Task set and prefrontal cortex
Affiliation.
- 1 Department of Cognitive Neuroscience, Graduate School of Medicine, The University of Tokyo, 7-3-1 Hongo, Bunkyo-ku, Tokyo 113-0033, Japan. [email protected]
- PMID: 18558854
- DOI: 10.1146/annurev.neuro.31.060407.125642
A task set is a configuration of cognitive processes that is actively maintained for subsequent task performance. Single-unit and brain-imaging studies have identified the neural correlates for task sets in the prefrontal cortex. Here I examine whether the neural data obtained thus far are sufficient to explain the behaviors that have been illustrated within the conceptual framework of task sets. I first discuss the selectivity of neural activity in representing a specific task. I then discuss the competitions between neural representations of task sets during task switch. Finally I discuss how, in neural terms, a task set is implemented to facilitate task performance. The processes of representing, updating, and implementing task sets occur in parallel at multiple levels of brain organization. Neural accounts of task sets demonstrate that the brain determines our thoughts and behaviors.
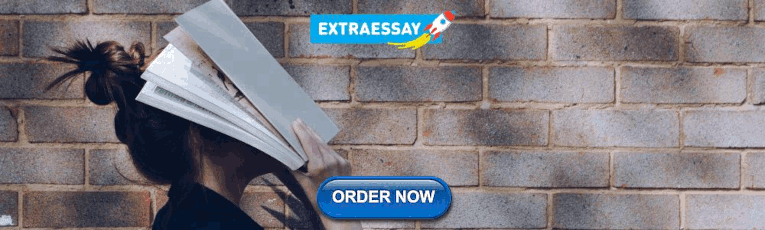
Publication types
- Research Support, Non-U.S. Gov't
- Action Potentials / physiology
- Behavior / physiology
- Brain Mapping
- Cognition / physiology*
- Mental Processes / physiology
- Nerve Net / physiology*
- Neurons / physiology*
- Prefrontal Cortex / anatomy & histology
- Prefrontal Cortex / physiology*
- Psychomotor Performance / physiology*
- Reference Manager
- Simple TEXT file
People also looked at
Original research article, what are task-sets: a single, integrated representation or a collection of multiple control representations.
- 1 Department Psychologie, Allgemeine und Experimentelle Psychologie I, Ludwig-Maximilians-Universität München, München, Germany
- 2 Birkbeck College, University of London, London, UK
Performing two randomly alternating tasks typically results in higher reaction times (RTs) following a task switch, relative to a task repetition. These task switch costs (TSC) reflect processes of switching between control settings for different tasks. The present study investigated whether task sets operate as a single, integrated representation or as an agglomeration of relatively independent components. In a cued task switch paradigm, target detection (present/absent) and discrimination (blue/green/right-/left-tilted) tasks alternated randomly across trials. The target was either a color or an orientation singleton among homogeneous distractors. Across two trials, the task and target-defining dimension repeated or changed randomly. For task switch trials, agglomerated task sets predict a difference between dimension changes and repetitions: joint task and dimension switches require full task set reconfiguration, while dimension repetitions permit re-using some control settings from the previous trial. By contrast, integrated task sets always require full switches, predicting dimension repetition effects (DREs) to be absent across task switches. RT analyses showed significant DREs across task switches as well as repetitions supporting the notion of agglomerated task sets. Additionally, two event-related potentials (ERP) were analyzed: the Posterior-Contralateral-Negativity (PCN) indexing spatial selection dynamics, and the Sustained-Posterior-Contralateral-Negativity (SPCN) indexing post-selective perceptual/semantic analysis. Significant DREs across task switches were observed for both the PCN and SPCN components. Together, DREs across task switches for RTs and two functionally distinct ERP components suggest that re-using control settings across different tasks is possible. The results thus support the “agglomerated-task-set” hypothesis, and are inconsistent with “integrated task sets.”
Introduction
Surviving in an environment in which both internal and external conditions change dynamically presupposes an ability to change between control settings for old and new tasks. A successful switch implies that the set of expectations about the environment (the topic of the present special issue) which was relevant in the previous task episode is replaced by one appropriate for the task at hand. Such switching processes are usually investigated in paradigms in which two or more different tasks vary across trials, requiring a change, on task-switch trials, in the internal control settings so as to fit the current task requirements. In cued task switching , prior to the stimulus display's onset, a cue is presented specifying the task to be performed on the upcoming trial. Across two consecutive trials, the task can either repeat or change. Reaction times (RTs) and errors are typically elevated for task switches relative to repetitions ( Allport et al., 1994 ; Rogers and Monsell, 1995 ). Such task switch costs (TSCs) imply the existence of extra, time-consuming processes invoked on task switch trials, but not (or to a lesser degree) on repetition trials. To comprehensively account for TSCs, answers to two related, yet separable questions are necessary: first, what cognitive mechanisms give rise to the TSCs, and, second, how are the representations on which these mechanisms operate organized? The present study focused on the latter issue—more precisely, on whether or not having to change some expectations automatically triggers a change in all expectations about task-relevant properties of the environment.
Determinants of TSCs
The available literature offers two dominant approaches to the question of what cognitive mechanisms give rise to TSCs. According to the first, TSCs reflect the extra time it takes to reconfigure control settings from the previously relevant to the currently relevant task demands ( Monsell and Driver, 2000 ; Monsell et al., 2003 ). Reconfiguration is achieved by means of a special executive function (or set of functions) which is active on task switches and inactive on task repetitions. An alternative approach assumes that TSCs reflect interference between the previously relevant and the currently required control settings, which are concurrently active on task switch trials ( Allport et al., 1994 ; Gilbert and Shallice, 2002 ; Waszak et al., 2003 ). TSCs arise because the interference is weaker on task repetition than on task switch trials. Finally, a hybrid, reconfiguration-interference account has also been proposed, postulating that TSCs reflect a mixture of reconfiguration and interference processes ( Meiran, 1996 , 2000 , but see Meiran et al., 2008 ). Critically, irrespectively of what mechanisms produce TSCs, all accounts assume that performance of a task is controlled by a set of representations that, following a task switch, are no longer appropriate. Thus, to meet the changed environmental demands, the control representations would have to change, too. Given this, the present study was designed to address the question of what representations change across task switches.
Conceptually, tasks can differ in all or some of the following respects: (i) criteria for spatial-attentional selection of the task-relevant stimulus; (ii) criteria for the identification of task-relevant stimulus properties; and (iii) task-appropriate stimulus-response (S-R) mappings. The set of cognitive representations specifying these criteria is considered collectively to constitute the task set. The available literature supports the notion of composite task set representations. For instance, Meiran (2000) demonstrated that while switching identification criteria can be performed in advance, actually performing the task is necessary for switching S-R mappings. Furthermore, Hübner et al. (2001) showed that the magnitude of TSCs increases with the number of task set components to be switched. Finally, a number of electrophysiological and imaging studies revealed neural correlates of a switch to co-vary with what task set component is being switched ( Rushworth et al., 2002 , 2005 ; Ravizza and Carter, 2008 ; Chiu and Yantis, 2009 ; Esterman et al., 2009 ; Hakun and Ravizza, 2012 ). In summary, the available evidence converges on the view that task sets consist of several dissociable representations controlling different cognitive processes in the stimulus-response chain. However, it remains unclear whether, on task switch trials, different components are changed relatively independently of each other, as would be predicted by the notion of “agglomerated task sets.”
Studies investigating whether or not it is possible to change only those task set components that require a change and to reuse shared components across different tasks yielded somewhat inconsistent findings. For example, Arrington et al. (2003) asked their participants to report, on different trials, either the stimulus height, width, color, or luminance. Smaller TSCs were found for switches across similar tasks (e.g., switching from color to luminance discrimination) relative to switches across dissimilar tasks (e.g., from height to luminance discrimination), suggesting that some reusing of control representations across different tasks is possible. By contrast, Vandierendonck et al. (2008) had their participants discriminate either the parity (odd/even) or the magnitude (greater/smaller than four) of stimuli consisting of several identical digits (e.g., five instances of the digit three). On different trials, either the number of digits or the digits themselves were task-relevant. The critical comparison was between trials on which both the identification criterion (parity vs. magnitude) and the stimulus attribute (number vs. digit) switched, and trials on which only one criterion switched (e.g., from digit parity to magnitude discrimination). Although partial switches in principle allowed for old control representations to be reused, no difference was observed between full and partial switches—indicating that, following a task switch, all task-set components are reset. Finally, in a study very similar to Kleinsorge (2004) ; Vandierendonck et al. (2008) (see also Kleinsorge and Heuer, 1999 ) observed partial repetition costs , that is, partial switches took actually more time to be implemented than full switches. Kleinsorge explained these findings by assuming a hierarchical organization of task sets, according to which having to change task set components situated earlier in the stimulus-response chain (e.g., identification criteria) would trigger a switch in all subsequent criteria (e.g., S-R mapping rules). In summary, the available literature suggests that following a task-switch, task-sets are sometime reset, sometimes switched, and sometimes reused. It should be borne in mind, however, that the various studies used (i) different paradigms and (ii) different types of switches. These differences will be discussed in more detail in the General Discussion.
Resetting, Switching or Reusing Task-Set Components
Depending on what tasks precisely vary across trials, task switching could necessitate changes of either all components (full switches) or only those components in which the two tasks differ (partial switches). To illustrate, consider a paradigm in which stimulus displays consist of many identical items with one of them (the singleton target) being different in either color or orientation from the rest. Participants are to, on different trials, either simply detect the presence vs. absence of the singleton target or discriminate its exact features, with a cue, presented prior to the display onset, announcing what task (detection or discrimination) is to be performed. Independently of the task sequence, the task-relevant dimension can also repeat or change. The dimension in which the singleton target is defined, although not informative about the response to be performed (i.e., knowing the dimension would not specify the exact response!), would be informative for both spatial-attentional selection and post-selective identification processes.
Concerning target selection, the available evidence suggests that when the dimension repeats across trials, spatial selection is speeded relative to dimension changes, as elaborated in the Dimension-Weighting Account of Müller and colleagues (e.g., Found and Müller, 1996 ; Müller and Krummenacher, 2006 ; Müller, 2010 ). Since both singleton detection and singleton discrimination tasks would require spatial selection, spatial selection processes for both tasks would be sensitive to the singleton dimension. Accordingly, dimension repetition effects (DREs) would be expected across (task repetition and switch) trials of both detection and discrimination tasks.
In contrast to the shared spatial selection, post-selective identification processes should differ between the two tasks. On the one hand, fast and accurate singleton detection can be achieved by simply determining the presence/absence of a singleton, while information about what the singleton features precisely are is not strictly necessary for performing the task. Consistent with this, there is evidence that the response-irrelevant singleton features are not processed up to the level at which they become available for explicit report ( Müller et al., 2004 ). On the other hand, encoding singleton features from a particular dimension is critical for the singleton discrimination task. With these differential task requirements in mind, it is likely that post-selective identification processes differ between tasks: the singleton dimension is important for identification in the discrimination, but not in the detection task (see Töllner et al., 2012b , for supporting EEG data).
Differences between detection and discrimination tasks would determine what can be and is reused across trials. Following performance of a target-present detection task, the dimension should have been encoded and thus be available for reuse only for spatial selection. Following a discrimination task, the dimension should have been encoded in and thus be available for reuse for both the spatial selection and post-selective identification processes. Thus, the task on trial n − 1, or prime trial, determines what is available for reusing. By contrast, the task on trial n , or probe trial, determines what is reused: in the detection task, reusing dimension information would facilitate just spatial selection, while in discrimination task reusing dimension information would facilitate both selection and identification processes. Consequently, on the hypothesis of agglomerated task sets, comparable DREs would be expected for detection → detection and detection → discrimination sequences, because across these sequences, only spatial selection criteria are available and reused. By contrast, following performance of the discrimination task, both spatial selection and identification criteria would be available for reuse, but they would be reused only on the current discrimination trial, predicting stronger DREs for discrimination → discrimination relative to discrimination → detection sequences.
In contrast to the notion of agglomerated task sets, that of integrated task sets would predict that following any switch, the task set would be reset; accordingly, there should not be a difference between full and partial switches. Finally, the notion of hierarchical task sets would predict a reversal of DREs, that is: switching from, e.g., detection to discrimination, would also switch the expected dimension, resulting in worse performance following dimension repetitions relative to dimension switches.
ERP Components Sensitive to the Spatial Selection and Post-Selective Identification Components
In the paradigm described above, preparatory adjustments with regard to the task-relevant (singleton) dimension are not possible since the task cue is not dimension-specific 1 . Consequently, the analyses of partial-switch effects for spatial selection and post-selective identification in the EEG domain have focused on areas and ERP components sensitive to the implementation of these processes.
As an index of spatial-attentional selection, parameters of the Posterior-Contralateral-Negativity (PCN, or N2-posterior-contralateral 2 ) have been analyzed. This component manifests as an increased negativity at posterior scalp electrode sites contralateral to the singleton position, relative to ipsilateral electrode sites, in the 175–300-ms time range post-stimulus. PCN parameters are considered to reflect the dynamics of spatial selection processes ( Luck and Hillyard, 1994 ; Eimer, 1996 ; Töllner et al., 2011 ). Importantly, PCN latencies are shorter for dimension repetitions relative to changes ( Töllner et al., 2008 ). On the assumption of agglomerated task sets, substantial DREs are expected for both task repetitions and switches. By contrast, the assumption of integrated tasks sets would predict no DRE across task switches.
The second component of interest was the Sustained Posterior Contralateral Negativity (SPCN), manifesting as an increased negativity over posterior electrodes contralateral to the target, relative to ipsilateral electrodes, starting from 350–400-ms post-stimulus. The SPCN component is considered to be sensitive to the processing demands following stimulus selection ( Jolicoeur et al., 2008 ). Importantly, the SPCN is weaker ( Mazza et al., 2007 ; Töllner et al., 2013 ) in tasks that do not necessarily require perceptual analysis following stimulus selection (e.g., singleton detection task), and more prominent (ibid.) in tasks that require stimulus analysis up to the feature levels (e.g., in singleton discrimination task). Thus, the available evidence predicts a stronger SPCN for the discrimination task, which requires explicit feature discrimination, relative to the detection task, in which post-selective processing is not necessary for an accurate response. Furthermore, the hypothesis of agglomerated task sets predicts stronger DREs in the SPCN time range on trials preceded by (a trial with) the discrimination task, in which post-selective identification should be sensitive to the dimensional identity of the singleton, relative to trials preceded by the detection task, for which it is sufficient to determine that the selected item is a singleton, without necessarily identifying its precise dimension or feature properties.
Participants
Sixteen males (mean age 29 years, 2 left-handed), all with normal color vision and normal or corrected-to-normal visual acuity, participated in the experiment for monetary compensation. All participants had extensive experience with psychophysical tasks and all were naïve as to the purpose of the experiment. Due to excessive eye blinking, two participants were excluded from the analyses.
Stimuli were presented on a 17″ CRT monitor with a 1024 × 768 pixels resolution and an 85-Hz refresh rate. Custom written C ++ code controlled stimulus presentation and recorded responses. The experiment was conducted in a dimly lit, acoustically and electromagnetically shielded room. Head-to-monitor distance was 60 cm.
Stimuli and Procedure
Stimulus displays (Figure 1 ) were presented on a gray background (19 cd/m 2 , CIE x = 0.292, y = 0.307) and consisted of 38 vertical yellow (0.388, 0.520) bars arranged around three concentric—inner, middle, and outer—circles with 8, 12, and 18 items, respectively. Single bars subtended 0.4 × 1.9° of visual angle, and the diameters of the three circles were 5, 10, and 15°, respectively. On target-present trials, one of the bars on the middle circle (excluding the two positions along the vertical meridian) was replaced by either a blue (0.275, 0.541) or a green (0.211, 0.263) color singleton target or a right-titled (12° clockwise from vertical) or left-tilted (12° counter-clockwise) orientation singleton target, matched in luminance to the distractor bars (68 cd/m 2 ).
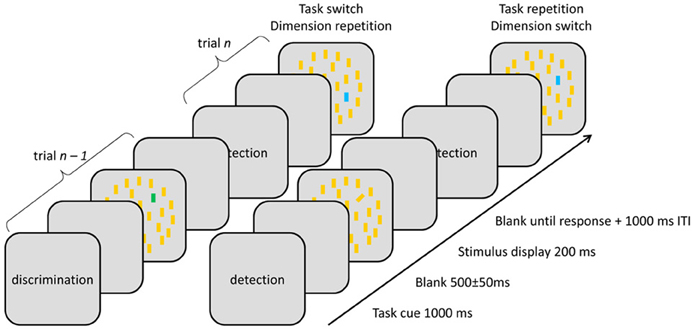
Figure 1. Illustration of stimulus displays across different inter-trial sequences .
Every trial started with a task cue (i.e., the word “detection” or “discrimination”) shown for 1000 ms, followed by a 500 ± 50-ms blank screen. Next, the stimulus display appeared for 200 ms, followed by a blank screen until response. In case of response errors, the standard intertrial interval (1000 ms) was doubled. Responses were given via pressing the left and right mouse keys using the left- and right-hand thumbs, respectively. Stimulus displays were identical for both tasks, the difference being that, in the detection task, participants were required to discern the presence (on 60% of detection task trials) vs. the absence of a singleton target by pressing the corresponding response key with two possible S-R mappings: (i) target-present → R 1 , -absent → R 2 and, respectively, (ii) target-absent → R 1 , -present → R 2 . In the discrimination task, a singleton was always present, with participants having to report the feature that distinguished it from distractors (blue, green, left-, and right tilted). Same-dimension singletons (e.g., blue and green) required different responses (e.g., R 1 and R 2 ), while singletons from different dimensions (e.g., blue and left-tilted) were mapped to a same response, resulting in four possible S-R mappings: (i) blue or left-tilted → R 1 , green or right-tilted → R 2 , (ii) green or left-tilted → R 1 , blue or right-tilted → R 2 , (iii) blue or right-tilted → R 1 , green or left-tilted → R 2 , and (iv) green or right-tilted → R 1 , blue or left-tilted → R 2 . The two possible S-R mappings in the detection task and the four S-R mappings in the discrimination task yielded eight different S-R mapping combinations, which were counterbalanced across participants.
The task (detection vs. discrimination) and the target's dimension (color vs. orientation) were randomly selected on every trial, resulting in four task sequences (detection on both prime and probe trials; discrimination on both trials; detection on prime, discrimination on probe trial; and discrimination on prime, detection on probe trial) and two dimension sequences (repetition/change) across consecutive trials. Relevant dimensions were sampled with equal probability, however, in order to ensure comparable numbers of target-present trials across the two tasks, the detection task was made more frequent (3:2 ratio).
Prior to experiment proper, participants received 1–4 practice blocks (80 trials per block); practice was terminated once a participant achieved ≤ 10% errors per block. All participants met the criterion after 2–4 blocks. Following practice, participants completed 1920 trials (in ca. 3 h), split in two equal-length sessions with a 15–30 min break in between.
EEG Recording and Analyses
The EEG was sampled at 1 KHz using Ag/AgCl active electrodes (actiCAP system; Brain Products, Munich) from 64 scalp sites, arranged according to the 10–10 System (American Electroencephalographic Society, 1994), and amplified using BrainAmp amplifiers (BrainProducts, Munich) with a 0.1–250-Hz bandpass filter. Impedances were kept below 5 kΩ. Electrodes were online referenced to FCz and re-referenced offline to averaged mastoids. Electrodes placed at the outer canthi of the eyes and the superior and inferior orbits monitored blinks and eye movements. Non-stereotyped noise was removed by visual inspection, followed by high-pass filtering using a Butterworth infinite impulse response filter at 0.5 Hz (24 dB/Oct). An infomax independent-component analysis was run to identify and remove effects of eye movements and blinks. Next, continuous EEG was epoched into −200–600 ms segments time-locked to stimulus display onset. Baseline correction was performed based on the −200–0 ms pre-stimulus interval. Target-absent trials, trials preceded by a target-absent trial, error response trials, as well as trials preceded by an error were excluded from the analyses. Finally, trials with (i) signals exceeding ±60 μV, (ii) bursts of electromyographic activity (permitted maximal voltage steps/sampling point of 50 μV), or (iii) activity lower than 0.5 μV within intervals of 500 ms (indicating dead channels) were removed from further analyses on an individual channel basis. The remaining trials (mean = 111 trials per participant per condition, SD = 5 trials) were sorted according to experimental conditions, and averaged on an individual-channel basis.
Data Analyses
Analysis of EEG signals focused on two event-related potentials (ERPs). The ERP components were quantified by subtracting ERPs measured at lateral parieto-occipital electrodes (PO7/PO8) ipsilateral to the target's location from contralateral ERPs. The PCN peak latencies and amplitudes were defined per participant as the maximum negative-going deflection in the time period 170–270 ms post-stimulus. SPCN amplitudes were defined as the average of the time window the 430–510-ms post-stimulus.
Mean RTs, error rates, PCN peak latencies and amplitudes, and SPCN mean amplitudes were computed for correct target-present probes for which the primes were also correct target-present trials. Dependent measures were submitted to repeated-measures ANOVAs (RANOVAs) in three different analyses. First, overall task differences and TSCs were assessed in a RANOVA with main terms for (i) task on probe trial (detection vs. discrimination) and (ii) task sequence (repetition vs. change) across prime and probe trials. The second analysis focused on indices of re-using processes across trials, that is, on dimension-repetition effects. Because re-use is expected to co-vary with what is available for re-using, which is determined on prime trials, the second set of analyses used a RANOVA with main terms for (i) task on prime trial, (ii) task on probe trial, and (iii) dimension sequence (repetition vs. change) across trials. Third, effects of response sequence on mean RTs and error rates were analyzed. Because only a small number of trials were available per cell for this analysis, the corresponding ERPs could not be examined.
Analyses of Overall Task Differences
Behavioral results.
Inspection of the overall mean RTs and error rates (shown in Table 1 ) revealed performance to be faster and more accurate for the detection task (mean RTs = 594 ms, mean error rate = 5.2%) than for the discrimination task (670 ms, 6.5%). Furthermore, task repetitions (598 ms, 4.5%) yielded faster and more accurate performance relative to task changes (666 ms, 7.1%), indicating substantial TSCs for both RTs and error rates (TSC RT = 68 ms, TSC errors = 2.6%). Finally, switching from discrimination to detection incurred greater TSCs (81 ms, 3%) than switching from detection to discrimination (57 ms, 2.3%).
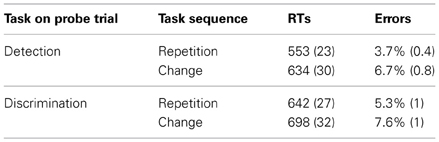
Table 1. Mean RTs (SE M ) and percentage of errors (SE M ) on probe trials for the two different tasks, dependent on the task sequence .
These observations were confirmed by RANOVAs of the mean RTs and error rates with main terms for task on probe trial (detection vs. discrimination) and task sequence (task- repetition vs. -change). The analysis of the mean RTs proved main effects of task, F (1,13) = 36.44, p < 0.01, η 2 p = 0.74, task sequence, F (1,13) = 18.65, p < 0.01, η 2 p = 0.60, as well as their interaction, F (1,13) = 6.18, p < 0.05, η 2 p = 0.32, to be significant. The RANOVA of the mean error rates yielded likewise a significant main effect of task sequence, F (1,13) = 20.94, p < 0.01, η 2 p = 0.62, without, however, any of the other effects reaching significance (all F < 2.17, all p > 0.16).
ERP results
The stimulus-locked ERP waves obtained for the detection and discrimination tasks are shown on Figure 2 . Figure 2A depicts the time course separately for electrodes contra- and ipsilateral to the target position, averaged across target positions 3 (left vs. right), while Figure 2B depicts the difference between contra- and ipsilateral electrodes. As can be seen from Figure 2B , substantial lateralization effects, confirmed by t -tests against zero, were observed in the PCN time range (170–270 ms) for both the detection (−2.17 μV, p < 0.01) and the discrimination task (−2.41, p < 0.01). Similar to what we already reported in Töllner et al. (2012a) , PCN amplitude was higher for the discrimination than for the detection task, with comparable PCN latencies across the two tasks (221 and 222 ms for detection and discrimination, respectively). The RANOVA of the PCN latencies with main terms for (i) task on probe trial and (ii) task sequence yielded no significant effects (all F < 1). The RANOVA of the PCN amplitudes yielded a significant main effect of task, F (1,13) = 6.61, p < 0.05, η 2 p = 0.43; the main effect of task sequence non-significant ( F < 1); the task × task sequence interaction approached significance, F (1,13) = 3.70, p = 0.08, η 2 p = 0.22), owing to the fact that switching task tended to increase the PCN amplitude for the detection task (−2.12 μV and −2.22 μV for detection → detection and discrimination → detection sequences, respectively), while tending to decrease the amplitude for the discrimination task (−2.52 and −2.29 μV for discrimination → discrimination and detection → discrimination sequences, respectively).
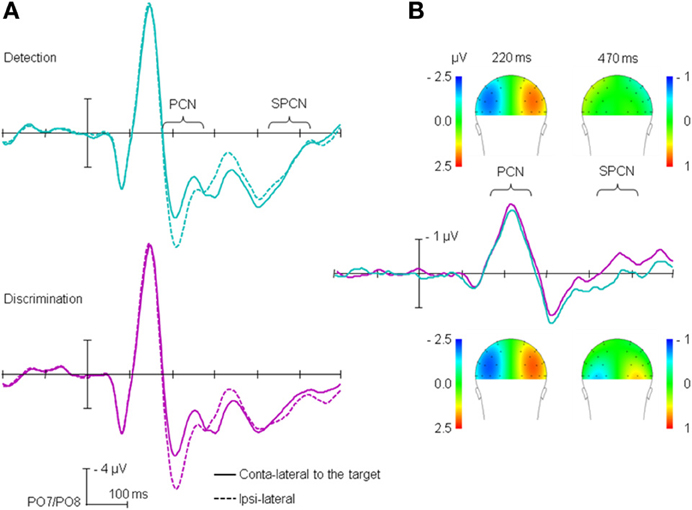
Figure 2. Stimulus-locked ERPs: (A) for the ipsi-and contralateral electrodes relative to the target position, separately for the detection and discrimination tasks; (B) difference between contra- and ipsilateral electrodes. For the purpose of the presentation, data are presented filtered with 30-Hz low-pass filter.
Furthermore, as Figure 2B shows, and as confirmed by t -tests against zero, lateralization effects in SPCN time range (430–510 ms) were observed for the discrimination task (mean amplitude = −0.36 μV, p < 0.01), but not for the detection task (0.14 μV, p = 0.18). A RANOVA of the SPCN mean amplitude with main terms for task and task sequence revealed the main effect of task to be significant, F (1,13) = 72.74, p < 0.01, η 2 p = 0.85, with no other effects reaching significance (all F < 1).
Analyses of Re-Using Control Settings Across Tasks
Figure 3 depicts the mean RT and error rate for a given task on the probe trial (detection, discrimination) dependent on the task on the prime trial (detection, discrimination), separately for dimension repetitions and changes (dimension sequence). As can be seen, RTs were faster for dimension repetitions (blue bars) than for dimension changes (red bars), in all conditions; error rates followed a similar pattern. These observations were confirmed by a RANOVA of the mean RTs, which revealed all three main effects (all F > 6.18, all p < 0.05), all two-way interactions (all F > 18.17, all p < 0.01), and the three-way interaction between task on prime trial, task on probe trial, and dimension sequence, F (1,13) = 21.27, p < 0.01, η 2 p = 0.62, to be significant. An analogous RANOVA of the error rates yielded the following significant effects: main effect of dimension sequence, F (1,13) = 11.39, p < 0.01, η 2 p = 0.47; task on probe trial × dimension sequence interaction, F (1,13) = 33.88, p < 0.05, η 2 p = 0.28; task on prime trial × task on probe trial interaction, F (1,13) = 20.94, p < 0.01, η 2 p = 0.62; and task on prime trial × task on probe trial × dimension sequence interaction, F (1,13) = 8.16, p < 0.5, η 2 p = 0.39.
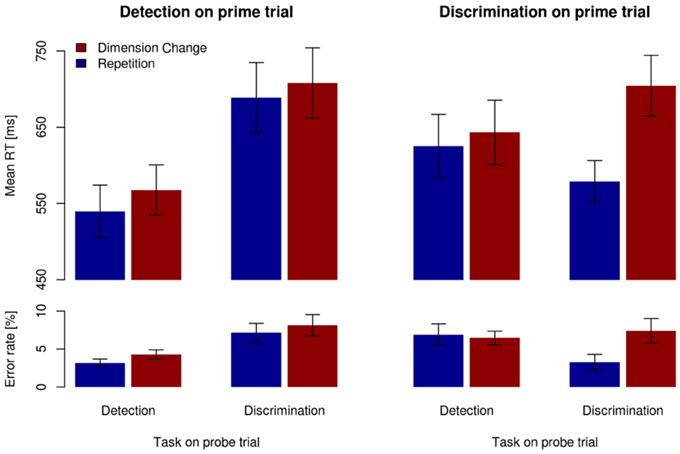
Figure 3. Mean RTs (upper panels) and error rates (lower panels) for the different task sequences, separately for dimension repetitions (blue bars) and changes (red bars). Vertical lines denote ±1SEM.
To further investigate the significant three-way interactions, separate RANOVAs were run dependent on the specific task on prime trials (detection and, respectively, discrimination), with main terms for task on probe trial and dimension sequence. With the detection task on prime trials (left-hand panels in Figure 3 ), the analysis of the (probe-trial) RTs revealed both main effects to be significant: task on probe trial [145-ms difference between discrimination and detection tasks, F (1,13) = 34.04, p < 0.01, η 2 p = 0.72] and dimension sequence [23-ms difference between dimension changes vs. -repetitions, F (1,13) = 22.00, p < 0.01, η 2 p = 0.63]; the interaction between the two was far from significance ( F < 1). The RANOVA of the error rates revealed a significant main effect of task on probe trials [3% difference between discrimination and detection, F (1,13) = 16.68, p < 0.01, η 2 p = 0.56], and a marginally significant main effect of dimension sequence, with dimension repetitions yielding 1.1% more accurate performance than dimension changes, F (1,13) = 4.35, p = 0.06; the interaction between the two was far from significance ( F < 1).
With the discrimination task on prime trials (right-hand panels in Figure 3 ), a RANOVA of the (probe-trial) RTs revealed the main effect of dimension sequence, F (1,13) = 36.32, p < 0.01, η 2 p = 0.81, and the task on probe trial × dimension sequence interaction, F (1,13) = 32.01, p < 0.01, η 2 p = 0.71, to be significant. The interaction was caused by the dimension repetition (vs. change) effect being much larger for discrimination → discrimination sequences (125 ms) than for discrimination → detection sequences (18 ms). For the errors (on probe trials), the main effect of dimension sequence proved significant, F (1,13) = 7.67, p < 0.05, η 2 p = 0.37, with accuracy being 1.4% higher for dimension repetitions relative to changes. The task on probe trial × dimension sequence interaction was also significant, F (1,13) = 8.31, p < 0.5, η 2 p = 0.40, with a stronger DRE for the discrimination relative to the detection task on the probe trial (3.2 vs. −0.3%, respectively).
In summary, significant DREs were observed in all experimental conditions. When the task on the prime trial required simple target detection, DREs were comparable for detection and discrimination tasks on the probe trial. By contrast, with the task on prime trial required target discrimination, stronger DREs were observed for the discrimination task, relative to the detection, to be performed on the probe trial.
Lateralized ERPs are depicted in Figure 4 for the probe trials, separately for the different tasks on prime and on probe trials, as well as across different dimension sequences. As can be seen from Figure 4 , the PCN latency was delayed for dimension changes (red lines) relative to dimension repetitions (blue lines), in all conditions. In the SPCN time range DREs were evident only for (probe) trials following the discrimination task.
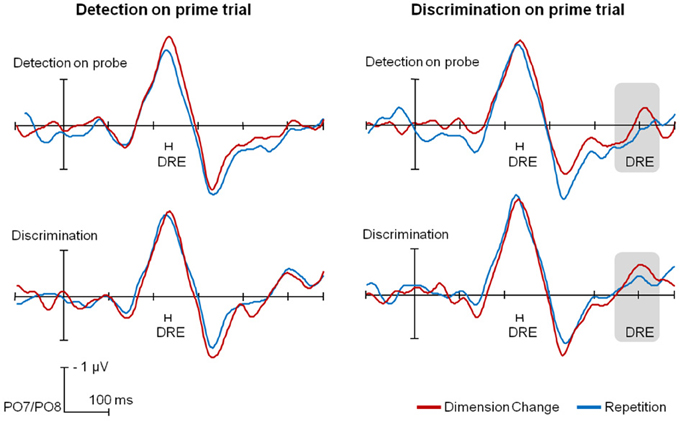
Figure 4. Group-averaged time course of PCN and SPCN components for the different task sequences, separately for dimension repetitions (blue) and changes (red). Significant dimension repetition effects for the peak PCN latency and the mean SPCN amplitude are indicated. For the purpose of presentation, a 30-Hz low-pass filter was applied; data analyses were performed over individual, unfiltered data.
PCN analyses
For (probe) trials preceded by the detection task (left-hand side of Figure 4 ), a RANOVA of the PCN peak latencies revealed only a significant main effect of dimension sequence, with dimension repetitions being 17 ms faster than dimension changes, F (1,13) = 15.41, p < 0.01, η 2 p = 0.54; no other effects reached significance (all F < 1). An analogous RANOVA for trials preceded by the discrimination task (right-hand side) also yielded only a significant main effect of dimension sequence [14-ms DRE, F (1,13) = 5.09, p < 0.05, η 2 p = 0.23], with no other effects reaching significance (all F < 1).
SPCN analyses
With the task on the prime trial requiring target detection, analysis of the (probe-trial) mean SPCN amplitudes revealed only a significant main effect of task on probe trial, with an overall stronger SPCN component for the discrimination relative to the detection task (−0.37 vs. 0.18 μV), F (1,13) = 25.70, p < 0.01, η 2 p = 0.66; no other effects reached significance (all F < 1.94, all p > 0.18). With the discrimination task on the prime trial, an analogous analysis also yielded a significant main effect of task on probe trial, F (1,13) = 30.16, p < 0.01, η 2 p = 0.70, with the significant SPCN for the discrimination relative to the insignificant SPCN for the detection task (−0.36 vs. 0.10 μ V). Importantly, the main effect of dimension sequence was also significant, F (1,13) = 5.33, p < 0.05, η 2 p = 0.29, with the SPCN amplitude being stronger for dimension changes relative to dimension repetitions (−0.23 vs. −0.02 μ V); the interaction task on probe trial x dimension sequence was far from significance ( F < 1).
In summary, analyses of the ERPs revealed longer PCN peak latencies for dimension changes vs. repetitions independently of the task on prime or probe trial; SPCN mean amplitudes were overall larger for the discrimination than for the detection task on probe trial, while significant DREs in the SPCN time interval were observed only for trials preceded by the discrimination task.
Analyses of Response-Sequence Effects
As can be seen from Table 2 , mean RTs were overall fastest for full repetitions (same task, dimension, and response), intermediate for partial repetitions, and slowest for full changes. By contrast, the errors varied as a function of task sequence. Importantly, though, there was no evidence that partial repetitions resulted in less accurate performance relative to full changes.
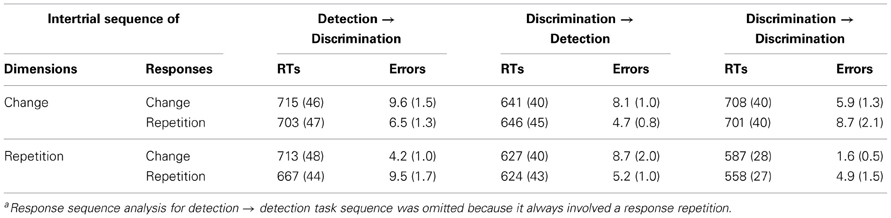
Table 2. Mean RTs (SE M ) and percentage of errors (SE M ) across different task-, dimension- and response a sequences .
These observations were confirmed by three-ways RANOVAs (task- × dimension- × response sequence) of the mean RTs and error rates with a focus on the main effect of and interactions involving response sequence. The RT analysis showed the main effect of response sequence to be significant, F (1,13) = 6.41, p < 0.05, η 2 p = 0.33, as well as the interaction of this factor with dimension sequence, F (1,13) = 9.04, p < 0.01, η 2 p = 0.41, owing to a larger response repetition benefit when the dimension repeated (30 ms, p < 0.01) rather than changed (5 ms, p = 0.49). The task sequence × response sequence interaction was marginally significant, F (2,26) = 1.67, p = 0.07, η 2 p = 0.18, suggestive of a larger response repetition benefit for the discrimination task (29 ms, p < 0.01, and 23 ms, p < 0.01, for detection → discrimination and discrimination → discrimination sequences, respectively) relative to the detection task (−1 ms, p = 0.93). The three-way interaction did not reach significance, F = 1.67, p = 0.27.
Concerning the error analysis, the main effect of response sequence was non-significant ( F < 1). However, response sequence interacted with dimension sequence, F (1,13) = 18.43, p < 0.01, η 2 p = 0.59, and task sequence, F (2,26) = 18.52, p < 0.01, η 2 p = 0.59; and the response- × dimension- × task sequence interaction was significant, F (2,26) = 8.55, p < 0.01, η 2 p = 0.40. Post-hoc analyses revealed the pattern of response sequence effects (repetition benefit vs. cost) to vary across task and dimension sequences. For discrimination → detection task sequences, dimension repetitions were associated with response repetition costs (−5.4%, p < 0.01), whereas dimension changes yielded response repetition benefits (4.1%, p < 0.01). By contrast, discrimination → detection task sequences resulted in response repetition benefits (3.5%, p < 0.01) independently of dimension sequence. Finally, discrimination → discrimination task sequences were associated with response repetition costs (−2.9%, p < 0.05) independently of dimension sequence.
In summary, analyses of response sequence effects on mean RTs showed either response repetition benefits or no effects of response sequence. This finding is at variance with hierarchical task sets, which predict response repetition costs following a task or dimension change. Integrated task sets, which predict no response sequence effects following a task or dimension switch, also account poorly for the present findings. The results for error rates were less consistent across task and dimension sequences, with different patterns of response sequence effects across different experimental conditions.
The present findings demonstrate that, consistent with “agglomerated task sets,” it is possible to reuse control settings across different tasks, and that the reusing is associated with distinct ERP components, depending on precisely which task set component (selection vs. identification criteria) is reused across tasks. In particular, switching tasks was revealed to be easier when the task-relevant dimension repeated relative to when it changed, as indexed by DREs on task switch trials. The notion of agglomerated task sets is fully compatible with existing computational models of cognitive control ( Logan and Gordon, 2001 ; Meiran et al., 2008 ). Both these accounts postulate several independent parameters influencing processes of selection, identification, and, respectively, responding. Importantly, since these parameters are independent, it is plausible that they can also be adjusted independently—which is the core assumption of the agglomerated-task-sets hypothesis. The compatibility with the computational models of cognitive control emphasizes another property of agglomerated task sets: their computational efficiency.
Note though that the DREs reported presently are consistent not only with agglomerated task sets, but also with two, relatively strong alternatives, namely: (i) switching between the detection and discrimination conditions did not involve a task switch, and (ii) the DREs are not dimension-specific, but rather feature- or response-specific. The former alternative would postulate that the two tasks used in the present study were effectively one task, in which participants always performed feature discrimination, but, depending on the cue, selected different responses. The latter alternative would imply that the substantial reusing of spatial selection and identification criteria (as revealed in the dimension-repetition effects) was not indicative of the reuse of task set components in general, but rather of the reuse of stimulus-response associations encountered on the previous trial ( Hommel et al., 2001 ; Dreisbach et al., 2006 , 2007 ).
The present findings argue against the hypothesis that no task switching took place in our paradigm. First, in the present study, substantial differences in mean RTs and errors rates were observed between the detection and discrimination tasks, suggesting that the two conditions were performed differently. Behavioral differences were accompanied by differences in the ERPs: a reliable SPCN component was observed for the discrimination, but not for the detection task. Taken together, these findings suggest that solving the detection and, respectively, discrimination tasks involved the use of different task sets. Second, switching between the two conditions incurred substantial switch costs. Importantly, the switch cost magnitude differed, with stronger costs for discrimination → detection relative to detection → discrimination sequences. The asymmetry in the switch costs—with switching to an easier, or dominant, task (presently, detection) being more effortful than switching to a more difficult task (presently, discrimination)—has frequently been reported in task-switching literature ( Allport et al., 1994 ; Wylie and Allport, 2000 ) and interpreted as an index of the interference between two concurrent task sets. On this background, it is likely that switching between the two tasks in the present study indeed involved task switch processes.
The second alternative explanation posits that DREs critically rely on repetitions of full stimulus-response episodes across trials, rather than on more abstract criterion repetitions. Studies investigating the role of S-R repetitions have typically found performance to be very good on full-repetition trials and worse on partial-repetition trials, that is, when either the stimulus or the response changed, while the other property repeated. Somewhat counter-intuitively, performance is typically better on full switches, that is, when both stimulus and response change, compared to partial repetitions ( Hommel, 1998 , 2005 ; Töllner et al., 2008 ; Zehetleitner et al., 2012 ). Applied to the present study, if DREs were actually S-R repetition effects, then changing the dimension and repeating the response (i.e., partial repetitions) should have resulted in worse performance relative to changing both the dimension and the response (full repetitions). However, analyses of response sequence effects showed this not to be the case, arguing that the DREs reported presently are not reducible to S-R repetition effects.
That partial-repetition costs were not prominent in the present study does not necessarily imply that the processes generating these effects were inactive; rather, the paradigm and dependent measures may not have been sensitive to these processes. Previous work investigating electrophysiological correlates of DREs in a single task paradigm ( Töllner et al., 2008 ) revealed partial repetition costs to correlate with ERP markers that were independent of the ERP markers for dimension and, respectively, response sequence effects. Importantly, the markers of partial-repetition costs were not investigated in the present study. Additionally, in a recent study of partial-repetition costs ( Zehetleitner et al., 2012 ), numerical simulations showed that three different sequence-sensitive mechanisms (dimension-, S-R mapping-, and motor-response-specific) combine and can produce any possible RT pattern, that is, with or without manifest partial repetition costs. Given this, until the boundary conditions for partial-repetition costs to arise are fully understood, it remains possible that the present paradigm simply did not meet these conditions.
Direction of Partial-Switch Effects: Reusing, Resetting, or Switching
While the present study, together with several previous investigations ( Arrington et al., 2003 ; Rangelov et al., 2011 , 2012 ), showed that reusing shared task set components across different tasks is possible, findings to the contrary have also been reported. In particular, Vandierendonck et al. (2008) failed to find any partial-switch effects, while Kleinsorge and colleagues (1999 , 2004 ) found partial-switch costs , relative to full switches, rather than benefits as reported presently. How can these disparate findings be reconciled?
A notable difference between the paradigms used in these investigations and the present study is in the stimulus material and the cueing procedure employed. More precisely, the previous studies used stimuli that were ambiguous with regard to both task-relevant stimulus attributes and identification criteria. Thus, cueing of both the relevant stimulus property (e.g., number or digit value in Vandierendonck et al., 2008 ) and identification criteria (parity vs. magnitude) was necessary on every trial (e.g., the string “number odd/even” served as a cue). This procedure produced partial overlap between cue strings on partial switches (e.g., “number odd/even” → “digit odd/even”) and no overlap on full switches (e.g., “number smaller/greater” → “digit odd/even”). As has been shown by several studies ( Logan and Schneider, 2006 ; Schneider and Logan, 2009 ), overlapping cues activate competing task sets, resulting in negative interference on partial-switch trials. As a consequence, any benefits from reusing shared control representations might have been masked by interference effects from overlapping cues, resulting in either insignificant partial-repetition effects or even partial-repetition costs. By contrast, the present study used cues that did not overlap between the different tasks. Furthermore, the task-relevant dimension was unambiguously specified by the stimulus displays themselves, so no dimension cues were necessary. Consequently, in the present experiment, the negative interference effects would have been minimal—and, correspondingly, partial-repetition effects turned out significant.
Electrophysiological Evidence for Separable Control Mechanisms
In contrast to the mixed behavioral findings regarding the direction of partial-switch effects, investigation of the ERP components related to the spatial selection and post-selective processing task components offers more conclusive findings. By assuming relative autonomy of the control systems for the different cognitive processes, “agglomerated task sets” predict the existence of multiple , relatively independent sources of DREs. Consistent with the prediction, EEG analyses revealed multiple ERP components to be sensitive to dimension sequences: dimension changes (relative to repetitions) resulted in longer PCN latencies as well as larger SPCN amplitudes.
Analyses of the PCN latencies showed dimension changes (relative to repetitions) to be associated with longer latencies independently of the task sequence (see also Töllner et al., 2008 , reporting similar findings in a single-task paradigm). This finding can be explained by assuming that both the detection and discrimination tasks required spatial selection processes, governed by a task set component sensitive to dimension sequences. Dimension repetitions would permit reusing control settings from the previous trial, generating DREs for the PCN latency. Importantly, on the agglomerated-task-set hypothesis, reusing settings is possible even across tasks that differ in other task set components—as evidenced by the present finding of DREs across task switches for the PCN timing.
Analyses of the SPCN amplitudes showed, consistent with our predictions, a more pronounced SPCN for discrimination relative to detection task trials. Apparently, the SPCN amplitude increases continuously with increases in demands for post-selective perceptual processing, from no SPCNs in singleton detection (present study) and singleton localization tasks ( Mazza et al., 2007 ) through singleton discrimination tasks (present study) to strong SPCNs in compound-search tasks ( Mazza et al., 2007 ; Töllner et al., 2013 ), in which one target dimension (e.g., color) is selection-relevant, whereas another dimension (e.g., orientation) is response-relevant.
Furthermore, the SPCN amplitude was sensitive to dimension sequence, but only on trials following the discrimination task. This finding is consistent with the conceptual analysis of the task sets for the detection and, respectively, the discrimination task and the functional interpretation of the SPCN component. Conceptual analysis of the detection task suggested that the singleton dimension is not mandatorily encoded in the post-selective identification settings (for the detection task) and it would not be available for reuse on the following trial, predicting no DRE for the SPCN component following detection task trials. By contrast, post-selective identification in the discrimination task necessarily involved determining the singleton dimension, predicting DREs for the SPCN component following trials of the discrimination task, consistent with the results of the present study.
The electrophysiological data are especially informative about the notion of a generalized switching mechanisms [as proposed by Kleinsorge and Heuer (1999) ], according to which one criterion switch enforces a switch in all criteria, rather than simple re-setting. If generalized switching were operating as a default task switch mechanism, then reconfiguring the spatial selection component should have triggered a switch in the post-selective identification component as well. This would predict an obligatory coupling between DREs for the PCN and SPCN parameters—a prediction that is not supported by the present findings. Thus, in summary, the present ERP findings are fully consistent with, and yet independent of the behavioral findings of DREs across task switches, in their support for the notion of agglomerated task sets.
Conclusions
The present study investigated the nature of task-set representations, defined as a set of criteria for spatial selection, post-selective identification, and S-R mapping rules. Two alternatives were considered: task sets as integral representations and task sets as agglomerations of relatively autonomous control settings guiding different processing stages. The key property of the “agglomerated-task-set” hypothesis is that following a task switch, different control instances can be reconfigured independently of each other, permitting reusing some of the control settings across different tasks. By contrast, the hypothesis of “integrated task sets” predicted no partial-switch effects, as changing any task set component would either reset all settings, or trigger a full switch. Consistent with agglomerated task sets, our findings demonstrate substantial partial-switch effects, operationalized as DREs. Most importantly, evidence of DREs over PCN latencies and SPCN amplitudes, two functionally distinct ERP components, further supports the idea of task sets as a collection of autonomous control instances governing processes of spatial selection and perceptual/symbolic analysis, respectively.
Conflict of Interest Statement
The authors declare that the research was conducted in the absence of any commercial or financial relationships that could be construed as a potential conflict of interest.
Acknowledgments
This research was supported by DFG grants RA 2191/1-1 (to Dragan Rangelov) and ZE 887/3-1 (to Michael Zehetleitner and Hermann J. Müller) as well as the German-Israeli Foundation for Scientific Research and Development grant 2011/158 (to Michael Zehetleitner and Hermann J. Müller).
1. ^ Note that most of the previous EEG studies on task switching used paradigms in which preparatory adjustments of the task set component of interest were possible, to some extent. This work typically revealed involvement of pre-frontal areas when preparing for a task switch ( Karayanidis et al., 2003 ; Nicholson et al., 2005 ; Karayanidis et al., 2009 ).
2. ^ As shown by Shedden and Nordgaard (2001 ; Töllner et al., 2012a ), the PCN is independent of both the amplitude and latency of the non-lateralized N2. Thus, to avoid potential confusion associated with the term “N2pc,” we prefer the neutral term “PCN.”
3. ^ Analyses of PCN and SPCN amplitudes revealed a significant target position effect for the PCN component (3.50 and 3.25 μV for left and right targets, respectively, F (1,13) = 4.81, p < 0.05, η 2 p = 0.27), and no difference between left and right targets in the SPCN component ( F = 1.62, p = 0.22). Most importantly, target position did not interact significantly with electrode site (ipsi- vs. contralateral), nor with any other experimental manipulation (all F s < 2.16, all p > 0.16)—indicating that the PCN and SPCN components were not specific for one visual hemifield.
Allport, D. A., Styles, E. A., and Hsieh, S. (1994). “Shifting intentional set: exploring the dynamic control of tasks,” in Attention and Performance , Vol. XV, eds C. Umilta and M. Moscovitch (Hillsdale, NJ: Erlbaum), 421–452.
Arrington, C. M., Altmann, E. M., and Carr, T. H. (2003). Tasks of a feather flock together: similarity effects in task switching. Mem. Cogn . 31, 781–789. doi: 10.3758/BF03196116
Pubmed Abstract | Pubmed Full Text | CrossRef Full Text
Chiu, Y.-C., and Yantis, S. (2009). A domain-independent source of cognitive control for task sets: shifting spatial attention and switching categorization rules. J. Neurosci . 29, 3930–3938. doi: 10.1523/JNEUROSCI.5737-08.2009
Dreisbach, G., Goschke, T., and Haider, H. (2006). Implicit task sets in task switching. J. Exp. Psychol. Learn. Mem. Cogn . 32, 1221–1233. doi: 10.1037/0278-7393.32.6.1221
Dreisbach, G., Goschke, T., and Haider, H. (2007). The role of task rules and stimulusresponse mappings in the task switching paradigm. Psychol. Res. Psychol. Forsch . 71, 383–392. doi: 10.1007/s00426-005-0041-3
Eimer, M. (1996). The N2pc component as an indicator of attentional selectivity. Electroencephalogr. Clin. Neurophysiol . 99, 225–234. doi: 10.1016/0013-4694(96)95711-9
Esterman, M., Chiu, Y.-C., Tamber-Rosenau, B. J., and Yantis, S. (2009). Decoding cognitive control in human parietal cortex. Proc. Natl. Acad. Sci. U.S.A . 106, 17974–17979. doi: 10.1073/pnas.0903593106
Found, A., and Müller, H. J. (1996). Searching for unknown feature targets on more than one dimension: investigating a “dimension-weighting” account. Percept. Psychophys . 58, 88–101. doi: 10.3758/BF03205479
Gilbert, S. J., and Shallice, T. (2002). Task switching: a PDP model. Cognit. Psychol . 44, 297–337. doi: 10.1006/cogp.2001.0770
Hakun, J. G., and Ravizza, S. M. (2012). Cognitive control: preparation of task switching components. Brain Res . 1451, 53–64. doi: 10.1016/j.brainres.2012.02.046
Hommel, B. (1998). Event files: evidence for automatic integration of stimulus-response episodes. Vis. Cogn . 5, 183. doi: 10.1080/713756773
CrossRef Full Text
Hommel, B. (2005). How much attention does an event file need. J. Exp. Psychol. Hum. Percept. Perform . 31, 1067–1082. doi: 10.1037/0096-1523.31.5.1067
Hommel, B., Müsseler, J., Aschersleben, G., and Prinz, W. (2001). The theory of event coding (TEC): a framework for perception and action planning. Behav. Brain Sci . 24, 849–878. doi: 10.1017/S0140525X01000103
Hübner, R., Futterer, T., and Steinhauser, M. (2001). On attentional control as a source of residual shift costs: evidence from two-component task shifts. J. Exp. Psychol. Learn. Mem. Cogn . 27, 640–653. doi: 10.1037/0278-7393.27.3.640
Jolicoeur, P., Brisson, B., and Robitaille, N. (2008). Dissociation of the N2pc and sustained posterior contralateral negativity in a choice response task. Brain Res . 1215, 160–172. doi: 10.1016/j.brainres.2008.03.059
Karayanidis, F., Coltheart, M., Michie, P. T., and Murphy, K. (2003). Electrophysiological correlates of anticipatory and poststimulus components of task switching. Psychophysiology 40, 329–348. doi: 10.1111/1469-8986.00037
Karayanidis, F., Mansfield, E. L., Galloway, K. L., Smith, J. L., Provost, A., and Heathcote, A. (2009). Anticipatory reconfiguration elicited by fully and partially informative cues that validly predict a switch in task. Cogn. Affect. Behav. Neurosci . 9, 202–215. doi: 10.3758/CABN.9.2.202
Kleinsorge, T. (2004). Hierarchical switching with two types of judgment and two stimulus dimensions. Exp. Psychol . 51, 145–149. doi: 10.1027/1618-3169.51.2.145
Kleinsorge, T., and Heuer, H. (1999). Hierarchical switching in a multi-dimensional task space. Psychol. Res . 62, 300–312. doi: 10.1007/s004260050060
Logan, G. D., and Gordon, R. D. (2001). Executive control of visual attention in dual-task situations. Psychol. Rev . 108, 393–434. doi: 10.1037/0033-295X.108.2.393
Logan, G. D., and Schneider, D. W. (2006). Priming or executive control? Associative priming of cue encoding increases “switch costs” in the explicit task-cuing procedure. Mem. Cogn . 34, 1250–1259. doi: 10.3758/BF03193269
Luck, S. J., and Hillyard, S. A. (1994). Spatial filtering during visual search: evidence from human electrophysiology. J. Exp. Psychol. Hum. Percept. Perform . 20, 1000–1014. doi: 10.1037/0096-1523.20.5.1000
Mazza, V., Turatto, M., Umiltà, C., and Eimer, M. (2007). Attentional selection and identification of visual objects are reflected by distinct electrophysiological responses. Exp. Brain Res . 181, 531–536. doi: 10.1007/s00221-007-1002-4
Meiran, N. (1996). Reconfiguration of processing mode prior to task performance. J. Exp. Psychol . 22, 1423–1442. doi: 10.1037/0278-7393.22.6.1423
Meiran, N. (2000). Modeling cognitive control in task-switching. Psychol. Res . 63, 234–249. doi: 10.1007/s004269900004
Meiran, N., Kessler, Y., and Adi-Japha, E. (2008). Control by action representation and input selection (CARIS): a theoretical framework for task switching. Psychol. Res . 72, 473–500. doi: 10.1007/s00426-008-0136-8
Monsell, S., and Driver, J. (2000). Control of Cognitive Processes: Attention and Performance XVIII . Available online at: http://eprints.ucl.ac.uk/18943/ (Accessed February 2, 2011).
Monsell, S., Sumner, P., and Waters, H. (2003). Task-set reconfiguration with predictable and unpredictable task switches. Mem. Cogn . 31, 327–342. doi: 10.3758/BF03194391
Müller, H. J., and Krummenacher, J. (2006). Visual search and selective attention. Vis. Cogn . 14, 389–410. doi: 10.1080/13506280500527676
Müller, H. J., Krummenacher, J., and Heller, D. (2004). Dimension-specific intertrial facilitation in visual search for pop-out targets: evidence for a top-down modulable visual short-term memory effect. Vis. Cogn . 11, 577–602. doi: 10.1080/13506280344000419
Müller, H. J., Töllner, T., Zehetleitner, M., Geyer, T., Rangelov, D., and Krummenacher, J. (2010). Dimension-based attention modulates feed-forward visual processing: a reply to Theeuwes (2010). Acta Psychol . 135, 117–122. doi: 10.1016/j.actpsy.2010.05.004
Nicholson, R., Karayanidis, F., Poboka, D., Heathcote, A., and Michie, P. T. (2005). Electrophysiological correlates of anticipatory task-switching processes. Psychophysiology 42, 540–554. doi: 10.1111/j.1469-8986.2005.00350.x
Rangelov, D., Müller, H. J., and Zehetleitner, M. (2011). Independent dimension-weighting mechanisms for visual selection and stimulus identification. J. Exp. Psychol . 37, 1369–1382. doi: 10.1037/a0024265
Rangelov, D., Müller, H. J., and Zehetleitner, M. (2012). The multiple-weighting-systems hypothesis: theory and empirical support. Attent. Percept. Psychophys . 74, 540–552. doi: 10.3758/s13414-011-0251-0252
Ravizza, S. M., and Carter, C. S. (2008). Shifting set about task switching: behavioral and neural evidence for distinct forms of cognitive flexibility. Neuropsychologia 46, 2924–2935. doi: 10.1016/j.neuropsychologia.2008.06.006
Rogers, R. D., and Monsell, S. (1995). Costs of a predictable switch between simple cognitive tasks. J. Exp. Psychol . 124, 207–231. doi: 10.1037/0096-3445.124.2.207
Rushworth, M. F. S., Passingham, R. E., and Nobre, A. C. (2002). Components of switching intentional set. J. Cogn. Neurosci . 14, 1139–1150. doi: 10.1162/089892902760807159
Rushworth, M. F. S., Passingham, R. E., and Nobre, A. C. (2005). Components of attentional set-switching. Exp. Psychol . 52, 83–98. doi: 10.1027/1618-3169.52.2.83
Schneider, D. W., and Logan, G. D. (2009). Selecting a response in task switching: testing a model of compound cue retrieval. J. Exp. Psychol. Learn. Mem. Cogn . 35, 122–136. doi: 10.1037/a0013744
Shedden, J. M., and Nordgaard, C. L. (2001). ERP time course of perceptual and post-perceptual mechanisms of spatial selection. Brain Res. Cogn. Brain Res . 11, 59–75. doi: 10.1016/S0926-6410(00)00064-1
Töllner, T., Conci, M., Rusch, T., and Müller, H. J. (2013). Selective manipulation of target identification demands in visual search: the role of stimulus contrast in CDA activations. J. Vis . 13:23. doi: 10.1167/13.3.23
Töllner, T., Gramann, K., Müller, H. J., Kiss, M., and Eimer, M. (2008). Electrophysiological markers of visual dimension changes and response changes. J. Exp. Psychol . 34, 531–542. doi: 10.1037/0096-1523.34.3.531
Töllner, T., Müller, H. J., and Zehetleitner, M. (2012a). Top-down dimensional weight set determines the capture of visual attention: evidence from the PCN component. Cereb. Cortex 22, 1554–1563. doi: 10.1093/cercor/bhr231
Töllner, T., Rangelov, D., and Müller, H. J. (2012b). How the speed of motor-response decisions, but not focal-attentional selection, differs as a function of task set and target prevalence. Proc. Natl. Acad. Sci. U.S.A . 109, E1990–E1999. doi: 10.1073/pnas.1206382109
Töllner, T., Zehetleitner, M., Gramann, K., and Müller, H. J. (2011). Stimulus saliency modulates pre-attentive processing speed in human visual cortex. PLoS ONE 6:e16276. doi: 10.1371/journal.pone.0016276
Vandierendonck, A., Christiaens, E., and Liefooghe, B. (2008). On the representation of task information in task switching: evidence from task and dimension switching. Mem. Cogn . 36, 1248–1261. doi: 10.3758/MC.36.7.1248
Waszak, F., Hommel, B., and Allport, A. (2003). Task-switching and long-term priming: role of episodic stimulus-task bindings in task-shift costs. Cogn. Psychol . 46, 361–413. doi: 10.1016/S0010-0285(02)00520-0
Wylie, G. R., and Allport, A. (2000). Task switching and the measurement of “switch costs.” Psychol. Res . 63, 212–233. doi: 10.1007/s004269900003
Zehetleitner, M., Rangelov, D., and Müller, H. J. (2012). Partial repetition costs persist in nonsearch compound tasks: evidence for multiple-weighting-systems hypothesis. Attent. Percept. Psychophys . 74, 879–890. doi: 10.3758/s13414-012-0287-y
Keywords: task switching, task sets, attentional selection, perceptual processing, electroencephalography, executive control
Citation: Rangelov D, Töllner T, Müller HJ and Zehetleitner M (2013) What are task-sets: a single, integrated representation or a collection of multiple control representations? Front. Hum. Neurosci . 7 :524. doi: 10.3389/fnhum.2013.00524
Received: 29 April 2013; Accepted: 13 August 2013; Published online: 03 September 2013.
Reviewed by:
Copyright © 2013 Rangelov, Töllner, Müller and Zehetleitner. This is an open-access article distributed under the terms of the Creative Commons Attribution License (CC BY) . The use, distribution or reproduction in other forums is permitted, provided the original author(s) or licensor are credited and that the original publication in this journal is cited, in accordance with accepted academic practice. No use, distribution or reproduction is permitted which does not comply with these terms.
*Correspondence: Dragan Rangelov, Department Psychologie, Allgemeine und Experimentelle Psychologie I, Ludwig-Maximilians-Universität München, Leopoldstr. 13, DE-80802 München, Germany e-mail: [email protected]
This article is part of the Research Topic
Attention, predictions and expectations, and their violation: attentional control in the human brain
- Search Menu
- Browse content in Arts and Humanities
- Browse content in Archaeology
- Anglo-Saxon and Medieval Archaeology
- Archaeological Methodology and Techniques
- Archaeology by Region
- Archaeology of Religion
- Archaeology of Trade and Exchange
- Biblical Archaeology
- Contemporary and Public Archaeology
- Environmental Archaeology
- Historical Archaeology
- History and Theory of Archaeology
- Industrial Archaeology
- Landscape Archaeology
- Mortuary Archaeology
- Prehistoric Archaeology
- Underwater Archaeology
- Urban Archaeology
- Zooarchaeology
- Browse content in Architecture
- Architectural Structure and Design
- History of Architecture
- Residential and Domestic Buildings
- Theory of Architecture
- Browse content in Art
- Art Subjects and Themes
- History of Art
- Industrial and Commercial Art
- Theory of Art
- Biographical Studies
- Byzantine Studies
- Browse content in Classical Studies
- Classical Literature
- Classical Reception
- Classical History
- Classical Philosophy
- Classical Mythology
- Classical Art and Architecture
- Classical Oratory and Rhetoric
- Greek and Roman Archaeology
- Greek and Roman Epigraphy
- Greek and Roman Law
- Greek and Roman Papyrology
- Late Antiquity
- Religion in the Ancient World
- Digital Humanities
- Browse content in History
- Colonialism and Imperialism
- Diplomatic History
- Environmental History
- Genealogy, Heraldry, Names, and Honours
- Genocide and Ethnic Cleansing
- Historical Geography
- History by Period
- History of Agriculture
- History of Education
- History of Emotions
- History of Gender and Sexuality
- Industrial History
- Intellectual History
- International History
- Labour History
- Legal and Constitutional History
- Local and Family History
- Maritime History
- Military History
- National Liberation and Post-Colonialism
- Oral History
- Political History
- Public History
- Regional and National History
- Revolutions and Rebellions
- Slavery and Abolition of Slavery
- Social and Cultural History
- Theory, Methods, and Historiography
- Urban History
- World History
- Browse content in Language Teaching and Learning
- Language Learning (Specific Skills)
- Language Teaching Theory and Methods
- Browse content in Linguistics
- Applied Linguistics
- Cognitive Linguistics
- Computational Linguistics
- Forensic Linguistics
- Grammar, Syntax and Morphology
- Historical and Diachronic Linguistics
- History of English
- Language Variation
- Language Families
- Language Acquisition
- Language Evolution
- Language Reference
- Lexicography
- Linguistic Theories
- Linguistic Typology
- Linguistic Anthropology
- Phonetics and Phonology
- Psycholinguistics
- Sociolinguistics
- Translation and Interpretation
- Writing Systems
- Browse content in Literature
- Bibliography
- Children's Literature Studies
- Literary Studies (Modernism)
- Literary Studies (Asian)
- Literary Studies (European)
- Literary Studies (Eco-criticism)
- Literary Studies (Romanticism)
- Literary Studies (American)
- Literary Studies - World
- Literary Studies (1500 to 1800)
- Literary Studies (19th Century)
- Literary Studies (20th Century onwards)
- Literary Studies (African American Literature)
- Literary Studies (British and Irish)
- Literary Studies (Early and Medieval)
- Literary Studies (Fiction, Novelists, and Prose Writers)
- Literary Studies (Gender Studies)
- Literary Studies (Graphic Novels)
- Literary Studies (History of the Book)
- Literary Studies (Plays and Playwrights)
- Literary Studies (Poetry and Poets)
- Literary Studies (Postcolonial Literature)
- Literary Studies (Queer Studies)
- Literary Studies (Science Fiction)
- Literary Studies (Travel Literature)
- Literary Studies (War Literature)
- Literary Studies (Women's Writing)
- Literary Theory and Cultural Studies
- Mythology and Folklore
- Shakespeare Studies and Criticism
- Browse content in Media Studies
- Browse content in Music
- Applied Music
- Dance and Music
- Ethics in Music
- Ethnomusicology
- Gender and Sexuality in Music
- Medicine and Music
- Music Cultures
- Music and Culture
- Music and Religion
- Music and Media
- Music Education and Pedagogy
- Music Theory and Analysis
- Musical Scores, Lyrics, and Libretti
- Musical Structures, Styles, and Techniques
- Musicology and Music History
- Performance Practice and Studies
- Race and Ethnicity in Music
- Sound Studies
- Browse content in Performing Arts
- Browse content in Philosophy
- Aesthetics and Philosophy of Art
- Epistemology
- Feminist Philosophy
- History of Western Philosophy
- Metaphysics
- Moral Philosophy
- Non-Western Philosophy
- Philosophy of Action
- Philosophy of Law
- Philosophy of Religion
- Philosophy of Science
- Philosophy of Language
- Philosophy of Mind
- Philosophy of Perception
- Philosophy of Mathematics and Logic
- Practical Ethics
- Social and Political Philosophy
- Browse content in Religion
- Biblical Studies
- Christianity
- East Asian Religions
- History of Religion
- Judaism and Jewish Studies
- Qumran Studies
- Religion and Education
- Religion and Health
- Religion and Politics
- Religion and Science
- Religion and Law
- Religion and Art, Literature, and Music
- Religious Studies
- Browse content in Society and Culture
- Cookery, Food, and Drink
- Cultural Studies
- Customs and Traditions
- Ethical Issues and Debates
- Hobbies, Games, Arts and Crafts
- Lifestyle, Home, and Garden
- Natural world, Country Life, and Pets
- Popular Beliefs and Controversial Knowledge
- Sports and Outdoor Recreation
- Technology and Society
- Travel and Holiday
- Visual Culture
- Browse content in Law
- Arbitration
- Browse content in Company and Commercial Law
- Commercial Law
- Company Law
- Browse content in Comparative Law
- Systems of Law
- Competition Law
- Browse content in Constitutional and Administrative Law
- Government Powers
- Judicial Review
- Local Government Law
- Military and Defence Law
- Parliamentary and Legislative Practice
- Construction Law
- Contract Law
- Browse content in Criminal Law
- Criminal Procedure
- Criminal Evidence Law
- Sentencing and Punishment
- Employment and Labour Law
- Environment and Energy Law
- Browse content in Financial Law
- Banking Law
- Insolvency Law
- History of Law
- Human Rights and Immigration
- Intellectual Property Law
- Browse content in International Law
- Private International Law and Conflict of Laws
- Public International Law
- IT and Communications Law
- Jurisprudence and Philosophy of Law
- Law and Society
- Law and Politics
- Browse content in Legal System and Practice
- Courts and Procedure
- Legal Skills and Practice
- Primary Sources of Law
- Regulation of Legal Profession
- Medical and Healthcare Law
- Browse content in Policing
- Criminal Investigation and Detection
- Police and Security Services
- Police Procedure and Law
- Police Regional Planning
- Browse content in Property Law
- Personal Property Law
- Study and Revision
- Terrorism and National Security Law
- Browse content in Trusts Law
- Wills and Probate or Succession
- Browse content in Medicine and Health
- Browse content in Allied Health Professions
- Arts Therapies
- Clinical Science
- Dietetics and Nutrition
- Occupational Therapy
- Operating Department Practice
- Physiotherapy
- Radiography
- Speech and Language Therapy
- Browse content in Anaesthetics
- General Anaesthesia
- Neuroanaesthesia
- Browse content in Clinical Medicine
- Acute Medicine
- Cardiovascular Medicine
- Clinical Genetics
- Clinical Pharmacology and Therapeutics
- Dermatology
- Endocrinology and Diabetes
- Gastroenterology
- Genito-urinary Medicine
- Geriatric Medicine
- Infectious Diseases
- Medical Oncology
- Medical Toxicology
- Pain Medicine
- Palliative Medicine
- Rehabilitation Medicine
- Respiratory Medicine and Pulmonology
- Rheumatology
- Sleep Medicine
- Sports and Exercise Medicine
- Clinical Neuroscience
- Community Medical Services
- Critical Care
- Emergency Medicine
- Forensic Medicine
- Haematology
- History of Medicine
- Medical Ethics
- Browse content in Medical Dentistry
- Oral and Maxillofacial Surgery
- Paediatric Dentistry
- Restorative Dentistry and Orthodontics
- Surgical Dentistry
- Browse content in Medical Skills
- Clinical Skills
- Communication Skills
- Nursing Skills
- Surgical Skills
- Medical Statistics and Methodology
- Browse content in Neurology
- Clinical Neurophysiology
- Neuropathology
- Nursing Studies
- Browse content in Obstetrics and Gynaecology
- Gynaecology
- Occupational Medicine
- Ophthalmology
- Otolaryngology (ENT)
- Browse content in Paediatrics
- Neonatology
- Browse content in Pathology
- Chemical Pathology
- Clinical Cytogenetics and Molecular Genetics
- Histopathology
- Medical Microbiology and Virology
- Patient Education and Information
- Browse content in Pharmacology
- Psychopharmacology
- Browse content in Popular Health
- Caring for Others
- Complementary and Alternative Medicine
- Self-help and Personal Development
- Browse content in Preclinical Medicine
- Cell Biology
- Molecular Biology and Genetics
- Reproduction, Growth and Development
- Primary Care
- Professional Development in Medicine
- Browse content in Psychiatry
- Addiction Medicine
- Child and Adolescent Psychiatry
- Forensic Psychiatry
- Learning Disabilities
- Old Age Psychiatry
- Psychotherapy
- Browse content in Public Health and Epidemiology
- Epidemiology
- Public Health
- Browse content in Radiology
- Clinical Radiology
- Interventional Radiology
- Nuclear Medicine
- Radiation Oncology
- Reproductive Medicine
- Browse content in Surgery
- Cardiothoracic Surgery
- Gastro-intestinal and Colorectal Surgery
- General Surgery
- Neurosurgery
- Paediatric Surgery
- Peri-operative Care
- Plastic and Reconstructive Surgery
- Surgical Oncology
- Transplant Surgery
- Trauma and Orthopaedic Surgery
- Vascular Surgery
- Browse content in Science and Mathematics
- Browse content in Biological Sciences
- Aquatic Biology
- Biochemistry
- Bioinformatics and Computational Biology
- Developmental Biology
- Ecology and Conservation
- Evolutionary Biology
- Genetics and Genomics
- Microbiology
- Molecular and Cell Biology
- Natural History
- Plant Sciences and Forestry
- Research Methods in Life Sciences
- Structural Biology
- Systems Biology
- Zoology and Animal Sciences
- Browse content in Chemistry
- Analytical Chemistry
- Computational Chemistry
- Crystallography
- Environmental Chemistry
- Industrial Chemistry
- Inorganic Chemistry
- Materials Chemistry
- Medicinal Chemistry
- Mineralogy and Gems
- Organic Chemistry
- Physical Chemistry
- Polymer Chemistry
- Study and Communication Skills in Chemistry
- Theoretical Chemistry
- Browse content in Computer Science
- Artificial Intelligence
- Computer Architecture and Logic Design
- Game Studies
- Human-Computer Interaction
- Mathematical Theory of Computation
- Programming Languages
- Software Engineering
- Systems Analysis and Design
- Virtual Reality
- Browse content in Computing
- Business Applications
- Computer Games
- Computer Security
- Computer Networking and Communications
- Digital Lifestyle
- Graphical and Digital Media Applications
- Operating Systems
- Browse content in Earth Sciences and Geography
- Atmospheric Sciences
- Environmental Geography
- Geology and the Lithosphere
- Maps and Map-making
- Meteorology and Climatology
- Oceanography and Hydrology
- Palaeontology
- Physical Geography and Topography
- Regional Geography
- Soil Science
- Urban Geography
- Browse content in Engineering and Technology
- Agriculture and Farming
- Biological Engineering
- Civil Engineering, Surveying, and Building
- Electronics and Communications Engineering
- Energy Technology
- Engineering (General)
- Environmental Science, Engineering, and Technology
- History of Engineering and Technology
- Mechanical Engineering and Materials
- Technology of Industrial Chemistry
- Transport Technology and Trades
- Browse content in Environmental Science
- Applied Ecology (Environmental Science)
- Conservation of the Environment (Environmental Science)
- Environmental Sustainability
- Environmentalist Thought and Ideology (Environmental Science)
- Management of Land and Natural Resources (Environmental Science)
- Natural Disasters (Environmental Science)
- Nuclear Issues (Environmental Science)
- Pollution and Threats to the Environment (Environmental Science)
- Social Impact of Environmental Issues (Environmental Science)
- History of Science and Technology
- Browse content in Materials Science
- Ceramics and Glasses
- Composite Materials
- Metals, Alloying, and Corrosion
- Nanotechnology
- Browse content in Mathematics
- Applied Mathematics
- Biomathematics and Statistics
- History of Mathematics
- Mathematical Education
- Mathematical Finance
- Mathematical Analysis
- Numerical and Computational Mathematics
- Probability and Statistics
- Pure Mathematics
- Browse content in Neuroscience
- Cognition and Behavioural Neuroscience
- Development of the Nervous System
- Disorders of the Nervous System
- History of Neuroscience
- Invertebrate Neurobiology
- Molecular and Cellular Systems
- Neuroendocrinology and Autonomic Nervous System
- Neuroscientific Techniques
- Sensory and Motor Systems
- Browse content in Physics
- Astronomy and Astrophysics
- Atomic, Molecular, and Optical Physics
- Biological and Medical Physics
- Classical Mechanics
- Computational Physics
- Condensed Matter Physics
- Electromagnetism, Optics, and Acoustics
- History of Physics
- Mathematical and Statistical Physics
- Measurement Science
- Nuclear Physics
- Particles and Fields
- Plasma Physics
- Quantum Physics
- Relativity and Gravitation
- Semiconductor and Mesoscopic Physics
- Browse content in Psychology
- Affective Sciences
- Clinical Psychology
- Cognitive Neuroscience
- Cognitive Psychology
- Criminal and Forensic Psychology
- Developmental Psychology
- Educational Psychology
- Evolutionary Psychology
- Health Psychology
- History and Systems in Psychology
- Music Psychology
- Neuropsychology
- Organizational Psychology
- Psychological Assessment and Testing
- Psychology of Human-Technology Interaction
- Psychology Professional Development and Training
- Research Methods in Psychology
- Social Psychology
- Browse content in Social Sciences
- Browse content in Anthropology
- Anthropology of Religion
- Human Evolution
- Medical Anthropology
- Physical Anthropology
- Regional Anthropology
- Social and Cultural Anthropology
- Theory and Practice of Anthropology
- Browse content in Business and Management
- Business History
- Business Strategy
- Business Ethics
- Business and Government
- Business and Technology
- Business and the Environment
- Comparative Management
- Corporate Governance
- Corporate Social Responsibility
- Entrepreneurship
- Health Management
- Human Resource Management
- Industrial and Employment Relations
- Industry Studies
- Information and Communication Technologies
- International Business
- Knowledge Management
- Management and Management Techniques
- Operations Management
- Organizational Theory and Behaviour
- Pensions and Pension Management
- Public and Nonprofit Management
- Strategic Management
- Supply Chain Management
- Browse content in Criminology and Criminal Justice
- Criminal Justice
- Criminology
- Forms of Crime
- International and Comparative Criminology
- Youth Violence and Juvenile Justice
- Development Studies
- Browse content in Economics
- Agricultural, Environmental, and Natural Resource Economics
- Asian Economics
- Behavioural Finance
- Behavioural Economics and Neuroeconomics
- Econometrics and Mathematical Economics
- Economic Methodology
- Economic Systems
- Economic History
- Economic Development and Growth
- Financial Markets
- Financial Institutions and Services
- General Economics and Teaching
- Health, Education, and Welfare
- History of Economic Thought
- International Economics
- Labour and Demographic Economics
- Law and Economics
- Macroeconomics and Monetary Economics
- Microeconomics
- Public Economics
- Urban, Rural, and Regional Economics
- Welfare Economics
- Browse content in Education
- Adult Education and Continuous Learning
- Care and Counselling of Students
- Early Childhood and Elementary Education
- Educational Equipment and Technology
- Educational Strategies and Policy
- Higher and Further Education
- Organization and Management of Education
- Philosophy and Theory of Education
- Schools Studies
- Secondary Education
- Teaching of a Specific Subject
- Teaching of Specific Groups and Special Educational Needs
- Teaching Skills and Techniques
- Browse content in Environment
- Applied Ecology (Social Science)
- Climate Change
- Conservation of the Environment (Social Science)
- Environmentalist Thought and Ideology (Social Science)
- Natural Disasters (Environment)
- Social Impact of Environmental Issues (Social Science)
- Browse content in Human Geography
- Cultural Geography
- Economic Geography
- Political Geography
- Browse content in Interdisciplinary Studies
- Communication Studies
- Museums, Libraries, and Information Sciences
- Browse content in Politics
- African Politics
- Asian Politics
- Chinese Politics
- Comparative Politics
- Conflict Politics
- Elections and Electoral Studies
- Environmental Politics
- European Union
- Foreign Policy
- Gender and Politics
- Human Rights and Politics
- Indian Politics
- International Relations
- International Organization (Politics)
- International Political Economy
- Irish Politics
- Latin American Politics
- Middle Eastern Politics
- Political Theory
- Political Methodology
- Political Communication
- Political Philosophy
- Political Sociology
- Political Behaviour
- Political Economy
- Political Institutions
- Politics and Law
- Public Administration
- Public Policy
- Quantitative Political Methodology
- Regional Political Studies
- Russian Politics
- Security Studies
- State and Local Government
- UK Politics
- US Politics
- Browse content in Regional and Area Studies
- African Studies
- Asian Studies
- East Asian Studies
- Japanese Studies
- Latin American Studies
- Middle Eastern Studies
- Native American Studies
- Scottish Studies
- Browse content in Research and Information
- Research Methods
- Browse content in Social Work
- Addictions and Substance Misuse
- Adoption and Fostering
- Care of the Elderly
- Child and Adolescent Social Work
- Couple and Family Social Work
- Developmental and Physical Disabilities Social Work
- Direct Practice and Clinical Social Work
- Emergency Services
- Human Behaviour and the Social Environment
- International and Global Issues in Social Work
- Mental and Behavioural Health
- Social Justice and Human Rights
- Social Policy and Advocacy
- Social Work and Crime and Justice
- Social Work Macro Practice
- Social Work Practice Settings
- Social Work Research and Evidence-based Practice
- Welfare and Benefit Systems
- Browse content in Sociology
- Childhood Studies
- Community Development
- Comparative and Historical Sociology
- Economic Sociology
- Gender and Sexuality
- Gerontology and Ageing
- Health, Illness, and Medicine
- Marriage and the Family
- Migration Studies
- Occupations, Professions, and Work
- Organizations
- Population and Demography
- Race and Ethnicity
- Social Theory
- Social Movements and Social Change
- Social Research and Statistics
- Social Stratification, Inequality, and Mobility
- Sociology of Religion
- Sociology of Education
- Sport and Leisure
- Urban and Rural Studies
- Browse content in Warfare and Defence
- Defence Strategy, Planning, and Research
- Land Forces and Warfare
- Military Administration
- Military Life and Institutions
- Naval Forces and Warfare
- Other Warfare and Defence Issues
- Peace Studies and Conflict Resolution
- Weapons and Equipment

- < Previous chapter
- Next chapter >
2 Tasks, Task Sets, and the Mapping Between Them
- Published: June 2014
- Cite Icon Cite
- Permissions Icon Permissions
This chapter asks what actually constitutes a task. It has long been suggested that task switching requires implementing a task set, but the nature of the task set itself often goes unspecified. How can we tell whether task set reconfiguration has occurred if we are not clear what a task set really is? It is argued here that task switching research—and its associated theorizing—has been hampered by poor definitions of what are tasks and task sets. The chapter provides a distinction between the two: a task is the representation of a set of instructions required to perform an activity accurately; a task set is the set of representations and processes that enable execution of the task. It argues that contrary to the often-held assumption that switching between two tasks in a task switching paradigm requires switching between two task sets, tasks and task sets do not necessarily have a one-to-one mapping. It provides recommendations for how to explore the nature of the relationship between tasks and task sets.
Signed in as
Institutional accounts.
- GoogleCrawler [DO NOT DELETE]
- Google Scholar Indexing
Personal account
- Sign in with email/username & password
- Get email alerts
- Save searches
- Purchase content
- Activate your purchase/trial code
Institutional access
- Sign in with a library card Sign in with username/password Recommend to your librarian
- Institutional account management
- Get help with access
Access to content on Oxford Academic is often provided through institutional subscriptions and purchases. If you are a member of an institution with an active account, you may be able to access content in one of the following ways:
IP based access
Typically, access is provided across an institutional network to a range of IP addresses. This authentication occurs automatically, and it is not possible to sign out of an IP authenticated account.
Sign in through your institution
Choose this option to get remote access when outside your institution. Shibboleth/Open Athens technology is used to provide single sign-on between your institution’s website and Oxford Academic.
- Click Sign in through your institution.
- Select your institution from the list provided, which will take you to your institution's website to sign in.
- When on the institution site, please use the credentials provided by your institution. Do not use an Oxford Academic personal account.
- Following successful sign in, you will be returned to Oxford Academic.
If your institution is not listed or you cannot sign in to your institution’s website, please contact your librarian or administrator.
Sign in with a library card
Enter your library card number to sign in. If you cannot sign in, please contact your librarian.
Society Members
Society member access to a journal is achieved in one of the following ways:
Sign in through society site
Many societies offer single sign-on between the society website and Oxford Academic. If you see ‘Sign in through society site’ in the sign in pane within a journal:
- Click Sign in through society site.
- When on the society site, please use the credentials provided by that society. Do not use an Oxford Academic personal account.
If you do not have a society account or have forgotten your username or password, please contact your society.
Sign in using a personal account
Some societies use Oxford Academic personal accounts to provide access to their members. See below.
A personal account can be used to get email alerts, save searches, purchase content, and activate subscriptions.
Some societies use Oxford Academic personal accounts to provide access to their members.
Viewing your signed in accounts
Click the account icon in the top right to:
- View your signed in personal account and access account management features.
- View the institutional accounts that are providing access.
Signed in but can't access content
Oxford Academic is home to a wide variety of products. The institutional subscription may not cover the content that you are trying to access. If you believe you should have access to that content, please contact your librarian.
For librarians and administrators, your personal account also provides access to institutional account management. Here you will find options to view and activate subscriptions, manage institutional settings and access options, access usage statistics, and more.
Our books are available by subscription or purchase to libraries and institutions.
- About Oxford Academic
- Publish journals with us
- University press partners
- What we publish
- New features
- Open access
- Rights and permissions
- Accessibility
- Advertising
- Media enquiries
- Oxford University Press
- Oxford Languages
- University of Oxford
Oxford University Press is a department of the University of Oxford. It furthers the University's objective of excellence in research, scholarship, and education by publishing worldwide
- Copyright © 2024 Oxford University Press
- Cookie settings
- Cookie policy
- Privacy policy
- Legal notice
This Feature Is Available To Subscribers Only
Sign In or Create an Account
This PDF is available to Subscribers Only
For full access to this pdf, sign in to an existing account, or purchase an annual subscription.
Thank you for visiting nature.com. You are using a browser version with limited support for CSS. To obtain the best experience, we recommend you use a more up to date browser (or turn off compatibility mode in Internet Explorer). In the meantime, to ensure continued support, we are displaying the site without styles and JavaScript.
- View all journals
- My Account Login
- Explore content
- About the journal
- Publish with us
- Sign up for alerts
- Open access
- Published: 08 December 2015
Cognitive caching promotes flexibility in task switching: evidence from event-related potentials
- Florian Lange 1 ,
- Caroline Seer 1 ,
- Dorothea Müller 1 &
- Bruno Kopp 1
Scientific Reports volume 5 , Article number: 17502 ( 2015 ) Cite this article
2151 Accesses
22 Citations
Metrics details
- Cognitive control
Time-consuming processes of task-set reconfiguration have been shown to contribute to the costs of switching between cognitive tasks. We describe and probe a novel mechanism serving to reduce the costs of task-set reconfiguration. We propose that when individuals are uncertain about the currently valid task, one task set is activated for execution while other task sets are maintained at a pre-active state in cognitive cache. We tested this idea by assessing an event-related potential (ERP) index of task-set reconfiguration in a three-rule task-switching paradigm involving varying degrees of task uncertainty. In high-uncertainty conditions, two viable tasks were equally likely to be correct whereas in low-uncertainty conditions, one task was more likely than the other. ERP and performance measures indicated substantial costs of task-set reconfiguration when participants were required to switch away from a task that had been likely to be correct. In contrast, task-set-reconfiguration costs were markedly reduced when the previous task set was chosen under high task uncertainty. These results suggest that cognitive caching of alternative task sets adds to human cognitive flexibility under high task uncertainty.
Similar content being viewed by others
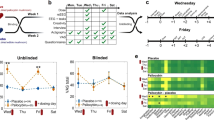
Microdosing with psilocybin mushrooms: a double-blind placebo-controlled study
Federico Cavanna, Stephanie Muller, … Enzo Tagliazucchi
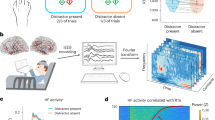
Neural evidence for attentional capture by salient distractors
Rongqi Lin, Xianghong Meng, … Benchi Wang
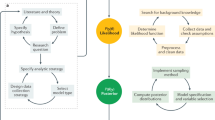
Bayesian statistics and modelling
Rens van de Schoot, Sarah Depaoli, … Christopher Yau
Introduction
Variable and uncertain environments pose an immense challenge on the flexibility of human cognition in everyday life. How do our minds and brains manage to keep up with environmental change and to shift between different cognitive tasks on a frequent basis? Research employing the experimental task-switching paradigm has provided some intriguing answers to this question by advancing our understanding of the mechanisms underlying and the limitations constraining cognitive flexibility 1 , 2 . When individuals are required to switch tasks, it is now widely accepted that time-consuming processes of active task-set reconfiguration (TSR) are recruited to allow for successful adaptation 3 . TSR is thought to involve the retrieval of an inactive task set from long-term memory into procedural working memory (WM) 2 , 4 . This notion does not necessarily imply that task-set activation occurs in an all-or-none fashion. In fact, influential task-switching models assume that all viable task sets are represented simultaneously while only quantitative differences in attentional weights determine which task will be executed 5 , 6 . It is unlikely that processing resources focus entirely on the relevant task set while the irrelevant task set is completely deactivated. Here, we build on the idea that task sets can not only be represented in an active or an inactive state, but also in a preactive state 7 . This third activation state may provide human cognition with an additional degree of flexibility. Specifically, we propose that when it is not clear which of two alternative tasks is most adaptive in a given situation, one task is retrieved for execution into WM, while the other task is maintained in a cognitive cache . When incoming information then favors a shift to the task set in cache, information relevant to the new task can be retrieved fast and efficiently.
This notion is especially appealing because a mechanism of cognitive caching would enable the concurrent pursuit of two different task goals 7 . The existence of a system simulating an alternative to overt action would raise human cognitive flexibility to a new level 7 . Drawing on a cognitive cache, human cognition would not only be adaptive, but preadaptive (i.e., able to adjust to changes that are sufficiently likely to occur in the future) 8 .
Our model of cognitive caching is closely related to notions of counterfactual thinking 9 , mental simulation 10 or what Jonathan Evans has called hypothetical thinking 11 . According to Evans 11 , the ability to mentally simulate future possibilities is characteristic for the distinctively human functions of the reflective mind. Consistent with this idea, fMRI studies have mapped the cognitive cache onto the frontopolar cortex 7 , 12 , 13 , a cortical region that has evolved late in phylogeny and that has no equivalent in the brain of non-human primates 14 .
Constrained by these neuroimaging data, our model conceives the involvement of a cognitive cache to be dependent on the uncertainty over task sets in a given situation. Cognitive caching occurs selectively when subjects are uncertain about which of two alternative tasks is currently prevailing (i.e., when it would be “too risky to simply abandon one” 7 (p. 595)). In other words, information relevant to an alternative task should only be cached when the task is sufficiently likely to be valid (see Fig. 1 ).
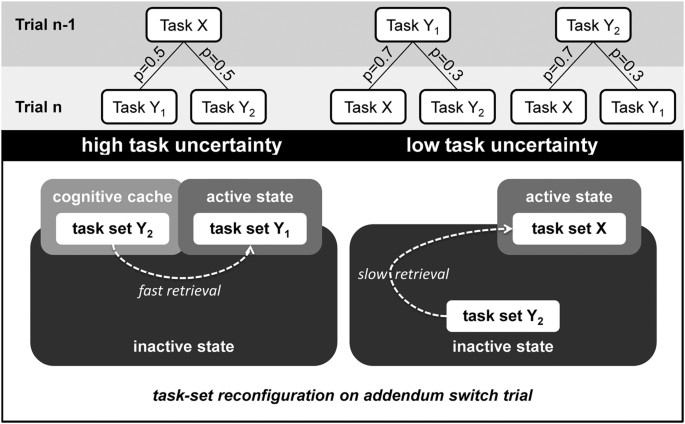
Illustration of our manipulation of task uncertainty (top) and its hypothesized effects on the activation of task sets (bottom).
Under high task uncertainty, participants had to decide between two tasks that were equally likely to be correct. We propose that in these situations, individuals activate one task set for execution and keep the unchosen task set in a pre-active state in cognitive cache, thereby facilitating later retrieval of this task set. Under low task uncertainty, participants knew that one task was more likely to be correct than the other. We propose that in these situations, individuals largely refrain from cognitive caching, rendering later retrieval of the alternative task set slow and inefficient.
The aim of the present analysis was twofold: First, we aimed at producing initial evidence for cognitive caching in the context of task switching and at examining whether this mechanism has the potential to promote cognitive flexibility. Second, we addressed the question whether recruitment of a cognitive cache is indeed sensitive to the probabilistic context, i.e., to the current level of task uncertainty.
Participants’ performance was examined when switching tasks under high-uncertainty (two tasks are equally likely) and low-uncertainty (one task is more likely than the other) conditions ( Fig. 1 ). We hypothesized that in low-uncertainty conditions, participants largely refrain from cognitive caching. When the initially chosen task proves to be invalid, information regarding the alternative task cannot be retrieved from cache, rendering TSR slow and inefficient. In high-uncertainty conditions, however, retrieval of the alternative task set should be less costly as it is kept at a pre-active representation level in cognitive cache.
Our analysis of behavioral performance was complemented by the investigation of switch-specific event-related potentials (ERPs). Task-switching is reliably associated with a prominent, positive-going waveform over parietal electrodes 15 , 16 , 17 , 18 , 19 , 20 . This posterior switch positivity (PSP) is commonly interpreted as a direct measure of the neural substrates underlying anticipatory TSR 21 . Being proportional to the neural costs of TSR, the amplitude of the PSP was expected to be lower when the alternative task set can be retrieved from cognitive cache (i.e., in high-uncertainty conditions). Similarly, in these conditions, response times and errors rates were predicted to be reduced when compared to low-uncertainty conditions without cache involvement.
Participants
Eighteen young and healthy students (14 female; one left-handed; mean age = 25.6 years, SD = 6.7 years, range 19–42 years) participated for course credit. All participants had normal or corrected-to-normal vision. In line with previous task-switching experiments using the ERP technique, we initially aimed at a sample size of 24 16 , 22 . Unfortunately, we had to exclude the first six participants because their EEG data could not be analyzed due to technical problems during the recording session. The study has been approved by the Ethics Committee of Hannover Medical School and was carried out in accordance with the approved guidelines. Informed consent was obtained from all subjects.
Task and Apparatus
Participants completed variants of a three-rule task-switching procedure modeled after the Wisconsin Card Sorting Test (WCST) 23 , 24 , 25 . The task was designed using Presentation® software (Neurobehavioral Systems, Albany, CA) and displayed on a 24 inch flat screen (Eizo EV2416W, Eizo, Hakusan, Japan). Responses were collected by a Cedrus response pad (RB 830, Cedrus, San Pedro, CA).
The task switching paradigm required participants to match a target card to one of four key cards according to a particular sorting rule that changed from time to time 17 , 26 , 27 . Cards were configured around the center of the screen with the target card appearing below the key cards (see Fig. 2 ). Participants indicated their sorting choice by pressing one of four keys on the response pad which mapped to the spatial position of the key cards on the screen. Target cards varied on three dimensions (color, shape, number) and these dimensions equaled the three viable task rules. As the target card never shared more than one attribute with any of the keycards, the applied sorting rule could unambiguously be identified 28 , 29 . Target displays remained on screen until a response was registered.
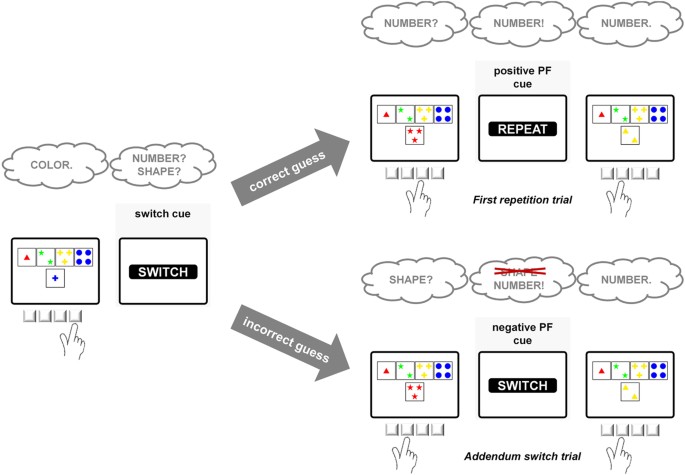
Task flow of the task-switching paradigm modeled after the Wisconsin Card Sorting Test.
Participants were instructed to match a target card (by pressing a respective key) to one of four key cards according to the correct task. Feedback cues following each sorting response indicated whether the applied task had to be switched or repeated. Following a switch cue, participants were required to guess which of the two remaining tasks was now correct. If they had guessed correctly, they were presented with positive post-switch feedback (positive PF) initiating a first repetition trial. If they had guessed incorrectly, they were presented with negative post-switch feedback (negative PF) initiating an addendum switch trial. Both positive and negative PF allowed inducing the correct task rule, but only negative PF required an additional switch of tasks.
After a fixed response-cue interval (RCI) of 800 ms, a feedback cue was presented for 400 ms indicating whether the applied sorting rule should be maintained (“REPEAT”, “STAY”) or changed (“SWITCH”) on the upcoming trial. Feedback cues were presented in white letters (31 point Arial) in a black box in the center of the screen. Subsequent target stimuli appeared after a fixed cue-target interval (CTI) of 1200 ms.
We used two different repeat cues to avoid confounding of task-switch costs and cue-switch costs 30 . Because the comparisons presented here are not affected by this confound, this methodological detail will not be of further relevance. As a side note, no significant main effect or interaction involving cue sequence could be detected on any of our measures.
The task switching paradigm we used resembled the search task introduced by Mayr and Keele with one critical exception 31 . In their paradigm, a task cue explicitly indicated which of three possible stimulus dimensions is currently relevant. In contrast, we used transition cues 32 that provided only implicit information about the currently valid task rule in order to be able to study the role of task uncertainty in task switching. Specifically, switch cues (i.e., “SWITCH” feedback after a series of rule repetitions) signaled that the previously adopted task rule had changed, thus rendering participants uncertain about which one of the two remaining rules was correct 33 . Ideally performing participants would choose one of the two remaining rules on the subsequent trial (i.e., the switch trial ). In the remainder of this article, the feedback cues that followed participants’ initial switching attempt are called post-switch feedback (PF) cues. PF could either be positive (positive PF) or negative (negative PF). While positive PF signaled that participants had chosen the correct rule which now can be maintained, negative PF indicated that participants had to switch rules again in order to find the correct new rule. We refer to the trials characterized by this demand for a further switch of sorting rules (i.e., the trials following negative PF) as addendum switch trials . In contrast, trials following positive PF are called first repetition trials .
Design and Procedure
Rules changed in an unpredictable manner 34 after runs of two or more repetition trials. Switch probability was manipulated by varying the mean length of task runs across blocks (high switch probability: M = three repetition trials; low switch probability: M = four repetition trials). Each participant completed three blocks of trials amounting to 112 task runs in both the high switch probability condition and the low switch probability condition. The order of high switch probability blocks and low switch probability blocks was counterbalanced across participants. Switch probability was manipulated to evaluate whether PSP amplitude simply varied as a function of event probability (see Discussion).
More crucially, we manipulated the conditional probability of one of the two remaining rules being correct when participants switched away from the third rule. In high-uncertainty conditions, both possible rules were equally likely to be correct. In low-uncertainty conditions, one of the possible rules was correct in 70% of the cases. By developing a pseudorandomized rule sequence, we ascertained that the actual frequencies of transitions from one rule to another corresponded to these conditional probabilities in each participant and in each of the switch probability conditions.
As a consequence of this manipulation, one of the three rules was more frequent than the other two. Participants were explicitly instructed about the most frequent rule and about the conditional probabilities for the rule transitions. The more frequent rule (color, shape, or number) was counterbalanced across participants.
Prior to each of the switch probability conditions, eight practice runs were administered. Participants were informed about the three possible sorting criteria and about the fact that the valid task rule would change from time to time in an unpredictable manner. They were told to attend to the feedback cues in order to infer the correct task rule.
Electrophysiological Recordings
Continuous electroencephalogram was recorded from 30 active Ag-AgCl electrodes (BrainProducts, Gilching, Germany) placed according to the international 10–20 system. BrainVision Recorder software (Brain Products, Gilching, Germany) was used. Electrode impedance was kept below 10 kΩ. Electrodes were referenced to FCz electrode. Vertical (vEOG) and horizontal (hEOG) electrooculogram were recorded with two additional electrodes positioned at the suborbital ridge and the external ocular canthus of the right eye. EEG and EOG channels were digitized at 250 Hz and amplified using a BrainAmp amplifier (Brain Products, Gilching, Germany).
Data Analysis
Statistical analyses were performed using SPSS 22.0 (IBM, Armonk, NY). The level of significance was set at α = 0.01. Effect size confidence intervals were calculated according to the method proposed by Steiger 35 using the syntax developed by Karl Wuensch (core.ecu.edu/psyc/wuenschk/SPSS/CI-R2-SPSS.zip).
Behavioral Data
Mean response times (RTs) and error rates (ER) were calculated separately for switch trials, addendum switch trials and first repetition trials. For RT analysis, we excluded RTs faster than 100 ms or slower than 3000 ms. Trials were only included when participants sorted according to a viable rule. Hence, switch trials were only included when participants switched to one of the two remaining rules (i.e., when they did not perseverate). Addendum switch trials were only considered when participants did not perseverate on the previous switch trial and when they switched to the correct rule on the addendum switch trial. First repetition trials were included when participants repeated sorting according to the correct rule. For ER analysis, trials were considered incorrect when participants did not sort according to a viable rule. Hence, responses on switch trials were only considered incorrect when participants committed a perseverative error. Responses on addendum switch trials (i.e., when participants were given the possibility to know the identity of the correct rule) were considered incorrect when participants did not identify the correct task. Addendum switch trials following a perseverative error on the switch trial were excluded from ER analysis because on these trials participants did not receive the information necessary to infer the correct rule. Responses on first repetition trials were considered incorrect when participants did not maintain the previously applied rule.
Effects of task uncertainty and switch probability on switching performance were evaluated by means of 2 (task uncertainty: high uncertainty vs. low uncertainty) × 2 (switch probability: high probability vs. low probability) × 2 (trial type: switch vs. first repetition) analyses of variance (ANOVAs) on RT and ER. Effects of task uncertainty and switch probability on addendum switching were evaluated by means of 2 (task uncertainty: high uncertainty vs. low uncertainty) × 2 (switch probability: high probability vs. low probability) × 2 (trial type: addendum switch vs. first repetition) analyses of variance (ANOVAs) on RT and ER.
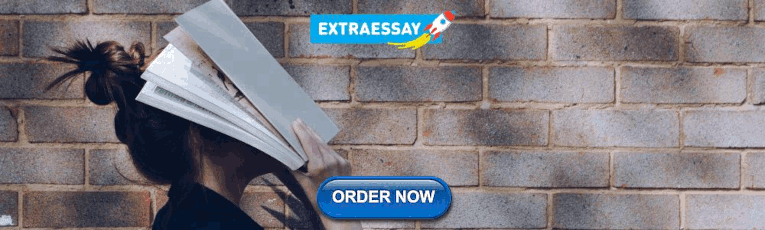
Electrophysiological data
Electrophysiological data were evaluated using BrainVision Analyzer 2.0 (Brain Products, Gilching, Germany). After filtering (high-pass: 0.5 Hz, low-pass: 70 Hz, notch: 50 Hz), data were screened for non-stereotyped artifacts and subjected to an ocular-correction independent component analysis 36 for further removal of ocular, muscular and cardiac artifacts. Data were re-referenced to a common average reference offline and segmented into epochs from −200 to 1000 ms relative to the onset of feedback cues. After baseline-correction (−200 to 0 ms), residual artifacts were rejected semi-automatically before data were averaged.
ERPs were locked to switch cues, positive PF cues and negative PF cues. PSP was analyzed in the time window from 600 to 800 ms at electrode Pz. PSP was defined as the switch-related amplitude increase of the sustained positive potential typically observed at this time point and location 37 , 17 . Effects of task uncertainty and switch probability on switch-related amplitude modulations were evaluated by means of a 2 (task uncertainty: high uncertainty vs. low uncertainty) × 2 (switch probability: high probability vs. low probability) × cue type (switch vs. positive PF) ANOVA. Effects of task uncertainty and switch probability on addendum switch-related amplitude modulations were evaluated by means of a 2 (task uncertainty: high uncertainty vs. low uncertainty) × 2 (switch probability: high probability vs. low probability) × cue type (negative PF vs. positive PF) ANOVA. Note that this latter ANOVA was performed to evaluate the key hypothesis of our study, namely that the neural costs of reconfiguration (as indexed by PSP amplitude) are lower when the alternative task set has been cached (i.e., in high-uncertainty conditions) before negative PF signals the need for a further task switch on addendum switch trials.
An overview of switch-specific and addendum-switch-specific statistical results including effect sizes and confidence intervals is given in Table 1 . In the following, effects will be described in more detail when their 98% confidence intervals do not include zero (corresponding to an α level of 0.01) 35 .
Switch-specific effects
The task uncertainty × switch probability × trial type ANOVA revealed a significant main effect of trial type on mean RT, F (1, 17) = 31.01, p < 0.001, η p 2 = 0.65. Responses were substantially slower on switch (955 ms) than on first repetition trials (799 ms). This effect was qualified by a significant task uncertainty × trial type interaction, F (1, 17) = 14.86, p = 0.001, η p 2 = 0.47, indicating that RT switch costs were lower in the low-uncertainty condition (82 ms) than in the high-uncertainty condition (230 ms; see Fig. 3 ).
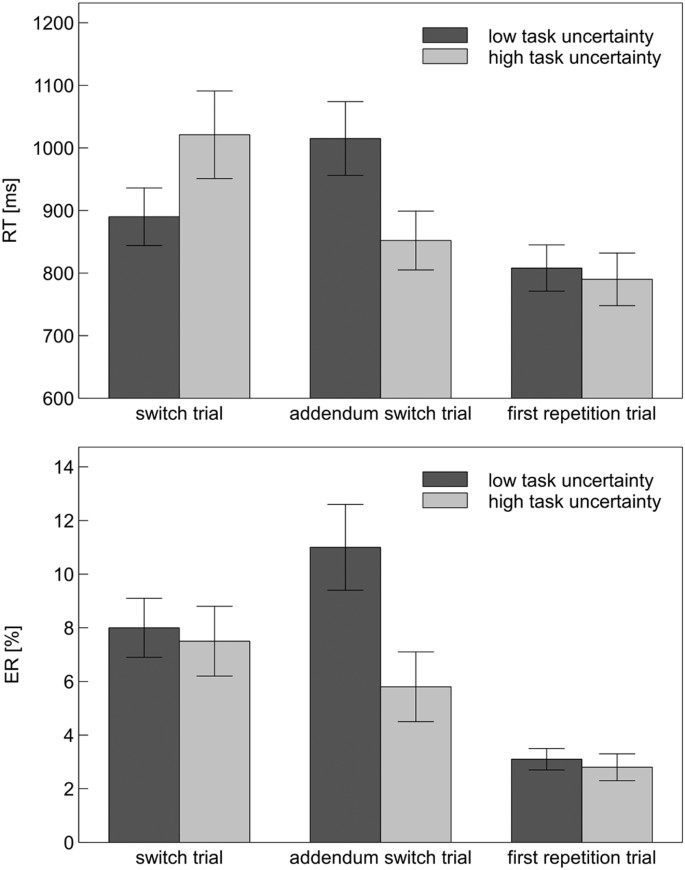
Mean latency and accuracy of responses on switch, addendum switch and first repetition trials.
Error bars indicate standard error of the mean.
With regard to ER, the task uncertainty × switch probability × trial type ANOVA showed an effect of trial type, F (1, 17) = 20.14, p <0.001, η p 2 = 0.54, with more errors on switch (8%) than on first repetition trials (3%).
The analysis of PSP amplitude at electrode Pz yielded a significant main effect of cue type, F (1, 17) = 24.65, p < 0.001, η p 2 = 0.59, in the absence of any significant effects or interactions involving the factors task uncertainty and switch probability. The sustained positive potential was larger following switch cues (1.97 μV) than after positive PF cues (−0.03 μV). This effect is displayed in Fig. 4 . Inspection of Fig. 4 also reveals that PSP amplitude is neither sensitive to the probability of a switching event nor to the degree of task uncertainty per se.
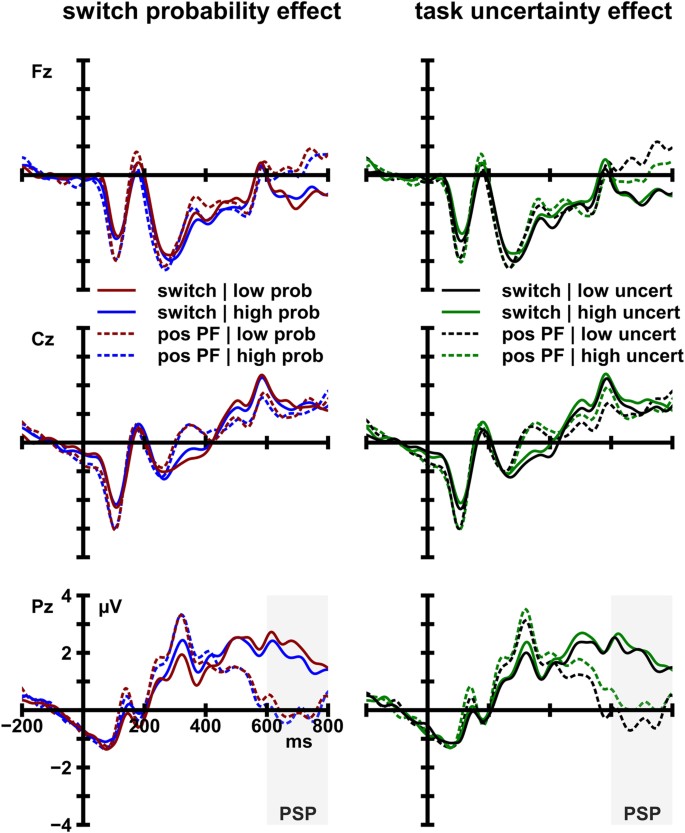
Grand average ERP waves at midline sites elicited by switch cues and positive post-switch feedback (pos PF) cues.
Waveforms are depicted as a function of switch probability (low probability [low prob] vs. high probability [high prob] (left) and task uncertainty (low task uncertainty [low uncert] vs. high task uncertainty [high uncert] (right).
Addendum-switch-specific effects
The task uncertainty × switch probability × trial type ANOVA revealed significant main effects of trial type, F (1, 17) = 35.30, p < 0.001, η p 2 = 0.68 and task uncertainty, F (1, 17) = 12.04, p = 0.003, η p 2 = 0.42, on mean RT. Responses were substantially slower on addendum switch (934 ms) as compared to first repetition (799 ms) trials and in low-uncertainty (912 ms) as compared to high-uncertainty conditions (821 ms). These effects were moderated by a significant task uncertainty × trial type interaction, F (1, 17) = 15.64, p = 0.001, η p 2 = 0.48, indicating that RT addendum switch costs were higher in the low-uncertainty condition (207 ms) than in the high-uncertainty condition (62 ms; see Fig. 3 ).
The same pattern could be observed with regard to ER, with significant main effects of trial type, F (1, 17) = 15.64, p = 0.001, η p 2 = 0.48 and task uncertainty, F (1, 17) = 21.31, p < 0.001, η p 2 = 0.56, as well as a task uncertainty × trial type interaction, F (1, 17) = 11.00, p = 0.004, η p 2 = 0.39. Participants committed more errors on addendum switch (8%) than on first repetition trials (3%) and in low-uncertainty (7%) as compared to high-uncertainty conditions (4%). ER switch costs were larger in low-uncertainty (8%) as compared to high-uncertainty conditions (3%).
Similar to the switch-cost analysis, the amplitude of the sustained positive potential was significantly larger following negative PF (1.67 μV) than after positive PF cues (-0.03 μV), F (1, 17) = 30.12, p < 0.001, η p 2 =0.64. Hence, a PSP could also be observed on addendum switch trials. Importantly, the amplitude of the PSP was substantially larger in low-uncertainty conditions (2.34 μV) as compared to high-uncertainty conditions (1.04 μV). A significant interaction of the factors cue type and task uncertainty, F (1, 17) = 15.23, p = 0.001, η p 2 = 0.41, corroborates that PSP amplitude was reliably modulated by task uncertainty ( Figs 5 and 6 ).
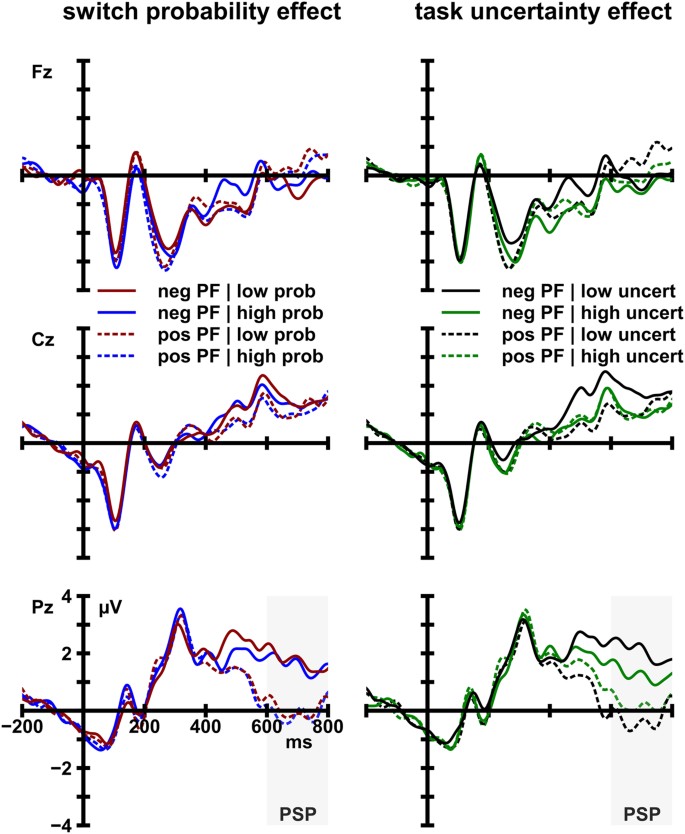
Grand average ERP waves at midline sites elicited by negative post-switch feedback (neg PF) cues and positive post-switch feedback (pos PF) cues.
Waveforms are depicted as a function of switch probability (low probability [low prob] vs. high probability [high prob]) (left) and task uncertainty (low task uncertainty [low uncert] vs. high task uncertainty [high uncert]) (right).
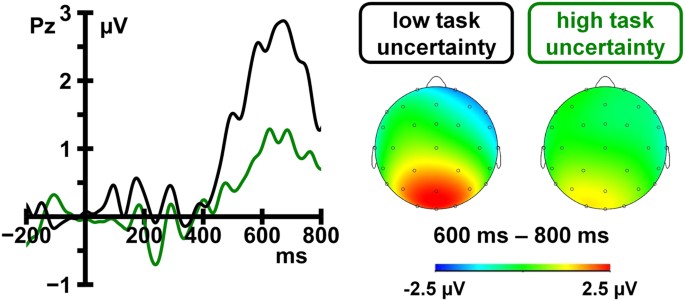
Difference waves and scalp maps illustrating the effect of task uncertainty on neural addendum switch costs.
ERP waveforms elicited by positive post-switch feedback cues were subtracted from ERP waveforms elicited by negative post-switch feedback cues to obtain the neural activity specific to addendum switch operations (left). Scalp maps (right) depict the topography of this addendum-switch-specific activity.
Using variants of a task-switching paradigm, we investigated the behavioral and neural costs of switching to a new task as a function of task uncertainty. Switching was faster and more efficient when uncertainty about the correct task was low. However, when the chosen task proved to be invalid (i.e., when participants had to switch again on addendum switch trials) the costs of switching to an alternative task were substantially increased in these low-uncertainty conditions. This pattern of increased addendum switch costs in low-uncertainty conditions was not only evident in the speed and accuracy of responses to target stimuli, but also in the amplitude of the PSP, an ERP measure of anticipatory TSR prior to target onset.
These data are suggestive of the uncertainty-dependent recruitment of a cognitive cache. When participants can be relatively certain about the currently valid task, they appear to commit to this particular task: they activate the corresponding task set for execution and do not (or to a substantially lesser extent) keep information relevant to an alternative task at a pre-active representation level in cognitive cache. When performance feedback then signals that participants have committed to the wrong task, retrieving the alternative task set from its relatively inactive state in long-term memory is costly and effortful (as indicated by increased RT, ER and PSP amplitudes). These costs of TSR are remarkably reduced when the alternative task set can be retrieved from a pre-active state in cognitive cache. We propose that the cognitive cache is selectively recruited in situations involving high uncertainty about the currently valid task. In these situations, participants do not only retrieve one task set into an active state for execution, but they also prepare for the hypothetical but not unlikely event that the chosen task is not correct. Specifically, our model assumes that they retrieve the alternative task set from long-term memory into a pre-active state in cognitive cache. This process of cognitive caching, mental simulation 10 , or hypothetical thinking 11 appears to substantially promote participants’ flexibility in the task-switching procedure: the alternative task set in cognitive cache is more readily accessible and, as a consequence, the behavioral and neural costs of TSR on addendum switch trials are lower in high-uncertainty conditions.
Our methodological approach of combining behavioral and electrophysiological measures and distinguishing between switch and addendum switch conditions allowed us to rule out some alternative explanations for the observed pattern of results. First, it might be argued that increased addendum switch costs in low-uncertainty conditions are not due to an increased demand for TSR but rather to stronger effects of task-set inertia 38 . When participants have to switch away from a task they have committed to, the activation of the associated task set and, hence, the interference with the response to the next target stimulus might be stronger. However, such a stronger tendency to sort a target stimulus according to the old task set would only explain effects of task uncertainty on behavioral addendum switch costs. The effect of task uncertainty on PSP amplitude was observed prior to target onset and can thus not be attributed to the interference of task-set inertia with processing of the target stimulus. Future studies manipulating the CTI and the RCI might further differentiate the processes contributing to the uncertainty-related modulation of addendum switch costs observed in our study. Second, the nature of our manipulation of task uncertainty dictates that negative PF is less likely in the low-uncertainty condition than in the high-uncertainty condition. Hence, larger PSP amplitudes following negative PF in the low-uncertainty condition might result from a potential effect of event probability on PSP amplitude. However, when investigating PSP responses to switch cues as a function of switch probability, we did not find PSP amplitude to be probability sensitive. This result is consistent with previous studies suggesting that late (>500 ms) PSP waveforms reflect TSR processes that are not affected by the switch probability context 22 , 39 . Third, it is possible that negative PF in low-uncertainty conditions render participants confused and uncertain about the currently valid task and increased PSP amplitudes might reflect processes of signaling or overcoming this uncertainty. Again, this explanation does not account for our data because PSP amplitudes on switch trials did not vary as a function of task uncertainty ( Fig. 7 ).
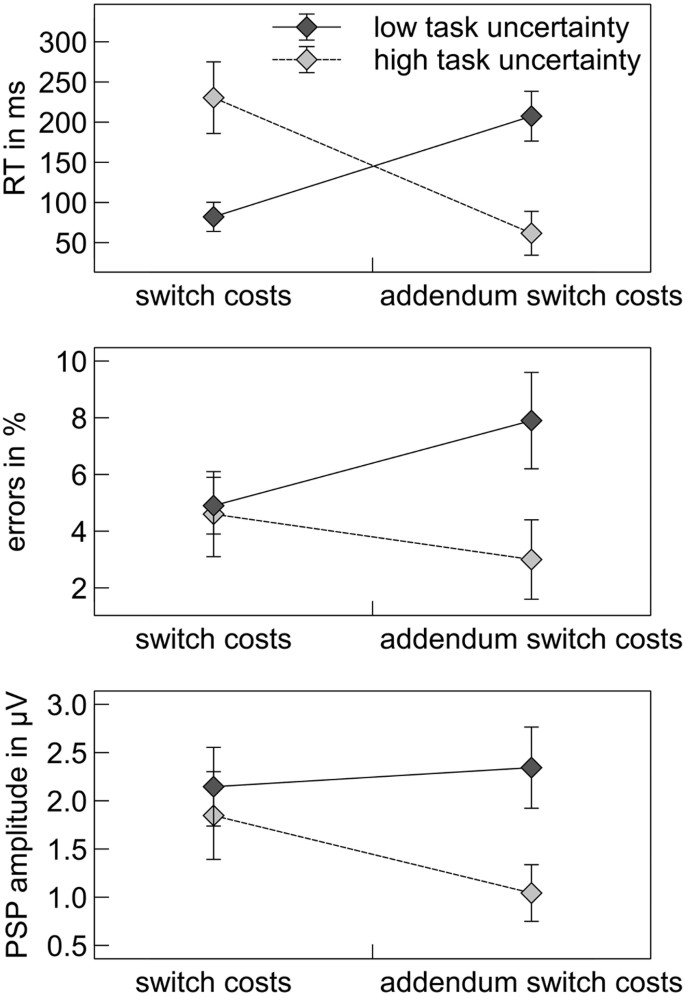
Behavioral and electrophysiological switch costs (switch trial – first repetition trial) and addendum switch costs (addendum switch trial – first repetition trial), separately for low-uncertainty conditions and high-uncertainty conditions.
In future studies, it may prove worthwhile to examine the similarities between the idea of cognitive caching and extant models of working memory processes. The models of Cowan 40 and Oberauer 41 , for example, refer to different functional states of information in working memory. According to these models, the information used in ongoing cognitive processes is held in a region of direct access (i.e., inside the focus of attention), whereas an additional subset of long-term memory is activated outside this focus of attention. In fact, this distinction between working memory information inside and outside the focus of attention has already been linked to the relationship between active task sets and cached task sets 42 . However, further theoretical and empirical work is required to investigate commonalities between these different approaches to human cognition.
To conclude, the observed pattern of reduced addendum switch costs following task selections under high uncertainty can be best explained by the strategic recruitment of a cognitive cache. Being uncertain about which task to pursue, individuals necessarily decide for one task, but they do not completely discard alternative tasks if these are also sufficiently likely to be valid. The process of simulating and preparing the alternative appears to be highly adaptive as it allows for smooth adjustments to constantly changing environmental demands.
Additional Information
How to cite this article : Lange, F. et al. Cognitive caching promotes flexibility in task switching: evidence from event-related potentials. Sci. Rep. 5 , 17502; doi: 10.1038/srep17502 (2015).
Kiesel, A. et al. Control and interference in task switching—A review. Psychol. Bull. 136, 849–874 (2010).
Article Google Scholar
Monsell, S. Task switching. Trends Cogn. Sci. 7, 134–140 (2003).
Vandierendonck, A., Liefooghe, B. & Verbruggen, F. Task switching: interplay of reconfiguration and interference control. Psychol. Bull. 136, 601–626 (2010).
Rubinstein, J. S., Meyer, D. E. & Evans, J. E. Executive control of cognitive processes in task switching. J. Exp. Psychol. Human 27, 763–797 (2001).
Article CAS Google Scholar
Meiran, N. Modeling cognitive control in task-switching. Psychol. Res. 63, 234–249 (2000).
Meiran, N., Kessler, Y. & Adi-Japha, E. Control by action representation and input selection (CARIS): A theoretical framework for task switching. Psychol. Res. 72, 473–500 (2008).
Koechlin, E. & Hyafil, A. Anterior prefrontal function and the limits of human decision-making. Science 318, 594–598 (2007).
Article CAS ADS Google Scholar
Fuster, J. M. The prefrontal cortex makes the brain a preadaptive system. Proc. IEEE 102, 414–426 (2014).
Byrne, R. M. Mental models and counterfactual thoughts about what might have been. Trends Cogn. Sci. 6, 426–431 (2002).
Kahneman, D. & Tversky, A. The simulation heuristic in Judgment under uncertainty: Heuristics and biases (eds. Kahneman, D. & Tversky, A. ) 201–208 (Cambridge University Press, 1982).
Evans, J. St. B. T. Hypothetical thinking: Dual processes in reasoning and judgment (Psychology Press, 2007).
Boorman, E. D., Behrens, T. E., Woolrich, M. W. & Rushworth, M. F. How green is the grass on the other side? Frontopolar cortex and the evidence in favor of alternative courses of action. Neuron 62, 733–743 (2009).
Crescentini, C. et al. Mechanisms of rule acquisition and rule following in inductive reasoning. J. Neurosci. 31, 7763–7774 (2011).
Domenech, P. & Koechlin, E. Executive control and decision-making in the prefrontal cortex. Curr. Opin. Behav. Sci. 1, 101–106 (2015).
Gajewski, P. D., Hengstler, J. G., Golka, K., Falkenstein, M. & Beste, C. The Met-allele of the BDNF Val66Met polymorphism enhances task switching in elderly. Neurobiol. Aging 32, 2327–e7 (2011).
Karayanidis, F. et al. Advance preparation in task-switching: converging evidence from behavioral, brain activation and model-based approaches. Front. Psychol. 1, 25 (2010).
PubMed PubMed Central Google Scholar
Kopp, B. & Lange, F. Electrophysiological indicators of surprise and entropy in dynamic task-switching environments. Front. Human Neurosci. 7, 300 (2013).
Kopp, B., Lange, F., Howe, J. & Wessel, K. Age-related changes in neural recruitment for cognitive control. Brain Cognition 85, 209–219 (2014).
Lange, F. et al. Impaired Set-Shifting in Amyotrophic Lateral Sclerosis: An Event-Related Potential Study of Executive Function. Neuropsychology, advance online publication (2015).
Nicholson, R., Karayanidis, F., Bumak, E., Poboka, D. & Michie, P. T. ERPs dissociate the effects of switching task-sets and task cues. Brain Res. 1095, 107–123 (2006).
Karayanidis, F. & Jamadar, S. ERP measures of proactive and reactive control processes in task-switching paradigms in Task Switching and Cognitive Control (eds Grange, J. & Houghton, G. ) 200–236 (Oxford University Press, 2014).
Nessler, D., Friedman, D. & Johnson, R. A new account of the effect of probability on task switching: ERP evidence following the manipulation of switch probability, cue informativeness and predictability. Biol. Psychol. 91, 245–262 (2012).
Berg, E. A. A simple objective technique for measuring flexibility in thinking. J. Gen. Psychol. 39, 15–22 (1948).
Grant, D. A. & Berg, E. A behavioral analysis of degree of reinforcement and ease of shifting to new responses in a Weigl-type card-sorting problem. J. Exp. Psychol. 38, 404–411 (1948).
Heaton, R. K., Chelune, G. J., Talley, J. L., Kay, G. G. & Curtiss, G. Wisconsin Card Sorting Test Manual–Revised and Expanded (Psychological Assessment Resources, 1993).
Barceló, F. & Knight, R. T. Both random and perseverative errors underlie WCST deficits in prefrontal patients. Neuropsychologia 40, 349–356 (2002).
Lange, F., Seer, C., Finke, M., Dengler, R. & Kopp, B. Dual routes to cortical orienting responses: Novelty detection and uncertainty reduction. Biol. Psychol. 105, 66–71 (2015).
Barceló, F. The Madrid card sorting test (MCST): a task switching paradigm to study executive attention with event-related potentials. Brain Res. Protoc. 11, 27–37 (2003).
Nelson, H. E. A modified card sorting test sensitive to frontal lobe defects. Cortex 12, 313–324 (1976).
Mayr, U. & Kliegl, R. Differential effects of cue changes and task changes on task-set selection costs. J. Exp. Psychol. Learn. 29, 362–372 (2003).
Mayr, U. & Keele, S. W. Changing internal constraints on action: the role of backward inhibition. J. Exp. Psychol. Gen. 129, 4–26 (2000).
Forstmann, B. U., Brass, M. & Koch, I. Methodological and empirical issues when dissociating cue-related from task-related processes in the explicit task-cuing procedure. Psychol. Res. 71, 393–400 (2007).
West, R., Langley, M. M. & Bailey, K. Signaling a switch: neural correlates of task switching guided by task cues and transition cues. Psychophysiology 48, 612–623 (2011).
Altmann, E. M. Advance Preparation in Task Switching: What Work Is Being Done? Psychol. Sci. 15, 616–622 (2004).
Steiger, J. H. Beyond the F test: Effect size confidence intervals and tests of close fit in the analysis of variance and contrast analysis. Psychol. Methods 9, 164–182 (2004).
Groppe, D. M., Makeig, S. & Kutas, M. Independent component analysis of event-related potentials. Cognitive Sci. Online 6, 1–44 (2008).
Google Scholar
Kopp, B., Tabeling, S., Moschner, C. & Wessel, K. Fractionating the neural mechanisms of cognitive control. J. Cognitive Neurosci. 18, 949–965 (2006).
Allport, A., Styles, E. A. & Hsieh, S. Shifting international set: Exploring the dynamic control of tasks in Attention and performance XV (eds Umilta, C. & Moscovitch, M. ) 421–452 (MIT Press, 1994).
De Baene, W. & Brass, M. Dissociating strategy-dependent and independent components in task preparation. Neuropsychologia 62, 331–340 (2014).
Cowan, N. in Models of working memory (eds Miyake, A. & Shah, P. ) Ch. 3, 62–101 (Cambridge University Press, 1999).
Oberauer, K. Access to information in working memory: exploring the focus of attention. J. Exp. Psychol. Learn. 28, 411–421 (2002).
Koechlin, E. An evolutionary computational theory of prefrontal executive function in decision-making. Phil. Trans. R. Soc. B, 369, 20130474 (2014).
Download references
Acknowledgements
F. Lange and D. Müller received funding from the German National Academic Foundation. The authors thank Alexander Steinke for helping with data preparation.
Author information
Authors and affiliations.
Department of Neurology, Hannover Medical School, Hannover, Germany
Florian Lange, Caroline Seer, Dorothea Müller & Bruno Kopp
You can also search for this author in PubMed Google Scholar
Contributions
F.L. and B.K. developed the study concept. All authors contributed to the study design. Testing and data collection were performed by F.L., D.M. and C.S., F.L. and B.K. performed the data analysis and interpretation. F.L. drafted the manuscript and C.S. and B.K. provided critical revisions. All authors approved the final version of the manuscript for submission.
Ethics declarations
Competing interests.
The authors declare no competing financial interests.
Rights and permissions
This work is licensed under a Creative Commons Attribution 4.0 International License. The images or other third party material in this article are included in the article’s Creative Commons license, unless indicated otherwise in the credit line; if the material is not included under the Creative Commons license, users will need to obtain permission from the license holder to reproduce the material. To view a copy of this license, visit http://creativecommons.org/licenses/by/4.0/
Reprints and permissions
About this article
Cite this article.
Lange, F., Seer, C., Müller, D. et al. Cognitive caching promotes flexibility in task switching: evidence from event-related potentials. Sci Rep 5 , 17502 (2015). https://doi.org/10.1038/srep17502
Download citation
Received : 25 August 2015
Accepted : 30 October 2015
Published : 08 December 2015
DOI : https://doi.org/10.1038/srep17502
Share this article
Anyone you share the following link with will be able to read this content:
Sorry, a shareable link is not currently available for this article.
Provided by the Springer Nature SharedIt content-sharing initiative
This article is cited by
Cognitive flexibility and n2/p3 event-related brain potentials.
- Alexander Steinke
- Antonino Visalli
Scientific Reports (2020)
Stimulus- and response-based interference contributes to the costs of switching between cognitive tasks
- Florian Lange
Psychological Research (2020)
Fast Neural Dynamics of Proactive Cognitive Control in a Task-Switching Analogue of the Wisconsin Card Sorting Test
- Gema Díaz-Blancat
- Juan García-Prieto
- Francisco Barceló
Brain Topography (2018)
By submitting a comment you agree to abide by our Terms and Community Guidelines . If you find something abusive or that does not comply with our terms or guidelines please flag it as inappropriate.
Quick links
- Explore articles by subject
- Guide to authors
- Editorial policies
Sign up for the Nature Briefing newsletter — what matters in science, free to your inbox daily.

- Print this article
Research Article
How task set and task switching modulate perceptual processes: is recognition of facial emotion an exception.
- Heike Elchlepp
- Stephen Monsell
- Stephen Monsell , Department of Psychology, University of Exeter, United Kingdom ORCID
- Aureliu Lavric , Department of Psychology, University of Exeter, United Kingdom ORCID
In Part 1 we review task-switching and other studies showing that, even with time for preparation, participants’ ability to shift attention to a relevant attribute or object before the stimulus onset is limited: there is a ‘residual cost’. In particular, several brain potential markers of perceptual encoding are delayed on task-switch trials, compared to task-repeat trials that require attention to the same attribute as before. Such effects have been documented even for a process often considered ‘automatic’ – visual word recognition: ERP markers of word frequency and word/nonword status are (1) delayed when the word recognition task follows a judgement of a perceptual property compared to repeating the lexical task, and (2) strongly attenuated during the perceptual judgements. Thus, even lexical access seems influenced by the task/attentional set.
In Part 2, we report in detail a demonstration of what seems to be a special case, where task-set and a task switch have no such effect on perceptual encoding. Participants saw an outline letter superimposed on a face expressing neutral or negative emotion, and were auditorily cued to categorise the letter as vowel/consonant, or the face as emotional/neutral. ERPs exhibited a robust emotional-neutral difference (Emotional Expression Effect) no smaller or later when switching to the face task than when repeating it; in the first half of its time-course it did not vary with the task at all. The initial encoding of the valence of a fixated facial emotional expression appears to be involuntary and invariant, whatever the endogenous task/attentional set.
- Page/Article: 36
- DOI: 10.5334/joc.179
- Accepted on 30 Jun 2021
- Published on 5 Aug 2021
- Peer Reviewed
Defining task-set reconfiguration: The case of reference point switching
- Brief Reports
- Published: February 2007
- Volume 14 , pages 118–125, ( 2007 )
Cite this article
- Darryl W. Schneider 1 &
- Gordon D. Logan 1
1223 Accesses
25 Citations
Explore all metrics
Some researchers argue that task switching involves task-set reconfiguration-that is, changing the existing task set to perform a different task. Although this idea is intriguing, it is often unclear what is reconfigured and which processes are involved in reconfiguration. We addressed these issues by defining task sets, identifying differences between them, and obtaining evidence that was diagnostic of reconfiguration. In two experiments, subjects performed relative judgment tasks that involved comparing a target with a reference point that repeated or switched across trials. The task was the same on every trial, but the task set was not, because a task-set parameter-the reference point-had to be changed. Target-reference point distance effects that changed with the reference point provided diagnostic evidence that reconfiguration occurred, and this reconfiguration led to switch costs. We discuss how our approach can be generalized to define reconfiguration more clearly in other task-switching situations. nt|mis|This research was supported by Grants BCS 0133202 and BCS 0446806 from the National Science Foundation to G.D.L.
Article PDF
Download to read the full article text
Avoid common mistakes on your manuscript.
Allport, A. , & Wylie, G. (2000). Task switching, stimulus-response bindings, and negative priming. In S. Monsell & J. Driver (Eds.), Control of cognitive processes: Attention and performance XVIII (pp. 35–70). Cambridge, MA: MIT Press.
Google Scholar
Arrington, C. M. , & Logan, G. D. (2004). Episodic and semantic components of the compound-stimulus strategy in the explicit taskcuing procedure. Memory & Cognition , 32 , 965–978.
Article Google Scholar
Banks, W. P. (1977). Encoding and processing of symbolic information in comparative judgments. In G. H. Bower (Ed.), The psychology of learning and motivation: Advances in research and theory (Vol. 11, pp. 101–159). New York: Academic Press.
Buckley, P. B. , & Gillman, C. B. (1974). Comparisons of digits and dot patterns. Journal of Experimental Psychology , 103 , 1131–1136.
Article PubMed Google Scholar
Dehaene, S. (1989). The psychophysics of numerical comparison: A reexamination of apparently incompatible data. Perception & Psychophysics , 45 , 557–566.
Fias, W. , & Fischer, M. H. (2005). Spatial representation of numbers. In J. I. D. Campbell (Ed.), Handbook of mathematical cognition (pp. 43–54). New York: Psychology Press.
Holyoak, K. J. (1978). Comparative judgments with numerical reference points. Cognitive Psychology , 10 , 203–243.
Link, S. W. (1975). The relative judgment theory of two choice response time. Journal of Mathematical Psychology , 12 , 114–135.
Logan, G. D. (1988). Toward an instance theory of automatization. Psychological Review , 95 , 492–527.
Logan, G. D. (2005). The time it takes to switch attention. Psychonomic Bulletin & Review , 12 , 647–653.
Logan, G. D. , & Bundesen, C. (2003). Clever homunculus: Is there an endogenous act of control in the explicit task-cuing procedure? Journal of Experimental Psychology: Human Perception & Performance , 29 , 575–599.
Logan, G. D. , & Gordon, R. D. (2001). Executive control of visual attention in dual-task situations. Psychological Review , 108 , 393–434.
Mayr, U. , & Kliegl, R. (2003). Differential effects of cue changes and task changes on task-set selection costs. Journal of Experimental Psychology: Learning, Memory, & Cognition , 29 , 362–372.
Meiran, N. (1996). Reconfiguration of processing mode prior to task performance. Journal of Experimental Psychology: Learning, Memory, & Cognition , 22 , 1423–1442.
Monsell, S. (2003). Task switching. Trends in Cognitive Sciences , 7 , 134–140.
Monsell, S. , & Mizon, G. A. (2006). Can the task-cuing paradigm mea sure an endogenous task-set reconfiguration process? Journal of Experimental Psychology: Human Perception & Performance , 32 , 493–516.
Moyer, R. S. , & Dumais, S. T. (1978). Mental comparison. In G. H. Bower (Ed.), The psychology of learning and motivation: Advances in research and theory (Vol. 12, pp. 117–155). New York: Academic Press.
Poltrock, S. E. (1989). A random walk model of digit comparison. Journal of Mathematical Psychology , 33 , 131–162.
Rogers, R. D. , & Monsell, S. (1995). Costs of a predictable switch between simple cognitive tasks. Journal of Experimental Psychology: General , 124 , 207–231.
Schneider, D. W. , & Logan, G. D. (2005). Modeling task switching without switching tasks: A short-term priming account of explicitly cued performance. Journal of Experimental Psychology: General , 134 , 343–367.
Waszak, F., Hommel, B. , & Allport, A. (2003). Task-switching and long-term priming: Role of episodic stimulus-task bindings in taskshift costs. Cognitive Psychology , 46 , 361–413.
Download references
Author information
Authors and affiliations.
Department of Psychology, Vanderbilt University, 37203, Nashville, TN
Darryl W. Schneider & Gordon D. Logan
You can also search for this author in PubMed Google Scholar
Corresponding author
Correspondence to Darryl W. Schneider .
Rights and permissions
Reprints and permissions
About this article
Schneider, D.W., Logan, G.D. Defining task-set reconfiguration: The case of reference point switching. Psychonomic Bulletin & Review 14 , 118–125 (2007). https://doi.org/10.3758/BF03194038
Download citation
Received : 28 October 2005
Accepted : 25 April 2006
Issue Date : February 2007
DOI : https://doi.org/10.3758/BF03194038
Share this article
Anyone you share the following link with will be able to read this content:
Sorry, a shareable link is not currently available for this article.
Provided by the Springer Nature SharedIt content-sharing initiative
- Reference Point
- Switch Cost
- Response Selection
- Negative Priming
- Memory Retrieval
- Find a journal
- Publish with us
- Track your research

An official website of the United States government
The .gov means it’s official. Federal government websites often end in .gov or .mil. Before sharing sensitive information, make sure you’re on a federal government site.
The site is secure. The https:// ensures that you are connecting to the official website and that any information you provide is encrypted and transmitted securely.
- Publications
- Account settings
Preview improvements coming to the PMC website in October 2024. Learn More or Try it out now .
- Advanced Search
- Journal List
- HHS Author Manuscripts

Is Set Shifting Really Impaired in Trait Anxiety? Only When Switching Away from an Effortfully Established Task Set
The current study investigated whether trait anxiety was systematically related to task-set shifting performance, using a task-switching paradigm in which one task was more attentionally demanding than the other. Specifically, taking advantage of a well-established phenomenon known as asymmetric switch costs, we tested the hypothesis that the association between trait anxiety and task-set shifting is most clearly observed when individuals must switch away from a more attentionally demanding task for which it was necessary to effortfully establish an appropriate task set. Ninety-one young adults completed an asymmetric switching task and trait-level mood questionnaires. Results indicated that higher levels of trait anxiety were systematically associated with greater asymmetry in reaction-time (RT) switch costs. Specifically, the RT costs for switching from the more attentionally demanding task to the less demanding task were significantly greater with higher levels of trait anxiety, whereas the RT costs for switching in the opposite direction were not significantly associated with trait anxiety levels. Further analyses indicated that these associations were not attributable to comorbid dysphoria or worry. These results suggest that levels of trait anxiety may not be related to general set-shifting ability per se, but, rather, that anxiety-specific effects may primarily be restricted to when one must efficiently switch away from (or let go of) an effortfully established task set.
People vary in the extent to which they experience anxiety during everyday situations. These individual differences in trait anxiety, even at the subclinical level, have been suggested to be predictive of performance on various cognitive measures, including executive functions (EFs), defined here as a set of domain-general control processes that regulate one’s thought and action ( Miyake et al., 2000 ). The current study focuses on one of the frequently studied EFs, task-set shifting (or simply shifting), and examines how it is related to levels of trait anxiety (i.e., the tendency to be anxious in general but not necessarily anxious in the moment). Specifically, in light of some limited but suggestive existing evidence briefly reviewed below, the current study proposes and tests the hypothesis that, in emotionally neutral (nonthreatening) situations, trait anxiety is related to shifting performance only under certain circumstances, namely, when one must switch away from an effortfully established task set.
Trait Anxiety and Task-Set Shifting: Prior Research and A New Hypothesis
The starting point for the current study was a theoretical claim on task-set shifting made by Attentional Control Theory (ACT), a leading theory on the relationship between subclinical levels of anxiety and EFs ( Eysenck, Derakshan, Santos, & Calvo, 2007 ). A major aim of ACT is to specify the relationship between anxiety and EF abilities, using Miyake et al.’s (2000) framework on EFs ( Eysenck et al., 2007 ). This framework (for an update, see Friedman & Miyake, in press ; Miyake & Friedman, 2012 ) focuses on three separable yet intercorrelated EFs: the updating of working memory representations (updating), the inhibition of prepotent or dominant responses (inhibition), and the efficient switching between multiple mental sets or tasks (shifting). 1 ACT claims that updating is generally spared in anxiety unless the situation is emotionally threatening, but that anxious individuals perform worse on the other two EFs — inhibition and shifting — whether the situation is threatening or neutral ( Eysenck et al., 2007 ). Specifically, with respect to shifting ability, ACT proposes that “anxiety impairs processing efficiency (and often performance effectiveness) on tasks involving the shifting function” ( Eysenck et al., 2007 , p. 346). In other words, ACT hypothesizes that individuals with higher levels of anxiety will perform more slowly (i.e., reduced processing efficiency) than those with lower levels of anxiety on set-shifting tasks and that this negative impact of anxiety may sometimes manifest itself as higher error rates as well (i.e., reduced performance effectiveness). In the current study, we evaluated this claim.
Given that ACT assumes that trait anxiety and situational stress jointly influence an individual’s performance on EF tasks ( Eysenck et al., 2007 ), its claim on the anxiety–shifting relationship is based on research using both state and trait measures of anxiety. Moreover, ACT’s scope encompasses not only emotionally neutral but also emotionally threatening situations. In this study, however, we restrict our scope by focusing on the associations between trait anxiety and shifting under situations involving only emotionally neutral stimuli. Despite this restricted scope, the current study is still highly relevant to the evaluation of ACT’s claim on set shifting, because it assumes trait anxiety as an important source of influence on shifting performance and also because unlike its claim on updating, ACT’s claim on the negative effect of anxiety on shifting performance does not exclude emotionally neutral situations ( Eysenck et al., 2007 ).
Although ACT’s theoretical claim on the effect of anxiety on shifting has remained essentially the same since its original conception (for more recent reviews, see Berggren & Derakshan, 2013 ; Eysenck & Derakshan, 2011 ), we see a strong need for further investigating the relationship between trait anxiety and shifting. This is primarily because the existing evidence for the negative impact of trait anxiety on shifting performance in emotionally neutral situations is highly limited and is mostly restricted to neuropsychological measures on set shifting. 2 In particular, even though more than 20 years have passed since the initial development of task-switching paradigms ( Allport, Styles, & Hsieh, 1994 ; Rogers & Monsell, 1995 ), little research on the EF correlates of anxiety has taken advantage of this experimental paradigm popular in cognitive psychology (for comprehensive reviews on the task switching literature, see Kiesel et al., 2010 ; Vandierendonck, Liefooghe, & Verbruggen, 2010 ). Moreover, the limited amount of evidence currently available on the relationship between trait anxiety and set-shifting performance is at best mixed, as we briefly review below. Thus, it is not only timely but also important to revisit ACT’s claim on shifting, especially using the task-switching paradigm.
Prior Evidence on the Relationship Between Trait Anxiety and Set Shifting
There is limited evidence that trait anxiety is associated with shifting in emotionally neutral situations. In fact, the only consistent evidence for the claim that higher trait anxiety is associated with impaired shifting performance comes from studies using the Wisconsin Cart Sorting Task (WCST). The WCST is a popular neuropsychological EF task, often associated with set shifting (e.g., Miyake et al., 2000 ), in which participants sort a deck of cards based on one of a few rules (e.g., number of objects on the card), but must avoid perseverating on old rules when, unbeknownst to them, these sorting rules are changed. Two studies have showed that individuals with higher levels of trait anxiety perform worse on measure of performance effectiveness, including total errors ( Casselli, Reiman, Hentz, Osborne, & Alexander, 2004 ), perseverative errors ( Caselli, et al., 2004 ), or the failure to maintain sets ( Gershuny & Sher, 1995 ). More recently, another study ( Edwards, Edwards, & Lyvers, 2015 ) examined performance on the WCST using a measure of processing efficiency (based on the reaction time [RT] on perseverative errors). Although their results suggested that the association between trait anxiety and WCST efficiency may be specific to certain situations (i.e., when mental effort was high, or when mental effort was low but levels of situational stress were high), performance was impaired in trait anxiety across most task conditions (the only situation that did not show an association with anxiety was when both mental effort and situational stress were low). Together, these WCST studies suggest that individuals with high levels of trait anxiety struggle more than their nonanxious counterparts in this set-shifting task, which necessitates overcoming a prepotent task representation that is abruptly changed (e.g., previously correct sorting rules).
In contrast, there is little evidence that anxiety is associated with shifting performance for another popular neuropsychological measure of set-shifting, the Trail Making Test (TMT). In the TMT, baseline trials in which participants sequentially trace only numbers (e.g., 1–25) or only alphabet letters (e.g., A-Z) are compared with key switching trials in which participants must switch back and forth between two equally practiced tracing rules (e.g., switching between numbers and letters, as in 1-A-2-B-3-C...). Thus, this task requires switching between two categories of stimuli (numbers and letters), but, unlike WCST, it does not implicate any overcoming of a prepotent task representation. To the best of our knowledge, the only TMT study that included a direct measure of trait anxiety, the State-Trait Anxiety Inventory (STAI), failed to show any clear association between trait anxiety and performance on the alternating trials ( Waldstein, Ryan, Jennings, Muldoon, & Manuck, 1997 ). Other work has suggested ( Orem, Petrac, & Bedwell, 2008 ) that perceived stress is associated with performance on the key switching trials, but this study did not directly assess trait anxiety, and the association with stress was not significant when switch costs (i.e., the difference between baseline and alternating trials) were examined. Thus, the existing studies using the TMT fail to provide much evidence for a robust relationship between trait anxiety and shifting ability.
Moreover, there is little evidence that trait anxiety is associated with shifting ability when assessed with a typical task-switching paradigm. The only published task-switching study ( Bunce, Handley, & Gaines, 2008 ) we know of that used only emotionally neutral stimuli (i.e., switching between color and shape judgments) did not find a significant correlation between trait anxiety and switching performance ( r = −.05), even though they used a large sample ( N = 300). Because the sample in this study included a wide age range of participants (18 to 85 years) and the main dependent measure was the switch-trial RTs (rather than RT switch costs), this null result must be interpreted with caution. Nevertheless, the results from the Bunce et al. (2008) study suggest that the evidence for shifting impairment in trait anxiety is weak when the task-switching paradigm uses only emotionally neutral stimuli and requires switching between two tasks that are equivalent in their attentional control demand.
A New Hypothesis
The prior evidence we just reviewed seems inconsistent with the hypothesis that trait anxiety is associated with across-the-board shifting impairments. We propose, however, that these prior results are consistent with an alternative hypothesis: The relationship between trait anxiety and shifting performance is most robust when there exists some asymmetry in prepotency or attentional control demand in the task setup, thus necessitating people to effortfully establish a mental set to avoid making errors on attentionally demanding trials. Once a new mental set is effortfully established to deal with attentional control demands, it might be more difficult for people to get out of that mental set to switch to a new mental set. Thus, when anxious individuals spend extra effort to establish task sets, such effort may ironically make it more difficult for them to switch away from those tasks (as in the WCST). When there is no such asymmetry in attentional control demands, however, the relationship between trait anxiety and shifting performance may be considerably weaker or even nonexistent (as in the TMT or symmetric task switching). The current study tested this alternative hypothesis about the relationship between trait anxiety and shifting by taking advantage of a phenomenon known as asymmetric switch costs.
Asymmetric Switching Paradigm
Asymmetric switch costs are a well-established phenomenon in the task switching literature (e.g., Allport et al., 1994 ; Bryck & Mayr, 2008 ; Meuter & Allport, 1999 ; Wylie & Allport, 2000 , Yeung & Monsell, 2003 ). Asymmetric switch costs are observed when the tasks that one needs to switch between differ in their levels of prepotency or attentional control demands. What is interesting about asymmetric switch costs is that the directionality of the effect is counterintuitive: Switch costs are greater for the switch from the more effortful and less prepotent task to the less attentionally demanding and more prepotent task than for the switch in the opposite direction. For example, if one uses Stroop-type stimuli (e.g., RED printed in blue ink), switching to the easier, more prepotent task (word reading) yields greater switch costs than switching to the more attentionally demanding task (color naming).
To clarify this pattern of asymmetric switch costs, we provide a graphic illustration of some hypothetical yet typical results in Figure 1 . As displayed in the graph, overall RTs are longer for the more difficult, attentionally demanding task than for the easier, more prepotent task. However, the switch cost itself (defined as switch-trial RTs minus repeat-trial RTs) is larger for the easier task (i.e., when switching away from the more attentionally demanding task) than for the more difficult task (i.e., when switching away from the easier task). Although the precise cognitive mechanism underlying such an asymmetry in switch costs is still debated ( Bryck & Mayr, 2008 ; Schneider & Anderson, 2010 ; Yeung & Monsell, 2003 ), one prevalent explanation for this asymmetric switch cost is that it reflects people’s difficulty getting out of (or letting go of) the more effortfully established task set (e.g., Wylie & Allport, 2000 ).

A visual illustration of a typical pattern of asymmetric switch costs. Even though RTs are generally slower on the more effortful task, switch cost RTs (i.e., Switch trial RT – repeat trial RT) are larger for the less effortful task.
This explanation of the asymmetric switch costs provides a novel, theoretically motivated way to investigate the anxiety–shifting relationship especially in light of another major component of ACT, the processing efficiency hypothesis ( Eysenck et al., 2007 ). This hypothesis states that anxiety primarily affects processing efficiency (e.g., RTs) rather than performance effectiveness (e.g., accuracy), and that “anxious individuals compensate for the adverse effects of anxiety on processing efficiency [...] by increased effort and use of resources ” ( Eysenck et al., 2007 , p. 341, emphasis added). Thus, according to this hypothesis, anxious individuals must exert extra effort to establish the task set for the more attentionally demanding task to achieve high levels of accuracy. If so, then switching away from that attentionally demanding task should be particularly challenging from them, thus resulting in even larger switch costs than those observed for nonanxious individuals. In contrast, switching in the opposite direction might not reveal much impact of trait anxiety because performing the more prepotent task does not require effortful establishment of the task set, even for individuals with high trait anxiety.
The Current Study
In the current study, we used an asymmetric switching paradigm to test the hypothesis that trait anxiety would be associated with shifting performance primarily when switching away from an effortfully established task set. In this paradigm, displayed in Figure 2 , participants switched between two tasks: (a) a less attentionally demanding task of identifying which side of the screen a simple arrow stimulus (<<<<<) is presented on, and (b) a more attentionally demanding task of identifying the direction that this stimulus points (left or right). Shortly (750 ms) before the presentation of the target stimulus, participants were instructed on which task to perform on that trial with an external cue (a plus sign “+” or an asterisk “*”). Consistent with the previous experimental results, we expected to find larger switch costs when participants switched from the more attentionally demanding direction task to the more prepotent location task.

Example trials of the switching task in the mixed blocks. Trial 2 represents a repeat trial because the same task was cued on Trial 1 (i.e., location judgment), and Trial 3 represents a switch trial because it cued a switch to the direction judgment. These ‘Repeat Trial’ and ‘Switch Trial’ labels were not actually presented on the screen.
To further examine the effect of effort and attentional demand on switch costs, we introduced a secondary manipulation to this paradigm: After performing four blocks of the switching task using a particular cue-task mapping rule (+ for the location task and * for the direction task), participants were asked to reverse the mapping rule in the remaining two blocks (* for the location task and + for the direction task). Because this cue reversal requires participants to abandon the previously learned and practiced mapping rule, we hypothesized that this manipulation would exaggerate the magnitudes of the asymmetric switch costs.
We made the following two predictions in this study. First, we predicted that larger switch costs (especially RT switch costs) for switching from the more effortful task (the direction task) to the less effortful task (the location task) would be exaggerated among individuals with higher levels of trait anxiety, whereas the switch costs for switching in the opposite direction would not be systematically related to trait-anxiety levels. Second, we also predicted that such an asymmetry in the switch costs exhibited by individuals with higher levels of trait anxiety would become even more exaggerated after the cue-mapping reversal.
We also addressed some additional questions in this study. First, levels of dysphoria (more generally, depression) are substantially correlated not only with levels of anxiety ( Ehring & Watkins, 2008 ; Mineka, Watson, & Clark, 1998 ) but also with broad impairments in EFs ( Snyder, 2013 ; Snyder, Miyake, & Hankin, 2015 ). Thus, we included a measure of dysphoria in an effort to distinguish the associations between trait anxiety and asymmetric switch costs from those between trait dysphoria and asymmetric switch costs (for examples of recent research using this type of analysis, see Edwards et al., 2015a , 2015b ; Gustavson & Miyake, 2016 ). Second, anxiety is not a unitary construct, and one of its subcomponents often discussed in the literature is worry ( Eysenck et al., 2007 ). Because some research has shown that it is the worry component of anxiety that is associated with variation in some EF abilities such as working memory updating (e.g., Gustavson & Miyake, 2016 ), we also tested the extent to which worry could explain the predicted association between trait anxiety and asymmetric switch costs.
Participants
Ninety-one undergraduate students (36 men and 55 women) completed this study for partial course credit. An additional 14 participants completed this study, but their data were excluded from the analyses because they performed at or below chance level on one of the initial pure blocks (performing just the location or direction task without any switching requirement), suggesting that they might have misunderstood the task instructions. 3
Materials and Procedure
Participants first performed a computerized measure of asymmetric task-switching, programmed in PsyScope 1.2.5 ( Cohen, MacWhinney, Flatt, & Provost, 1993 ). RTs were recorded with a ms-accurate button box (ioLab Systems). After this computerized task, participants completed several mood-related questionnaires. 4 We administered the questionnaires after task switching to avoid potential induction of anxiety and dysphoria due to their responses to a series of emotionally charged questions.
Task-switching paradigm
Figure 2 displays some example trials of the asymmetric task-switching paradigm used in this study. On each trial, a cue (+ or *) appeared in the middle of the screen for 750 ms, indicating the dimension (location or direction) of the arrow stimulus (>>>>> or <<<<<) to which participants should attend. The + cue meant to respond with the location of the arrow, and the * cue meant to respond with the arrow’s direction. This cue-to-stimulus interval of 750 ms was chosen because prior research has established that it typically takes over 500–600 ms to process the cue and prepare for the forthcoming stimulus as much as possible (e.g., Rogers & Monsell, 1995 ). Immediately after the cue was removed, arrow stimuli were presented on either the left or right side of the screen. After each response, the next cue was presented on the screen with no delay. To minimize the number of trials in which participants could respond correctly regardless of whether they were performing the correct task, the locations and directions of arrows were incongruent on 75% of trials (e.g., if the stimulus was presented on the right side of the screen, it pointed left 75% of the time).
Initially, participants performed the location task alone, and then the direction task alone (72 trials each). These pure blocks were introduced before the mixed blocks to make sure that participants had a complete understanding and mastery of the task requirement and stimulus-response mapping rules. Next, four mixed blocks containing both tasks were performed (112 trials per block, excluding 4 warm-up trials at the beginning of each block that we did not analyze). Mixed block trials were pseudorandomly ordered so that after switching tasks, participants would repeat the same task for 0–3 trials. For each task within these mixed blocks, participants switched after completing either 0-repeat trials (12 times per block), 1-repeat trial (8 times per block), 2-repeat trials (4 times per block), or 3-repeat trials (4 times per block). Therefore, in sum, each block contained 28 switch trials, 16 1-repeat, 8 2-repeat, and 4 3-repeat trials per task (56 for the location task and 56 for the direction task).
Finally, participants performed two more mixed blocks (112 trials per block, again excluding 4 initial warm-up trials) with the reverse cue-to-task mapping, so that + cued the direction task and * cued the location task. After the completion of Block 4, participants were told about this cue-mapping reversal and asked to complete the next two blocks (Blocks 5–6) with this new rule. Although participants completed 6 mixed blocks in total (4 before the cue reversal and 2 afterward), analyses were performed collapsing across pairs of blocks (Blocks 1–2 vs. 3–4 vs. 5–6) so that we could analyze practice effects on trials before the cue reversal (Blocks 1–2 vs. 3–4) and the direct effect of the reversal itself (Blocks 3–4 vs. 5–6).
Questionnaires
Levels of trait anxiety and dysphoria were measured with the Beck Anxiety Inventory (BAI; Beck, Epstein, Brown, & Steer, 1988 ) and the Beck Depression Inventory (BDI; Beck & Steer, 1987 ), respectively. Both questionnaires had 21-items and were scored with a 4-point rating scale per question. Although the most frequent measure of trait anxiety in this literature is the STAI ( Ansari, Derakshan, & Richards, 2008 ; Derakshan, Smyth, & Eysenck, 2009 ), we chose the BAI instead because there is evidence that the BAI may be more effective than the STAI at differentiating anxiety from depressive symptoms ( Bieling, Antony, & Swinson, 1998 ; Caci, Bayle, Dossios, Robert, & Boyer, 2003 ; Creamer, Foran & Bell, 1995 ). Finally, worry was measured with the 16-item Penn State Worry Questionnaire (PSWQ; Meyer, Miller, Metzer, & Borkovec, 1990 ).
Data Trimming and Analyses
RT analyses were based on correct trials with RTs greater than 150 ms. In addition to removing error trials, trials immediately following those error trials were also excluded from analyses, as is typically done in task-switching studies (e.g., Meiran, 1996 ; Meiran, Chorev, & Sapir, 2000 ; Rogers & Monsell, 1995 ). Excluding trials following errors is important because, after an error is made, it is difficult to tell whether the current trial is a switch or repeat trial.
RTs were trimmed using methods recommended by Wilcox and Keselman (2003) , as our research group has typically done with RT-based measures of EF (e.g., Friedman et al., 2008 ; Ito et al., 2015 ), to reduce undue influences from outliers and improve reliability. Specifically, participant RT means excluded RTs outside of 3.32 times the median absolute deviation from each participant’s median by trial type (removing 10.3% of trials). In addition, to minimize undue influences from participants with extreme mean RTs and error rates, we set limits at 3 standard deviations from the group mean for each measure, and outlier participants’ values were replaced with the nearest limit.
Error rate analyses were performed on raw error rates. We also conducted the analysis using arcsine-transformed scores because the error rates were generally low, showing some tendency for the ceiling effect. However, because this transformation did not change the statistical results, the raw data are reported here.
Clinical Measures
Trait anxiety ( M = 12.0, SD = 7.8) and dysphoria levels ( M = 10.6, SD = 8.3) were relatively low in this nonclinical sample, but were moderately correlated with each other, r (89) = .51, p < .001. Although this finding is not surprising, it nonetheless highlights the importance of statistically controlling for dysphoria when assessing the specific association between trait anxiety and task switching performance. Both measures had high reliability (Cronbach’s alpha = .86 for BAI and .89 for BDI). Levels of worry, measured with PSWQ ( M = 49.7, SD = 14.2, Cronbach’s alpha = .73), were also correlated substantially with both trait anxiety, r (89) = .38, p < .001, and dysphoria, r (89) = .52, p < .001.
Although this was a nonclinical sample, the questionnaire responses indicated that some individuals had “severe” levels of anxiety or dysphoria, according to the conventional cutoff values used for the BAI (>25, n = 9) and BDI (>28, n = 4). Because excluding these participants from analysis did not change the overall pattern of results, we report below the analyses based on all 91 participants.
Preliminary Analyses: Basic Experimental Effects
Before presenting the results of the associations between trait anxiety and task switching performance, we first report the basic experimental effects to document the asymmetric effect of switch costs at the overall group level (see Table 1 ). All ANOVAs we conducted included the within-subjects factors of Task (location vs. direction) and Switch (switch vs. repeat trials). Depending on the analyses, we also included Block as another within-subjects factor. We report partial eta-squared (η 2 p ) as our measure of effect sizes. 5
Experimental Effects of the Asymmetric Switching Task
Note : Reaction times, error rates, and switch costs for trials by task (location or direction), switch (switch or repeat), and before and after the cue-to-task mapping reversal.
Because those experimental effects per se were not the main focus of our study and because the patterns were consistent with previous research on asymmetric switch costs, our presentation of the experimental effects in this section focuses only on the asymmetric switch cost effect, as indicated by the Task×Switch interaction (or the Task×Switch×Block interaction). For completeness, however, a summary of the ANOVA results for the experimental effects are presented in the Appendix for both the RT and error data.
Before cue reversal (Blocks 1–4)
We replicated the expected asymmetric switch cost effect for both RTs and error rates. Specifically, the Task×Switch interaction was significant for both the RT data, F (1,90) = 18.74, η 2 p = .17, p < .001, and the error data, F (1, 90) = 49.26, η 2 p = .35, p < .001, indicating that the switch cost was larger for the less attentionally demanding location task than for the more demanding direction task (see Table 1 ). Although there were some practice effects across Blocks 1–4, the magnitudes of the switch-cost asymmetry remained relatively stable across the blocks, as indicated by the lack of a significant Task×Switch×Block interaction (the effect, however, was marginally significant for the RT data).
After cue reversal (Blocks 5–6)
When focusing on blocks after the reversal, the Task×Switch interaction was also significant in both the RT data, F (1, 90) = 19.85, η 2 p = .18, p < .001, and the error data, F (1, 90) = 27.91, η 2 p = .24, p < .001.
Effects of cue reversal (Blocks 3–4 vs. 5–6)
To examine whether asymmetric switch costs increased after the cue reversal, we compared RTs and error rates for the two blocks immediately preceding the reversal (Blocks 3–4) to those after the reversal (Blocks 5–6). As shown in Table 2 and the Appendix , overall switch costs were larger after the reversal (Switch×Block interaction) for both the RT and error data. However, as Table 1 indicates, even though the degree of asymmetry in switch costs seemed greater during the trials following the reversal (Blocks 5–6) compared to those before the reversal (Blocks 3–4), this effect (Task×Switch×Block) was not significant in either the RT or error rate data.
Summary of the Regression Results Involving Trait Anxiety
Note : The interactions between levels of anxiety and the within-subjects ANOVA factors. In all models, levels of dysphoria were also included as continuous predictors. Degrees of freedom for all comparisons were F (1, 88) except when noted ( a ), where degrees of freedom were F (2, 88).
These results demonstrated the robust effects of asymmetric switch costs across all 6 mixed blocks. As expected, the switch costs were larger for the less attentionally demanding location task (i.e., when switching away from the more demanding direction task) than for the more demanding direction task (i.e., when switching away from the less demanding task), even though the direction task was associated with much longer RTs and higher error rates overall. However, although overall switch costs were significantly greater after the cue reversal (Blocks 5–6) than before the reversal (Blocks 3–4), this cue reversal did not significantly magnify the degree of asymmetry in the switch costs between the location and direction tasks.
Primary Analyses: Association Between Trait Anxiety and Asymmetric Switch Costs
After establishing the asymmetric switch cost effect for both RT and error data, we examined whether the magnitudes of such switch cost asymmetry was systematically related to individual differences in trait anxiety, both before and after the cue reversal. To do so, we conducted a series of regression analyses ( Judd, McClelland, & Ryan, 2008 ) in which levels of trait anxiety were allowed to interact with the within-subjects factors of the ANOVAs described above: Task, Switch, and, in some analyses, Block. The regression models also included higher-order interactions between trait anxiety and within-subjects factors (e.g., Task×Switch×Anxiety). In all analyses reported here, the levels of dysphoria were statistically controlled for, following the procedure recommended by Yzerbyt, Muller, and Judd (2004) . We treated all of the individual differences measures in this study (e.g., trait anxiety, dysphoria) as continuous variables to avoid well-known statistical problems associated with the still-prevalent practice of creating dichotomous variables with arbitrary cut-off values such as the median score ( Irwin & McClelland, 2003 ; MacCallum, Zhang, Preacher, & Rucker, 2002 ).
The complete results of these analyses involving trait anxiety are summarized in Table 2 , for both the RT and error data. As shown in Table 2 , however, because levels of trait anxiety were primarily related to RT results, but not to error results, only the analyses with RT data will be discussed in this section. The finding that the correlations with trait anxiety were consistently observed only for RT data (even though the experimental effects of asymmetric switch costs were robustly observed for both RT and error data) is consistent with ACT’s processing efficiency hypothesis, which states that the effects of anxiety are often observed for measures of processing efficiency (RTs) than for performance effectiveness (error rates).
The primary hypothesis concerned the moderating role of trait anxiety in the degree of asymmetry in switch costs for the location and direction tasks. As predicted, for trials before the cue reversal, the degree of asymmetry in switch costs for the two tasks were larger for individuals higher in trait anxiety (Task×Switch×Anxiety), F (1, 88) = 4.97, η 2 p = .05, p = .028 (see Table 2a ). In other words, although trait anxiety was associated with larger overall switch costs (Switch×Anxiety), F (1, 88) = 4.31, η 2 p = .05, p = .041, the association with trait anxiety was greater for the less effortful location task than for the more effortful direction task. Figure 3 illustrates this pattern as regression lines of trait anxiety level versus RT by trial type, for Blocks 1–2 ( Figure 3a ) and Blocks 3–4 ( Figure 3b ). In these figures, switch costs can be seen by the difference between the solid and dotted lines for each task (i.e., switch vs. repeat trials), and this difference is considerably larger for the location task lines than for the direction task lines (i.e., the asymmetric switch costs). Importantly, at high levels of anxiety, this asymmetry in switch costs was much more pronounced than at low levels of anxiety.

Anxiety effects with RTs by task and by switch, both before (A and B) and after (C) the cue-to-task mapping reversal. Levels of trait anxiety were associated with larger asymmetric switch costs. Namely, location task lines diverge substantially (i.e., exhibiting larger switch costs) for those with high anxiety, but diverge at a much smaller degree for the direction task, even for those high in trait anxiety. Dysphoria (as measured by the BDI) was controlled for in all linear models.
To confirm that these results were driven by location-task trials (i.e., when switching away from the more attentionally demanding direction task), we analyzed the location-task and direction-task trials separately. On location-task trials only, higher trait anxiety was associated with significantly larger switch costs, collapsed across Blocks 1–4, F (1, 88) = 4.41, η 2 p = .05, p = .039. In contrast, levels of anxiety were not associated with switch costs for the direction-task trials, again collapsed across Blocks 1–4, F (1, 88) = .41, η 2 p = .01, p = .524. Therefore, the RT switch costs for the location task primarily accounted for the increased asymmetry in switch costs among individuals with higher levels of trait anxiety. Not surprisingly given these results, the overall association between trait anxiety and RTs was significant, F (1, 88) = 7.89, η 2 p = .08, p = .006, and the association with anxiety was larger for the location task than for the direction task (Task×Anxiety), F (1, 88) = 6.77, η 2 p = .07, p = .011. Finally, there was no evidence that the associations with trait anxiety changed over time (i.e., no interactions involving both Anxiety and Block were significant, all F s(1, 88) < .86, η 2 p < .01, p s > .355).
Next, we examined the results for trait anxiety on only those blocks following the reversal (Blocks 5–6). As shown in Figure 3c and Table 2b , the asymmetric switch cost associated with anxiety remained statistically significant after the cue reversal (Task×Switch×Anxiety), F (1, 88) = 6.91, η 2 p = .07, p = .010. Levels of trait anxiety were again related to larger switch costs (Switch×Anxiety), F (1, 88) = 10.38, η 2 p = .07, p = .002, and worse performance on the location task compared to the direction task (Task×Switch), F (1, 88) = 5.47, η 2 p = .06, p = .022.
Finally, we compared the associations with trait anxiety before the reversal (Blocks 3–4) to those after the reversal (Blocks 5–6) to examine whether the cue reversal magnified these asymmetric effects associated with anxiety ( Table 2c ). There was no evidence that the associations between trait anxiety and asymmetric switch costs were larger after the reversal (Task×Switch×Block×Anxiety), F (1, 88) = 1.03, η 2 p = .01, p = .314. There was also no strong evidence that the association between trait anxiety and overall switch cost was larger after the reversal, although this difference was marginally significant (Switch×Block×Anxiety), F (1, 88) = 3.48, η 2 p = .04, p = .066. These findings suggest that, although the cue reversal manipulation demonstrated the experimental effect of increased switch costs, it did not robustly moderate the associations observed for trait anxiety.
As predicted, individuals with higher trait anxiety tended to have greater levels of asymmetry in switch costs. More specifically, participants generally experienced greater difficulty switching to the location task than to the direction task, but this tendency was exaggerated for people with higher levels of trait anxiety. Furthermore, although there was some suggestion that the associations between trait anxiety and RT switch costs were larger after the cue reversal, this difference was not statistically significant.
These results from the primary analyses suggest that trait anxiety is not universally related to switching performance, but, rather, that the relationship between trait anxiety and switching may be observed only under some circumstances (i.e., when there is a clear asymmetry in effort expended between the two tasks). Moreover, these results are consistent with the proposal that anxious individuals exerted more effort in establishing the more attentionally demanding task set for the direction task, hence later experiencing greater difficulty when they need to switch away from that task to perform an easier, more automatic location task.
Secondary Analyses: Associations Between Dysphoria/Worry and Asymmetric Switch Costs
In all regression models reported for our primary analyses, we statistically controlled for the level of dysphoria by allowing dysphoria levels to interact with the within-subjects factors in the same way as trait anxiety ( Yzerbyt et al., 2004 ). In this section, we first report the results for the main and interaction effects involving the dysphoria variable in the above models, controlling for levels of trait anxiety. We then describe the results of additional analyses conducted to examine the extent to which the results that we reported above for trait anxiety could be accounted for by the worry component of anxiety.
Dysphoria was not systematically related to switching performance for the RT data. Specifically, unlike levels of trait anxiety, levels of dysphoria did not interact with any of the within-subjects experimental variables, before, after, or across the reversal, all F s(1, 88) < 2.62, η 2 p < .03, p s > .108. Levels of dysphoria were not associated with overall RTs in any of these models, either (i.e., no main effects of dysphoria), all F s(1, 88) < .06, η 2 p < .01, p s > .814.
The error data showed the same pattern of results, with one exception: a significant Task×Dysphoria interaction was obtained on some blocks, indicating that participants with higher dysphoria made more errors on the more effortful direction task than on the less effortful location task, F (1, 88) = 7.61, η 2 p = .08, p = .007, for Blocks 5–6 only, F (1, 88) = 8.79, η 2 p = .09, p = .004, for Blocks 3–4 vs. 5–6 (Task×Dysphoria×Block). These results suggest that the associations observed for dysphoria may be more pronounced for performance effectiveness for attentionally demanding tasks, rather than the efficiency of task switching per se. Importantly, these results also suggest that the key associations reported for our primary analyses (significant Task×Switch×Anxiety interactions) are specific to trait anxiety and cannot be attributed to a substantial amount of variance shared between anxiety and dysphoria.
We also examined the potential role of worry by adding levels of worry to the regression models (with anxiety and dysphoria above) as another continuous predictor that could interact with the experimental factors. As was the case with the analyses with dysphoria, worry was essentially unrelated to performance on the asymmetric switching task. For both RT and error data, worry did not generally interact with any experimental factors, all F s(1, 87) < 2.57, η 2 p < .03, p s > .111, for RT data, all F s(1, 87) < 2.67, η 2 p < .03, p s > .105, for error data. Although there were two exceptions (both observed for the RT data), 6 these results depended on the inclusion of the trait anxiety variable in the model. In other words, neither of these associations remained significant if anxiety was removed from the model. Thus, it is not clear whether these results reflect real associations with worry or are possibly spurious findings (e.g., due to the large number of statistical tests performed). Important to note, in these analyses involving worry, all of the significant results for trait anxiety reported for our primary analyses above remained statistically significant, and no associations that were not statistically significant before became significant after the addition of worry in the model. Thus, the systematic relationship reported above for trait anxiety cannot be accounted for by the worry component of trait anxiety.
The purpose of this study was to test the hypothesis that trait anxiety is related to shifting performance in emotionally neutral contexts primarily when individuals must switch away from an effortfully established task set. To test this new hypothesis, we used an asymmetric task switching paradigm in which participants switched between two tasks differing in their attentional demands. Replicating the basic asymmetric switching effect, we demonstrated that both RT and error switch costs were larger for the less attentionally demanding location task than for the more demanding direction task. More important, in line with our hypothesis, we found that individuals with higher trait anxiety exhibited a greater asymmetry in their RT switch costs (but not error switch costs) than those with lower trait anxiety. These results support the proposal that individuals with higher trait anxiety have difficulty switching away from more effortfully established task sets, but do not necessarily have general difficulty with switching per se. Furthermore, we also found that these results were specific to trait anxiety and unrelated to variance shared between anxiety and dysphoria or a subcomponent of trait anxiety, worry.
Although our primary hypothesis was confirmed, we did not observe strong evidence for our secondary hypothesis that the relationship between trait anxiety and the degree of asymmetry in switch costs would become even stronger after the cue-mapping reversal. There was some indication that the associations between trait anxiety and RT switch costs were larger after the cue reversal, but the cue-mapping reversal neither significantly amplified the asymmetric switching effect at the experimental level nor moderated the strength of the association between anxiety and switch costs in the regression analyses. In hindsight, this finding makes sense, considering that reversing the cue-mapping rules was a one-time change introduced between Blocks 4 and 5 and did not involve constant changes in task rules. This cue-mapping reversal was likely challenging to participants right after its introduction (i.e., at the beginning of Block 5), but, once this rule change was mentally established shortly thereafter, no such further reversal was required. Thus, these results suggest that the effects of trait anxiety on shifting may be strongest immediately or shortly after when individuals must switch away from effortfully established task sets, but that such effects may not persist across many trials in the absence of constant shifting requirements.
In the rest of this section, we discuss how these results (a) reconcile the existing evidence on trait anxiety and shifting, (b) inform other work on anxiety and shifting more broadly, and (c) can be used to refine ACT and extend it in new directions.
Specifying the Relationship Between Trait Anxiety and Shifting
On the basis of the limited prior evidence regarding the relationship between trait anxiety and shifting in emotionally neutral situations, we proposed a new hypothesis that the negative effect of trait anxiety on shifting performance may be observed primarily when individuals must switch away from an effortfully established task set. As noted in the introduction, this hypothesis provides a coherent explanation of the seemingly mixed previous evidence on trait anxiety and shifting performance. Specifically, existing evidence suggests that an association between trait anxiety and shifting can be observed for the WCST, a task that requires participants to effortfully build a task representation and then quickly override it, but not for the TMT and symmetric switching, which require constant switching between two task representations relatively equivalent in attentional demands (e.g., counting 1–9 vs. A-Z in the TMT, making color vs. shape judgments in symmetric task switching). These prior results are consistent with the alternative hypothesis on the anxiety–shifting relationship tested and supported in the current study.
It is important to acknowledge, however, that two existing studies that examined switch costs in a task-switching paradigm may potentially challenge our hypothesis ( Ansari et al., 2008 ; Ansari & Derakshan, 2011 ). Specifically, in these studies, participants switched, on the basis of a visual cue, between trials in which they needed to quickly attend to a stimulus on the same side of the screen (prosaccade) or on the opposite side of the screen (antisaccade). In both studies, RT switch costs were larger for the high-anxiety group (STAI scores > 44 or 45) compared to the low-anxiety group (STAI scores < 34 or 38), but only for the more attentionally demanding antisaccade task. In other words, the effect of trait anxiety was observed not when participants were switching away from the more attentionally demanding task but, rather, when they were switching back to that attentionally demanding task.
Upon close examination, however, it is unclear whether the results from these two studies directly contradict our hypothesis ( Ansari et al., 2008 ; Ansari & Derakshan, 2011 ). This is primarily because the patterns of switch costs in these studies deviate, in some important ways, from typical findings based on the asymmetric switching paradigm (i.e., their data did not show the widely replication pattern illustrated in Figure 1 ). First, in the mixed prosaccade/antisaccade blocks, there was actually a switching RT benefit, rather than a cost, when switching to the more attentionally demanding task. Second, high error rates for the antisaccade task (as high as 25–30% on switch trials in the mixed blocks) indicate that participants in the Ansari and Derakshan (2011) study likely failed to consistently establish the more attentionally demanding task set before switching, 7 which we consider vital to our suggestion that the anxiety–shifting relationship is most robust when one is switching away from the more demanding task.Together, these considerations suggest that, even though Ansari and colleagues used an asymmetric switching paradigm, these two prior studies might have primarily tapped participants’ inhibition performance, rather than shifting performance, especially given that the antisaccade task is a commonly used measure of response inhibition (e.g., Miyake et al., 2000 ).
Extending the results to other aspects of shifting
Although we investigated the relationship between trait anxiety and shifting performance using the asymmetric switching paradigm, asymmetric switch costs are only one of the well-established phenomena in the task-switching literature. In fact, various other task-switching phenomena demonstrated in the literature (for reviews, see Kiesel et al., 2010 ; Vandierendonck et al., 2010 ) can be used to examine which aspects or components of switching may be affected in trait anxiety.
It is possible, for example, to examine whether the associations with trait anxiety may extend to repeat trials in the mixed blocks as well by looking at another commonly used dependent measure in task-switching studies known as the mixing cost. Unlike switch costs, the mixing costs compare performance on repeat trials in baseline (or pure) blocks consisting of only repeat trials to performance on repeat trials in mixed blocks (i.e., when participants are also switching). If levels of trait anxiety are systematically related to the magnitudes of mixing costs, such an association would suggest that trait anxiety may be related to one’s ability to minimize the negative influence from the other task set that should not be currently active. Indeed, follow-up analyses in our own RT data suggested that, like the effects for switch costs, trait anxiety was associated with larger mixing costs for location-task trials, and not for direction-task trials. 8 Future research could also capitalize on manipulating preparation time (cue-stimulus intervals), given that the time participants have to prepare for a given switch may affect the efficiency of disengaging from a previous task set and establishing a new one.
Shifting Impairments in Different Aspects of Anxiety
A secondary goal of this study was to examine which aspects of trait anxiety were primarily associated with shifting in the context of this asymmetric switching paradigm. As has been suggested by recent studies that focused on working memory updating ( Gustavson & Miyake, 2016 ; Stout, Shackman, Johnson, & Larson, 2015 ), the association between anxiety and shifting observed in the current study could primarily reflected the worry subcomponent of trait anxiety. In fact, ACT has suggested that worry is responsible for the association between anxiety and processing efficiency (and sometimes also performance effectiveness), at least with regard to state worry ( Eysenck, et al., 2007 ). The results of the current study, however, do not reveal any systematic relations between trait levels of worry and asymmetric switch costs, suggesting that the worry component of anxiety could not account for the association between anxiety and shifting described here. These results, in turn, suggest that other components of trait anxiety assessed with the BAI (e.g., anxious arousal, fear) may be able to better predict shifting performance, although this speculative influence must be tested more directly in future studies.
Although the current study focused on trait anxiety, there is some evidence suggesting that our hypothesis may extend to the relationship between state anxiety and shifting as well. Specifically, Derakshan et al. (2009) observed an association between state anxiety and RT switch costs when switching between multiplication and division tasks, but not when switching between addition and subtraction tasks. Derakshan et al. (2009) explain these findings as an effect of task complexity (i.e., multiplication and division require complex mental operations than additional and subtraction), but an alternative interpretation is that these results were driven by the large asymmetry in the difficulty between the multiplication and division tasks (RT switch costs were not reported separately, but the multiplication-task RTs were about 5,000 ms larger than the division-task RTs in baseline blocks, whereas addition and subtraction RTs were comparable, ~3,000 ms each). Thus, although task complexity may have also contributed, these results are also consistent with the idea that RT switch costs in anxiety are most pronounced when there is a clear asymmetry in task demands.
Theoretical Implications for ACT
The results of the current study have some important theoretical implications for ACT, even though the scope of this study was restricted to the association between trait anxiety and task switching in neutral contexts. First, our results were only partially consistent with ACT’s original claim on the anxiety-shifting relationship ( Eysenck et al., 2007 ). Specifically, our results were consistent with ACT’s claim that the effects of anxiety are most salient in terms of processing efficiency, rather than performance effectiveness. In particular, at the overall group level, both the RT and error data demonstrated the predicted asymmetry in switch costs, but only the RT data revealed a significant relationship between levels of trait anxiety and the degree of asymmetry in switch costs between the location and direction tasks. These results, in turn, are consistent with our explanation that, to achieve the same degree of performance effectiveness (i.e., accuracy), individuals with high trait anxiety must exert stronger attentional control (i.e., more effort) to perform well on the more attentionally demanding task. As a result, rather ironically, individuals with high levels of trait anxiety find it more difficult to get out of (or let go of) that task set, hence demonstrating a greater asymmetry in RT switch costs than those with low levels of trait anxiety.
Our results, however, are not consistent with ACT’s claim that shifting performance is generally impaired in anxiety, even in emotionally neutral contexts. Instead, our results suggest that the association between anxiety (or at least trait anxiety) and shifting performance may be restricted primarily to the process of switching away from effortfully established task sets. Thus, the results from the current study invite closer reexaminations of the anxiety–shifting relationship. Our alternative hypothesis is more specific and more precise than ACT’s original hypothesis on shifting, is clearly testable, and could potentially be generalized to the effect of state anxiety. Thus, we believe that our alternative hypothesis help guide future theoretically motivated investigations on the anxiety–shifting relationship.
More generally, it may also be time for the other EF-related hypotheses of ACT to be reexamined and, if necessary, revised. Since its initial conception ( Eysenck et al., 2007 ), ACT has provided simple, clearly stated hypotheses regarding anxiety and different EF components (i.e., shifting and inhibition abilities are impaired in anxiety, but updating ability is not, unless tested under threatening situations). In light of newer evidence, however, it is important to evaluate and potentially refine these general theoretical claims. First, given that inhibition is a multifaceted construct ( Nigg, 2000 ; Friedman & Miyake, 2004 ), it is possible that some aspects of inhibitory control abilities may be more or less strongly associated with anxiety than others. Second, although ACT has claimed that updating ability is generally spared in anxiety, a recent meta-analysis involving 177 studies (total N = 22,061) found a significant association between anxiety and working memory capacity ( Moran, 2016 ). Moreover, recent research has also found that levels of trait anxiety may be systematically related to the processing efficiency of updating performance when RT measures were used ( Gustavson & Miyake, 2016 ). Finally, although different facets of EFs (e.g., inhibition, updating, and shifting) have been known to be substantially correlated (e.g., Miyake et al., 2000 ), ACT has so far emphasized the separability of EFs. Given that a recent review of the clinical evidence ( Snyder et al., 2015 ) points to a potentially important role in psychopathology of general EF ability (i.e., what is common to different EF components; Friedman & Miyake, in press ; Miyake & Friedman, 2012 ), it will be useful to examine how anxiety is related to general EF ability. As these possible future directions indicate, refining and rigorously testing the current ACT hypotheses will help substantially advance our understanding of the anxiety–EF relationship.
Acknowledgments
This research was supported by Grant MH016880 from the National Institutes of Health.
We thank Vicky Grunberg, Bess Reynolds, Janelle Kramer, Auburn Meisner, and Anna Zelinskaya for data collection.
Basic Experimental Effects on the Asymmetric Switching Task
Note: Degrees of freedom for all comparisons were F (1, 90) except when noted ( a ), where degrees of freedom were F (2, 90).
1 Throughout this article, we use the terms shifting and switching in different ways. We use the term (task-set) shifting to refer to a type of EF postulated in the theoretical framework proposed by Miyake et al. (2000) and later adopted by Eysenck et al. (2007) . In contrast, we use the term switching (or task switching ) in a narrower sense, referring to a class of experimental paradigms used in cognitive psychology, in which participants must switch back and forth between two (or more) tasks, often on the basis of external cues.
2 Although the original ACT article ( Eysenck et al., 2007 ) cited the results from prospective memory studies as evidence for shifting impairments in anxiety ( Cockburn & Smith, 1994 ; Harris & Cumming, 2003 ; Harris & Menzies, 1999 ), we will not review such studies in this article because the extent to which prospective memory tasks selectively implicate set-shifting ability is unclear. Similarly, we will also not discuss here two recent studies that examined the relationship between trait anxiety and shifting using a dual-task paradigm ( Edwards, Moore, Champion, & Edwards, 2015 ; Johnson & Gronlund, 2009 ), because there is some evidence that dual-tasking ability might be separable from shifting ability (e.g., Alzahabi & Becker, 2013 ; Miyake et al., 2000 ).
3 A close examination of the actual responses made during the initial pure block indicated that, despite the explicit instructions, almost all of these participants performed the wrong task (the direction task) in the first pure block (all participants were supposed to perform the location task in the first pure block, as noted in the procedure section). Given that performing simple judgments during the initial pure blocks is fairly easy, it is not clear why a substantial number of participants made such an error, though we speculate that it may have to do with the ambiguous (bivalent) nature of the arrow stimuli. Because we cannot ensure that these participants fully understood the task requirements or paid close attention to the initial instructions, we excluded their data from analyses.
4 To fully disclose all the individual differences measures administered in the current study, we note here that, besides the measures described in the Method section, all participants completed two additional tasks (a White Bear thought suppression task and a goal-neglect Stroop task) and four other questionnaires (the Habit Index of Negative Thinking, the Five Factor Mindfulness Questionnaire, the Ruminative Responses Scale, and the Inventory of Depression and Anxiety Symptoms). These additional measures were administered as part of a separate study on the relationship between shifting and trait tendencies to experience repetitive negative thoughts.
5 A recent tutorial review of effect sizes ( Richardson, 2011 ) provides some rough guidelines as to how to interpret the magnitudes of effect sizes expressed as η 2 p on the basis of an initial proposal from Cohen (1969) : .01 as a small effect, .06 as a medium effect, and .14 as a large effect ( Richardson, 2011 , p. 142).
6 First, in blocks before the reversal only (Blocks 1–2 & 3–4), worry was associated with smaller overall switch costs (Switch x Worry), F (1, 87) = 4.04, η 2 p = .04, p = .048. Second, in the same model comparing Blocks 1–2 and 3–4, there was some suggestion that levels of worry were associated with greater RT reduction for the direction task than for the location task (Task x Block x Worry), F (1, 88) = 7.16, η 2 p = .08, p = .009.
7 Further consistent with this interpretation, the Ansari and Derakshan (2011) study found that these effects were specific to situations with short cue-stimulus intervals, namely, when participants did not have enough time to interpret the cue and effortfully engage in advance task-set configuration. In contrast, we used a cue-stimulus interval of 750 ms in this study to allow for active establishment of task sets.
8 These analyses were based on mixing cost RTs, operationalized as the difference between repeat-trial RTs in mixed blocks and repeat-trial RTs in the baseline pure block, computed separately for blocks 1–2, 3–4, and 5–6. Significantly larger mixing costs in high anxiety were observed for the location task across all blocks, all F s(1, 88) > 4.46, η 2 p > .04, p s < .039, but not for the direction task, all F s(1, 88) < 1.62, η 2 p < .02, p s > .206 (all analyses statistically controlled for levels of dysphoria).
- Allport A, Styles EA, Hsieh S. Shifting intentional set: Exploring the dynamic control of tasks. In: Umilta C, Moscovitch M, editors. Attention and performance XV. Cambridge, MA: MIT Press; 1994. pp. 421–452. [ Google Scholar ]
- Alzahabi R, Becker MW. The association between media multitasking, task-switching, and dual-tasking. Journal of Experimental Psychology: Human Perception and Performance. 2013; 39 :1485–1495. [ PubMed ] [ Google Scholar ]
- Ansari TL, Derakshan N, Richards A. Effects of anxiety on task switching: evidence from the mixed antisaccade task. Cognitive, Affective & Behavioral Neuroscience. 2008; 8 :229–238. [ PubMed ] [ Google Scholar ]
- Ansari TL, Derakshan N. The neural correlates of cognitive effort in anxiety: Effects on processing efficiency. Biological Psychology. 2011; 86 :337–348. [ PubMed ] [ Google Scholar ]
- Berggren N, Derakshan N. Attentional control deficits in trait anxiety: Why you see them and why you don’t. Biological Psychology. 2013; 92 :440–446. [ PubMed ] [ Google Scholar ]
- Beck A, Epstien N, Brown G, Steer R. An inventory for measuring clinical anxiety: Psychometric properties. Journal of Consulting and Clinical Psychology. 1988; 56 :893–897. [ PubMed ] [ Google Scholar ]
- Beck AT, Steer RA. Manual for the Revised Beck Depression Inventory. San Antonio, TX: Psychological Corp; 1987. [ Google Scholar ]
- Bieling PJ, Antony MM, Swinson RP. The state-trait anxiety inventory, trait version: Structure and content re-examined. Behavior Research and Therapy. 1998; 36 :777–788. [ PubMed ] [ Google Scholar ]
- Bunce D, Handley R, Gaines SO. Depression, anxiety, and within-person variability in adults aged 18 to 85 years. Psychology and Aging. 2008; 23 :848–858. [ PubMed ] [ Google Scholar ]
- Bryck RL, Mayr U. Task selection cost asymmetry without task switching. Psychonomic Bulletin & Review. 2008; 15 :128–134. [ PubMed ] [ Google Scholar ]
- Caci H, Bayle FJ, Dossios C, Robert P, Boyer P. The Spielberger Trait Anxiety Inventory measures more than anxiety. European Psychiatry. 2003; 18 :394–400. [ PubMed ] [ Google Scholar ]
- Caselli RJ, Reiman EM, Hentz JG, Osborne D, Alexander GE. A distinctive interaction between chronic anxiety and problem solving in asymptomatic APOE e4 homozygotes. Journal of Neuropsychiatry and Clinical Neurosciences. 2004; 16 :320–329. [ PubMed ] [ Google Scholar ]
- Cockburn J, Smith PT. Anxiety and errors of prospective memory among elderly people. British Journal of Psychology. 1994; 85 :273–282. [ PubMed ] [ Google Scholar ]
- Cohen J. Statistical power analysis for the behavioral sciences. New York: Academic Press; 1969. [ Google Scholar ]
- Cohen JD, MacWhinney B, Flatt M, Provost J. PsyScope: A new graphic interactive environment for designing psychology experiments. Behavioral Research Methods, Instruments, & Computers. 1993; 25 :257–271. [ Google Scholar ]
- Creamer M, Foran J, Bell R. The Beck Anxiety Inventory in a non-clinical sample. Behaviour Research and Therapy. 1995; 33 :477–485. [ PubMed ] [ Google Scholar ]
- Derakshan N, Smyth S, Eysenck MW. Effects of state anxiety on performance using a task-switching paradigm: An investigation of attentional control theory. Psychonomic Bulletin & Review. 2009; 16 :1112–1117. [ PubMed ] [ Google Scholar ]
- Edwards EJ, Edwards MS, Lyvers M. Cognitive trait anxiety, situational stress, and mental effort predict shifting efficiency: Implications for attentional control theory. Emotion. 2015; 15 :350–359. [ PubMed ] [ Google Scholar ]
- Edwards MS, Moore P, Champion JC, Edwards EJ. Effects of trait anxiety and situational stress on attentional shifting are buffered by working memory capacity. Anxiety, Stress, & Coping. 2015; 28 :1–16. [ PubMed ] [ Google Scholar ]
- Ehring T, Watkins E. Repetitive negative thinking as a transdiagnostic process. International Journal of Cognitive Therapy. 2008; 1 :192–205. [ Google Scholar ]
- Eysenck M, Derakshan N. New perspectives in attentional control theory. Personality and Individual Differences. 2011; 50 :955–960. [ Google Scholar ]
- Eysenck M, Santos R, Derakshan N, Calvo M. Anxiety and cognitive performance: Attentional control theory. Emotion. 2007; 7 :336–353. [ PubMed ] [ Google Scholar ]
- Friedman NP, Miyake A. The relations among inhibition and interference control functions: A latent-variable analysis. Journal of Experimental Psychology: General. 2004; 133 :101–135. [ PubMed ] [ Google Scholar ]
- Friedman NP, Miyake A. Unity and diversity of executive functions: Individual differences as a window of cognitive structure. Cortex. (in press) [ PMC free article ] [ PubMed ] [ Google Scholar ]
- Friedman NP, Miyake A, Young SE, DeFries JC, Corley RP, Hewitt JK. Individual differences in executive functions are almost entirely genetic in origin. Journal of Experimental Psychology: General. 2008; 137 :201–225. [ PMC free article ] [ PubMed ] [ Google Scholar ]
- Gershuny BS, Sher KJ. Compulsive checking and anxiety in a nonclinical sample: Differences in cognition, behavior, personality, and affect. Journal of Psychopathology and Behavioral Assessment. 1995; 17 :19–38. [ Google Scholar ]
- Gustavson DE, Miyake A. Trait worry is associated with difficulties in working memory updating. Cognition and Emotion. 2016 Advance online publication. [ PMC free article ] [ PubMed ] [ Google Scholar ]
- Harris LM, Cumming SR. An examination of the relationship between anxiety and performance on prospective and retrospective memory tasks. Australian Journal of Psychology. 2003; 55 :51–55. [ Google Scholar ]
- Harris LM, Menzies RG. Mood and prospective memory. Memory. 1999; 7 :117–127. [ PubMed ] [ Google Scholar ]
- Irwin JR, McClelland GH. Negative consequences of dichotomizing continuous predictor variables. Journal of Marketing Research. 2003; 40 :366–371. [ Google Scholar ]
- Ito TA, Friedman NP, Bartholow BD, Correll J, Altamirano LJ, Loersch C, Miyake A. Toward a comprehensive model of executive cognitive function in implicit racial bias. Journal of Personality and Social Psychology. 2015; 108 :187–218. [ PMC free article ] [ PubMed ] [ Google Scholar ]
- Johnson DR, Gronlund SD. Individuals lower in working memory capacity are particularly vulnerable to anxiety's disruptive effect on performance. Anxiety, Stress, & Coping. 2009; 22 :201–213. [ PubMed ] [ Google Scholar ]
- Judd C, McClelland G, Ryan C. Data analysis: A model comparison approach. 2nd. New York: Routledge Press; 2008. [ Google Scholar ]
- Kiesel A, Steinhauser M, Wendt M, Falkenstein M, Jost K, Philipp AM, Koch I. Control and interference in task switching—A review. Psychological Bulletin. 2010; 136 :849–874. [ PubMed ] [ Google Scholar ]
- MacCallum RC, Zhang S, Preacher KJ, Rucker DD. On the practice of dichotomization of quantitative variables. Psychological Methods. 2002; 7 :19–40. [ PubMed ] [ Google Scholar ]
- Meiran N. Reconfiguration of processing mode prior to task performance. Journal of Experimental Psychology: Learning, Memory, and Cognition. 1996; 22 :1423–1442. [ Google Scholar ]
- Meiran N, Chorev Z, Sapir A. Component processes in task switching. Cognitive Psychology. 2000; 41 :211–253. [ PubMed ] [ Google Scholar ]
- Meuter RFI, Allport A. Bilingual language switching in naming: Asymmetrical costs of language switching. Journal of Memory and Language. 1999; 40 :25–40. [ Google Scholar ]
- Meyer T, Miller M, Metzger R, Borkovec T. Development and validation of the Penn State Worry Questionnaire. Behavior Research and Therapy. 1990; 28 :487–495. [ PubMed ] [ Google Scholar ]
- Mineka S, Watson D, Clark LA. Comorbidity of anxiety and unipolar mood disorders. Annual Review of Psychology. 1998; 49 :377–412. [ PubMed ] [ Google Scholar ]
- Miyake A, Friedman NP. The nature and organization of individual differences in executive functions: Four general conclusions. Current Directions in Psychological Science. 2012; 21 :8–14. [ PMC free article ] [ PubMed ] [ Google Scholar ]
- Miyake A, Friedman NP, Emerson MJ, Witzki AH, Howerter A, Wager TD. The unity and diversity of executive functions and their contributions to complex “frontal lobe” tasks: A latent variable analysis. Cognitive Psychology. 2000; 41 :49–100. [ PubMed ] [ Google Scholar ]
- Moran TP. Anxiety and working memory capacity: A meta-analysis and narrative review. Psychological Bulletin. 2016 Advance online publication. [ PubMed ] [ Google Scholar ]
- Nigg JT. On inhibition/disinhibition in developmental psychopathology: Views from cognitive and personality psychology and a working inhibition taxonomy. Psychological Bulletin. 2000; 126 :220–246. [ PubMed ] [ Google Scholar ]
- Orem DM, Petrac DC, Bedwell JS. Chronic self-perceived stress and set-shifting performance in undergraduate students. Stress. 2008; 11 :73–78. [ PubMed ] [ Google Scholar ]
- Richardson JTE. Eta squared and partial eta squared as measures of effect size in educational research. Educational Research Review. 2011; 6 :135–147. [ Google Scholar ]
- Rogers RD, Monsell S. Costs of a predictable switch between simple cognitive tasks. Journal of Experimental Psychology: General. 1995; 124 :207–231. [ Google Scholar ]
- Schneider DW, Anderson JR. Asymmetric switch costs as sequential difficulty effects. The Quarterly Journal of Experimental Psychology. 2010; 63 :1873–1894. [ PMC free article ] [ PubMed ] [ Google Scholar ]
- Snyder HR. Major depressive disorder is associated with broad impairments on neuropsychological measure of executive function: A meta-analysis and review. Psychological Bulletin. 2013; 139 :81–132. [ PMC free article ] [ PubMed ] [ Google Scholar ]
- Snyder HR, Miyake A, Hankin BL. Advancing understanding of executive function impairments and psychopathology: Bridging the gap between clinical and cognitive approaches. Frontiers in Psychology. 2015; 6 :328. [ PMC free article ] [ PubMed ] [ Google Scholar ]
- Stout DM, Shackman AJ, Johnson JS, Larson CL. Worry is associated with impaired gating of threat from working memory. Emotion. 2015; 15 :6–11. [ PMC free article ] [ PubMed ] [ Google Scholar ]
- Vandierendonck A, Liefooghe B, Verbruggen F. Task switching: Interplay of reconfiguration and interference control. Psychological Bulletin. 2010; 136 :601–626. [ PubMed ] [ Google Scholar ]
- Waldstein SR, Ryan CM, Jennings JR, Muldoon MF, Manuck SB. Self-reported levels of anxiety do not predict neuropsychological performance in healthy men. Archives of Clinical Neuropsychology. 1997; 12 :567–574. [ PubMed ] [ Google Scholar ]
- Wilcox RR, Keselman HJ. Modern robust data analysis methods: Measures of central tendency. Psychological Methods. 2003; 8 :254–274. [ PubMed ] [ Google Scholar ]
- Wylie G, Allport A. Task switching and the measurement of “switch costs” Psychological Research. 2000; 63 :212–233. [ PubMed ] [ Google Scholar ]
- Yeung N, Monsell S. Switching between tasks of unequal familiarity: The role of stimulus-attribute and response-set selection. Journal of Experimental Psychology: Human Perception and Performance. 2003; 29 :455–469. [ PubMed ] [ Google Scholar ]
- Yzerbyt VY, Muller D, Judd CM. Adjusting researchers’ approach to adjustment: On the use of covariates when testing interactions. Journal of Experimental Social Psychology. 2004; 40 :424–431. [ Google Scholar ]
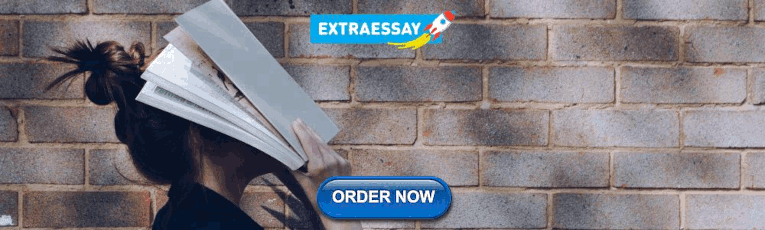
IMAGES
VIDEO
COMMENTS
A task set is a configuration of cognitive processes that is actively maintained for subsequent task performance. Single-unit and brain-imaging studies have identified the neural correlates for task sets in the prefrontal cortex. Here I examine whether the neural data obtained thus far are sufficient to explain the behaviors that have been ...
Task switching (psychology) Task switching, or set-shifting, is an executive function that involves the ability to unconsciously shift attention between one task and another. In contrast, cognitive shifting is a very similar executive function, but it involves conscious (not unconscious) change in attention.
Performing two randomly alternating tasks typically results in higher reaction times (RTs) following a task switch, relative to a task repetition. These task switch costs (TSC) reflect processes of switching between control settings for different tasks. The present study investigated whether task sets operate as a single, integrated representation or as an agglomeration of relatively ...
Psychology. Adaptive behaviour requires the ability to focus on a task and protect it from distraction (cognitive stability) and to rapidly switch tasks when circumstances change (cognitive ...
It is argued here that task switching research—and its associated theorizing—has been hampered by poor definitions of what are tasks and task sets. The chapter provides a distinction between the two: a task is the representation of a set of instructions required to perform an activity accurately; a task set is the set of representations and ...
For task-set reconfiguration to be a useful explanatory construct, it is important to define task sets, identify differ-ences between them, and obtain evidence that is diagnos- ... (Psychology Software Tools, Pittsburgh, PA) operating on computers that registered input from keyboards and displayed the output on monitors. Each experi-
In turn, task switching has become a major research paradigm in experimental psychology and cognitive neuroscience. In the present chapter, we review the research on task switching with an emphasis on behavioral findings and cognitive theories. Readers interested more specifically in the neuroscience research on cognitive control in task ...
Most theories describing the cognitive processes underlying task switching allow for contributions of active task-set reconfiguration and task set inertia. Manipulations of the Cue-to-Stimulus-Interval (CSI) are generally thought to influence task set reconfiguration, while Response-to-Cue (RCI) manipulations are thought to influence task set inertia. Together, these intervals compose the ...
Time-consuming processes of task-set reconfiguration have been shown to contribute to the costs of switching between cognitive tasks. We describe and probe a novel mechanism serving to reduce the ...
Switching between mental sets has been extensively investigated in both experimental and individual differences research using a wide range of task-switch paradigms. However, it is yet unclear whether these different tasks measure a unitary shifting ability or reflect different facets thereof. In this study, 20 task pairs were administered to 119 young adults to assess 5 proposed components of ...
The present study examined the cognitive representation of tasks ("task sets") using the task-switching paradigm. To do so, we manipulated the task-set components "judgment" (i. e., stimulus categories) and "response modality" orthogonally in two-componential switching experiments. In Experiment 1, we additionally manipulated the type of cues, whereas we manipulated the cue-stimulus interval ...
Task sets have been argued to play an important role in cognition, giving rise to the notions of needing to switch between active task sets and to control competing task sets in selective attention tasks. For example, it has been argued that Stroop interference results from two categories of conflict: informational and task (set) conflict. Informational conflict arises from processing the word ...
In Part 1 we review task-switching and other studies showing that, even with time for preparation, participants' ability to shift attention to a relevant attribute or object before the stimulus onset is limited: there is a 'residual cost'. In particular, several brain potential markers of perceptual encoding are delayed on task-switch trials, compared to task-repeat trials that require ...
We report a novel approach for assessing task-set inertia in a memory experiment using event-related potentials (ERPs). After a study phase, participants completed a test block in which they switched between a memory task (retrieving information from the study phase) and a perceptual task. These tasks alternated every two trials.
The task was the same on every trial, but the task set was not, because a task-set parameter-the reference point-had to be changed. ... In G. H. Bower (Ed.),The psychology of learning and motivation: Advances in research and theory (Vol. 11, pp. 101-159). New York: Academic Press. Google Scholar Buckley, P. B., & Gillman, C. B. (1974 ...
Different contexts in daily life often require varying levels of cognitive flexibility. Previous research has shown that people adapt their level of flexibility to match changing contextual demands for task switching in cued-switching paradigms that vary the proportion of switch trials within lists of trials. Specifically, the behavioral costs of switching as opposed to repeating tasks scale ...
Trait Anxiety and Task-Set Shifting: Prior Research and A New Hypothesis. The starting point for the current study was a theoretical claim on task-set shifting made by Attentional Control Theory (ACT), a leading theory on the relationship between subclinical levels of anxiety and EFs (Eysenck, Derakshan, Santos, & Calvo, 2007).
Testing the hypothesis of a close relationship between the intentional component of task-set switching and long-term memory (LTM) retrieval and results were consistent with the hypothesis that the intentional switch-cost component reflects the time demands of retrieving appropriate task rules from LTM. The authors tested the hypothesis of a close relationship between the intentional component ...
The authors tested the hypothesis of a close relationship between the intentional component of task-set switching ("advance reconfiguration;" R. D. Rogers & S. Monsell, 1995) and long-term memory (LTM) retrieval. Consistent with this hypothesis, switch costs are reported to be larger when the switched-to task involves high retrieval demands (i.e., retrieval of episodic information) than when ...