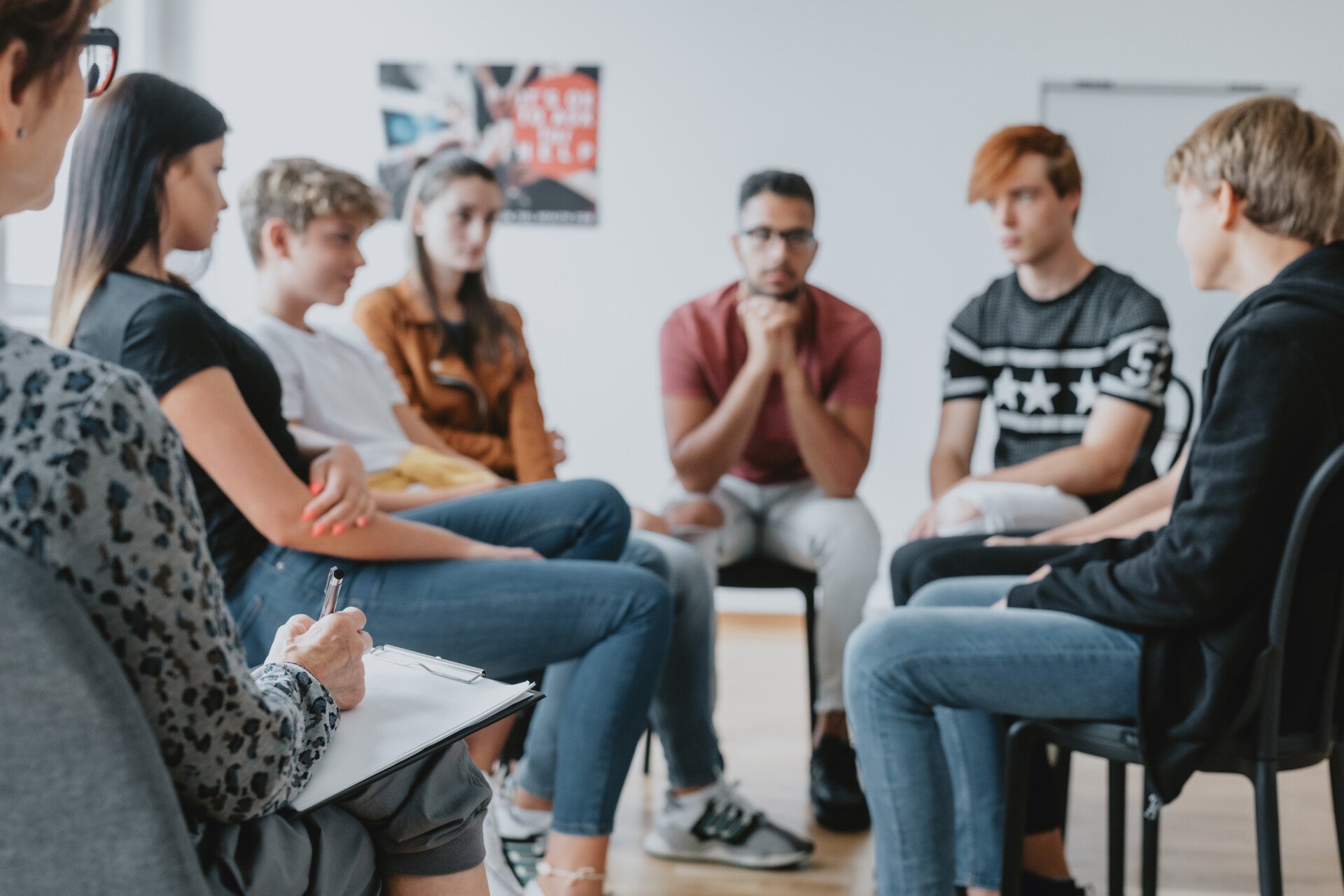
What Is a Focus Group?
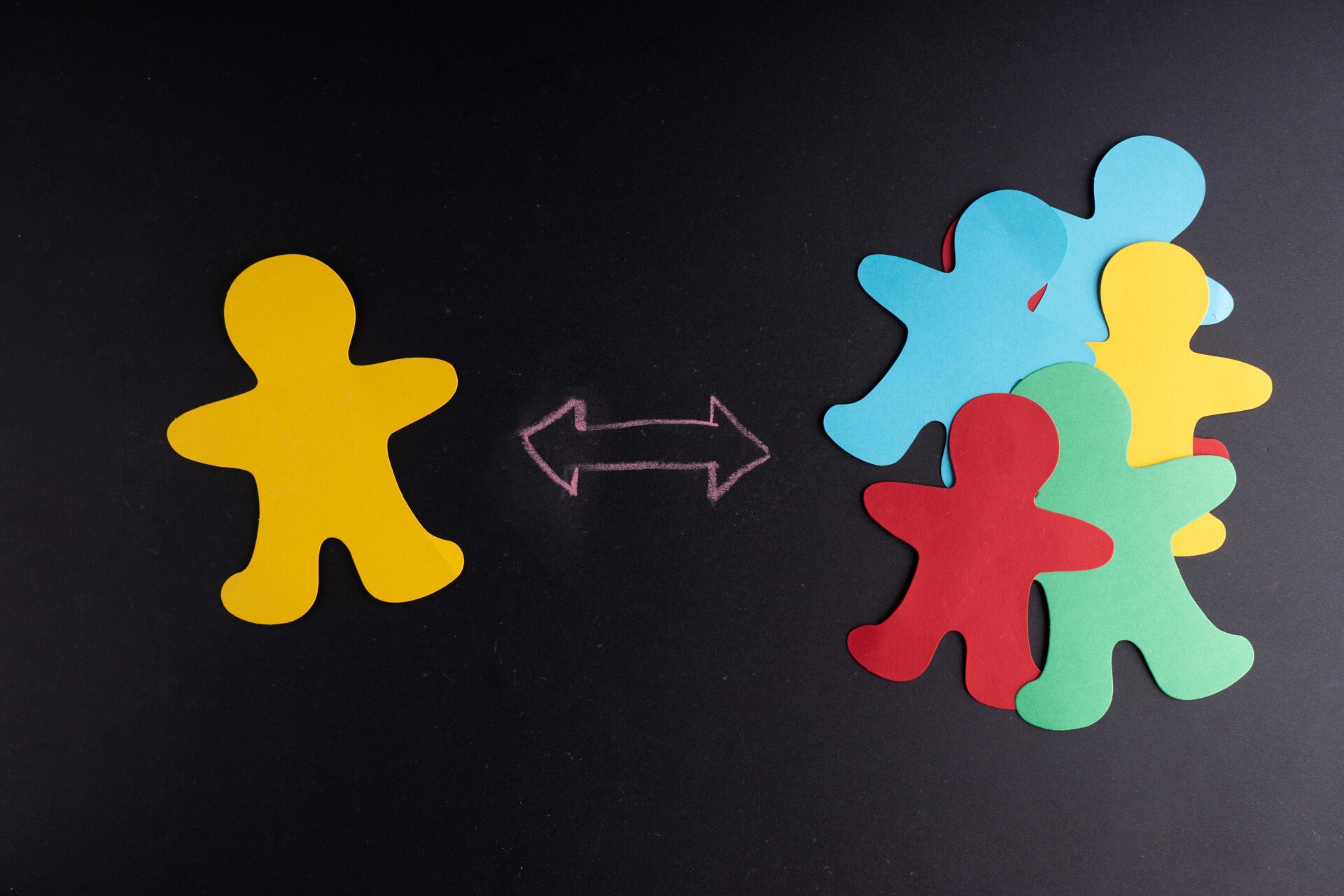
Cross-Cultural Research Methodology In Psychology
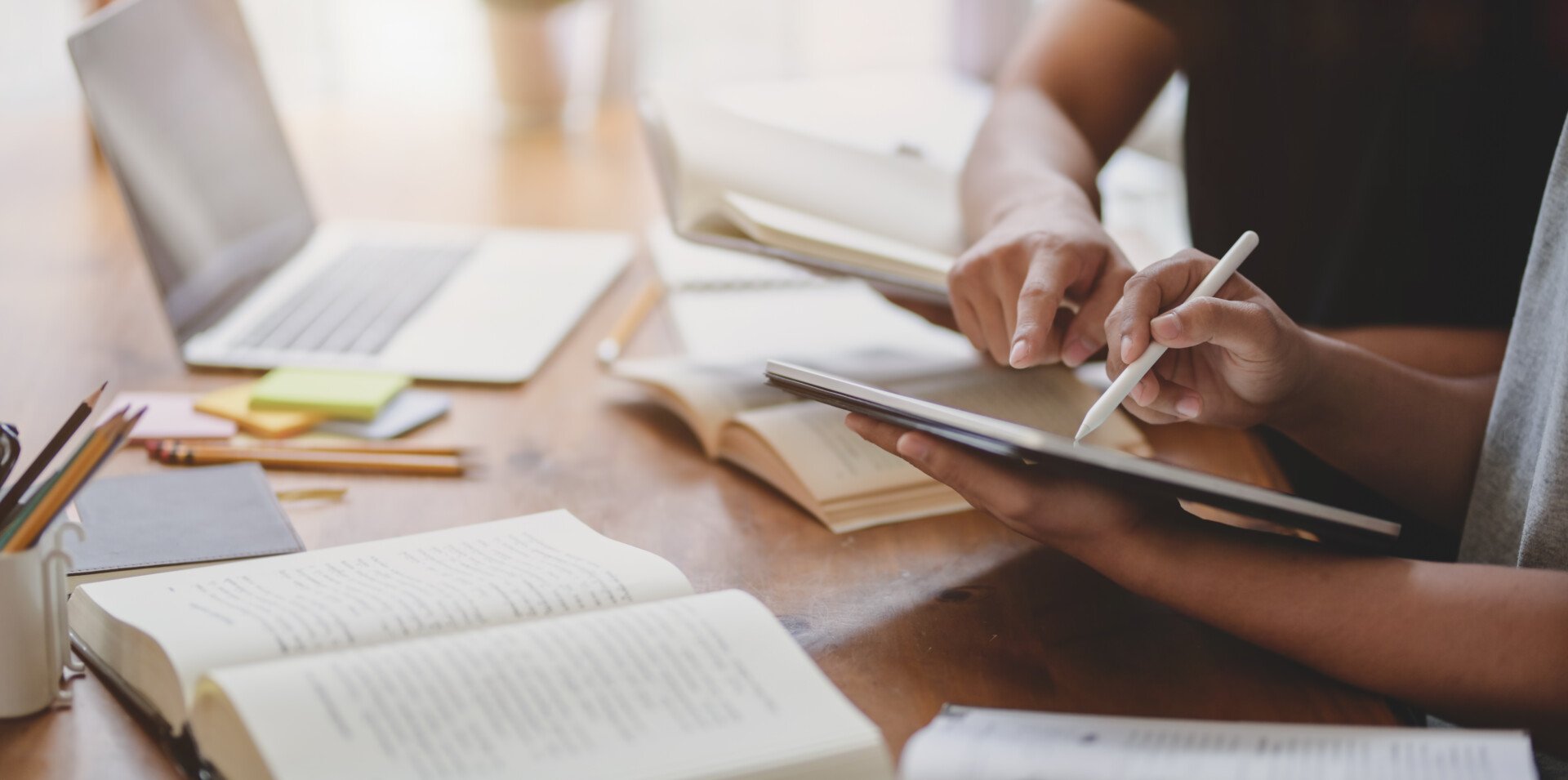
What Is Internal Validity In Research?
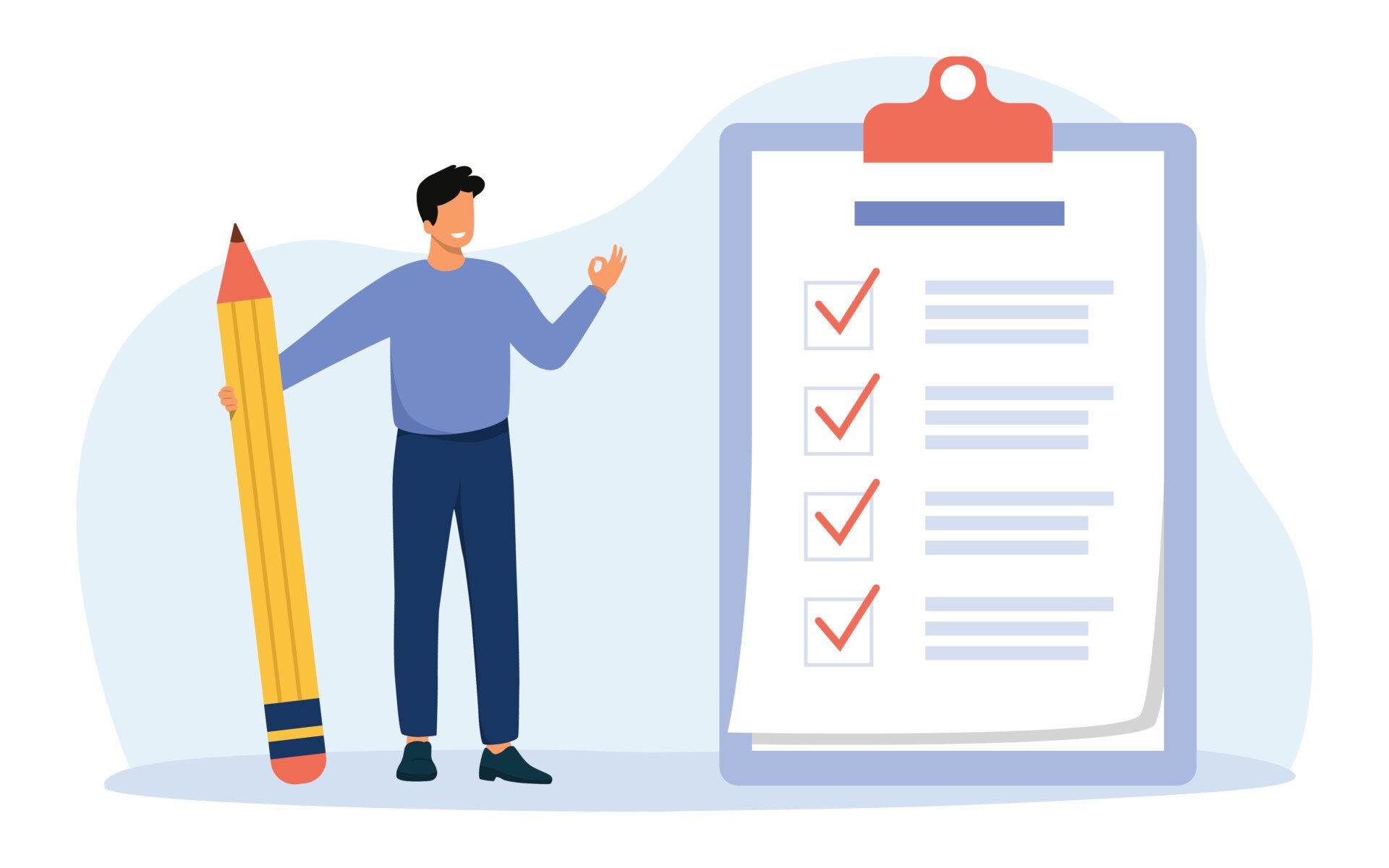
Research Methodology , Statistics
What Is Face Validity In Research? Importance & How To Measure
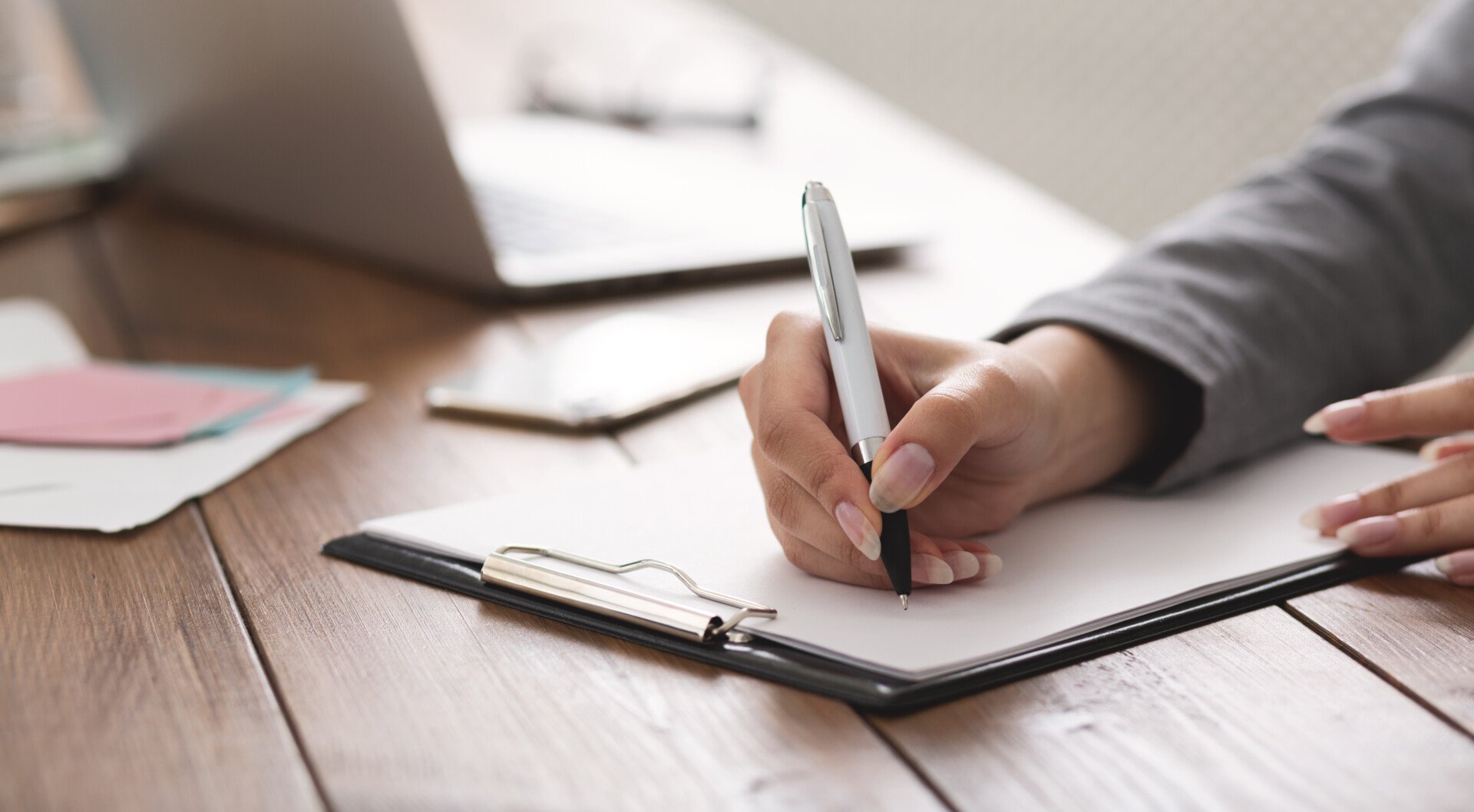
Criterion Validity: Definition & Examples
Live revision! Join us for our free exam revision livestreams Watch now →
Reference Library
Collections
- See what's new
- All Resources
- Student Resources
- Assessment Resources
- Teaching Resources
- CPD Courses
- Livestreams
Study notes, videos, interactive activities and more!
Psychology news, insights and enrichment
Currated collections of free resources
Browse resources by topic
- All Psychology Resources
Resource Selections
Currated lists of resources
Random Allocation
Random allocation of participants to experimental and control conditions is an extremely important process in research. Random allocation greatly decreases systematic error, so individual differences in responses or ability are far less likely to affect the results.
- Share on Facebook
- Share on Twitter
- Share by Email
Example Answers for Research Methods: A Level Psychology, Paper 2, June 2019 (AQA)
Exam Support
Research Methods: MCQ Revision Test 1 for AQA A Level Psychology
Topic Videos
Example Answers for Research Methods: A Level Psychology, Paper 2, June 2018 (AQA)
A level psychology topic quiz - research methods.
Quizzes & Activities
Our subjects
- › Criminology
- › Economics
- › Geography
- › Health & Social Care
- › Psychology
- › Sociology
- › Teaching & learning resources
- › Student revision workshops
- › Online student courses
- › CPD for teachers
- › Livestreams
- › Teaching jobs
Boston House, 214 High Street, Boston Spa, West Yorkshire, LS23 6AD Tel: 01937 848885
- › Contact us
- › Terms of use
- › Privacy & cookies
© 2002-2024 Tutor2u Limited. Company Reg no: 04489574. VAT reg no 816865400.

An official website of the United States government
The .gov means it’s official. Federal government websites often end in .gov or .mil. Before sharing sensitive information, make sure you’re on a federal government site.
The site is secure. The https:// ensures that you are connecting to the official website and that any information you provide is encrypted and transmitted securely.
- Publications
- Account settings
Preview improvements coming to the PMC website in October 2024. Learn More or Try it out now .
- Advanced Search
- Journal List
- Br J Cancer
- v.104(11); 2011 May 24
Statistical issues in the use of dynamic allocation methods for balancing baseline covariates
1 Department of Oncology, McMaster University, Ontario Clinical Oncology Group, Research Centre at Juravinski Hospital, G(60) Wing, 1st Floor, 711 Concession Street, Hamilton, ON, L8V 1C3 Canada
Background:
The procedure for allocating patients to a treatment arm in comparative clinical trials is frequently chosen with only minor deliberation. This decision may, however, ultimately impact the trial inference, credibility, and even validity of the trial analysis. Cancer researchers are increasingly using dynamic allocation (DA) procedures, which balance treatment arms across baseline prognostic factors for clinical trials in place of historical methods such as simple randomisation or allocation via the random permuted blocks.
This article gives an overview of DA methods, the statistical controversy that surrounds these procedures, and the potential impact on a clinical trial results.
Simple examples are provided to illustrate the use of DA methods and the inferential mistakes, notably on the P -value, if incorrect analyses are performed.
Interpretation:
The decision about which method to use for allocating patients should be given as much consideration as other aspects of a clinical trial. Appropriately choosing between methods can affect the statistical tests required and what inferences are possible, while affecting the trial credibility. Knowledge of the different methods is key to appropriate decision-making.
Randomised clinical trials have long been considered the gold standard in clinical research ( Concato et al , 2000 ). Strictly speaking, a randomised clinical trial is one where the allocation of patients to treatment arm occurs according to a random mechanism. In practice, this is typically performed using some sort of computer-generated list or random number generator. The allocation procedure is termed simple random sampling ( Zelen, 1974 ) and gives every patient the exact same chance of being allocated to receive each treatment. The use of a random mechanism is the cornerstone of these trials and is the basis for statistical theory and analysis of these trials ( Greenland, 1990 ). However, not all clinical trials use a strict randomisation procedure to allocate patients. Dynamic allocation (DA) methods ( Pocock and Simon, 1975 ), which balance prognostic factors between treatment groups, often referred to as minimisation ( Taves, 1974 ), are a primarily deterministic, non-random algorithm being implemented with increasing regularity by cancer researchers in clinical trials ( Pond et al , 2010 ). The effect on clinical trial interpretation when using these methods is not necessarily trivial, and has caused substantial debate regarding their usefulness and validity ( Senn, 2000 ). Some authors argue that for clinical trials, ‘if randomisation is the gold standard, minimisation may be the platinum standard’ ( Treasure and MacRae, 1998 ). Other authors have claimed these techniques are not necessary, possibly even detrimental, and use of these methods should be ‘strongly discouraged’ ( Committee for Proprietary Medicinal Products, 2003 ; Senn, 2004 ).
While this controversy appears to be well discussed in the statistical literature ( Rosenberger and Lachin, 2002 ; Buyse and McEntegart, 2004a ; Roes, 2004 ; Taves, 2004 ; Day et al , 2005 ; Senn et al , 2010 ), anecdotally it appears less appreciated in the clinical cancer research literature. Further, and possibly of greater concern, is there may be an underappreciation of the effect of non-randomised allocation on results, including the P -value, when using standard statistical analyses. Therefore, this manuscript was written with an aim to inform investigators who plan to incorporate DA methods in their clinical trials of some of the strengths and limitations of these techniques.
What is dynamic allocation?
Randomisation permits an unbiased comparison between patients allocated to different treatments ( Altman and Bland, 1999 ). Use of randomisation ensures asymptotic balancing of patients to treatment, and of baseline prognostic factors, including factors that are unknown at the time of randomisation. That is, the number of patients allocated to each treatment arm will approach equality, and prognostic factors will be equally balanced within patients across different treatments, in a clinical trial as the sample size increases infinitely . For any given trial, which has a finite sample size, however, there may be an imbalance between treatment arms in one, or more, known or unknown prognostic factors. Standard statistical theory is able to objectively quantify the possibility of an imbalance and even correct or adjust results for imbalances when they do occur ( Lachin, 1988 ). However, performing statistical adjustments for imbalanced prognostic factors are not recommended if the adjustment was not initially planned as this can lead to questions of multiple testing ( Pocock et al , 1987 ). Even when adjusting for an imbalanced prognostic factor is planned initially, a clinical trial having an imbalance, especially a large one, can be very concerning and possibly even detrimentally affect the credibility of the trial ( Buyse and McEntegart, 2004b ). Methods that reduce the possibility of a trial having a large imbalance between treatment arms for a known prognostic factor have therefore been proposed. Taves (1974) proposed an algorithm called minimisation, which is a deterministic method to allocate patients to treatment. The following year, Pocock and Simon (1975) independently presented a more general family of algorithms, called DA methods of which minimisation is one specific approach. Although earlier reviews indicated these methods were used very infrequently ( Altman and Dore, 1990 ; Lee and Feng, 2005 ), a recent cancer-specific review of multi-arm clinical trials indicated their use is increasing and no longer uncommon ( Pond et al , 2010 ).
To illustrate how DA methods work, let us consider a hypothetical two-arm clinical trial in breast cancer where three patient baseline covariates are considered prognostic: Her2-neu status (positive or negative), menopausal status (post-menopausal or pre-/peri-menopausal), and stage of disease (II or III). Assume the breakdown by treatment of the baseline prognostic factors for the first 19 patients is summarised in Table 1 and the 20th patient, a post-menopausal, Her2-neu-negative patient with stage II disease, is ready to be enrolled in the trial.
Using Taves minimisation algorithm, or equivalently the Pocock–Simon range method with allocation probability of 1, the number of previously enrolled patients with the same prognostic factor as the new patient is counted. The new patient would then be allocated to the treatment arm for which the sum of the previously enrolled patient prognostic factor counts is smallest. That is, if the 20th patient was allocated to treatment A, then there would be 5+1 Her2-neu-negative patients, 6+1 post-menopausal patients, and 7+1 stage II patients assigned to treatment A, which is summed to be (5+1)+(6+1)+(7+1)=21. Alternatively, if the 20th patient was allocated to treatment B, a total sum of (3+1)+(4+1)+(2+1)=12 is obtained. Since 12<21, the patient would be assigned to treatment B, minimising the imbalance.
Many authors have proposed, and continue to propose, modifications to these algorithms ( Wei, 1977 ; Begg and Iglewicz, 1990 ; Heritier et al , 2005 ; Perry et al , 2010 ). While some of these methods may modestly increase efficiency, most are rarely used. One modification that does appear to be utilised regularly is to add in a random component; thus, allocation is no longer completely deterministic. For example, in the hypothetical trial above, patient 20 would be allocated to treatment B with probability P , 0.5< P <1. A probability of P =0.8 has been shown to be most efficient ( Brown et al , 2005 ).
Other common allocation methods
Simple random sampling is the most basic allocation method ( Zelen, 1974 ). Each patient is assigned with equal probability to different treatment arms, regardless of all other considerations. Generally performed in practice by creating a randomisation list based on a random number table, or a computerised random number generator, it is equivalent to the notion of allocating patients by flipping a fair coin.
Another frequently used method is allocation via the random permuted blocks ( Zelen, 1974 ). Also known as block randomisation, stratified random sampling, or permuted block sampling, the patient allocation list is grouped into blocks of size 2 k (assuming 1 : 1 allocation) with k patients in each block assigned to each treatment. For example, if the block size is set to 4, then there are six ways, or permutations, in which two patients can be allocated to each treatment within a given block: AABB, ABAB, ABBA, BAAB, BABA, and BBAA. For each block, a permutation is selected at random and patients are assigned to treatment as they are enrolled according to that permutation. When that block is full, another permutation is selected for the next group of patients. A separate list of permuted blocks is created for each combination of strata. In the illustrative example, there would be 2 × 2 × 2=8 lists created, one for each combination of Her2-neu status, menopausal status, and disease stage. Although not well understood, allocation via the random permuted blocks method is not an entirely random technique. The last patient allocated within each block is completely determined by the allocation of the previous patients within that block. To reduce potential selection bias, a simple modification is to vary the block size throughout the trial.
Another dynamic procedure is a biased coin method ( Efron, 1971 ). Using this method, a patient is randomly allocated to the treatment arm which has fewer patients already accrued with probability P , where 0.5< P <1. If there is no difference in the number of patients treated in each arm, the next patient has an equal probability of being assigned to each treatment. An adaptive biased coin design ( Hofmeijer et al , 2008 ) is one where the value of P for each patient allocation depends on the degree of imbalance in the number of patients previously enrolled to each arm. Alternatively, one could perform simple random sampling as long as the imbalance in the number of patients previously enrolled to each arm overall, or within a centre or some other prognostic factor, is less than some value m , but switching to a biased coin design when the imbalance is m or larger.
Response-adaptive allocation is another DA method ( Zelen, 1969 ; Zhou et al , 2008 ). Patients are allocated to treatment as in a biased coin design, that is, allocated to one treatment arm with probability P , however, the value of P is determined based on the outcomes of patients previously enrolled in the study. If a treatment effect is observed, the value of P changes to allow patients a better opportunity of receiving the treatment with the best results. Over the course of the trial, this allocation method aims to optimise patient outcomes and more patients will receive the superior treatment.
Considerations when selecting an allocation method
A number of points should be considered when selecting an allocation method for use in a particular trial. For example, trials implementing DA methods must have sufficient statistical and programming support available to prevent avoidable algorithm and programming errors. An accessible and reliable centralised database is required for investigators to register patients and to perform the treatment allocation. Depending on the trial, this database may need to be coordinated with centre pharmacies or companies shipping treatment to the study centre. While this support is likely available within most large, cooperative groups, it may be less accessible in smaller centres or companies doing only a limited number of clinical trials. The cost and time required to develop these systems may not be feasible or viable given the small savings in sample size afforded by a balanced trial ( Senn, 2004 ). Alternatively, for very expensive novel therapies, a small savings in sample size could be financially advantageous, particularly if many of the systems are already in place. One might additionally consider potential imbalances in costs between individual study centres if discrepancies were to occur in the number of patients receiving each treatment at different sites. This might occur when there are differences in supportive care costs or in the number of follow-up visits – especially if a costly imaging procedure is included at each follow-up – between treatment arms.
Scientifically, the number and importance of known prognostic factors should factor in the decision of which allocation method to use. If the number and effect on the outcome of prognostic factors is large relative to the total trial sample size, preventing an imbalance might be of greater concern than a trial with few prognostic factors, which has only a modest effect on the outcome and a large sample size. The selection of method to use might be affected if some prognostic factors have a greater effect on outcome than others. Alternatively, one might be concerned with and choose an allocation algorithm based on the issue of selection bias, which might arise in an open-label trial or when the comparison treatments are extremely dissimilar (e.g., surgery vs non-surgical therapies). Selection bias arises when investigators can guess with improved probability the treatment future patients will be assigned to receive. Although concerning for deterministic algorithms when physicians know the characteristics of previous patients enrolled, the ability to guess assignment for future patients becomes negligible when centre is not used in the algorithm scheme or a random probability is included in the algorithm ( Brown et al , 2005 ).
Finally, one must think about the ultimate analysis and conclusions that might be inferred from a particular trial. Is it likely a sceptic will discount a result if an imbalance is present? Do investigators have sufficient statistical support to address any potential inferential concerns if they are raised? If the trial is being conducted in preparation for a regulatory submission, have the authorities provided any guidance? As recently as 2003, the Committee for Proprietary Medicinal Products noted that DA methods remained highly controversial and strongly advised against their use ( Committee for Proprietary Medicinal Products, 2003 ). Their concern is driven by logistical and practical flaws in previous applications using DA methods, and due to theoretical concerns ( Day et al , 2005 ). Although one certainly does not want to use DA methods which could prove problematic if there is no perceived benefit ( Day et al , 2005 ), the logistical and practical concerns can be addressed with proper algorithmic testing and attention ( Buyse and McEntegart, 2004a ). Of greater issue is the theoretical concerns which results from the fact that standard statistical analysis techniques are based on random allocation methods and DA methodology is not random. The distribution of possible outcomes depends on the allocation method used. Consequently, P -values obtained using tests which assume random allocation will not be correct when a DA algorithm was used. To illustrate the extent of this potential problem, an example is provided in the next section.
Illustrative example
Assume eight patients are allocated as part of a clinical trial to one of two treatment arms, as illustrated in Table 2 . For simplicity, let the rank order of the patient outcomes be listed and one-sided tests were performed to better illustrate the P -value calculations. Two-sided P -values could be calculated by doubling the one-sided P -value.

Additional information is available given that we know the prognostic factor status of all eight patients. A classical randomisation-based analysis might be to use linear regression, adjusting for the prognostic factor if, similar to the t -test, an investigator ignored that the data were rank order data and assumed the underlying distribution of the data was normal. In this case, the one-sided P -value is 0.0033. To do a permutation test, one starts with the four prognostic factor-positive patients and calculating the six ways in which these four patients can be allocated such that two patients receive treatment A and two receive treatment B (AABB, ABAB, ABBA, BBAA, BABA, and BAAB). This is similar to using a permuted block allocation method with block size of 4. Of the factor-positive patients, the observed outcome is the most extreme outcome in favour of treatment arm A, since the two patients allocated to arm A (patients 1 and 7) had better outcomes than the two patients allocated to arm B (patients 6 and 3). Therefore, the probability of this (for prognostic factor-positive patients) is 1/6. Similarly, of the factor-negative patients, the two patients allocated to arm A (patients 4 and 8) had better outcomes than the two patients allocated to treatment B (patients 2 and 5). Overall, then, the one-sided P -value is 1/6 × 1/6=0.0278.
The P -value is different, however, had one used Taves’ deterministic minimisation procedure. Using this algorithm, there are only four allocation possibilities for the factor-positive patients and four possibilities for the factor-negative patients. This is because it is impossible for the first two patients with identical prognostic factor status both to be allocated to the same treatment arm. Patient 1 is allocated to treatment arm A with probability 0.5 which means the next factor-positive patient (patient 3) is deterministically allocated to treatment arm B to minimise the imbalance. The next factor-positive patient (patient 6) is allocated to treatment arm B with probability 0.5, therefore, the next factor-positive patient (patient 7) must receive the opposite treatment (A). Hence, there are only four allocation possibilities: ABAB, ABBA, BABA, and BAAB.
In this situation, patients are paired. Patients 1 and 3 receive opposite treatments, as do patients 6 and 7. Of factor-negative patients, patients 2 and 4 receive opposite treatments, as do patients 5 and 8. In all pairs of patients, the patient who received treatment A did better than the patient who received treatment B. There is no possible way of allocating patients using minimisation, which would create a more extreme outcome favouring treatment A. Then, the one-sided P -value is 1/4 × 1/4=0.0625.
Finally, assume one used a biased coin method with P =0.8. There remain six possible ways of allocating two of four patients to treatment arm A, within each prognostic factor stratum. However, in this case, the probabilities are different for each possibility, unlike the random permuted blocks method. To calculate the P -value, one must calculate the probability of each scenario occurring. The resulting one-sided P -value is 0.0434.
In summary, obtaining the correct P -value depends on using the correct test for the allocation method which is used (see Table 3 ). The difference in the P -value because of different allocation methods can change the result from a ‘statistically significant at the α =0.05 level’ result to a non-statistically significant result.
Despite increasingly frequent implementation of DA methods which aim to balance prognostic factors between treatment arms ( Pond et al , 2010 ), their use remains controversial ( Senn, 2000 ). Many of the early criticisms of these methods, that they are too complex, might hinder investigators from performing clinical trials, or that they require a centralised database which might be practically difficult ( Peto et al , 1976 ), are less consequential today due to more powerful computers, instant communication, and increasing awareness of the need for comparative clinical trials ( Schulz et al , 2010 ). Other criticisms remarking on programming errors can be remedied through vigilance and repeated testing of allocation programs by dedicated statistical and programming teams. Finally, there are concerns that blinding can be compromised when using these methods ( Day et al , 2005 ). A number of common strategies can be employed to reduce the possibility of unblinding, such as adding a random component and not using centre as a stratification factor ( Brown et al , 2005 ). Importantly, one should not reveal the allocation procedure to investigators involved in enrolling patients and, whenever possible, blind them to the treatment the patients actually receive.
The bigger inferential concern remains, that of using common, but incorrect, statistical analyses which assume random allocation of patients. The use of common statistical tests is in part due to the wide recognition of these tests, but also because it is extremely complex (if not impossible) to perform the correct permutation test when sample sizes, and the number of stratification factors, increase ( Knijnenburg et al , 2009 ). One argument for the validity of these tests is that the order of patient accrual can be considered random, although this is not universally accepted ( Simon, 1979 ). Simulations have shown the impact of using random allocation tests instead of permutation tests is small when sample sizes are reasonably large and adjustment for prognostic factors is performed ( Birkett, 1985 ; Kalish and Begg, 1987 ; Tu et al , 2000 ); thus, the use of standard tests should not be much of a concern ( McEntegart, 2003 ). It is further noted that use of statistical tests which assume random allocation are frequently applied when using permuted block methods, another non-random procedure, and there is little concern of the impact on these results.
While most authors advocate adjusting for covariates used as stratification factors in the final analysis, the argument of increased credibility of DA methods over simple random sampling is related to the unadjusted, univariate model ( Buyse and McEntegart, 2004a ). As the presented example shows there might be substantial differences between the unadjusted and adjusted analyses, and it is always important to investigate when there are differences.
Numerous options are available to investigators conducting a cancer clinical trial for treatment allocation between comparison arms. Careful consideration should occur in the trial design phase to select the method best suited for a given trial. This decision could impact the inferential ability and credibility of a trial and should not be perceived as trivial. Knowledge of the impact of the allocation method on the trial is essential to proper understanding of the results, regardless of procedure used.
In summary, DA should be considered a valid alternative to randomisation or allocation via the random permuted blocks method, particularly for small to moderate-sized clinical trials with multiple significant prognostic factors having modest to large treatment effects, as is common in oncology. While it is recommended that only a few factors be used when using the random permuted blocks method – as a rule of thumb, the total number of cells should be less than n /2 – DA methods can handle many factors without difficulty ( Therneau, 1993 ). Even with DA methods, however, it is advised that only factors with a known, large prognostic effect be included and there should be at least five patients per cell ( Rovers et al , 2000 ). Using a balanced coin algorithm and incorporating a random element with probability P=0.8 is most efficient for reducing the ability to predict future patient allocations while maintaining good balance ( Brown et al , 2005 ). While statistical tests based on the assumption of randomisation may give similar results, the P -value will only be precisely correct when using the statistical test corresponding to the allocation algorithm used. At a minimum, investigators should perform multivariable analyses which adjust for all factors used in the DA algorithm and perform appropriate sensitivity analyses ( Committee for Proprietary Medicinal Products, 2003 ).
Presented in part at the 45th Annual Meeting of the American Society of Clinical Oncology, Orlando, FL, USA in June 2009.
The author declares no conflict of interest.
- Altman DG, Bland JM (1999) Treatment allocation in controlled trials: why randomise ? Br Med J 318 : 1209. [ PMC free article ] [ PubMed ] [ Google Scholar ]
- Altman DG, Dore CJ (1990) Randomisation and baseline comparisons in clinical trials . Lancet 335 : 149–153 [ PubMed ] [ Google Scholar ]
- Begg CB, Iglewicz B (1990) A treatment allocation procedure for sequential clinical trials . Biometrics 36 : 81–90 [ PubMed ] [ Google Scholar ]
- Birkett NJ (1985) Adaptive allocation in randomized control trials . Control Clin Trials 6 : 146–155 [ PubMed ] [ Google Scholar ]
- Brown S, Thorpe H, Hawkins K, Brown J (2005) Minimization – reducing predictability for multi-centre trials whilst retaining balance within centre . Stat Med 24 : 3715–3727 [ PubMed ] [ Google Scholar ]
- Buyse M, McEntegart DJ (2004a) Achieving balance in clinical trials: an unbalanced view from the European regulators . Appl Clin Trials 13 (5): 36–40 [ Google Scholar ]
- Buyse M, McEntegart D (2004b) More nonSennse about balance in clinical trials . Appl Clin Trials 14 (2): 13.7 [ Google Scholar ]
- Committee for Proprietary Medicinal Products (2003) Points to Consider on Adjustment for Baseline Covariates . CPMP/EWP/2863/99. Retrieved from http://www.emea.europa.eu/pdfs/human/ewp/286399en.pdf [ PubMed ]
- Concato J, Shah N, Horwitz RI (2000) Randomized, controlled trials, observational studies, and the hierarchy of research designs . N Engl J Med 342 (25): 1887–1892 [ PMC free article ] [ PubMed ] [ Google Scholar ]
- Day S, Grouin J-M, Lewis JA (2005) Achieving balance in clinical trials: to say the guidance does not cite any references to support its view is an irrelevant argument . Appl Clin Trials 14 (1): 24 [ Google Scholar ]
- Efron B (1971) Forcing a sequential experiment to be balanced . Biometrika 58 (3): 403–417 [ PubMed ] [ Google Scholar ]
- Greenland S (1990) Randomization, statistics, and causal inference . Epidemiology 1 : 421–429 [ PubMed ] [ Google Scholar ]
- Heritier S, Gebski V, Pillai A (2005) Dynamic balancing randomization in controlled clinical trials . Stat Med 24 : 3729–3741 [ PubMed ] [ Google Scholar ]
- Hofmeijer J, Anema PC, van der Tweel I (2008) New algorithm for treatment allocation reduced selection bias and loss of power in small trials . J Clin Epidemiol 61 (2): 119–124 [ PubMed ] [ Google Scholar ]
- Kalish LA, Begg CB (1987) The impact of treatment allocation procedures on nominal significance levels and bias . Control Clin Trials 8 : 121–135 [ PubMed ] [ Google Scholar ]
- Knijnenburg TA, Wessels LFA, Reinders MJT, Shmulevich I (2009) Fewer permutations, more accurate P -values . Bioinformatics 25 : 161–168 [ PMC free article ] [ PubMed ] [ Google Scholar ]
- Lachin JM (1988) Statistical properties of randomization in clinical trials . Control Clin Trials 9 (4): 289–311 [ PubMed ] [ Google Scholar ]
- Lee JJ, Feng L (2005) Randomized phase II designs in cancer clinical trials: current status and future directions . J Clin Oncol 23 (19): 4450–4457 [ PubMed ] [ Google Scholar ]
- McEntegart DJ (2003) The pursuit of balance using stratified and dynamic randomization techniques: an overview . Drug Inf J 37 (3): 293–308 [ Google Scholar ]
- Perry M, Faes M, Reelick MF, Olde Rikkert MG, Borm GF (2010) Studywise minimization: a treatment allocation method that improves balance among treatment groups and makes allocation unpredictable . J Clin Epidemiol 63 (10): 1118–1122 [ PubMed ] [ Google Scholar ]
- Peto R, Pike MC, Armitage P, Breslow NE, Cox DR, Howard SV, Mantel N, McPherson K, Peto J, Smith PG (1976) Design and analysis of randomized clinical trials requiring prolonged observation of each patient. I. Introduction and design . Br J Cancer 34 (6): 585–612 [ PMC free article ] [ PubMed ] [ Google Scholar ]
- Pocock SJ, Hughes MD, Lee RJ (1987) Statistical problems in the reporting of clinical trials. A survey of three medical journals . N Engl J Med 317 (7): 426–432 [ PubMed ] [ Google Scholar ]
- Pocock SJ, Simon R (1975) Sequential treatment assignment with balancing for prognostic factors in the controlled clinical trial . Biometrics 31 : 103–115 [ PubMed ] [ Google Scholar ]
- Pond GR, Tang PA, Welch SA, Chen EX (2010) Trends in the application of dynamic allocation methods in multi-arm cancer clinical trials . Clin Trials 7 (3): 227–234 [ PubMed ] [ Google Scholar ]
- Ranstam J (2009) Sampling uncertainty in medical research . Osteoarthritis Cartilage 17 (11): 1416–1419 [ PubMed ] [ Google Scholar ]
- Roes KCB (2004) Dynamic allocation as a balancing act . Pharm Stat 3 : 187–191 [ Google Scholar ]
- Rosenberger WF, Lachin JM (2002) Randomisation in Clinical Trials. Theory and Practice . New York: Wiley [ Google Scholar ]
- Rovers MM, Straatman H, Zielhuis GA, Ingels K, van der Wilt GJ (2000) Using a balancing procedure in multicenter clinical trials: simulation of patient allocation based on a trial of ventilation tubes for otitis media with effusion in infants . Int J Technol AssessHealth Care 16 : 276–280 [ PubMed ] [ Google Scholar ]
- Schulz KF, Altman DG, Moher D, for the CONSORT Group (2010) CONSORT 2010 statement: updated guidelines for reporting parallel group randomised trials . BMC Med 8 : 18. [ PMC free article ] [ PubMed ] [ Google Scholar ]
- Senn SJ (2000) Consensus and controversy in pharmaceutical statistics (with discussion) . Statistician 49 : 135–176 [ Google Scholar ]
- Senn SJ (2004) Unbalanced claims for balance . Appl Clin Trials 13 (6): 14–15 [ Google Scholar ]
- Senn S, Anisimov VV, Fedorov VV (2010) Comparisons of minimization and Atkinson's algorithm . Stat Med 29 : 721–730 [ PubMed ] [ Google Scholar ]
- Simon R (1979) Restricted randomization designs in clinical trials . Biometrics 35 : 503–512 [ PubMed ] [ Google Scholar ]
- Taves DR (1974) Minimization: a new method of assigning patients to treatment and control groups . Clin Pharmacol Ther 15 : 443–453 [ PubMed ] [ Google Scholar ]
- Taves DR (2004) Faulty assumptions in Atkinson's criteria for clinical trial design . J R Stat Soc Series A 167 (1): 179–180 [ Google Scholar ]
- Therneau TM (1993) How many stratification factors are ‘too many’ to use in a randomization plan ? Control Clin Trials 14 : 98–108 [ PubMed ] [ Google Scholar ]
- Treasure T, MacRae K (1998) Minimisation: the platinum standard for trials? (Randomisation doesn’t guarantee similarity of groups; minimisation does) . Br Med J 317 (5): 362–363 [ PMC free article ] [ PubMed ] [ Google Scholar ]
- Tu D, Shalay K, Pater J (2000) Adjustments of treatment effect for covariates in clinical trials: statistical and regulatory issues . Drug Inf J 34 : 511–523 [ Google Scholar ]
- Wei L-J (1977) A class of designs for sequential clinical trials . J Am Stat Assoc 72 (358): 382–386 [ Google Scholar ]
- Zelen M (1969) Play the winner rule and the controlled clinical trial . J Am Stat Assoc 64 : 131–146 [ Google Scholar ]
- Zelen M (1974) The randomization and stratification of patients to clinical trials . J Chronic Dis 27 : 365–375 [ PubMed ] [ Google Scholar ]
- Zhou X, Liu S, Kim ES, Herbst RS, Lee JJ (2008) Bayesian adaptive design for targeted therapy development in lung cancer – a step toward personalized medicine . Clin Trials 5 : 181–193 [ PMC free article ] [ PubMed ] [ Google Scholar ]
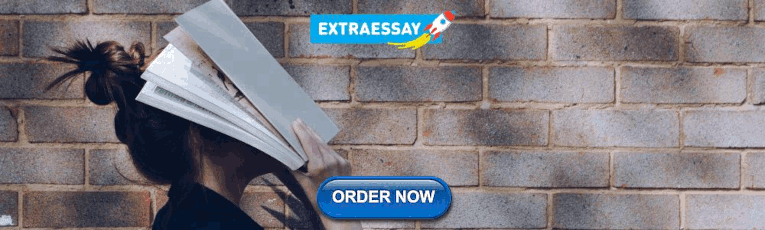
IMAGES
VIDEO
COMMENTS
Random allocation is a technique that chooses individuals for treatment groups and control groups entirely by chance with no regard to the will of researchers or patients' condition and preference. This allows researchers to control all known and unknown factors that may affect results in treatment groups and control groups.
Randomization is a statistical process in which a random mechanism is employed to select a sample from a population or assign subjects to different groups. The process is crucial in ensuring the random allocation of experimental units or treatment protocols, thereby minimizing selection bias and enhancing the statistical validity. It facilitates the objective comparison of treatment effects in ...
1. All of the students select a marble from a bag, and the 50 students with green marbles participate. 2. Jared asks 50 of his friends to participate in the study. 3. The names of all of the students in the school are put in a bowl and 50 names are drawn. 4.
No. Random selection, also called random sampling, is the process of choosing all the participants in a study. After the participants are chosen, random allocation, also called random assignment ...
Random Assignment in Experiments | Introduction & Examples. Published on March 8, 2021 by Pritha Bhandari.Revised on June 22, 2023. In experimental research, random assignment is a way of placing participants from your sample into different treatment groups using randomization. With simple random assignment, every member of the sample has a known or equal chance of being placed in a control ...
7.3 Random allocation vs random sampling. Random sampling and random allocation are two different concepts (Fig. 7.4), that serve two different purposes, but are often confused:. Random sampling allows results to be generalised to a larger population, and impacts external validity. It concerns how the sample is found to study.; Random allocation tries to eliminate confounding issues, by ...
by Zach Bobbitt February 9, 2021. In the field of statistics, randomization refers to the act of randomly assigning subjects in a study to different treatment groups. For example, suppose researchers recruit 100 subjects to participate in a study in which they hope to understand whether or not two different pills have different effects on blood ...
A static random element means that each allocation is made with a pre-defined probability. The coin-toss method does this. With a dynamic element the probability of allocation is always changing in relation to the information received, meaning that the probability of allocation can only be worked out with knowledge of the algorithm together ...
Random assignment or random placement is an experimental technique for assigning human participants or animal subjects to different groups in an experiment (e.g., a treatment group versus a control group) using randomization, such as by a chance procedure (e.g., flipping a coin) or a random number generator. This ensures that each participant or subject has an equal chance of being placed in ...
Random Allocation Randomly allocating participants to independent variable conditions means that all participants should have an equal chance of taking part in each condition. The principle of random allocation is to avoid bias in how the experiment is carried out and limit the effects of participant variables.
Random assignment helps you separation causation from correlation and rule out confounding variables. As a critical component of the scientific method, experiments typically set up contrasts between a control group and one or more treatment groups. The idea is to determine whether the effect, which is the difference between a treatment group ...
The key phrase in an RCT is "random allocation" and it must be done properly, using two steps: generating the random sequence, implementing the sequence in a way that it is concealed. One should consider using a random numbers table or computer program to generate the random allocation sequence.
The different genotypes possible from the same mating have been beautifully randomised by the meiotic process. 22. This principle—that analysis of genetic data is analogous to that of a randomized experiment—has, in epidemiology, been termed "Mendelian randomization." 23 This depends on the basic (but approximate) laws of Mendelian ...
Random allocation is a technique that chooses individuals for treatment groups and control groups entirely by chance with no regard to the will of researchers or patients' condition and preference. This allows researchers to control all known and unknown factors that may affect results in treatment groups and control groups.
Neyman allocation In Lecture 19, we described theoptimal allocation schemeforstrati ed random sampling, calledNeyman allocation. Neyman allocation schememinimizes variance V[X n] subject to P N k=1 n = n. Theorem The sample sizes n 1;:::;n L that solve the optimization problem V[X n] = XL k=1!2 k ˙2 k n k!min s:t: XL k=1 n k = n are given by ...
Random allocation greatly decreases systematic error, so individual differences in responses or ability are far less likely to affect the results. Random allocation of participants to experimental and control conditions is an extremely important process in research. Random allocation greatly decreases systematic error, so individual differences ...
Randomized schemes for treatment allocation are preferable in most circumstances. When choosing an allocation scheme for a clinical trial, there are three technical considerations: reducing bias; producing a balanced comparison; quantifying errors attributable to chance. Randomization procedures provide the best opportunity for achieving these ...
This is a proportional allocation that will maintain a steady sampling fraction throughout the population. n h = n ⋅ N h N. This does not take into consideration the variability within each stratum and is not the optimal choice. If the cost of sampling from each stratum is the same, then the optimal allocation (the allocation with the lowest ...
Allocation bias happens when researchers don't use an appropriate randomization technique, leading to marked, systematic differences between experimental groups and control groups. It can also happen further down the line, if clinical staff don't follow the procedures set in place by the researchers. One study (Salonen et al, 1992 ...
The randomized controlled trial (RCT) has become the standard by which studies of therapy are judged. The key to the RCT lies in the random allocation process. When done correctly in a large enough sample, random allocation is an effective measure in reducing bias. In this article we describe the random allocation process.
Other methods include using a shuffled deck of cards (e.g., even - control, odd - treatment) or throwing a dice (e.g., below and equal to 3 - control, over 3 - treatment). A random number table found in a statistics book or computer-generated random numbers can also be used for simple randomization of subjects.
Random allocation is essential for randomized controlled trials and is considered one of the hallmarks of a quality trial. Allocation concealment is a way to ensure proper randomization; without it, selection bias and confounding biases render the study invalid. In addition, trials that don't have proper concealment may report much larger ...
It is further noted that use of statistical tests which assume random allocation are frequently applied when using permuted block methods, another non-random procedure, and there is little concern of the impact on these results. ... Greenland S (1990) Randomization, statistics, and causal inference. Epidemiology 1: 421-429 [Google Scholar]