- Email Alert
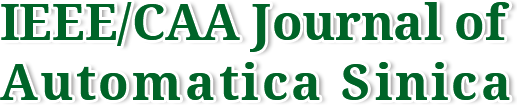
论文 全文 图 表 新闻
- Abstracting/Indexing
- Journal Metrics
- Current Editorial Board
- Early Career Advisory Board
- Previous Editor-in-Chief
- Past Issues
- Current Issue
- Special Issues
- Early Access
- Online Submission
- Information for Authors
- Share facebook twitter google linkedin
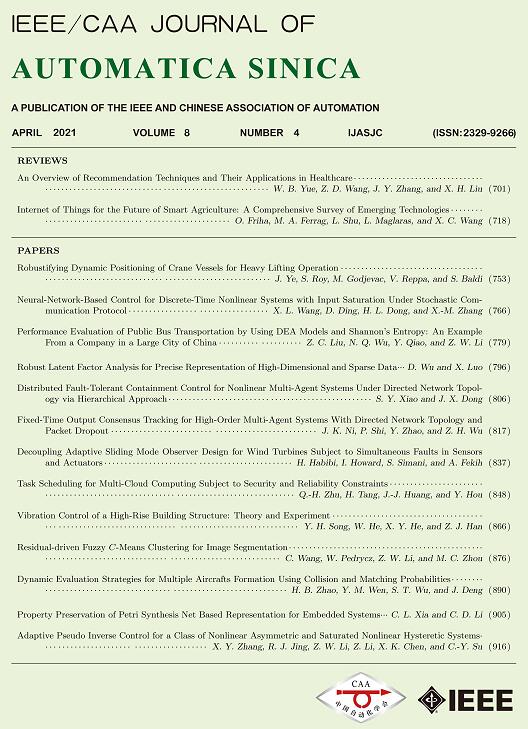
IEEE/CAA Journal of Automatica Sinica
- JCR Impact Factor: 11.8 , Top 4% (SCI Q1) CiteScore: 17.6 , Top 3% (Q1) Google Scholar h5-index: 77, TOP 5
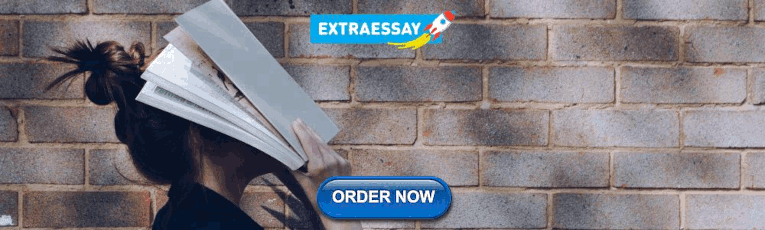
Internet of Things for the Future of Smart Agriculture: A Comprehensive Survey of Emerging Technologies
Doi: 10.1109/jas.2021.1003925.
- Othmane Friha 1 , ,
- Mohamed Amine Ferrag 2 , ,
- Lei Shu 3, 4 , , ,
- Leandros Maglaras 5 , ,
- Xiaochan Wang 6 ,
Networks and Systems Laboratory, University of Badji Mokhtar-Annaba, Annaba 23000, Algeria
Department of Computer Science, Guelma University, Gulema 24000, Algeria
College of Engineering, Nanjing Agricultural University, Nanjing 210095, China
School of Engineering, University of Lincoln, Lincoln LN67TS, UK
School of Computer Science and Informatics, De Montfort University, Leicester LE1 9BH, UK
Department of Electrical Engineering, Nanjing Agricultural University, Nanjing 210095, China
Othmane Friha received the master degree in computer science from Badji Mokhtar-Annaba University, Algeria, in 2018. He is currently working toward the Ph.D. degree in the University of Badji Mokhtar-Annaba, Algeria. His current research interests include network and computer security, internet of things (IoT), and applied cryptography
Mohamed Amine Ferrag received the bachelor degree (June, 2008), master degree (June, 2010), Ph.D. degree (June, 2014), HDR degree (April, 2019) from Badji Mokhtar-Annaba University, Algeria, all in computer science. Since October 2014, he is a Senior Lecturer at the Department of Computer Science, Guelma University, Algeria. Since July 2019, he is a Visiting Senior Researcher, NAULincoln Joint Research Center of Intelligent Engineering, Nanjing Agricultural University. His research interests include wireless network security, network coding security, and applied cryptography. He is featured in Stanford University’s list of the world’s Top 2% Scientists for the year 2019. He has been conducting several research projects with international collaborations on these topics. He has published more than 60 papers in international journals and conferences in the above areas. Some of his research findings are published in top-cited journals, such as the IEEE Communications Surveys and Tutorials , IEEE Internet of Things Journal , IEEE Transactions on Engineering Management , IEEE Access , Journal of Information Security and Applications (Elsevier), Transactions on Emerging Telecommunications Technologies (Wiley), Telecommunication Systems (Springer), International Journal of Communication Systems (Wiley), Sustainable Cities and Society (Elsevier), Security and Communication Networks (Wiley), and Journal of Network and Computer Applications (Elsevier). He has participated in many international conferences worldwide, and has been granted short-term research visitor internships to many renowned universities including, De Montfort University, UK, and Istanbul Technical University, Turkey. He is currently serving on various editorial positions such as Editorial Board Member in Journals (Indexed SCI and Scopus) such as, IET Networks and International Journal of Internet Technology and Secured Transactions (Inderscience Publishers)
Lei Shu (M’07–SM’15) received the B.S. degree in computer science from South Central University for Nationalities in 2002, and the M.S. degree in computer engineering from Kyung Hee University, South Korea, in 2005, and the Ph.D. degree from the Digital Enterprise Research Institute, National University of Ireland, Ireland, in 2010. Until 2012, he was a Specially Assigned Researcher with the Department of Multimedia Engineering, Graduate School of Information Science and Technology, Osaka University, Japan. He is currently a Distinguished Professor with Nanjing Agricultural University and a Lincoln Professor with the University of Lincoln, U.K. He is also the Director of the NAU-Lincoln Joint Research Center of Intelligent Engineering. He has published over 400 papers in related conferences, journals, and books in the areas of sensor networks and internet of things (IoT). His current H-index is 54 and i10-index is 197 in Google Scholar Citation. His current research interests include wireless sensor networks and IoT. He has also served as a TPC Member for more than 150 conferences, such as ICDCS, DCOSS, MASS, ICC, GLOBECOM, ICCCN, WCNC, and ISCC. He was a Recipient of the 2014 Top Level Talents in Sailing Plan of Guangdong Province, China, the 2015 Outstanding Young Professor of Guangdong Province, and the GLOBECOM 2010, ICC 2013, ComManTel 2014, WICON 2016, SigTelCom 2017 Best Paper Awards, the 2017 and 2018 IEEE Systems Journal Best Paper Awards, the 2017 Journal of Network and Computer Applications Best Research Paper Award, and the Outstanding Associate Editor Award of 2017, and the 2018 IEEE ACCESS. He has also served over 50 various Co-Chair for international conferences/workshops, such as IWCMC, ICC, ISCC, ICNC, Chinacom, especially the Symposium Co-Chair for IWCMC 2012, ICC 2012, the General Co-Chair for Chinacom 2014, Qshine 2015, Collaboratecom 2017, DependSys 2018, and SCI 2019, the TPC Chair for InisCom 2015, NCCA 2015, WICON 2016, NCCA 2016, Chinacom 2017, InisCom 2017, WMNC 2017, and NCCA 2018
Leandros Maglaras (SM’15) received the B.Sc. degree from Aristotle University of Thessaloniki, Greece, in 1998, M.Sc. in industrial production and management from University of Thessaly in 2004, and M.Sc. and Ph.D. degrees in electrical & computer engineering from University of Volos in 2008 and 2014, respectively. He is the Head of the National Cyber Security Authority of Greece and a Visiting Lecturer in the School of Computer Science and Informatics at the De Montfort University, U.K. He serves on the Editorial Board of several International peer-reviewed journals such as IEEE Access , Wiley Journal on Security & Communication Networks , EAI Transactions on e-Learning and EAI Transactions on Industrial Networks and Intelligent Systems . He is an author of more than 80 papers in scientific magazines and conferences and is a Senior Member of IEEE. His research interests include wireless sensor networks and vehicular ad hoc networks
Xiaochan Wang is currently a Professor in the Department of Electrical Engineering at Nanjing Agricultural University. His main research fields include intelligent equipment for horticulture and intelligent measurement and control. He is an ASABE Member, and the Vice Director of CSAM (Chinese Society for Agricultural Machinery), and also the Senior Member of Chinese Society of Agricultural Engineering. He was awarded the Second Prize of Science and Technology Invention by the Ministry of Education (2016) and the Advanced Worker for Chinese Society of Agricultural Engineering (2012), and he also gotten the “Blue Project” in Jiangsu province young and middle-aged academic leaders (2010)
- Corresponding author: Lei Shu, e-mail: [email protected]
- Revised Date: 2020-11-25
- Accepted Date: 2020-12-30
- Agricultural internet of things (IoT) ,
- internet of things (IoT) ,
- smart agriculture ,
- smart farming ,
- sustainable agriculture
Smart Farming and Sustainable Agriculture Research Paper
Introduction, what is smart farming, smart farming in detail, future scope.
The drive toward efficient yet sustainable agriculture in the contemporary world necessitates the adoption of smart farming. Smart farming refers to a set of solutions that increase agricultural efficiency, mainly through the use of information and communication technologies.
Data acquisition, data evaluation, and precision application allow improving yields while minimizing the losses. The Internet of Things is of particular use in smart farming, as it makes managing the farm’s assets much easier and also allows creating highly automatized solutions. Blockchain is another promising technology since it not only facilitates safe storage and sharing of information but also contributes to creating a democratically organized international farming community. Increased robotization and interoperability are among the solutions that will likely shape smart farming in the foreseeable future. As a result, smart farming has the potential to revolutionize the world’s agriculture in the years and decades to come.
Considering the rapid growth of the Earth’s population, the problem of sustainable agriculture being able to feed the ever-increasing number of people remains an issue of current interest. However, the opportunities for extensive agriculture expansion are mostly exhausted, which means that the future of agricultural cultivation should look for intensive methods. One of the ways to intensify agriculture and make it more effective overall is precision cultivation that tailors agricultural decisions with regard to the site and the type of crops.
Precision cultivation depends to a high degree on the use of digital technologies in agriculture, which is generally defined as smart farming. Smart farming allows for a wide range of options, from robotization and satellite imagery to the Internet of Things and the blockchain technology that increases the efficiency of crop cultivation by optimizing the use of resources. Providing data acquisition, data evaluation, and precision application with the help of the Internet of Things and blockchain, smart farming may impact agriculture all over the world and has immense potential for the future.
In the coming years, one may expect precision cultivation to have a considerable impact on agricultural businesses, large-scale and small-scale organizations alike. Different countries adopt smart farming methods in their agricultural production to address the issues they confront. As of 2016, the country producing the highest number of scholarly publications on smart farming was China, with 31.84 percent of all academic texts in the field (Pivoto et al., 2017). The USA was responsible for 8.94 percent of scholarly publications, South Korea – for 8.38 percent, and Germany and Japan accounted for 6.15 and 5.59 percent, respectively (Pivoto et al., 2017).
Thus, the world’s nations are interested in smart farming regardless of the differences in particular conditions of agricultural cultivation in their respective countries. For instance, the most significant concern for South Korea is maximizing the efficiency of cultivation for its small amount of arable land (Pivoto et al., 2017). India, as a country expected to suffer from water scarcity, is most interested in adopting smart watering systems (Gupta, Mishra, Bogde, & Kulat, 2016). These examples demonstrate that smart farming makes it possible to address numerous and varying problems all over the world.
The term “smart farming” refers to using information and communication technologies (ICT) to enable precision agriculture with greater accuracy and regardless of the field’s size. Balafoutis et al. (2017) define precision agriculture as “the management of spatial and temporal variation in the fields with regard to soil, atmosphere and plants” (p. 22). The idea of precision cultivation is not new, as small farms aim to tailor their efforts for each particular crop in each specific field since time immemorial. However, the old-style precision cultivation relies mostly on the farmer’s personal inspection of the field and draws on individual experience, which makes it inapplicable on a greater scale.
This limitation is why smart farming technologies are immensely important for enabling precision cultivation regardless of scale. Smart farming is, therefore, farming that relies on a set of technological solutions “drawing from research in precision farming, farm management information systems (FMIS) and agricultural automation and robotics” (Balafoutis et al., 2017, p. 23). The variety of technologies that fall under the term allow smart farming to address many different tasks involved in crop cultivation.
Data Acquisition, Data Evaluation, and Precision Application
Data acquisition technologies represent the first type of smart farming technologies that enable increasing agricultural productivity to a considerable extent. This category includes solutions designed to measure and record crop and field characteristics. One of the most common uses of data acquisition technologies is mapping, achieved through either satellite imagery or unmanned aerial vehicles (UAVs) (Tripicchio, Satler, Dabisias, Ruffalsi, & Avizzano, 2015).
Mapping allows the farmer to quickly and relatively assess the patterns that would be much harder to notice if inspecting the fields on foot. Another type of data acquisition is assessing environmental properties, such as the normalized difference vegetation index (NDVI) or soil moisture, which enables the farmer to monitor the state of crops and soils constantly and efficiently (Balafoutis et al., 2017). Apart from that, data acquisition technologies also include global navigation satellite systems (GNSS) used in the smart farming machinery, which is often autonomous (Balafoutis et al., 2017). In general, data acquisition in smart farming allows making more informed decisions with regards to fields, soils, crops, and machines used in agriculture.
Another possible application of smart farming technologies is data evaluation since the data gathered may only be of use if one analyzes it accurately and makes correct conclusions. One of the ways in which technologies can help the farmer in this respect is management zone delineation, which allows defining “parts of the field with common characteristics that can be managed separately” (Balafoutis et al., 2017, p. 45).
Another possible use of smart farming technologies for data evaluation is decision support systems, which, as follows from the name, inform the farmer’s decisions regarding farm management. Some of such solutions offer “comprehensive and scalable analysis, recommendation/visualization, or sharing of crop performance data among farmers, growers, biologists, government, and commercial organizations” (Jayaraman, Yavari, Georgakopoulos, Morshed, & Zaslavsky, 2016, p. 2). Hence, smart farming not only provides a broad range of basic information regarding the fields and crops but also facilitates decision-making based on this information.
Finally, the third aspect of smart farming technologies is a precision application, which refers to the high-accuracy digitized performance of numerous agricultural tasks. For instance, a wireless network, including temperature and moisture sensors, allows implementing precision irrigation that would address the needs of the crops without wasting any water (Viani, 2016). Another example is the variable-rate “tillage and fertilizer application machinery” that enables distributing granulated fertilizer, lime, manure, and pesticides to a precisely needed degree (Nhamo, Chikoye, & Gondwe, 2017, p. 17).
Since, for instance, under-application of lime may cause considerable yield losses, but its over-application leads to problems with specific nutrients, applying it to an accurate degree is immensely important (Balafoutis et al., 2017). Apart from that, the auto-guidance also increases farming efficiency, as it allows the farm machinery “to follow straight lines to reduce overlaps of the tractor and equipment passes” (Balafoutis et al., 2017, p. 58). Therefore, smart farming technologies open the possibilities for highly efficient use of available resources with maximum productivity.
Internet of Things in Smart Farming
One of the particularly notable technological solutions in smart farming is the Internet of Things (IoT). IoT refers to digitized networks of physical objects, each of which possesses a unique identifier. When applied to agriculture, IoT enables real-time monitoring of large numbers of units, which may prove especially useful in animal husbandry. For instance, in Australia, it is mandatory to “affix passive RFID ear tags to their cattle and to report movements between farms to an online national database” (Kamilaris, Gao, Prenafeta-Boldu, & Ali, 2016, p. 442). As a result, IoT allows managing the farm’s assets with greater precision and efficiency, and with a fraction of effort, it would have required otherwise.
However, IoT displays its true potential in smart farming not when applied to animal husbandry, but when used to interconnect the numerous pieces of machinery involved in the cultivation process. It was already mentioned above that smart farming technologies allow addressing a broad range of tasks, from mapping and moisture measurement to watering and variable rate distribution of fertilizer, lime, manure, and pesticides.
Yet for all the advantages offered by the machines performing these tasks, each of them only realizes a fraction of its potential if used independently, as each has to be put to action manually. IoT frameworks, on the other hand, allow integrating the smart farming machinery to create highly automated digitized frameworks sharing and implementing the information in real-time.
For instance, IoT enables combining “data from a fertilizer sprayer on a tractor… with the data obtained from soil moisture sensors” to make automated decisions informed by both (Jayaraman et al. 2016, p. 2). Taken separately, the pieces of smart farming machinery are only tools for solving specialized tasks, but when united via IoT framework, they form a highly effective and automated network addressing the crop needs in real-time.
Blockchain in Smart Farming
As noted above, smart farming is mainly based on ICT, which means it invariably involves data sharing on a grand scale. Jayaraman et al. (2016) point specifically to the “enormous velocity of data” generated, stored, and shared when applying smart farming in practice (p. 3). The necessity to safely operate large amounts of information produces a demand for the corresponding data security measures, and blockchain technology may be of great use in this particular respect. Lin et al. (2017) remind that the weakest link in any data safety system is people whose biases usually constitute “the most important factor affecting objective prioritization” (p. 2).
These biases create a potential for unscrupulous manipulation of smart farming databases. For example, governments may bias large agriculture systems “to maximize inexpensive food supplies from rural agriculture to urban infrastructure, where the majority of elected officials’ constituents reside” (Lin et al., 2017, p. 2). Blockchain technology, on the other hand, distributes database management among multiple actors, thus reducing the likelihood of any system-wide data manipulation (Lin et al., 2017). Therefore, the application of blockchain allows addressing data safety concerns inevitably involved in smart farming.
Yet one should also be aware of another advantage of blockchain technology in smart farming: using it fosters a network of co-dependent actors that functions democratically. As mentioned above, centralized ways of storing and sharing data, such as centralized and stringently regulated networks or even, to some degree, cloud computing enable the manipulation of data (Lin et al., 2017, p. 2).
By eliminating this potential, the networks created through the use of blockchain technology – as opposed to those with central authority governing information sharing and distribution – contributes to community building. Blockchain empowers the users to regulate the information themselves instead of putting their trust in centralized authority, and, as a result, one cannot deny its “contribution to digital democratization” (Lin et al., 2017, p. 9).
More importantly, still, the users themselves recognize this advantage and stress that creating an international community of farmers is an essential outcome of smart farming. One of the farmers interviewed by Carolan (2018) emphasized that sharing information was “about building social networks, building communities” (p. 754). Blockchain technology allows building these communities democratically, which is yet another positive influence on farming.
As a rapidly developing field that answers the essential challenges of its time, smart farming has highly promising perspectives, and one of them lies in the increased robotization of agriculture. Among other robots, UAVs demonstrate the potential to generate the highest income due to their effectiveness in solving their tasks. Employing the UAVs enables the farmers to use their fields with higher efficiency for a better overall outcome.
For instance, it helps in delineating management zones with different soil characteristics, which, in turn, allows decreasing the plowing depth by changing plowing techniques correspondingly (Tripicchio et al., 2015). This is only one example of how increased robotization may make farming more productive and sustainable at the same time – and, considering this, robotization is a priority for smart farming in the foreseeable future.
Another aspect of smart farming, which is of even greater importance for the future as it promises potentially immense advantages, is ensuring interoperability between different devices and machinery used. As of now, there are ready solutions for smart farming that – thanks to the IoT – use information sharing between different devices in real-time (Jayaraman et al. 2016), However, these solutions “can only utilize a small number of specific IoT devices” and also usually demand those to come from a specific producer (Jayaraman et al. 2016, p. 2).
Such an approach limits the number of options available to a farmer severely and also impacts the overall efficiency of the system negatively. For instance, it deprives a farmer of an opportunity to use cheaper or more effective sensors simply because these come from a different producer (Jayaraman et al. 2016). Hence, IoT solutions that would allow interoperability of devices and machinery regardless of the producing company have the potential to increase farming efficiency immensely and will be essential in the future of smart farming.
As one can see, smart farming allows data acquisition, data evaluation, and precision application with the help of the blockchain, and IoT may reshape the world’s agriculture in the future. Using information and communication technologies in farming provides for greater efficiency, as recognized by many nations from the USA and China to India and South Korea. IoT and blockchain are of particular importance, as they allow creating highly automatized autonomous systems, enable safe data sharing, and contribute to creating a democratic international community of farmers. As for future prospects, increased robotization and interoperability of hardware have the potential to make smart farming even more effective in the years to come.
Balafoutis, A.T., Beck, B. Fountas, S., Tsiropolous, Z., Vangeyte, J., Wal, T., … Pedersen, S.M. (2017). Smart farming technologies – Description, taxonomy, and economic impact. In S.M. Pedersen & K.M. Lind (Eds.), Precision agriculture: Technology and economic perspectives (pp. 21-77). Berlin, Germany: Springer.
Carolan, M. (2018). ‘Smart’ farming techniques as political ontology: Access, sovereignty and the performance of neoliberal and not-so-neoliberal worlds. Sociologia Ruralis, 58 (4), 745-764.
Gupta, A., Mishra, S., Bokde, N., & Kulat, K. (2016). Need of smart water systems in India. International Journal of Applied Engineering Research, 11 (4), 2216-2223.
Jayaraman, P.P., Yavari, A., Georgakopoulos, D., Morshed, A., & Zaslavsky, A. (2016). Internet of Things platform for smart farming: Experiences and lessons learnt. Sensors , 16 (11), 1-17.
Kamilaris, A., Gao, F., Prenafeta-Boldu, F.X., & Ali, M.I. (2016). Agri-IoT: A semantic framework for Internet of Things-enabled smart farming applications. In Proceedings from 2016 IEEE 3rd World Forum on Internet of Things (WF-IoT) (pp. 442-447). Reston, VA: IEEE.
Lin, Y.P., Petway, J.R., Anthony, J., Mukhtar, H., Liao, S.W., Chou, C.F., & Ho, Y.F. (2017). Blockchain: The evolutionary next step for ICT E-agriculture. Environments, 4 (3), 1-13.
Nhamo, N., Chikoye, D., & Gondwe, T. (2017). Smart technologies for sustainable smallholder agriculture: Upscaling in developing countries . London, United Kingdom: Academic Press.
Pivoto, D., Waquil, P.D., Talamini, E., Finocchio, C.P.S., Corte, V.F.D., & Mores, G.V. (2018). Scientific development of smart farming technologies and their application in Brazil. Information Processing in Agriculture, 5 (1), 21-32.
Tripicchio, T., Satler, M., Dabisias, G., Ruffaldi, E., & Avizzano, C.A. (2015). Towards smart farming and sustainable agriculture with drones. In Proceedings from In Intelligent Environments ’15: International Conference on Intelligent Environments (pp. 1-4). Prague, Czech Republic: CPS.
Viani, F. (2016). Experimental validation of the wireless system for irrigation management in smart farming applications . Microwave and Optical Technology Letters, 58 (9), 2186-2189.
- Use of Blockchain in Healthcare: Benefit From the Blockchain Technology
- Using IoT Low-Cost Sensors for Smallholder Farms
- Application of the Internet of Things (IoT) in the Healthcare Industry
- Livestock Food Production Issues
- Soybean and Deforestation in the United States
- Cocoa Production and Its Environmental Performance
- Water Treatment Impact on Wheat Plant Growth
- Emily Baumgaertner: Crop Viruses and Food Security
- Chicago (A-D)
- Chicago (N-B)
IvyPanda. (2021, July 2). Smart Farming and Sustainable Agriculture. https://ivypanda.com/essays/smart-farming-and-sustainable-agriculture/
"Smart Farming and Sustainable Agriculture." IvyPanda , 2 July 2021, ivypanda.com/essays/smart-farming-and-sustainable-agriculture/.
IvyPanda . (2021) 'Smart Farming and Sustainable Agriculture'. 2 July.
IvyPanda . 2021. "Smart Farming and Sustainable Agriculture." July 2, 2021. https://ivypanda.com/essays/smart-farming-and-sustainable-agriculture/.
1. IvyPanda . "Smart Farming and Sustainable Agriculture." July 2, 2021. https://ivypanda.com/essays/smart-farming-and-sustainable-agriculture/.
Bibliography
IvyPanda . "Smart Farming and Sustainable Agriculture." July 2, 2021. https://ivypanda.com/essays/smart-farming-and-sustainable-agriculture/.
Internet-of-Things (IoT)-Based Smart Agriculture: Toward Making the Fields Talk
Ieee account.
- Change Username/Password
- Update Address
Purchase Details
- Payment Options
- Order History
- View Purchased Documents
Profile Information
- Communications Preferences
- Profession and Education
- Technical Interests
- US & Canada: +1 800 678 4333
- Worldwide: +1 732 981 0060
- Contact & Support
- About IEEE Xplore
- Accessibility
- Terms of Use
- Nondiscrimination Policy
- Privacy & Opting Out of Cookies
A not-for-profit organization, IEEE is the world's largest technical professional organization dedicated to advancing technology for the benefit of humanity. © Copyright 2024 IEEE - All rights reserved. Use of this web site signifies your agreement to the terms and conditions.
Agriculture’s connected future: How technology can yield new growth
The agriculture industry has radically transformed over the past 50 years. Advances in machinery have expanded the scale, speed, and productivity of farm equipment, leading to more efficient cultivation of more land. Seed, irrigation, and fertilizers also have vastly improved, helping farmers increase yields. Now, agriculture is in the early days of yet another revolution, at the heart of which lie data and connectivity. Artificial intelligence, analytics, connected sensors, and other emerging technologies could further increase yields, improve the efficiency of water and other inputs, and build sustainability and resilience across crop cultivation and animal husbandry.
The future of connectivity
As the world experiences a quantum leap in the speed and scope of digital connections, industries are gaining new and enhanced tools to boost productivity and spur innovation. Over the next decade, existing technologies like fiber, low-power wide-area networks (LPWAN), Wi-Fi 6, low- to mid-band 5G, and short-range connections like radio-frequency identification (RFID) will expand their reach as networks are built out and adoption grows. At the same time, new generations of these technologies will appear, with upgraded standards. In addition, new types of more revolutionary—and more capital-intensive—frontier connectivity, like high-band 5G and low-Earth-orbit (LEO) satellites, will begin to come online.
Together, these technological developments will unlock powerful new capabilities across industries. Near-global coverage will allow the expansion of use cases even to remote areas and will enable constant connectivity universally. Massive use of Internet of Things (IoT) applications and use cases will be enabled as new technologies allow very high device densities. And mission-critical services will take advantage of ultralow-latency, high-reliability, and high-security connections.
Without a solid connectivity infrastructure, however, none of this is possible. If connectivity is implemented successfully in agriculture, the industry could tack on $500 billion in additional value to the global gross domestic product by 2030, according to our research. This would amount to a 7 to 9 percent improvement from its expected total and would alleviate much of the present pressure on farmers. It is one of just seven sectors that, fueled by advanced connectivity, will contribute $2 trillion to $3 trillion in additional value to global GDP over the next decade, according to research by the McKinsey Center for Advanced Connectivity and the McKinsey Global Institute (MGI) (see sidebar “The future of connectivity”).
Demand for food is growing at the same time the supply side faces constraints in land and farming inputs. The world’s population is on track to reach 9.7 billion by 2050, 1 The World Population Prospects: 2015 Revision, United Nations, Department of Economic and Social Affairs, Population Division, 2015. requiring a corresponding 70 percent increase in calories available for consumption, even as the cost of the inputs needed to generate those calories is rising. 2 World Resources Report: Creating a Sustainable Food Future, United Nations, World Resources Institute, and the World Bank, 2013. By 2030, the water supply will fall 40 percent short of meeting global water needs, 3 World Could Face Water Availability Shortfall by 2030 if Current Trends Continue, Secretary-General Warns at Meeting of High-Level Panel, United Nations, 2016. and rising energy, labor, and nutrient costs are already pressuring profit margins. About one-quarter of arable land is degraded and needs significant restoration before it can again sustain crops at scale. 4 The State of the World’s Land and Water Resources for Food and Agriculture: Managing systems at risk, Food and Agriculture Organization of the United Nations and Earthscan, 2011. And then there are increasing environmental pressures, such as climate change and the economic impact of catastrophic weather events, and social pressures, including the push for more ethical and sustainable farm practices, such as higher standards for farm-animal welfare and reduced use of chemicals and water.
To address these forces poised to further roil the industry, agriculture must embrace a digital transformation enabled by connectivity. Yet agriculture remains less digitized compared with many other industries globally. Past advances were mostly mechanical, in the form of more powerful and efficient machinery, and genetic, in the form of more productive seed and fertilizers. Now much more sophisticated, digital tools are needed to deliver the next productivity leap. Some already exist to help farmers more efficiently and sustainably use resources, while more advanced ones are in development. These new technologies can upgrade decision making, allowing better risk and variability management to optimize yields and improve economics. Deployed in animal husbandry, they can enhance the well-being of livestock, addressing the growing concerns over animal welfare.
Demand for food is growing at the same time the supply side faces constraints in land and farming inputs.
But the industry confronts two significant obstacles. Some regions lack the necessary connectivity infrastructure, making development of it paramount. In regions that already have a connectivity infrastructure, farms have been slow to deploy digital tools because their impact has not been sufficiently proven.
The COVID-19 crisis has further intensified other challenges agriculture faces in five areas: efficiency, resilience, digitization, agility, and sustainability. Lower sales volumes have pressured margins, exacerbating the need for farmers to contain costs further. Gridlocked global supply chains have highlighted the importance of having more local providers, which could increase the resilience of smaller farms. In this global pandemic, heavy reliance on manual labor has further affected farms whose workforces face mobility restrictions. Additionally, significant environmental benefits from decreased travel and consumption during the crisis are likely to drive a desire for more local, sustainable sourcing, requiring producers to adjust long-standing practices. In short, the crisis has accentuated the necessity of more widespread digitization and automation, while suddenly shifting demand and sales channels have underscored the value of agile adaptation.
Current connectivity in agriculture
In recent years, many farmers have begun to consult data about essential variables like soil, crops, livestock, and weather. Yet few if any have had access to advanced digital tools that would help to turn these data into valuable, actionable insights. In less-developed regions, almost all farmwork is manual, involving little or no advanced connectivity or equipment.
Even in the United States, a pioneer country in connectivity, only about one-quarter of farms currently use any connected equipment or devices to access data, and that technology isn’t exactly state-of-the-art, running on 2G or 3G networks that telcos plan to dismantle or on very low-band IoT networks that are complicated and expensive to set up. In either case, those networks can support only a limited number of devices and lack the performance for real-time data transfer, which is essential to unlock the value of more advanced and complex use cases.
Nonetheless, current IoT technologies running on 3G and 4G cellular networks are in many cases sufficient to enable simpler use cases, such as advanced monitoring of crops and livestock. In the past, however, the cost of hardware was high, so the business case for implementing IoT in farming did not hold up. Today, device and hardware costs are dropping rapidly, and several providers now offer solutions at a price we believe will deliver a return in the first year of investment.
These simpler tools are not enough, though, to unlock all the potential value that connectivity holds for agriculture. To attain that, the industry must make full use of digital applications and analytics, which will require low latency, high bandwidth, high resiliency, and support for a density of devices offered by advanced and frontier connectivity technologies like LPWAN, 5G, and LEO satellites (Exhibit 1).
The challenge the industry is facing is thus twofold: infrastructure must be developed to enable the use of connectivity in farming, and where connectivity already exists, strong business cases must be made in order for solutions to be adopted. The good news is that connectivity coverage is increasing almost everywhere. By 2030, we expect advanced connectivity infrastructure of some type to cover roughly 80 percent of the world’s rural areas; the notable exception is Africa, where only a quarter of its area will be covered. The key, then, is to develop more—and more effective—digital tools for the industry and to foster widespread adoption of them.
As connectivity increasingly takes hold, these tools will enable new capabilities in agriculture:
- Massive Internet of Things. Low-power networks and cheaper sensors will set the stage for the IoT to scale up, enabling such use cases as precision irrigation of field crops, monitoring of large herds of livestock, and tracking of the use and performance of remote buildings and large fleets of machinery.
- Mission-critical services. Ultralow latency and improved stability of connections will foster confidence to run applications that demand absolute reliability and responsiveness, such as operating autonomous machinery and drones.
- Near-global coverage. If LEO satellites attain their potential, they will enable even the most remote rural areas of the world to use extensive digitization, which will enhance global farming productivity.
Connectivity’s potential for value creation
By the end of the decade, enhanced connectivity in agriculture could add more than $500 billion to global gross domestic product, a critical productivity improvement of 7 to 9 percent for the industry. 5 This represents our estimate of the total potential for value added in agricultural production; it is not an estimate of the agritech and precision-agriculture market size. Much of that value, however, will require investments in connectivity that today are largely absent from agriculture. Other industries already use technologies like LPWAN, cloud computing, and cheaper, better sensors requiring minimal hardware, which can significantly reduce the necessary investment. We have analyzed five use cases—crop monitoring, livestock monitoring, building and equipment management, drone farming, and autonomous farming machinery—where enhanced connectivity is already in the early stages of being used and is most likely to deliver the higher yields, lower costs, and greater resilience and sustainability that the industry needs to thrive in the 21st century (Exhibit 2).
It’s important to note that use cases do not apply equally across regions. For example, in North America, where yields are already fairly optimized, monitoring solutions do not have the same potential for value creation as in Asia or Africa, where there is much more room to improve productivity. Drones and autonomous machinery will deliver more impact to advanced markets, as technology will likely be more readily available there (Exhibit 3).
About the use-case research
The value of our agriculture-connectivity use cases resides primarily in labor efficiencies, input optimization, yield increases, reduced overhead, and improvements in operation and maintenance of machinery. Each use case enables a series of improvement levers in those areas that promise to enhance the productivity of farming (exhibit).
We applied those levers to the profitability drivers of agricultural production to derive an economic potential for the industry as a whole. For example, a use case might enable a 5 to 10 percent reduction in fertilizer usage, saving costs for the farmer, or enable 3 percent higher yields, leading to greater revenues for the farmer. In fact, higher yields represent the largest opportunity, with advanced connectivity potentially adding some $350 billion of value to global food production without additional inputs or labor costs.
Potential value initially will accrue to large farms that have more investing power and better incentives to digitize. Connectivity promises easier surveying of large tracts, and the fixed costs of developing IoT solutions are more easily offset in large production facilities than on small family farms. Crops like cereals, grains, fruits, and vegetables will generate most of the value we identified, for similar reasons. Connectivity enables more use cases in these sectors than in meat and dairy, because of the large average size of farms, relatively higher player consolidation, and better applicability of connected technologies, as IoT networks are especially adapted to static monitoring of many variables. It’s also interesting to note that Asia should garner about 60 percent of the total value simply because it produces the biggest volume of crops (see sidebar “About the use-case research”).
Use case 1: Crop monitoring
Connectivity offers a variety of ways to improve the observation and care of crops. Integrating weather data, irrigation, nutrient, and other systems could improve resource use and boost yields by more accurately identifying and predicting deficiencies. For instance, sensors deployed to monitor soil conditions could communicate via LPWAN, directing sprinklers to adjust water and nutrient application. Sensors could also deliver imagery from remote corners of fields to assist farmers in making more informed and timely decisions and getting early warnings of problems like disease or pests.
Smart monitoring could also help farmers optimize the harvesting window. Monitoring crops for quality characteristics—say, sugar content and fruit color—could help farmers maximize the revenue from their crops.
Most IoT networks today cannot support imagery transfer between devices, let alone autonomous imagery analysis, nor can they support high enough device numbers and density to monitor large fields accurately. Narrowband Internet of Things (NB-IoT) and 5G promise to solve these bandwidth and connection-density issues. The use of more and smoother connections between soil, farm equipment, and farm managers could unlock $130 billion to $175 billion in value by 2030.
Use case 2: Livestock monitoring
Preventing disease outbreaks and spotting animals in distress are critical in large-scale livestock management, where most animals are raised in close quarters on a regimen that ensures they move easily through a highly automated processing system. Chips and body sensors that measure temperature, pulse, and blood pressure, among other indicators, could detect illnesses early, preventing herd infection and improving food quality. Farmers are already using ear-tag technology from providers such as Smartbow (part of Zoetis) to monitor cows’ heat, health, and location, or technology from companies such as Allflex to implement comprehensive electronic tracing in case of disease outbreaks.
Similarly, environmental sensors could trigger automatic adjustments in ventilation or heating in barns, lessening distress and improving living conditions that increasingly concern consumers. Better monitoring of animal health and growth conditions could produce $70 billion to $90 billion in value by 2030.
Would you like to learn more about the McKinsey Center for Advanced Connectivity ?
Use case 3: building and equipment management.
Chips and sensors to monitor and measure levels of silos and warehouses could trigger automated reordering, reducing inventory costs for farmers, many of whom are already using such systems from companies like Blue Level Technologies. Similar tools could also improve shelf life of inputs and reduce post-harvest losses by monitoring and automatically optimizing storage conditions. Monitoring conditions and usage of buildings and equipment also has the potential to reduce energy consumption. Computer vision and sensors attached to equipment and connected to predictive-maintenance systems could decrease repair costs and extend machinery and equipment life.
Such solutions could achieve $40 billion to $60 billion in cost savings by 2030.
Use case 4: Farming by drone
Agriculture has been using drones for some two decades, with farmers around the world relying on pioneers like Yamaha’s RMAX remote-controlled helicopter to help with crop spraying. Now the next generation of drones is starting to impact the sector, with the ability to survey crops and herds over vast areas quickly and efficiently or as a relay system for ferrying real-time data to other connected equipment and installations. Drones also could use computer vision to analyze field conditions and deliver precise interventions like fertilizers, nutrients, and pesticides where crops most need them. Or they could plant seed in remote locations, lowering equipment and workforce costs. By reducing costs and improving yields, the use of drones could generate between $85 billion and $115 billion in value.
Use case 5: Autonomous farming machinery
More precise GPS controls paired with computer vision and sensors could advance the deployment of smart and autonomous farm machinery. Farmers could operate a variety of equipment on their field simultaneously and without human intervention, freeing up time and other resources. Autonomous machines are also more efficient and precise at working a field than human-operated ones, which could generate fuel savings and higher yields. Increasing the autonomy of machinery through better connectivity could create $50 billion to $60 billion of additional value by 2030.
Additional sources of value
Connected technologies offer an additional, indirect benefit, the value of which is not included in the estimates given in these use cases. The global farming industry is highly fragmented, with most labor done by individual farm owners. Particularly in Asia and Africa, few farms employ outside workers. On such farms, the adoption of connectivity solutions should free significant time for farmers, which they can use to farm additional land for pay or to pursue work outside the industry.
We find the value of deploying advanced connectivity on these farms to achieve such labor efficiencies represents almost $120 billion, bringing the total value of enhanced connectivity from direct and indirect outcomes to more than $620 billion by 2030. The extent to which this value will be captured, however, relies largely on advanced connectivity coverage, which is expected to be fairly low, around 25 percent, in Africa and poorer parts of Asia and Latin America. Achieving the critical mass of adopters needed to make a business case for deploying advanced connectivity also will be more difficult in those regions, where farming is more fragmented than in North America and Europe.
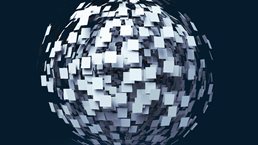
Connected world: An evolution in connectivity beyond the 5G revolution
Implications for the agricultural ecosystem.
As the agriculture industry digitizes, new pockets of value will likely be unlocked. To date, input providers selling seed, nutrients, pesticides, and equipment have played a critical role in the data ecosystem because of their close ties with farmers, their own knowledge of agronomy, and their track record of innovation. For example, one of the world’s largest fertilizer distributors now offers both fertilizing agents and software that analyzes field data to help farmers determine where to apply their fertilizers and in what quantity. Similarly, a large-equipment manufacturer is developing precision controls that make use of satellite imagery and vehicle-to-vehicle connections to improve the efficiency of field equipment.
Advanced connectivity does, however, give new players an opportunity to enter the space. For one thing, telcos and LPWAN providers have an essential role to play in installing the connectivity infrastructure needed to enable digital applications on farms. They could partner with public authorities and other agriculture players to develop public or private rural networks, capturing some of the new value in the process.
Agritech companies are another example of the new players coming into the agriculture sphere. They specialize in offering farmers innovative products that make use of technology and data to improve decision making and thereby increase yields and profits. Such agritech enterprises could proffer solutions and pricing models that reduce perceived risk for farmers—with, for example, subscription models that remove the initial investment burden and allow farmers to opt out at any time—likely leading to faster adoption of their products. An Italian agritech is doing this by offering to monitor irrigation and crop protection for wineries at a seasonal, per-acre fee inclusive of hardware installation, data collection and analysis, and decision support. Agritech also could partner with agribusinesses to develop solutions.
Still, much of this cannot happen until many rural areas get access to a high-speed broadband network. We envision three principal ways the necessary investment could take place to make this a reality:
- Telco-driven deployment. Though the economics of high-bandwidth rural networks have generally been poor, telcos could benefit from a sharp increase in rural demand for their bandwidth as farmers embrace advanced applications and integrated solutions.
- Provider-driven deployment. Input providers, with their existing industry knowledge and relationships, are probably best positioned to take the lead in connectivity-related investment. They could partner with telcos or LPWAN businesses to develop rural connectivity networks and then offer farmers business models integrating connected technology and product and decision support.
- Farmer-driven deployment. Farm owners, alone or in tandem with LPWAN groups or telcos, could also drive investment. This would require farmers to develop the knowledge and skills to gather and analyze data locally, rather than through third parties, which is no small hurdle. But farmers would retain more control over data.
How to do it
Regardless of which group drives the necessary investment for connectivity in agriculture, no single entity will be able to go it alone. All of these advances will require the industry’s main actors to embrace collaboration as an essential aspect of doing business. Going forward, winners in delivering connectivity to agriculture will need deep capabilities across various domains, ranging from knowledge of farm operations to advanced data analytics and the ability to offer solutions that integrate easily and smoothly with other platforms and adjacent industries. For example, data gathered by autonomous tractors should seamlessly flow to the computer controlling irrigation devices, which in turn should be able to use weather-station data to optimize irrigation plans.
Connectivity pioneers in the industry, however, have already started developing these new capabilities internally. Organizations prefer keeping proprietary data on operations internal for confidentiality and competitive reasons. This level of control also makes the data easier to analyze and helps the organization be more responsive to evolving client needs.
But developing new capabilities is not the end game. Agriculture players able to develop partnerships with telcos or LPWAN players will gain significant leverage in the new connected-agriculture ecosystem. Not only will they be able to procure connectivity hardware more easily and affordably through those partnerships, they will also be better positioned to develop close relationships with farmers as connectivity becomes a strategic issue. Input providers or distributors could thus find themselves in a connectivity race. If input providers manage to develop such partnerships, they could connect directly with farmers and cut out distributors entirely. If distributors win that race, they will consolidate their position in the value chain by remaining an essential intermediary, closer to the needs of farmers.
The public sector also could play a role by improving the economics of developing broadband networks, particularly in rural areas. For example, the German and Korean governments have played a major role in making network development more attractive by heavily subsidizing spectrum or providing tax breaks to telcos. 6 “Das Breitbandförderprogramm des Bundes” [in German], Bundesministerium für Verkehr und digitale Infrastruktur, 2020, bmvi.de; 5G in Korea: Volume 1: Get a taste of the future, Samsung Electronics, 2019, samsungnetworks.com. Other regions could replicate this model, accelerating development of connective products by cost-effectively giving input providers and agritech companies assurance of a backbone over which they could deliver services. Eventual deployment of LEO satellite constellations would likely have a similar impact.
Agriculture, one of the world’s oldest industries, finds itself at a technological crossroads. To handle increasing demand and several disruptive trends successfully, the industry will need to overcome the challenges to deploying advanced connectivity. This will require significant investment in infrastructure and a realignment of traditional roles. It is a huge but critical undertaking, with more than $500 billion in value at stake. The success and sustainability of one of the planet’s oldest industries may well depend on this technology transformation, and those that embrace it at the outset may be best positioned to thrive in agriculture’s connectivity-driven future.
Lutz Goedde is a senior partner and global leader of McKinsey’s Agriculture Practice in the Denver office; Joshua Katz is a partner in the Stamford office; and Alexandre Menard is a senior partner in the Paris office, where Julien Revellat is an associate partner.
The authors wish to thank Nicolas D. Estais, Claus Gerckens, Vincent Tourangeau, and the McKinsey Center for Advanced Connectivity for their contributions to the article.
This article was edited by Daniel Eisenberg, a senior editor in the New York office.
Explore a career with us
Related articles.

Agriculture supply-chain optimization and value creation
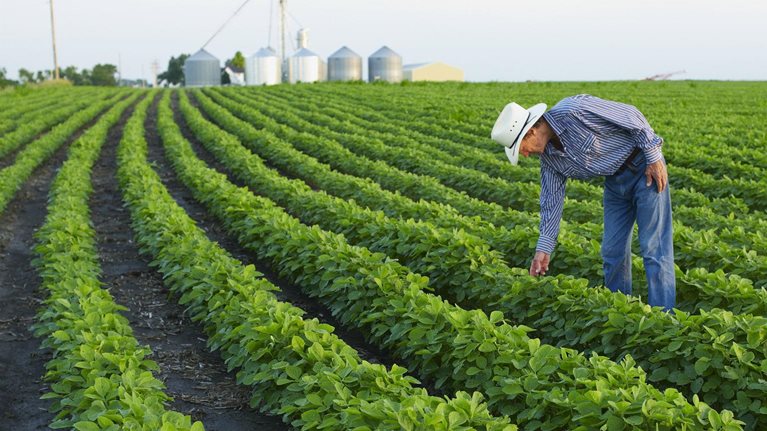
How to incentivize food systems to meet the realities of the 21st century
- Understanding Poverty
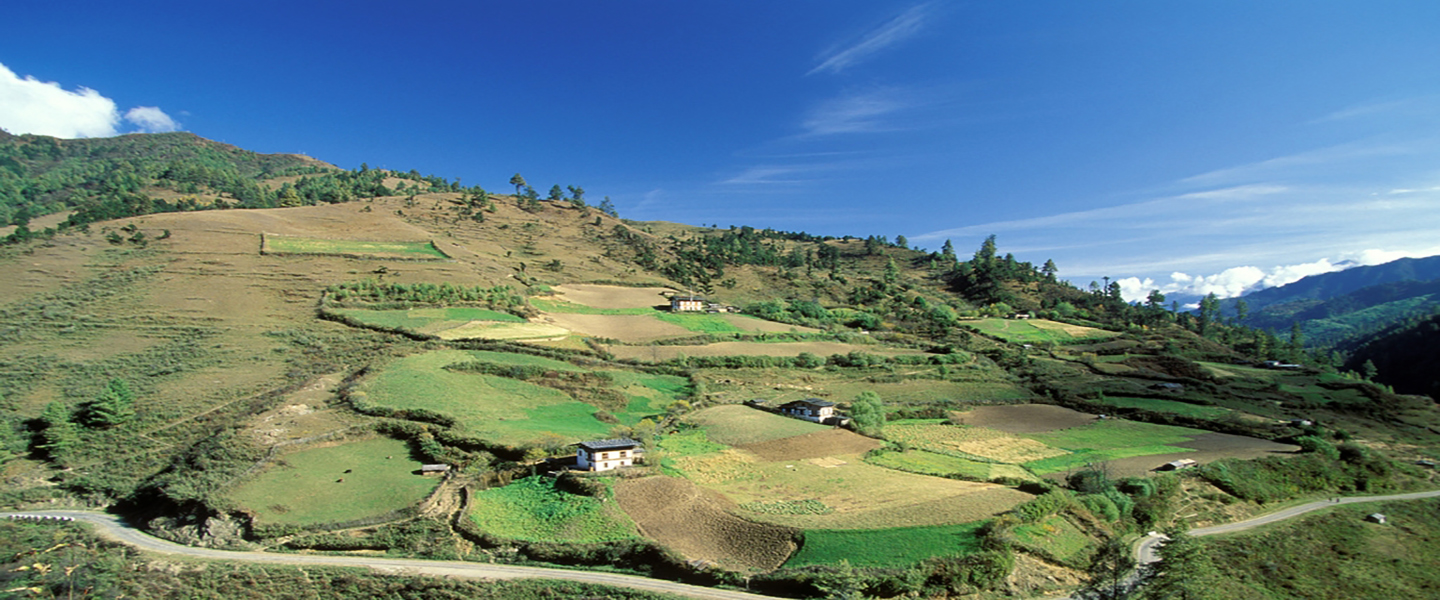
Climate-smart agriculture
Climate-smart agriculture (csa) is an integrated approach to managing landscapes—cropland, livestock, forests and fisheries--that address the interlinked challenges of food security and climate change..
Climate change and food and nutrition insecurity pose two of the greatest development challenges of our time. Yet a more sustainable food system can not only heal the planet, but ensure food security for all.
Today, the global agrifood system emits one-third of all emissions. Global food demand is estimated to increase to feed a projected global population of 9.7 billion people by 2050. Traditionally, the increase in food production has been linked to agricultural expansion, and unsustainable use of land and resources. This creates a vicious circle, leading to an increase in emissions.
Food systems are the leading source of methane emissions and biodiversity loss, and they use around 70% of fresh water. If food waste were a country, it would be the third highest emitter in the world. Meanwhile, emissions from agriculture are increasing in developing countries – a worrying trend which must be reversed.
Without significant climate mitigation action in the agri-food sector, the Paris Agreement goals cannot be reached. Agriculture is the primary cause of deforestation, threatening pristine ecosystems such as the Amazon and the Congo Basin. Without action, emissions from food systems will rise even further, with increasing food production.
Achieving the Triple Win of CSA
The global agrifood system must therefore deliver on multiple fronts. It must feed the world, adapt to climate change, and drastically reduce its greenhouse gas emissions. In response to these challenges, the concept of Climate-smart Agriculture (CSA) has emerged as a holistic approach to end food security and promote sustainable development while addressing climate change issues.
CSA is a set of agricultural practices and technologies which simultaneously boost productivity, enhance resilience and reduce GHG emissions. Although it is built on existing agricultural knowledge, technologies, and sustainability principles, CSA is distinct in several ways. First, it has an explicit focus on addressing climate change in the agrifood system. Second, CSA systematically considers the synergies and tradeoffs that exist between productivity, adaptation, and mitigation. And third, CSA encompasses a range of practices and technologies that are tailored to specific agro-ecological conditions and socio-economic contexts including the adoption of climate-resilient crop varieties, conservation agriculture techniques, agroforestry, precision farming, water management strategies, and improved livestock management. By implementing these practices, triple win results can be achieved:
1. Increased productivity: Produce more and higher quality food without putting an additional strain on natural resources, to improve nutrition security and boost incomes, especially for 75 percent of the world’s poor who live in rural areas and mainly rely on agriculture for their livelihoods.
2. Enhanced resilience: Reduce vulnerability to droughts, pests, diseases and other climate-related risks and shocks; and improve the capacity to adapt and grow in the face of longer-term stresses like increased seasonal variability and more erratic weather patterns.
3. Reduced emissions: Reduce greenhouse gas emissions of the food system, avoid deforestation due to cropland expansion, and increase the carbon sequestration of plants and soils.
Finally, funding for CSA needs to be increased to align available finance with the relevance of the sector. Despite causing one third of global greenhouse gas emissions, agrifood systems receive 4% of climate finance, with only a fifth of this going to smallholders. Current financial flows need to be realigned in order to support a sustainable agrifood system transformation.
Climate-Smart Agriculture and the World Bank Group
The World Bank has significantly scaled up its engagement and investment in climate-smart agriculture (CSA). In its Climate Change Action Plan (2021- 2025), the World Bank has identified Agriculture, Food, Water and Land as one of the five key transitions needed to tackle the Paris Agreement. Since the adoption of the Paris Agreement, the World Bank has increased financing for CSA by eight times, to almost $3 billion annually.
As of July 2023, all new World Bank operations must be aligned with the goals of the Paris Agreement , meaning that CSA is at the core of all the World Bank’s new agriculture and food operations. To this end, the World Bank has prepared a Sector Note of Paris Alignment of its Agriculture and Food operations. Furthermore, all projects are screened for climate and disaster risks. Climate change indicators are used to measure outputs and outcomes, and greenhouse gas accounting of projects is conducted prior to approval . These actions will help client countries implement their Nationally Determined Contributions (NDCs) in the agriculture sector, and will contribute to progress on the Sustainable Development Goals (SDGs) for climate action, poverty, and the eradication of hunger.
The World Bank engages strategically with countries, supporting them to enhance productivity, improve resilience and reduce greenhouse gas emissions. The World Bank uses the following tools, diagnostics and other analytics to help countries in the transition towards sustainable agriculture.
- Country Climate and Development Reports (CCDRs), new core diagnostics, help countries prioritize the most impactful actions that can reduce greenhouse gas emissions and boost adaptation, while delivering on broader development goals. CCDRs identify climate impacts on countries’ agrifood systems, such as reduced yields and increased food prices, and present a variety of country-specific technology options as well as policy reforms under the umbrella of CSA.
- Climate-Smart Agriculture (CSA) Country Profiles developed by the World Bank and partners, give an overview of the agricultural challenges in countries around the world, and how CSA can help them adapt to and mitigate climate change. They bridge knowledge gaps by providing clarity on CSA terminology, components, relevant issues, and how to contextualize them under different country conditions.
- Climate-Smart Agriculture Investment Plans (CSAIPs) developed for a subset of client countries aim to mainstream CSA into national agricultural policies and to identify investment opportunities in CSA. The World Bank provides technical assistance and financial support to help countries develop and implement CSAIPs. These plans prioritize investments in climate-resilient infrastructure, capacity building, and knowledge sharing to promote sustainable agricultural practices. CSAIPs are available, or currently under preparation, for Bangladesh , Belize, Burkina Faso, Cote D’Ivoire , Cameroon, the Republic of Congo, Ethiopia, Ghana , Iraq, Jordan, Kenya, Lesotho , Madagascar, Mali , Morocco , Nepal , Senegal, Zambia , and Zimbabwe .
- The World Bank also supports research programs such as with the CGIAR , which develops and supports climate-smart technologies and management methods, early warning systems, risk insurance, and other innovations that promote resilience and combat climate change.”
Working Toward Resilience and Food and Nutrition Security, while Curbing GHG Emissions
The Bank’s support of CSA is making a difference across the globe, for example:
- A new US$345 million loan for the China Green Agricultural and Rural Revitalization Program for Results will support China’s global public goods agenda by promoting the greening of agriculture and rural development in Hubei and Hunan provinces in central China. The program will reduce greenhouse gas (GHG) emissions from crop and livestock farming, increase carbon sequestration in farmlands, and improve biodiversity protection and restoration in agricultural ecosystems, while strengthening the institutional capacity of local governments to integrate environmental and decarbonization objectives in government rural revitalization plans and investments. World Bank financing will complement a US$4.1 billion commitment by the Government of China (GoC).
- The US$621 million Food Systems Resilience Program for Eastern and Southern Africa (Phase 3) FSRP Project in Kenya, Comoros, Malawi, Somalia aims to increase the resilience of food systems and the recipients’ preparedness for food insecurity. The project has six components, including building resilient agricultural production capacity to strengthen the productivity and resilience of domestic food production to shocks and stressors, by supporting the development and adoption of improved agricultural inputs and services and climate-smart and gender-sensitive farming technologies in the crops, livestock, and fisheries sectors.
- A US$200 million credit for the Punjab Resilient and Inclusive Agriculture Transformation Project (PRIAT) will help Pakistan enhance access to, and productivity of, agricultural water, and improve incomes of farmers supported by the project. PRIAT will notably reduce the differences in water availability among head, middle, and tail end users of watercourses, increase agricultural output per unit of water used at farm level for selected crops, increase the share of area under high-value crops cultivation, and increase agriculture incomes of households participating in project activities, yielding important climate change adaptation and mitigation co-benefits.
- The US$125 million Agriculture Resilience, Value Chain Development and Innovation (ARDI) program will play a pivotal role in strengthening the transition Jordan’s agri-food sector. It supports Jordan's National Sustainable Agriculture Plan and aims to enhance climate resilience, competitiveness, and inclusivity of the agri-food sector. Over the next five years, it will support 30,000 farming household with the adoption of climate-smart and water-efficient agricultural practices, provide needs-based training, create about 12,000 employment opportunities, and promote value chain and export promotion through advanced market diagnostics. A particular focus will be on strengthening the participation of women, youth and refugees.
Last Updated: Feb 26, 2024
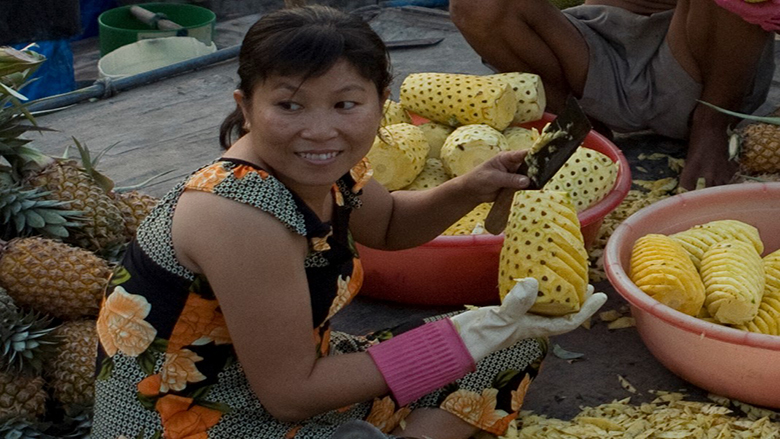
Agriculture and Food
Go back to main topic.
- 'Recipe for a Livable Planet' report launch event, May 22 - Watch live stream
- Recipe for a Livable Planet: Achieving Net Zero Emissions in the Agrifood System
- Climate-Smart Agriculture Country Profiles
- Climate-Smart Agriculture Investment Plans
- Future of Food: Shaping a Climate-Smart Global Food System
- Podcast: Can the World Feed Itself as the Climate Changes?
- Brief: Livestock and Sustainability at the World Bank
- Bringing the Concept of Climate-Smart Agriculture to Life
A Better Food System for Healthier People, Planet and Economy
Advertisement
Climate-smart agriculture: adoption, impacts, and implications for sustainable development
- Original Article
- Open access
- Published: 29 April 2024
- Volume 29 , article number 44 , ( 2024 )
Cite this article
You have full access to this open access article
- Wanglin Ma ORCID: orcid.org/0000-0001-7847-8459 1 &
- Dil Bahadur Rahut ORCID: orcid.org/0000-0002-7505-5271 2
1537 Accesses
9 Altmetric
Explore all metrics
The 19 papers included in this special issue examined the factors influencing the adoption of climate-smart agriculture (CSA) practices among smallholder farmers and estimated the impacts of CSA adoption on farm production, income, and well-being. Key findings from this special issue include: (1) the variables, including age, gender, education, risk perception and preferences, access to credit, farm size, production conditions, off-farm income, and labour allocation, have a mixed (either positive or negative) influence on the adoption of CSA practices; (2) the variables, including labour endowment, land tenure security, access to extension services, agricultural training, membership in farmers’ organizations, support from non-governmental organizations, climate conditions, and access to information consistently have a positive impact on CSA adoption; (3) diverse forms of capital (physical, social, human, financial, natural, and institutional), social responsibility awareness, and digital advisory services can effectively promote CSA adoption; (4) the establishment of climate-smart villages and civil-society organizations enhances CSA adoption by improving their access to credit; (5) CSA adoption contributes to improved farm resilience to climate change and mitigation of greenhouse gas emissions; (6) CSA adoption leads to higher crop yields, increased farm income, and greater economic diversification; (7) integrating CSA technologies into traditional agricultural practices not only boosts economic viability but also contributes to environmental sustainability and health benefits; and (8) there is a critical need for international collaboration in transferring technology for CSA. Overall, the findings of this special issue highlight that through targeted interventions and collaborative efforts, CSA can play a pivotal role in achieving food security, poverty alleviation, and climate resilience in farming communities worldwide and contribute to the achievements of the United Nations Sustainable Development Goals.
Similar content being viewed by others
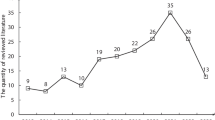
A systematic literature review of factors influencing the adoption of climate-smart agricultural practices
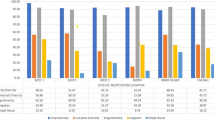
Farm households’ adoption of climate-smart agricultural practices: empirical evidence from Seychelles
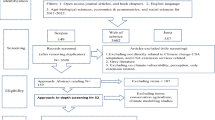
Climate-smart agriculture (CSA) adaptation, adaptation determinants and extension services synergies: a systematic review
Avoid common mistakes on your manuscript.
1 Introduction
Climate change reduces agricultural productivity and leads to greater instability in crop production, disrupting the global food supply and resulting in food and nutritional insecurity. In particular, climate change adversely affects food production through water shortages, pest outbreaks, and soil degradation, leading to significant crop yield losses and posing significant challenges to global food security (Kang et al. 2009 ; Läderach et al. 2017 ; Arora 2019 ; Zizinga et al. 2022 ; Mirón et al. 2023 ). United Nations reported that the human population will reach 9.7 billion by 2050. In response, food-calorie production will have to expand by 70% to meet the food demand of the growing population (United Nations 2021 ). Hence, it is imperative to advocate for robust mitigation strategies that counteract the negative impacts of climate change and enhance the flexibility and speed of response in smallholder farming systems.
A transformation of the agricultural sector towards climate-resilient practices can help tackle food security and climate change challenges successfully. Climate-smart agriculture (CSA) is an approach that guides farmers’ actions to transform agrifood systems towards building the agricultural sector’s resilience to climate change based on three pillars: increasing farm productivity and incomes, enhancing the resilience of livelihoods and ecosystems, and reducing and removing greenhouse gas emissions from the atmosphere (FAO 2013 ). Promoting the adoption of CSA practices is crucial to improve smallholder farmers’ capacity to adapt to climate change, mitigate its impact, and help achieve the United Nations Sustainable Development Goals.
Realizing the benefits of adopting CSA, governments in different countries and international organizations such as the Consultative Group on International Agricultural Research (CGIAR), the Food and Agriculture Organisation (FAO) of the United Nations, and non-governmental organizations (NGOs) have made great efforts to scale up and out the CSA. For example, climate-smart villages in India (Alam and Sikka 2019 ; Hariharan et al. 2020 ) and civil society organizations in Africa, Asia, and Latin America (Waters-Bayer et al. 2015 ; Brown 2016 ) have been developed to reduce information costs and barriers and bridge the gap in finance access to promote farmers’ adoption of sustainable agricultural practices, including CSA. Besides, agricultural training programs have been used to enhance farmers’ knowledge of CSA and their adoption of the technology in Ghana (Zakaria et al. 2020 ; Martey et al. 2021 ).
As a result, smallholder farmers worldwide have adopted various CSA practices and technologies (e.g., integrated crop systems, drop diversification, inter-cropping, improved pest, water, and nutrient management, improved grassland management, reduced tillage and use of diverse varieties and breeds, restoring degraded lands, and improved the efficiency of input use) to reach the objectives of CSA (Kpadonou et al. 2017 ; Zakaria et al. 2020 ; Khatri-Chhetri et al. 2020 ; Aryal et al. 2020a ; Waaswa et al. 2022 ; Vatsa et al. 2023 ). In the Indian context, technologies such as laser land levelling and the happy seeder have been promoted widely for their potential in climate change adaptation and mitigation, offering benefits in terms of farm profitability, emission reduction, and water and land productivity (Aryal et al. 2020b ; Keil et al. 2021 ). In some African countries such as Tanzania and Kenya, climate-smart feeding practices in the livestock sector have been suggested to tackle challenges in feed quality and availability exacerbated by climate change, aiming to improve livestock productivity and resilience (García de Jalón et al. 2017 ; Shikuku et al. 2017 ; Radeny et al. 2022 ).
Several studies have investigated the factors influencing farmers’ decisions to adopt CSA practices. They have focused on, for example, farmers’ characteristics (e.g., age, gender, and education), farm-level characteristics (e.g., farm size, land fertility, and land tenure security), socioeconomic factors (e.g., economic conditions), institutional factors (e.g., development programs, membership in farmers’ organizations, and access to agricultural training), climate conditions, and access to information (Aryal et al. 2018 ; Tran et al. 2020 ; Zakaria et al. 2020 ; Kangogo et al. 2021 ; Diro et al. 2022 ; Kifle et al. 2022 ; Belay et al. 2023 ; Zhou et al. 2023 ). For example, Aryal et al. ( 2018 ) found that household characteristics (e.g., general caste, education, and migration status), plot characteristics (e.g., tenure of plot, plot size, and soil fertility), distance to market, and major climate risks are major factors determining farmers’ adoption of multiple CSA practices in India. Tran et al. ( 2020 ) reported that age, gender, number of family workers, climate-related factors, farm characteristics, distance to markets, access to climate information, confidence in the know-how of extension workers, membership in social/agricultural groups, and attitude toward risk are the major factors affecting rice farmers’ decisions to adopt CSA technologies in Vietnam. Diro et al.’s ( 2022 ) analysis revealed that coffee growers’ decisions to adopt CSA practices are determined by their education, extension (access to extension services and participation on field days), and ownership of communication devices, specifically radio in Ethiopia. Zhou et al. 2023 ) found that cooperative membership significantly increases the adoption of climate-smart agricultural practices among banana-producing farmers in China. These studies provide significant insights regarding the factors influencing farmers’ decisions regarding CSA adoption.
A growing body of studies have also estimated the effects of CSA adoption. They have found that CSA practices enhance food security and dietary diversity by increasing crop yields and rural incomes (Amadu et al. 2020 ; Akter et al. 2022 ; Santalucia 2023 ; Tabe-Ojong et al. 2023 ; Vatsa et al. 2023 ; Omotoso and Omotayo 2024 ). For example, Akter et al. ( 2022 ) found that adoption of CSA practices was positively associated with rice, wheat, and maize yields and household income, contributing to household food security in Bangladesh. By estimating data from rice farmers in China, Vatsa et al. ( 2023 ) reported that intensifying the adoption of climate-smart agricultural practices improved rice yield by 94 kg/mu and contributed to food security. Santalucia ( 2023 ) and Omotoso and Omotayo ( 2024 ) found that adoption of CSA practices (improved maize varieties and maize-legume intercropping) increases household dietary diversity and food security among smallholders in Tanzania and Nigeria, respectively.
Agriculture is crucial in climate change, accounting for roughly 20% of worldwide greenhouse gas (GHG) emissions. Additionally, it is responsible for approximately 45% of the global emissions of methane, a potent gas that significantly contributes to heat absorption in the atmosphere. CSA adoption improves farm resilience to climate variability (e.g., Makate et al. 2019 ; Jamil et al. 2021 ) and mitigates greenhouse gas emissions (Israel et al. 2020 ; McNunn et al. 2020 ). For example, Makate et al. ( 2019 ) for southern Africa and Jamil et al. ( 2021 ) for Pakistan found that promoting CSA innovations is crucial for boosting farmers’ resilience to climate change. McNunn et al. ( 2020 ) reported that CSA adoption significantly reduces greenhouse gas emissions from agriculture by increasing soil organic carbon stocks and decreasing nitrous oxide emissions.
Although a growing number of studies have enriched our understanding of the determinants and impacts of ICT adoption, it should be emphasized that no one-size-fits-all approach exists for CSA technology adoption due to geographical and environmental variability. The definitions of CSA should also be advanced to better adapt to changing climate and regional production conditions. Clearly, despite the extensive research on CSA, several gaps remain. First, there is a lack of comprehensive studies that consolidate findings across different geographical regions to inform policymaking effectively. The calls for studies on literature review and meta-analysis to synthesize the findings of the existing studies to make our understanding generalized. Second, although the literature on determinants of CSA adoption is becoming rich, there is a lack of understanding of how CSA adoption is influenced by different forms of capital, social responsibility awareness of farmers’ cultivating family farms, and digital advisory services. Third, there is a lack of understanding of how climate-smart villages and civil society organizations address farmers’ financial constraints and encourage them to adopt modern sustainable agricultural practices, including CSA practices. Fourth, very few studies have explored how CSA adoption influences the benefit–cost ratio of farm production, factor demand, and input substitution. Fifth, no previous studies have reported the progress of research on CSA. Addressing these gaps is crucial for designing and implementing effective policies and programs that support the widespread adoption of CSA practices, thereby contributing to sustainable agricultural development and climate resilience.
We address the research gaps mentioned above and extend the findings in previous studies by organizing a Special Issue on “Climate-Smart Agriculture: Adoption, Impacts, and Implications for Sustainable Development” in the Mitigation and Adaptation Strategies for Global Change (MASGC) journal. We aim to collect high-quality theoretical and applied research papers discussing CSA and seek to comprehensively understand the associations between CSA and sustainable rural and agricultural development. To achieve this goal, we aim to find answers to these questions: What are the CSA practices and technologies (either single or multiple) that are currently adopted in smallholder farming systems? What are the key barriers, challenges, and drivers of promoting CSA practices? What are the impacts of adopting these practices? Answers to these questions will help devise appropriate solutions for promoting sustainable agricultural production and rural development. They will also provide insights for policymakers to design appropriate policy instruments to develop agricultural practices and technologies and promote them to sustainably enhance the farm sector’s resilience to climate change and increase productivity.
Finally, 19 papers were selected after a rigorous peer-review process and published in this special issue. We collected 10 papers investigating the determinants of CSA adoption. Among them, four papers investigated the determinants of CSA adoption among smallholders by reviewing and summarizing the findings in the literature and conducting a meta-analysis. Three papers explored the role of social-economic factors on ICT adoption, including capital, social responsibility awareness, and digital advisory services. Besides, three papers examined the associations between external development interventions, including climate-smart villages and civil-society initiatives, and CSA adoption. We collected eight papers exploring the impacts of CSA adoption. Among them, one paper conducted a comprehensive literature review to summarize the impacts of CSA adoption on crop yields, farm income, and environmental sustainability. Six papers estimated the impacts of CSA adoption on crop yields and farm income, and one paper focused on the impact of CSA adoption on factor demand and input substitution. The last paper included in this special issue delved into the advancements in technological innovation for agricultural adaptation within the context of climate-smart agriculture.
The structure of this paper is as follows: Section 2 summarizes the papers received in this special issue. Section 3 introduces the international conference that was purposely organized for the special issue. Section 4 summarizes the key findings of the 19 papers published in the special issue, followed by a summary of their policy implications, presented in Section 5 . The final section provides a brief conclusion.
2 Summary of received manuscripts
The special issue received 77 submissions, with the contributing authors hailing from 22 countries, as illustrated in Fig. 1 . This diversity highlights the global interest and wide-ranging contributions to the issue. Notably, over half of these submissions (53.2%) originated from corresponding authors in India and China, with 29 and 12 manuscripts, respectively. New Zealand authors contributed six manuscripts, while their Australian counterparts submitted four. Following closely, authors from the United Kingdom and Kenya each submitted three manuscripts. Authors from Thailand, Pakistan, Japan, and Germany submitted two manuscripts each. The remaining 12 manuscripts came from authors in Vietnam, Uzbekistan, the Philippines, Nigeria, the Netherlands, Malaysia, Italy, Indonesia, Ghana, Ethiopia, Brazil, and Bangladesh.
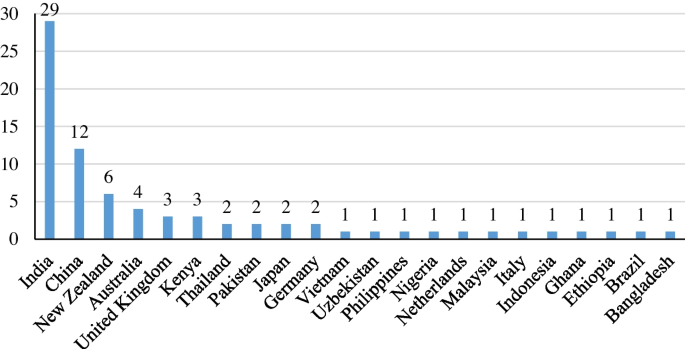
Distributions of 77 received manuscripts by corresponding authors' countries
Among the 77 received manuscripts, 30 were desk-rejected by the guest editors because they did not meet the aims and scope of the special issue, and the remaining 47, considered candidate papers for the special issue, were sent for external review. The decision on each manuscript was made based on review reports of 2–4 experts in this field. The guest editors also read and commented on each manuscript before they made decisions.
3 ADBI virtual international conference
3.1 selected presentations.
The guest editors from Lincoln University (New Zealand) and the Asian Development Bank Institute (ADBI) (Tokyo, Japan) organized a virtual international conference on the special issue theme “ Climate-Smart Agriculture: Adoption, Impacts, and Implications for Sustainable Development ”. The conference was organized on 10–11 October 2023 and was supported by the ADBI. Footnote 1 As previously noted, the guest editors curated a selection of 47 manuscripts from the pool of 77 submissions, identifying them as potential candidates for inclusion in the special issue, and sent them out for external review. Given the logistical constraints of orchestrating a two-day conference, the guest editors ultimately extended invitations to 20 corresponding authors. These authors were invited to present their work at the virtual international conference.
Figure 2 illustrates the native countries of the presenters, showing that the presenters were from 10 different countries. Most of the presenters were from India, accounting for 40% of the presenters. This is followed by China, where the four presenters were originally from. The conference presentations and discussions proved immensely beneficial, fostering knowledge exchange among presenters, discussants, and participants. It significantly allowed presenters to refine their manuscripts, leveraging the constructive feedback from discussants and fellow attendees.
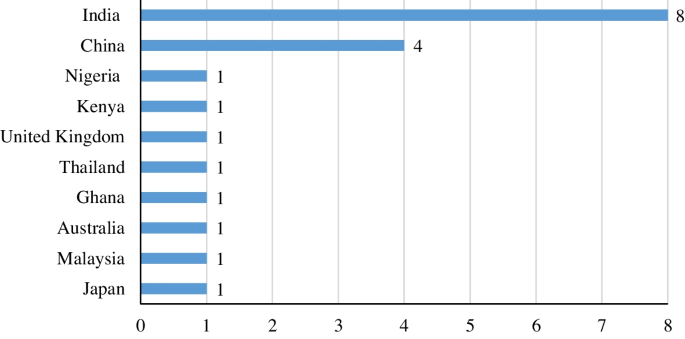
Distributions of selected presentations by corresponding authors' countries
3.2 Keynote speeches
The guest editors invited two keynote speakers to present at the two-day conference. They were Prof. Edward B. Barbier from the Colorado State University in the United States Footnote 2 and Prof. Tatsuyoshi Saijo from Kyoto University of Advanced Science in Japan. Footnote 3
Prof. Edward Barbier gave a speech, “ A Policy Strategy for Climate-Smart Agriculture for Sustainable Rural Development ”, on 10th October 2023. He outlined a strategic approach for integrating CSA into sustainable rural development, particularly within emerging markets and developing economies. He emphasized the necessity of CSA and nature-based solutions (NbS) to tackle food security, climate change, and rural poverty simultaneously. Highlighting the substantial investment needs and the significant role of international and domestic financing, Prof. Barbier advocated reducing harmful subsidies in agriculture, forestry, fishing, and fossil fuel consumption to redirect funds toward CSA and NbS investments. He also proposed the implementation of a tropical carbon tax as an innovative financing mechanism. By focusing on recycling environmentally harmful subsidies and leveraging additional funding through public and private investments, Prof. Barbier’s strategy aims to foster a “win–win” scenario for climate action and sustainable development, underscoring the urgency of adopting comprehensive policies to mobilize the necessary resources for these critical investments.
Prof. Tatsuyoshi Saijo, gave his speech, “ Future Design ”, on 11th October 2023. He explored the significant impact of the Haber–Bosch process on human civilization and the environment. Prof. Saijo identifies this process, which synthetically fixed nitrogen from the atmosphere to create ammonia for fertilizers and other products, as the greatest invention from the twentieth century to the present, fundamentally transforming the world’s food production and enabling the global population and industrial activities to expand dramatically. He also discussed the environmental costs of this technological advancement, including increased greenhouse gas emissions, pollution, and contribution to climate change. Prof. Saijo then introduced the concept of “Future Design” as a method to envision and implement sustainable social systems that consider the well-being of future generations. He presented various experiments and case studies from Japan and beyond, showing how incorporating perspectives of imaginary future generations into decision-making processes can lead to more sustainable choices. By doing so, Prof. Saijo suggested that humanity can address the “Intergenerational Sustainability Dilemma” and potentially avoid the ecological overshoot and collapse faced by past civilizations like Easter Island. He called for a redesign of social systems to activate “futurability”, where individuals derive happiness from decisions that benefit future generations, ultimately aiming to ensure the long-term survival of humankind amidst environmental challenges.
4 Summary of published articles
As a result of a rigorous double-anonymized reviewing process, the special issue accepted 19 articles for publication. These studies have investigated the determinants and impacts of CSA adoption. Table 1 in the Appendix summarises the CSA technologies and practices considered in each paper. Below, we summarize the key findings of the contributions based on their research themes.
4.1 Determinants of CSA adoption among smallholders
4.1.1 influencing factors of csa adoption from literature review.
Investigating the factors influencing farmers’ adoption of CSA practices through a literature review helps offer a comprehensive understanding of the multifaceted determinants of CSA adoption. Investigating the factors influencing farmers’ adoption of CSA practices through a literature review helps provide a comprehensive understanding of the determinants of CSA adoption. Such analyses help identify consistent trends and divergences in how different variables influence farmers’ CSA adoption decisions. In this special issue, we collected four papers that reviewed the literature and synthesized the factors influencing farmers’ decisions to adopt CSA.
Li, Ma and Zhu’s paper, “ A systematic literature review of factors influencing the adoption of climate-smart agricultural practices ”, conducted a systematic review of the literature on the adoption of CSA, summarizing the definitions of CSA practices and the factors that influence farmers’ decisions to adopt these practices. The authors reviewed 190 studies published between 2013 and 2023. They broadly defined CSA practices as “agricultural production-related and unrelated practices that can help adapt to climate change and increase agricultural outputs”. Narrowly, they defined CSA practices as “agricultural production-related practices that can effectively adapt agriculture to climate change and reinforce agricultural production capacity”. The review identified that many factors, including age, gender, education, risk perception, preferences, access to credit, farm size, production conditions, off-farm income, and labour allocation, have a mixed (positive or negative) influence on the adoption of CSA practices. Variables such as labour endowment, land tenure security, access to extension services, agricultural training, membership in farmers’ organizations, support from non-governmental organizations (NGOs), climate conditions, and access to information were consistently found to positively influence CSA practice adoption.
Thottadi and Singh’s paper, “ Climate-smart agriculture (CSA) adaptation, adaptation determinants and extension services synergies: A systematic review ””, reviewed 45 articles published between 2011 and 2022 to explore different CAS practices adopted by farmers and the factors determining their adoption. They found that CSA practices adopted by farmers can be categorized into five groups. These included resilient technologies (e.g., early maturing varieties, drought-resistant varieties, and winter ploughing), management strategies (e.g., nutrient management, water management, and pest management), conservation technologies (e.g., vermicomposting and residue management, drip and sprinkler irrigation, and soil conservation), diversification of income security (e.g., mixed farming, livestock, and crop diversification), and risk mitigation strategies (e.g., contingent planning, adjusting plant dates, and crop insurance). They also found that farmers’ decisions to adopt CSA practices are mainly determined by individual characteristics (age, gender, and education), socioeconomic factors (income and wealth), institutional factors (social group, access to credit, crop insurance, distance, land tenure, and rights), behavioural factors (climate perception, farmers’ perception on CSA, Bookkeeping), and factor endowments (family labour, machinery, and land size). The authors emphasized that extension services improved CSA adaptation by reducing information asymmetry.
Naveen, Datta, Behera and Rahut’s paper, “ Climate-Smart Agriculture in South Asia: Exploring Practices, Determinants, and Contribution to Sustainable Development Goals ”, offered a comprehensive systematic review of 78 research papers on CSA practice adoption in South Asia. Their objective was to assess the current implementation of CSA practices and to identify the factors that influence farmers’ decisions to adopt these practices. They identified various CSA practices widely adopted in South Asia, including climate-resilient seeds, zero tillage, water conservation, rescheduling of planting, crop diversification, soil conservation and water harvesting, and agroforestry. They also identified several key factors that collectively drive farmers’ adoption of CSA practices. These included socioeconomic factors (age, education, livestock ownership, size of land holdings, and market access), institutional factors (access to information and communication technology, availability of credit, input subsidies, agricultural training and demonstrations, direct cash transfers, and crop insurance), and climatic factors (notably rising temperatures, floods, droughts, reduced rainfall, and delayed rainfall).
Wang, Wang and Fu’s paper, “ Can social networks facilitate smallholders’ decisions to adopt Climate-smart Agriculture technologies? A three-level meta-analysis ”, explored the influence of social networks on the adoption of CSA technologies by smallholder farmers through a detailed three-level meta-analysis. This analysis encompassed 26 empirical studies, incorporating 150 effect sizes. The authors reported a modest overall effect size of 0.065 between social networks and the decision-making process for CSA technology adoption, with an 85.21% variance observed among the sample effect sizes. They found that over half (55.17%) of this variance was attributed to the differences in outcomes within each study, highlighting the impact of diverse social network types explored across the studies as significant contributors. They did not identify publication bias in this field. Among the three types of social networks (official-advising network, peer-advising network, and kinship and friendship network), kinship and friendship networks are the most effective in facilitating smallholders’ decisions to adopt climate-smart agriculture technologies.
4.1.2 Socioeconomic factors influencing CSA adoption
We collected three papers highlighting the diverse forms of capital, social responsibility awareness, and effectiveness of digital advisory services in promoting CSA in India, China and Ghana. These studies showcase how digital tools can significantly increase the adoption of CSA technologies, how social responsibility can motivate CSA practices and the importance of various forms of capital in CSA strategy adoption.
Sandilya and Goswami’s paper, “ Effect of different forms of capital on the adoption of multiple climate-smart agriculture strategies by smallholder farmers in Assam, India ”, delved into the determinants behind the adoption of CSA strategies by smallholder farmers in Nagaon district, India, a region notably prone to climate adversities. The authors focused on six types of capital: physical, social, human, financial, natural, and institutional. They considered four CSA practices: alternate land use systems, integrated nutrient management, site-specific nutrient management, and crop diversification. Their analyses encompassed a dual approach, combining a quantitative analysis via a multivariate probit model with qualitative insights from focus group discussions. They found that agricultural cooperatives and mobile applications, both forms of social capital, play a significant role in facilitating the adoption of CSA. In contrast, the authors also identified certain barriers to CSA adoption, such as the remoteness of farm plots from all-weather roads (a component of physical capital) and a lack of comprehensive climate change advisories (a component of institutional capital). Furthermore, the authors highlighted the beneficial impact of irrigation availability (a component of physical capital) on embracing alternate land use and crop diversification strategies. Additionally, the application of indigenous technical knowledge (a component of human capital) and the provision of government-supplied seeds (a component of institutional capital) were found to influence the adoption of CSA practices distinctly.
Ye, Zhang, Song and Li’s paper, “ Social Responsibility Awareness and Adoption of Climate-smart Agricultural Practices: Evidence from Food-based Family Farms in China ”, examined whether social responsibility awareness (SRA) can be a driver for the adoption of CSA on family farms in China. Using multiple linear regression and hierarchical regression analyses, the authors analyzed data from 637 family farms in five provinces (Zhejiang, Shandong, Henan, Heilongjiang, and Hebei) in China. They found that SRA positively impacted the adoption of CSA practice. Pro-social motivation and impression management motivation partially and completely mediated the relationship between SRA and the adoption of CSA practices.
Asante, Ma, Prah and Temoso’s paper, “ Promoting the adoption of climate-smart agricultural technologies among maize farmers in Ghana: Using digital advisory services ”, investigated the impacts of digital advisory services (DAS) use on CSA technology adoption and estimated data collected from 3,197 maize farmers in China. The authors used a recursive bivariate probit model to address the self-selection bias issues when farmers use DAS. They found that DAS notably increases the propensity to adopt drought-tolerant seeds, zero tillage, and row planting by 4.6%, 4.2%, and 12.4%, respectively. The average treatment effect on the treated indicated that maize farmers who use DAS are significantly more likely to adopt row planting, zero tillage, and drought-tolerant seeds—by 38.8%, 24.9%, and 47.2%, respectively. Gender differences in DAS impact were observed; male farmers showed a higher likelihood of adopting zero tillage and drought-tolerant seeds by 2.5% and 3.6%, respectively, whereas female farmers exhibited a greater influence on the adoption of row planting, with a 2.4% probability compared to 1.5% for males. Additionally, factors such as age, education, household size, membership in farmer-based organizations, farm size, perceived drought stress, perceived pest and disease incidence, and geographic location were significant determinants in the adoption of CSA technologies.
4.1.3 Climate-smart villages and CSA adoption
Climate-Smart Villages (CSVs) play a pivotal role in promoting CSA by significantly improving farmers’ access to savings and credit, and the adoption of improved agricultural practices among smallholder farmers. CSV interventions demonstrate the power of community-based financial initiatives in enabling investments in CSA technologies. In this special issue, we collected two insightful papers investigating the relationship between CSVs and the adoption of CSA practices, focusing on India and Kenya.
Villalba, Joshi, Daum and Venus’s paper, “ Financing Climate-Smart Agriculture: A Case Study from the Indo-Gangetic Plains ”, investigated the adoption and financing of CSA technologies in India, focusing on two capital-intensive technologies: laser land levelers and happy seeders. Conducted in Karnal, Haryana, within the framework of Climate-Smart-Villages, the authors combined data from a household survey of 120 farmers, interviews, and focus group discussions with stakeholders like banks and cooperatives. The authors found that adoption rates are high, with 77% for laser land levelers and 52% for happy seeders, but ownership is low, indicating a preference for renting from Custom-Hiring Centers. Farmers tended to avoid formal banking channels for financing, opting instead for informal sources like family, savings, and money lenders, due to the immediate access to credit and avoidance of bureaucratic hurdles. The authors suggested that institutional innovations and governmental support could streamline credit access for renting CSA technologies, emphasizing the importance of knowledge transfer, capacity building, and the development of digital tools to inform farmers about financing options. This research highlights the critical role of financing mechanisms in promoting CSA technology adoption among smallholder farmers in climate-vulnerable regions.
Asseldonk, Oostendorp, Recha, Gathiaka, Mulwa, Radeny Wattel and Wesenbeeck’s paper, “ Distributional impact of climate‑smart villages on access to savings and credit and adoption of improved climate‑smart agricultural practices in the Nyando Basin, Kenya ”, investigated the impact of CSV interventions in Kenya on smallholder farmers’ access to savings, credit, and adoption of improved livestock breeds as part of CSA practices. The authors employed a linear probability model to estimate a balanced panel of 118 farm households interviewed across 2017, 2019, and 2020. They found that CSV interventions significantly increased the adoption of improved livestock breeds and membership in savings and credit groups, which further facilitated the adoption of these improved breeds. The findings highlighted that community-based savings and loan initiatives effectively enable farmers to invest in CSA practices. Although there was a sustained positive trend in savings and loans group membership, the adoption of improved livestock did not show a similar sustained increase. Moreover, the introduction of improved breeds initially benefited larger livestock owners more. However, credit availability was found to reduce this inequity in ownership among participants, making the distribution of improved livestock more equitable within CSVs compared to non-CSV areas, thus highlighting the potential of CSV interventions to reduce disparities in access to improved CSA practices.
4.1.4 Civil-society initiatives and CSA adoption
Civil society initiatives are critical in promoting CSA by embedding its principles across diverse agricultural development projects. These initiatives enhance mitigation, adaptation, and food security efforts for smallholder farmers, demonstrating the importance of varied implementation strategies to address the challenges of CSA. We collected one paper investigating how civil society-based development projects in Asia and Africa incorporated CSA principles to benefit smallholder farmers and local communities.
Davila, Jacobs, Nadeem, Kelly and Kurimoto’s paper, “ Finding climate smart agriculture in civil-society initiatives ”, scrutinized the role of international civil society and non-government organizations (NGOs) in embedding CSA principles within agricultural development projects aimed at enhancing mitigation, adaptation, and food security. Through a thematic analysis of documentation from six projects selected on the basis that they represented a range of geographical regions (East Africa, South, and Southeast Asia) and initiated since 2009, the authors assessed how development programs incorporate CSA principles to support smallholder farmers under CSA’s major pillars. They found heterogeneous application of CSA principles across the projects, underscoring a diversity in implementation strategies despite vague definitions and focuses of CSA. The projects variedly contributed to greening and forests, knowledge exchange, market development, policy and institutional engagement, nutrition, carbon and climate action, and gender considerations.
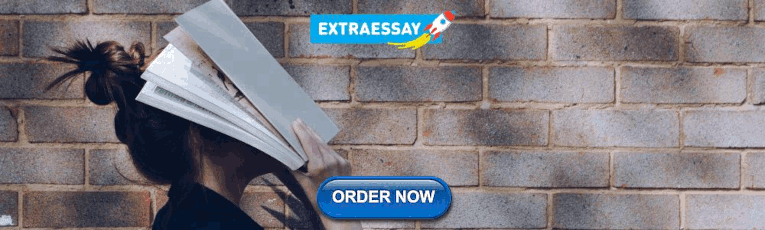
4.2 Impacts of CSA adoption
4.2.1 impacts of csa adoption from literature review.
A comprehensive literature review on the impacts of CSA adoption plays an indispensable role in bridging the gap between theoretical knowledge and practical implementation in the agricultural sector. In this special issue, we collected one paper that comprehensively reviewed the literature on the impacts of CSA adoption from the perspective of the triple win of CSA.
Zheng, Ma and He’s paper, “ Climate-smart agricultural practices for enhanced farm productivity, income, resilience, and Greenhouse gas mitigation: A comprehensive review ”, reviewed 107 articles published between 2013–2023 to distill a broad understanding of the impacts of CSA practices. The review categorized the literature into three critical areas of CSA benefits: (a) the sustainable increase of agricultural productivity and incomes; (b) the adaptation and enhancement of resilience among individuals and agrifood systems to climate change; and (c) the reduction or avoidance of greenhouse gas (GHG) emissions where feasible. The authors found that CSA practices significantly improved farm productivity and incomes and boosted technical and resource use efficiency. Moreover, CSA practices strengthened individual resilience through improved food consumption, dietary diversity, and food security while enhancing agrifood systems’ resilience by mitigating production risks and reducing vulnerability. Additionally, CSA adoption was crucial in lowering Greenhouse gas emissions and fostering carbon sequestration in soils and biomass, contributing to improved soil quality.
4.2.2 Impacts on crop yields and farm income
Understanding the impact of CSA adoption on crop yields and income is crucial for improving agricultural resilience and sustainability. In this special issue, we collected three papers highlighting the transformative potential of CSA practices in boosting crop yields, commercialization, and farm income. One paper focuses on India and the other concentrates on Ghana and Kenya.
Tanti, Jena, Timilsina and Rahut’s paper, “ Enhancing crop yields and farm income through climate-smart agricultural practices in Eastern India ”, examined the impact of CSA practices (crop rotation and integrated soil management practices) on crop yields and incomes. The authors used propensity score matching and the two-stage least square model to control self-selection bias and endogeneity and analyzed data collected from 494 farm households in India. They found that adopting CSA practices increases agricultural income and paddy yield. The crucial factor determining the adoption of CSA practices was the income-enhancing potential to transform subsistence farming into a profoundly ingrained farming culture.
Asante, Ma, Prah and Temoso’s paper, “ Farmers’ adoption of multiple climate-smart agricultural technologies in Ghana: Determinants and impacts on maize yields and net farm income ”, investigated the factors influencing maize growers’ decisions to adopt CSA technologies and estimated the impact of adopting CSA technologies on maize yields and net farm income. They considered three CSA technology types: drought-resistant seeds, row planting, and zero tillage. The authors used the multinomial endogenous switching regression model to estimate the treatment effect of CSA technology adoption and analyze data collected from 3,197 smallholder farmers in Ghana. They found that farmer-based organization membership, education, resource constraints such as lack of land, access to markets, and production shocks such as perceived pest and disease stress and drought are the main factors that drive farmers’ decisions to adopt CSA technologies. They also found that integrating any CSA technology or adopting all three CSA technologies greatly enhances maize yields and net farm income. Adopting all three CSA technologies had the largest impact on maize yields, while adopting row planting and zero tillage had the greatest impact on net farm income.
Mburu, Mburu, Nyikal, Mugera and Ndambi’s paper, “ Assessment of Socioeconomic Determinants and Impacts of Climate-Smart Feeding Practices in the Kenyan Dairy Sector ”, assessed the determinants and impacts of adopting climate-smart feeding practices (fodder and feed concentrates) on yield, milk commercialization, and household income. The authors used multinomial endogenous switching regression to account for self-selection bias arising from observable and unobservable factors and estimated data collected from 665 dairy farmers in Kenya. They found that human and social capital, resource endowment, dairy feeding systems, the source of information about feeding practices, and perceived characteristics were the main factors influencing farmers’ adoption of climate-smart feeding practices. They also found that combining climate-smart feed concentrates and fodder significantly increased milk productivity, output, and dairy income. Climate-smart feed concentrates yielded more benefits regarding dairy milk commercialization and household income than climate-smart fodder.
4.2.3 Impacts on crop yields
Estimating the impacts of CSA adoption on crop yields is crucial for enhancing food security, improving farmers’ resilience to climate change, and guiding policy and investment towards sustainable agricultural development. In this special issue, we collected one paper that provided insights into this field.
Singh, Bisaria, Sinha, Patasaraiya and Sreerag’s paper, “ Developing A Composite Weighted Indicator-based Index for Monitoring and Evaluating Climate-Smart Agriculture in India ”, developed a composite index based on a weighted index to calculate the Climate Smart Score (CSS) at the farm level in India and tested the relationship between computed CSS and farm-level productivity. Through an intensive literature review, the authors selected 34 indicators, which were then grouped into five dimensions for calculating CSS. These dimensions encompassed governance (e.g., land ownership, subsidized fertilizer, and subsidized seeds), farm management practices (mulching, zero tillage farming, and inter-cropping and crop diversification), environment management practices (e.g., not converting forested land into agricultural land and Agroforestry/plantation), energy management (e.g., solar water pump and Biogas digester), and awareness and training (e.g., knowledge of climate-related risk and timely access to weather and agro-advisory). They tested the relationship between CSS and farm productivity using data collected from 315 farmers. They found that improved seeds, direct seeding of rice, crop diversification, zero tillage, agroforestry, crop residue management, integrated nutrient management, and training on these practices were the most popular CSA practices the sampled farmers adopted. In addition, there was a positive association between CSS and paddy, wheat, and maize yields. This finding underscores the beneficial impact of CSA practices on enhancing farm productivity.
4.2.4 Impacts on incomes and benefit–cost ratio
Understanding the income effects of CSA adoption is crucial for assessing its impact on household livelihoods, farm profitability, and income diversity. Quantifying income enhancements would contribute to informed decision-making and investment strategies to improve farming communities’ economic well-being. In this special issue, we collected two papers looking into the effects of CSA adoption on income.
Sang, Chen, Hu and Rahut’s paper, “ Economic benefits of climate-smart agricultural practices: Empirical investigations and policy implications ”, investigated the impact of CSA adoption intensity on household income, net farm income, and income diversity. They used the two-stage residual inclusion model to mitigate the endogeneity of CSA adoption intensity and analyzed the 2020 China Rural Revitalization Survey data. They also used the instrumental-variable-based quantile regression model to investigate the heterogeneous impacts of CSA adoption intensity. The authors found that the education level of the household head and geographical location determine farmers’ adoption intensity of CSAs.CSA practices. The higher levels of CSA adoption were positively and significantly associated with higher household income, net farm income, and income diversity. They also found that while the impact of CSA adoption intensity on household income escalates across selected quantiles, its effect on net farm income diminishes over these quantiles. Additionally, the study reveals that CSA adoption intensity notably enhances income diversity at the 20th quantile only.
Kandulu, Zuo, Wheeler, Dusingizimana and Chagund’s paper, “ Influence of climate-smart technologies on the success of livestock donation programs for smallholder farmers in Rwanda ”, investigated the economic, environmental, and health benefits of integrating CSA technologies —specifically barns and biogas plants—into livestock donation programs in Rwanda. Employing a stochastic benefit–cost analysis from the perspective of the beneficiaries, the authors assessed the net advantages for households that receive heifers under an enhanced program compared to those under the existing scheme. They found that incorporating CSA technologies not only boosts the economic viability of these programs but also significantly increases the resilience and sustainability of smallholder farming systems. More precisely, households equipped with cows and CSA technologies can attain net benefits up to 3.5 times greater than those provided by the current program, with the benefit–cost ratios reaching up to 5. Furthermore, biogas technology reduces deforestation, mitigating greenhouse gas emissions, and lowering the risk of respiratory illnesses, underscoring the multifaceted advantages of integrating such innovations into livestock donation initiatives.
4.2.5 Impacts on factor demand and input substitution
Estimating the impacts of CSA adoption on factor demand and input substitution is key to optimizing resource use, reducing environmental footprints, and ensuring agricultural sustainability by enabling informed decisions on efficient input use and technology adoption. In this field, we collected one paper that enriched our understanding in this field. Understanding the impacts of CSA adoption on factor demand, input substitution, and financing options is crucial for promoting sustainable farming in diverse contexts. In this special issue, we collected one paper comprehensively discussing how CSA adoption impacted factor demand and input substitution.
Kehinde, Shittu, Awe and Ajayi’s paper, “ Effects of Using Climate-Smart Agricultural Practices on Factor Demand and Input Substitution among Smallholder Rice Farmers in Nigeria ”, examined the impacts of agricultural practices with CSA potential (AP-CSAPs) on the demand of labour and other production factors (seed, pesticides, fertilizers, and mechanization) and input substitution. The AP-CSAPs considered in this research included zero/minimum tillage, rotational cropping, green manuring, organic manuring, residue retention, and agroforestry. The authors employed the seemingly unrelated regression method to estimate data collected from 1,500 smallholder rice farmers in Nigeria. The authors found that labour and fertilizer were not easily substitutable in the Nigerian context; increases in the unit price of labour (wage rate) and fertilizer lead to a greater budget allocation towards these inputs. Conversely, a rise in the cost of mechanization services per hectare significantly reduced labour costs while increasing expenditure on pesticides and mechanization services. They also found that most AP-CSAPs were labour-intensive, except for agroforestry, which is labor-neutral. Organic manure and residue retention notably conserved pesticides, whereas zero/minimum tillage practices increased the use of pesticides and fertilizers. Furthermore, the demand for most production factors, except pesticides, was found to be price inelastic, indicating that price changes do not significantly alter the quantity demanded.
4.3 Progress of research on CSA
Understanding the progress of research on CSA is essential for identifying and leveraging technological innovations—like greenhouse advancements, organic fertilizer products, and biotechnological crop improvements—that support sustainable agricultural adaptation. This knowledge enables the integration of nature-based strategies, informs policy, and underscores the importance of international cooperation in overcoming patent and CSA adoption challenges to ensure global food security amidst climate change. We collected one paper in this field.
Tey, Brindal, Darham and Zainalabidin’s paper, “ Adaptation technologies for climate-smart agriculture: A patent network analysis ”, delved into the advancements in technological innovation for agricultural adaptation within the context of CSA by analyzing global patent databases. The authors found that greenhouse technologies have seen a surge in research and development (R&D) efforts, whereas composting technologies have evolved into innovations in organic fertilizer products. Additionally, biotechnology has been a significant focus, aiming to develop crop traits better suited to changing climate conditions. A notable emergence is seen in resource restoration innovations addressing climate challenges. These technologies offer a range of policy options for climate-smart agriculture, from broad strategies to specific operational techniques, and pave the way for integration with nature-based adaptation strategies. However, the widespread adoption and potential impact of these technologies may be hindered by issues related to patent ownership and the path dependency this creates. Despite commercial interests driving the diffusion of innovation, international cooperation is clearly needed to enhance technology transfer.
5 Summary of key policy implications
The collection of 19 papers in this special issue sheds light on the critical aspects of promoting farmers’ adoption of CSA practices, which eventually help enhance agricultural productivity and resilience, reduce greenhouse gas emissions, improve food security and soil health, offer economic benefits to farmers, and contribute to sustainable development and climate change adaptation. We summarize and discuss the policy implications derived from this special issue from the following four aspects:
5.1 Improving CSA adoption through extension services
Extension services help reduce information asymmetry associated with CSA adoption and increase farmers’ awareness of CSA practices’ benefits, costs, and risks while addressing their specific challenges. Therefore, the government should improve farmers’ access to extension services. These services need to be inclusive and customized to meet the gender-specific needs and the diverse requirements of various farming stakeholders. Additionally, fostering partnerships between small and medium enterprises and agricultural extension agents is crucial for enhancing the local availability of CSA technologies. Government-sponsored extension services should prioritize equipping farmers with essential CSA skills, ensuring they are well-prepared to implement these practices. This structured approach will streamline the adoption process and significantly improve the effectiveness of CSA initiatives.
5.2 Facilitating CSA adoption through farmers’ organizations
Farmers’ organizations, such as village cooperatives, farmer groups, and self-help groups, play a pivotal role in facilitating farmers’ CSA adoption and empowering rural women’s adoption through effective information dissemination and the use of agricultural apps. Therefore, the government should facilitate the establishment and development of farmers’ organizations and encourage farmers to join those organizations as members. In particular, the proven positive impacts of farmer-based organizations (FBOs) highlight the importance of fostering collaborations between governments and FBOs. Supporting farmer cooperatives with government financial and technical aid is essential for catalyzing community-driven climate adaptation efforts. Furthermore, the successful use of DAS in promoting CSA adoption underscores the need for government collaboration with farmer groups to expand DAS utilization. This includes overcoming usage barriers and emphasizing DAS’s reliability as a source of climate-smart information. By establishing and expanding digital hubs and demonstration centres in rural areas, farmers can access and experience DAS technologies firsthand, leading to broader adoption and integration into their CSA practices.
5.3 Enhancing CSA adoption through agricultural training and education
Agricultural training and education are essential in enhancing farmers’ adoption of CSA. To effectively extend the reach of CSA practices, the government should prioritize expanding rural ICT infrastructure investments and establish CSA training centres equipped with ICT tools that target key demographics such as women and older people, aiming to bridge the digital adoption gaps. Further efforts should prioritize awareness and training programs to ensure farmers can access weather and agro-advisory services. These programs should promote the use of ICT-based tools through collaborations with technology providers and include regular CSA training and the establishment of demonstration fields that showcase the tangible benefits of CSA practices.
Education plays a vital role in adopting CAPs, suggesting targeted interventions such as comprehensive technical training to assist farmers with limited educational backgrounds in understanding the value of CAPs, ultimately improving their adoption rates. Establishing robust monitoring mechanisms is crucial to maintaining farmer engagement and success in CSA practices. These mechanisms will facilitate the ongoing adoption and evaluation of CSA practices and help educate farmers on the long-term benefits. Centralizing and disseminating information about financial products and subsidies through various channels, including digital platforms tailored to local languages and contexts, is essential. This approach helps educate farmers on financing options and requirements, supporting the adoption of CSA technologies among smallholder farmers. Lastly, integrating traditional and local knowledge with scientific research and development can effectively tailor CSA initiatives. This integration requires the involvement of a range of stakeholders, including NGOs, to navigate the complexities of CSA and ensure that interventions are effective but also equitable and sustainable. The enhanced capacity of institutions and their extension teams will further support these CSA initiatives.
5.4 Promoting CSA adoption through establishing social networks and innovating strategies
The finding that social networks play a crucial role in promoting the adoption of CSA suggests that implementing reward systems to incentivize current CSA adopters to advocate for climate-smart practices within their social circles could be an effective strategy to promote CSA among farmers. The evidence of a significant link between family farms’ awareness of social responsibility and their adoption of CSA highlights that governments should undertake initiatives, such as employing lectures and pamphlets, to enhance family farm operating farmers’ understanding of social responsibility. The government should consider introducing incentives that foster positive behavioural changes among family farms to cultivate a more profound commitment to social responsibility. The government can also consider integrating social responsibility criteria into the family farm awards and recognition evaluation process. These measures would encourage family farms to align their operations with broader social and environmental goals, promoting CSA practices.
Combining traditional incentives, such as higher wages and access to improved agricultural inputs, with innovative strategies like community-driven development for equipment sharing and integrating moral suasion with Payment for Ecosystem Services would foster farmers’ commitment to CSA practices. The finding that technological evolution plays a vital role in shaping adaptation strategies for CSA highlights the necessity for policy instruments that not only leverage modern technologies but also integrate them with traditional, nature-based adaptation strategies, enhancing their capacity to address specific CSA challenges. Policymakers should consider the region’s unique socioeconomic, environmental, and geographical characteristics when promoting CSA, moving away from a one-size-fits-all approach to ensure the adaptability and relevance of CSA practices across different agricultural landscapes. They should foster an environment that encourages the reporting of all research outcomes to develop evidence-based policies that are informed by a balanced view of CSA’s potential benefits and limitations.
Finally, governance is critical in creating an enabling environment for CSA adoption. Policies should support CSA practices and integrate environmental sustainability to enhance productivity and ecosystem health. Development programs must offer financial incentives, establish well-supported voluntary schemes, provide robust training programs, and ensure the wide dissemination of informational tools. These measures are designed to help farmers integrate CAPs into their operations, improving economic and operational sustainability.
6 Concluding remarks
This special issue has provided a wealth of insights into the adoption and impact of CSA practices across various contexts, underscoring the complexity and multifaceted nature of CSA implementation. The 19 papers in this special issue collectively emphasize the importance of understanding local conditions, farmer characteristics, and broader socioeconomic and institutional factors that influence CSA adoption. They highlight the crucial role of extension services, digital advisory services, social responsibility awareness, and diverse forms of capital in facilitating the adoption of CSA practices. Moreover, the findings stress the positive impact of CSA on farm productivity, income diversification, and resilience to climate change while also pointing out the potential for CSA practices to address broader sustainability goals.
Significantly, the discussions underline the need for policy frameworks that are supportive and adaptive, tailored to specific regional and local contexts to promote CSA adoption effectively. Leveraging social networks, enhancing access to financial products and mechanisms, and integrating technological innovations with traditional agricultural practices are vital strategies for scaling CSA adoption. Furthermore, the discussions advocate for a balanced approach that combines economic incentives with moral persuasion and community engagement to foster sustainable agricultural practices.
These comprehensive insights call for concerted efforts from policymakers, researchers, extension agents, and the agricultural community to foster an enabling environment for CSA. Such an environment would support knowledge exchange, financial accessibility, and the adoption of CSA practices that contribute to the resilience and sustainability of agricultural systems in the face of climate change. As CSA continues to evolve, future research should focus on addressing the gaps identified, exploring innovative financing and technology dissemination models, and assessing the long-term impacts of CSA practices on agricultural sustainability and food security. This special issue lays the groundwork for further exploration and implementation of CSA practices, aiming to achieve resilient, productive, and sustainable agricultural systems worldwide and contribute to the achievements of the United Nations Sustainable Development Goals.
Data availability
No new data were created or analyzed during this study. Data sharing is not applicable to this article.
The conference agenda, biographies of the speakers, and conference recordings are available at the ADBI website: https://www.adb.org/news/events/climate-smart-agriculture-adoption-impacts-and-implications-for-sustainable-development .
Profile of Prof. Edward B. Barbie: http://www.edwardbbarbier.com/ .
Google Scholar of Prof. Tatsuyoshi Saijo: https://scholar.google.co.nz/citations?user=ju72inUAAAAJ&hl=en&oi=ao .
Akter A, Geng X, EndelaniMwalupaso G et al (2022) Income and yield effects of climate-smart agriculture (CSA) adoption in flood prone areas of Bangladesh: farm level evidence. Clim Risk Manag 37:100455. https://doi.org/10.1016/j.crm.2022.100455
Article Google Scholar
Alam MF, Sikka AK (2019) Prioritizing land and water interventions for climate-smart villages. Irrig Drain 68:714–728. https://doi.org/10.1002/ird.2366
Amadu FO, McNamara PE, Miller DC (2020) Yield effects of climate-smart agriculture aid investment in southern Malawi. Food Policy 92:101869. https://doi.org/10.1016/j.foodpol.2020.101869
Arora NK (2019) Impact of climate change on agriculture production and its sustainable solutions. Environ Sustain 2:95–96. https://doi.org/10.1007/s42398-019-00078-w
Aryal JP, Rahut DB, Maharjan S, Erenstein O (2018) Factors affecting the adoption of multiple climate-smart agricultural practices in the Indo-Gangetic Plains of India. Nat Resour Forum 42:141–158. https://doi.org/10.1111/1477-8947.12152
Aryal JP, Farnworth CR, Khurana R et al (2020a) Does women’s participation in agricultural technology adoption decisions affect the adoption of climate-smart agriculture? Insights from Indo-Gangetic Plains of India. Rev Dev Econ 24:973–990. https://doi.org/10.1111/rode.12670
Aryal JP, Rahut DB, Sapkota TB et al (2020b) Climate change mitigation options among farmers in South Asia. Environ Dev Sustain 22:3267–3289. https://doi.org/10.1007/s10668-019-00345-0
Belay AD, Kebede WM, Golla SY (2023) Determinants of climate-smart agricultural practices in smallholder plots: evidence from Wadla district, northeast Ethiopia. Int J Clim Chang Strateg Manag. https://doi.org/10.1108/IJCCSM-06-2022-0071
Brown T (2016) Civil society organizations for sustainable agriculture: negotiating power relations for pro-poor development in India. Agroecol Sustain Food Syst 40:381–404. https://doi.org/10.1080/21683565.2016.1139648
Diro S, Tesfaye A, Erko B (2022) Determinants of adoption of climate-smart agricultural technologies and practices in the coffee-based farming system of Ethiopia. Agric Food Secur 11:1–14. https://doi.org/10.1186/s40066-022-00385-2
FAO (2013) Climate smart agriculture sourcebook. Food and agriculture organization of the United Nations
García de Jalón S, Silvestri S, Barnes AP (2017) The potential for adoption of climate smart agricultural practices in Sub-Saharan livestock systems. Reg Environ Chang 17:399–410. https://doi.org/10.1007/s10113-016-1026-z
Hariharan VK, Mittal S, Rai M et al (2020) Does climate-smart village approach influence gender equality in farming households? A case of two contrasting ecologies in India. Clim Chang 158:77–90. https://doi.org/10.1007/s10584-018-2321-0
Israel MA, Amikuzuno J, Danso-Abbeam G (2020) Assessing farmers’ contribution to greenhouse gas emission and the impact of adopting climate-smart agriculture on mitigation. Ecol Process 9. https://doi.org/10.1186/s13717-020-00249-2
Jamil I, Jun W, Mughal B et al (2021) Does the adaptation of climate-smart agricultural practices increase farmers’ resilience to climate change? Environ Sci Pollut Res 28:27238–27249. https://doi.org/10.1007/s11356-021-12425-8
Kang Y, Khan S, Ma X (2009) Climate change impacts on crop yield, crop water productivity and food security - a review. Prog Nat Sci 19:1665–1674. https://doi.org/10.1016/j.pnsc.2009.08.001
Kangogo D, Dentoni D, Bijman J (2021) Adoption of climate-smart agriculture among smallholder farmers: does farmer entrepreneurship matter? Land Use Policy 109:105666. https://doi.org/10.1016/j.landusepol.2021.105666
Keil A, Krishnapriya PP, Mitra A et al (2021) Changing agricultural stubble burning practices in the Indo-Gangetic plains: is the Happy Seeder a profitable alternative? Int J Agric Sustain 19:128–151. https://doi.org/10.1080/14735903.2020.1834277
Khatri-Chhetri A, Regmi PP, Chanana N, Aggarwal PK (2020) Potential of climate-smart agriculture in reducing women farmers’ drudgery in high climatic risk areas. Clim Chang 158:29–42. https://doi.org/10.1007/s10584-018-2350-8
Kifle T, Ayal DY, Mulugeta M (2022) Factors influencing farmers adoption of climate smart agriculture to respond climate variability in Siyadebrina Wayu District, Central Highland of Ethiopia. Clim Serv 26:100290. https://doi.org/10.1016/j.cliser.2022.100290
Kpadonou RAB, Owiyo T, Barbier B et al (2017) Advancing climate-smart-agriculture in developing drylands: joint analysis of the adoption of multiple on-farm soil and water conservation technologies in West African Sahel. Land Use Policy 61:196–207. https://doi.org/10.1016/j.landusepol.2016.10.050
Läderach P, Ramirez-Villegas J, Navarro-Racines C et al (2017) Climate change adaptation of coffee production in space and time. Clim Chang 141:47–62. https://doi.org/10.1007/s10584-016-1788-9
Makate C, Makate M, Mango N, Siziba S (2019) Increasing resilience of smallholder farmers to climate change through multiple adoption of proven climate-smart agriculture innovations. Lessons from Southern Africa. J Environ Manag 231:858–868. https://doi.org/10.1016/j.jenvman.2018.10.069
Martey E, Etwire PM, Mockshell J (2021) Climate-smart cowpea adoption and welfare effects of comprehensive agricultural training programs. Technol Soc 64:101468. https://doi.org/10.1016/j.techsoc.2020.101468
McNunn G, Karlen DL, Salas W et al (2020) Climate smart agriculture opportunities for mitigating soil greenhouse gas emissions across the U.S. corn-belt. J Clean Prod 268:122240. https://doi.org/10.1016/j.jclepro.2020.122240
Article CAS Google Scholar
Mirón IJ, Linares C, Díaz J (2023) The influence of climate change on food production and food safety. Environ Res 216:114674. https://doi.org/10.1016/j.envres.2022.114674
Omotoso AB, Omotayo AO (2024) Enhancing dietary diversity and food security through the adoption of climate-smart agricultural practices in Nigeria: a micro level evidence. Environ Dev Sustain. https://doi.org/10.1007/s10668-024-04681-8
Radeny M, Rao EJO, Ogada MJ et al (2022) Impacts of climate-smart crop varieties and livestock breeds on the food security of smallholder farmers in Kenya. Food Secur 14:1511–1535. https://doi.org/10.1007/s12571-022-01307-7
Santalucia S (2023) Nourishing the farms , nourishing the plates : association of climate ‐ smart agricultural practices with household dietary diversity and food security in smallholders. 1–21. https://doi.org/10.1002/agr.21892
Shikuku KM, Valdivia RO, Paul BK et al (2017) Prioritizing climate-smart livestock technologies in rural Tanzania: a minimum data approach. Agric Syst 151:204–216. https://doi.org/10.1016/j.agsy.2016.06.004
Tabe-Ojong MP, Aihounton GBD, Lokossou JC (2023) Climate-smart agriculture and food security: cross-country evidence from West Africa. Glob Environ Chang 81:102697. https://doi.org/10.1016/j.gloenvcha.2023.102697
Tran NLD, Rañola RF, Ole Sander B et al (2020) Determinants of adoption of climate-smart agriculture technologies in rice production in Vietnam. Int J Clim Chang Strateg Manag 12:238–256. https://doi.org/10.1108/IJCCSM-01-2019-0003
United Nations (2021) Economic and social council: population, food security, nutrition and sustainable development. Oxford Handb United Nations 1–20. https://www.un.org/development/desa/pd/sites/www.un.org.development.desa.pd/files/undesa_pd_2021_e_cn.9_2021_2_advanceunedited.pdf . Accessed 1 Feb 2024
Vatsa P, Ma W, Zheng H, Li J (2023) Climate-smart agricultural practices for promoting sustainable agrifood production: yield impacts and implications for food security. Food Policy 121:102551. https://doi.org/10.1016/j.foodpol.2023.102551
Waaswa A, OywayaNkurumwa A, MwangiKibe A, NgenoKipkemoi J (2022) Climate-Smart agriculture and potato production in Kenya: review of the determinants of practice. Clim Dev 14:75–90. https://doi.org/10.1080/17565529.2021.1885336
Waters-Bayer A, Kristjanson P, Wettasinha C et al (2015) Exploring the impact of farmer-led research supported by civil society organizations. Agric Food Secur 4:1–7. https://doi.org/10.1186/s40066-015-0023-7
Zakaria A, Azumah SB, Appiah-Twumasi M, Dagunga G (2020) Adoption of climate-smart agricultural practices among farm households in Ghana: the role of farmer participation in training programmes. Technol Soc 63:101338. https://doi.org/10.1016/j.techsoc.2020.101338
Zhou X, Ma W, Zheng H et al (2023) Promoting banana farmers’ adoption of climate-smart agricultural practices: the role of agricultural cooperatives. Clim Dev:1–10. https://doi.org/10.1080/17565529.2023.2218333
Zizinga A, Mwanjalolo J-GM, Tietjen B et al (2022) Climate change and maize productivity in Uganda: simulating the impacts and alleviation with climate smart agriculture practices. Agric Syst 199:103407. https://doi.org/10.1016/j.agsy.2022.103407
Download references
Acknowledgements
We want to thank all the authors who have submitted papers for the special issue and the reviewers who reviewed manuscripts on time. We acknowledge the Asian Development Bank Institute (ADBI) for supporting the virtual international conference on “ Climate-smart Agriculture: Adoption, Impacts, and Implications for Sustainable Development ” held on 10-11 October 2023. Special thanks to the invited keynote speakers, Prof. Edward Barbier and Prof. Tatsuyoshi Saijo. Finally, we would like to express our thanks, gratitude, and appreciation to the session chairs (Prof. Anita Wreford, Prof. Jianjun Tang, Prof. Alan Renwick, and Assoc. Prof. Sukanya Das), ADBI supporting team (Panharoth Chhay, Mami Nomoto, Mami Yoshida, and Raja Rajendra Timilsina), and discussants who made substantial contributions to the conference.
Open Access funding enabled and organized by CAUL and its Member Institutions
Author information
Authors and affiliations.
Department of Global Value Chains and Trade, Faculty of Agribusiness and Commerce, Lincoln University, Christchurch, New Zealand
Asian Development Bank Institute, Tokyo, Japan
Dil Bahadur Rahut
You can also search for this author in PubMed Google Scholar
Corresponding author
Correspondence to Wanglin Ma .
Ethics declarations
Conflict of interests.
The authors declare no known interests related to their submitted manuscript.
Additional information
Publisher's note.
Springer Nature remains neutral with regard to jurisdictional claims in published maps and institutional affiliations.
This article is part of the Topical Collection on Climate-smart Agriculture
See Table 1 .
Rights and permissions
Open Access This article is licensed under a Creative Commons Attribution 4.0 International License, which permits use, sharing, adaptation, distribution and reproduction in any medium or format, as long as you give appropriate credit to the original author(s) and the source, provide a link to the Creative Commons licence, and indicate if changes were made. The images or other third party material in this article are included in the article's Creative Commons licence, unless indicated otherwise in a credit line to the material. If material is not included in the article's Creative Commons licence and your intended use is not permitted by statutory regulation or exceeds the permitted use, you will need to obtain permission directly from the copyright holder. To view a copy of this licence, visit http://creativecommons.org/licenses/by/4.0/ .
Reprints and permissions
About this article
Ma, W., Rahut, D.B. Climate-smart agriculture: adoption, impacts, and implications for sustainable development. Mitig Adapt Strateg Glob Change 29 , 44 (2024). https://doi.org/10.1007/s11027-024-10139-z
Download citation
Received : 08 April 2024
Accepted : 17 April 2024
Published : 29 April 2024
DOI : https://doi.org/10.1007/s11027-024-10139-z
Share this article
Anyone you share the following link with will be able to read this content:
Sorry, a shareable link is not currently available for this article.
Provided by the Springer Nature SharedIt content-sharing initiative
- Climate-smart agriculture
- Influencing factors
- Crop yields
- Farm incomes
- Research progress
- Development programs
- Find a journal
- Publish with us
- Track your research

An official website of the United States government
The .gov means it’s official. Federal government websites often end in .gov or .mil. Before sharing sensitive information, make sure you’re on a federal government site.
The site is secure. The https:// ensures that you are connecting to the official website and that any information you provide is encrypted and transmitted securely.
- Publications
- Account settings
Preview improvements coming to the PMC website in October 2024. Learn More or Try it out now .
- Advanced Search
- Journal List
- Sensors (Basel)

A Systematic Review of IoT Solutions for Smart Farming
Emerson navarro.
1 School of Technology and Management, Computer Science and Communication Research Centre, Polytechnic Institute of Leiria, Campus 2, Morro do Lena—Alto do Vieiro, Apartado 4163, 2411-901 Leiria, Portugal; tp.airielpi.ym@3320812 (E.N.); [email protected] (N.C.)
António Pereira
2 INOV INESC Inovação, Institute of New Technologies, Leiria Office, Campus 2, Morro do Lena—Alto do Vieiro, Apartado 4163, 2411-901 Leiria, Portugal
The world population growth is increasing the demand for food production. Furthermore, the reduction of the workforce in rural areas and the increase in production costs are challenges for food production nowadays. Smart farming is a farm management concept that may use Internet of Things (IoT) to overcome the current challenges of food production. This work uses the preferred reporting items for systematic reviews (PRISMA) methodology to systematically review the existing literature on smart farming with IoT. The review aims to identify the main devices, platforms, network protocols, processing data technologies and the applicability of smart farming with IoT to agriculture. The review shows an evolution in the way data is processed in recent years. Traditional approaches mostly used data in a reactive manner. In more recent approaches, however, new technological developments allowed the use of data to prevent crop problems and to improve the accuracy of crop diagnosis.
1. Introduction
The challenge of food production in the 21st century is an increasingly relevant theme as population growth increases year after year. It is estimated that by 2050 the world will have between 9.4 and 10.1 billion people who depend on the world’s biodiversity to live, increasing the demand for dedicated food production areas—specifically for planting and livestock [ 1 ]. Environmental changes caused by human beings could potentially cause conditions in which the development of new crops is not possible. Likewise, the growing urbanization decreases labor in areas typically involved in food production, increases costs and reduces the productive capacity of the sector [ 2 ]. In view of this, smart farming is a new farm management concept that employs techniques and technologies at various levels and scales of agricultural production, enabling to overcome the challenges in food production demands and reduction in the workforce [ 3 , 4 ]. For example smart farming may use different types of sensors to collect data (e.g., temperature, humidity, light, pressure, presence, etc.), communication networks to send and receive data which is then managed and analyzed by management information systems and data analysis solutions [ 5 ]. This system of interconnected devices is commonly referred to as internet of things (IoT) [ 6 ]. The use of the data provided by smart farming helps boosting productivity and minimizing waste by allowing necessary actions to be carried out at the right time, quantity and place [ 7 ].
Moreover, recent technological developments in areas relevant to IoT facilitates an easier adoption and use of smart farming with IoT [ 8 ]. Such technological developments include, for example, network communications, reduction of hardware size, optimization of power consumption and devices cheapening. Furthermore, the World’s largest agricultural producers are promoting the usage of IoT in smart farming by creating incentive programs and public policies to fund research and training [ 9 , 10 ].
Several reviews have been published on IoT solutions for smart agriculture in recent years which denotes that this research field is being constantly receiving new contributions and constant improvement. Existing reviews usually focus on topics like network technologies, embedded system platforms, unmanned aerial vehicles (UAV) devices, network protocols and topologies and enabling cloud platforms. For instance [ 11 ] focuses on arable farming from year 2008 to 2018 and surveys communication technologies and protocols, the generation and analysis of data, IoT architectures and applications and highlights the challenges and future directions related with the application of IoT technologies on arable farming. Review [ 12 ] presents technologies used for communication and data collection within IoT solutions for smart farming as well as several cloud-based IoT platforms used for IoT solutions for smart farming. Additionally, authors present several use cases for the identified applications of IoT for smart farming. Review [ 13 ] presents a systematic review of papers published between 2006 and 2016 and classifies these papers in application domains, such as monitoring, controlling, logistic and prediction. Within these domains, authors also identified the data visualization strategies and the technologies used for communication and edge computing. Review [ 14 ] presents a review of papers published between 2010 and 2016. The authors rely on an IoT architecture with three layers (perception, network, application) to analyze the reviewed papers in terms of perception devices, network technologies and applications. With this, they identify embedded platforms and communication technologies used in IoT solutions as well as the application of such IoT solutions. Finally, [ 15 ] reviewed papers published between 2010 and 2015 and presents a state-of-the-art of IoT solutions for smart farming and smart agriculture. Authors relied on an IoT architecture with three layers (perception, network and application) to analyze the application of sensor and actuator devices and communication technologies within several farming domains, such as agriculture, food consumption, livestock farming, among others.
This study aims to identify how IoT is used with smart farming by (i) presenting a systematic review of the state of the art of the IoT adoption in smart agriculture and (ii) identifying the most commonly used hardware, platforms, network protocols and technologies and their applicability to the proposed solutions. To give a more up-to-date view to the reader about the smart agriculture research field, this review article surveys literature until year 2020 and evaluates the acceptance of (new) IT technologies, such as big data, computer vision, artificial intelligence, blockchain and fuzzy logic in the smart agriculture field. This review complements the analysis already carried out by the academy by performing a comprehensive review of the state-of-the-art of IoT in smart farming. To do so, we rely on an IoT architecture with 4 layers (perception, network, processing and application) to identify the technologies that enable IoT in smart farming. From this approach, the present work identifies an increasing use of modern techniques and technologies for processing the collected data in recent years, such as big data, machine learning, computer vision and blockchain. Moreover, this review contributes to the existent literature by reporting a change in the treatment of data in recent works: while previous work showed that the majority of decision support systems used simple processing mechanisms to handle data collected in real-time, more recent work showed an increasing number of management systems that use complementary technologies that rely on cloud and big data computing for processing large amounts of data. In terms of research domain, this article addresses the agriculture economic sector, including indoor and outdoor agriculture (greenhouse, hydroponics, crop beds, pots, orchards, permanent crops, and arable lands). However, the livestock farming (both indoors and outdoors) was left out because, due to its specificities like impact on nature, associated mobility, different species (mammals, birds, fishing) deserves a separated and specific review.
For this purpose, the remaining work is organized as follows: Section 2 presents the introductory concepts discussed in this study, Section 3 presents the methodology followed for the systematic review, Section 4 details the results obtained, Section 5 presents the considerations and Section 6 presents the conclusions.
2. Concepts
To better understand the questions related to the use of IoT in agriculture during the 21st century, it is important go deeper in some fundamental concepts.
2.1. Smart Farming
Smart farming can be defined as the application of supplementary technologies to agricultural production techniques to help minimize waste and boost productivity.
For such, intelligent farms use technological resources that help in various stages of the production process, such as monitoring of plantations, soil management, irrigation, pest control, delivery tracking, etc. [ 16 ]. Such resources include, among others, temperature, luminosity, humidity, pressure, ground chemical concentration, unmanned flying equipment, video cameras, agricultural information management systems, global positioning systems (GPS) and communication networks [ 17 ].
The integration of technological resources into the agricultural production process is a relevant issue. From an economic point of view, the precision agriculture market is expected to have a revenue of US$10 billion in 2023 [ 18 ] with opportunities for technology providers, agricultural equipment and machinery providers, producers and others involved in this business. In addition, smart farms are expected to be able to optimize food production by improving the application of nutrients to the soil, reducing the amount of pesticides and water consumption in irrigation [ 19 ].
2.2. Internet of Things
IoT can be understood as a network of interconnected intelligent devices capable of communicating with each other, generating relevant data about the environment in which they operate. Thus, virtually any device capable of establishing a connection to the Internet can be considered a “thing” within the context of IoT, such as household appliances, electronics, furniture, agricultural or industrial machinery and even people [ 6 ].
Although the idea of IoT is not new, its adoption has increased in recent years, mainly thanks to the development of technologies that support it, among which the improvement of hardware—with the consequent reduction in size and power consumption—improvements in connectivity with the Internet and between devices via wireless connection, cloud computing, artificial intelligence and big data. All these technological components help build a network of devices capable of sharing data and information, as well as acting actively based on network inputs [ 20 ].
According to [ 21 ], the architecture of IoT systems is similar to the architecture of other computer systems but it must take into account the particularities of this paradigm, such as the limited computing capabilities of the devices, identification, detection and control of remote objects.
2.3. Intelligent Agriculture
The IoT architecture proposed in [ 22 ] and [ 23 ], and shown in Figure 1 , presents four layers, considering the main components of an IoT solution: devices, network, services and application.

IoT solution architecture that includes 4 layers: perception, transport, processing and application, based on [ 22 , 23 ].
The perception layer relates to the physical devices in the solution and how they interact with each other and with the transport layer. These devices are responsible for collecting data, enabling the communication of the so-called “things”. This can be done by using commercial solutions—such as UAV devices [ 24 ], sensor nodes [ 25 ]—or new devices, developed with components like sensors and single-board computers (SBC)—such as Arduino or Raspberry Pi—to build sensor nodes and communication gateways. Sensor nodes, for example, are used to monitor plant diseases [ 26 ], control environmental variables in greenhouses [ 27 ] and external crops [ 28 , 29 , 30 ], among others. The interaction between the devices that belong to the perception layer and the services that belongs to the processing layer is intermediated by the transport layer and might occur in several ways, such as through the direct communication between sensor nodes and a data processing platform (such as FIWARE [ 31 ], SmartFarmNet [ 32 ] and Thinger.io [ 33 ]) or through a gateway that, besides intermediating the communication between sensor nodes and the internet, acts as a data hub and enables the communication between network protocols that are originally incompatible, such as ZigBee and the Internet [ 28 ].
The transport layer refers to the network and transport capabilities such as network and application protocols [ 22 ]. IoT solutions use network protocols to enable communication between the perception layer and the processing layer. These protocols are used to create the so-called wireless sensor networks (WSN), that allows wireless communication between sensor nodes and applications. Each protocol has important characteristics, such as the data exchange rate, range and power consumption. Based on these characteristics such protocols can be classified in short-range, cellular networks and long-range [ 34 ]. Protocols for short-range networks (e.g., Bluetooth, ZigBee and Wi-Fi) enable communication in short distances. According to [ 34 ], usually such protocols have a high data transmission rate and low power consumption. Therefore, they are used for the communication between devices that are near each other. Protocols for cellular networks (e.g., GPRS, 3G) enable communication in long distances and with a high data transmission rate. However, they have a high power consumption [ 35 ] and costs for licensing [ 34 ]. Protocols for long-range networks (e.g., LoRaWAN and Sigfox) enable communication in very long distances [ 34 ]. These protocols are used to establish the low power wide area networks (LPWAN) due to the fact that they have a low power consumption [ 36 ]. However, the data transmission rate of these protocols is low. Therefore, these protocols are appropriate for use when the solution needs to transmit a few amounts of data in very long distances. Table 1 presents the characteristics of some network technologies used for IoT.
Examples of network technologies used in IoT [ 34 ].
As shown in Table 1 there is a trade-off between coverage, data rate and energy consumption. Considering the technologies for star networks presented in Table 1 , it is possible to notice that energy consumption is higher in technologies with a high data rate and short coverage. On the other hand, LoRa has a small data rate but a large coverage and low power consumption. These questions are especially relevant when considering agriculture because agricultural scenarios often have limited or no energy supply and obstacles for wireless communication.
Different topologies can be used for implementing networks, such as tree, star and mesh. Star networks have a central node and several peripheral nodes. The communication in such topology occurs as follows: peripheral nodes send data directly to the central node. The central node can implement capabilities for routing messages and communicating through multiple network protocols [ 23 ]. Tree networks are composed of router nodes and leaf nodes. Such networks can be understood as a cluster of star networks. Within each cluster, leaf nodes send messages their father node. In mesh networks, in theory, each node can be a router with rerouting capability. Thus, messages in mesh networks are routed hop by hop until reaching the final destination [ 37 ].
Data is sent to the destination through application protocols such as the message queueing telemetry transport (MQTT) [ 38 ] or the constrained application protocol (CoAP) [ 39 ]. MQTT is an open-source messaging protocol that enables communication between constrained devices and in unreliable networks [ 40 ]. MQTT runs over TPC/IP or similar protocols (e.g., Bluetooth) [ 41 ], which makes the use of MQTT appropriate for different IoT solutions. The MQTT protocol, which is based on the publish/subscribe architecture, allows communication between devices to take place in the following way. First, devices publish messages that are structured in topics on a message broker. Then, other devices read these messages by subscribing to relevant topics on the message broker. These topics allow the organization of messages based on categories, subjects, etc. [ 42 ]. The use of MQTT for communication between device allows low coupling between the device that publishes the message and the devices that listen to the messages, the so-called “one-to-many” communication [ 38 ]. Like MQTT, CoAP is a communication protocol optimized for constrained devices and unreliable networks. However, CoAP messages are interchanged using User Datagram Protocol (UDP) and the CoAP protocol is based on the client/server architecture. This architecture requires that a connection is established between devices before any messages are transmitted [ 38 ]. For this reason, communication using CoAP works in the following way. First, the device that sends messages needs to know the address of each device that is expected to receive messages. Then, messages are sent over UDP to the specified address. Due to the use of UDP, CoAP messages are classified accordingly to the required status of confirmation of receival, for example, confirmable or non-confirmable [ 39 ]. The CoAP protocol does not implement a structure of topics for messages. However, a similar approach can be implemented using application programming interface (API). Nonetheless, the use of CoAP creates a high coupling between the device that sends messages and the device that is expected to receive messages, as the communication is “one-to-one” [ 39 ].
The processing layer comprises data storage, visualization and processing resources. In this context, big data allows distributed storage and parallel data processing, enabling the extraction of information in the shortest possible time [ 43 ]. Such information are used as models by artificial intelligence (AI) systems—which, according to [ 44 ], can be understood as the ability of a system to operate as if it had the thinking capacity of a human being—and machine learning—that, according to [ 45 ] is a data processing technique to detect patterns and correlation among complex and unrelated data—for the development of decision support systems and automation of irrigation control systems [ 46 ], monitoring [ 47 ] and diseases detection in crops [ 48 ], for example.
Finally, the application layer comprises IoT applications that, supported by the other mentioned layers, provide management information to farmers, being able to manage the entire production process in the plantations.
Several related works are being developed in recent years. This rich literature has already been analyzed by the academia from multiple perspectives with objective of determining the state of the smart farming development. Thus [ 49 ] presented a systematic review of precision livestock farming in the poultry sector and [ 50 ] made a review of state of the art of technologies used in precision agriculture, focusing in the innovations, measured parameters, technologies and application areas. On the other hand [ 4 ] has focused on the use of big data as a tool to support agriculture, pointing out the main opportunities and challenges of using this technology. Finally, [ 51 ] presented a quantitative literature review on smart farming related papers, helping to outline an overview of academic production related to the subject. In this way, the present work aims to complement such analyses by making a systematic review of IoT solutions applied to smart farming.
To reach the proposed objectives, this study has used the Preferred Reporting Items for Systematic Reviews (PRISMA) methodology, which is a framework developed to support reports and systematic reviews of literature [ 52 ].
As a research strategy, in October 2019 a search was made in the Scopus database through the search tool available on the website. In addition, in June 2020 a new search was made in the same database to include papers published in 2020. The choice of this database took into consideration its scope and relevance in the academia, since this database indexes several journals and catalogues, such as IEEE, ACM and Elsevier, besides being widely used in similar bibliographic reviews, as in [ 4 ] and [ 51 ]. In addition, in February 2020 a new search was performed in the same database. The strategy adopted for the work research in this database looked for terms used to refer to the application of technology in the area of agriculture, such as “Precision Agriculture”, “Precision Farming”, “Smart Farming” and “Smart Agriculture” in association with “IoT” and synonyms terms. The publication date of the articles was not a criterion for ignoring them. The scope of the research was limited to documents such as journal and conference articles, published in English, Portuguese or Spanish, and whose access was fully available. Thus, the resulting search instruction for the database was as follows:
(“Smart Farming” OR “Smart Agriculture” OR “Precision Farming” OR “Precision Agriculture”) AND (“IoT” OR “Internet of Things” OR “internet-of-things”) AND (LIMIT-TO(ACCESSTYPE(OA)))
It should be noted that the quotation marks have the function of ensuring that terms composed of multiple words were searched together, thus preventing words from being considered individually.
After extracting the articles that resulted from the search, they were manually reviewed through the analysis of the title, keywords, abstract and text. Initially, based on this review, the works identified in the researched database were consolidated, thus eliminating duplicate articles.
Subsequently, the articles were validated as to their framing in the objectives proposed for this study and considered valid when: (i) they were not a review or bibliographical research (ii) they were related to theme (iii) they presented a technology or solution based on IoT to solve problems related to agriculture (iv) they were published in English, Portuguese or Spanish. Furthermore, works were also excluded when they were related to livestock activities instead of agriculture.
The process of searching and selecting papers for this study followed the workflow summarized in Figure 2 , where it can be observed that the initial search resulted in a total of 463 articles, which were analyzed, filtered and classified in a narrowing process that culminated in the selection of 159 articles.

PRISMA flowchart of the systematic review on state-of-the-art IoT solutions in smart farming.
In the identification phase 463 articles were selected with the search tool.
During the screening phase, a manual review of the articles was carried out to identify in the titles, abstract and key words the papers adherent to the objectives proposed for this study, following the criteria mentioned in this section. Among these, 257 were considered invalid and discarded. About 62% of the discarded items did not consider smart farming to be the focus of the work, although some presented improvements for IoT that could benefit smart farming indirectly. Additionally, almost 31% of the discarded papers were studies or literature reviews related to smart farming and the use of various technologies. A smaller number of papers related to smart farming but not addressing IoT (about 5%) and papers where the abstract or text were not available (about 2%) were also discarded.
During the eligibility phase, the content of the 206 resulting articles were reviewed and the papers were classified using the same criteria used in the previous step. In this phase 47 articles were discarded. Among the discarded articles 29% were not related to IoT and 30% were not related to smart farming. The other 41% of the discarded papers were paper reviews or papers without content available. This analysis resulted in 159 articles considered eligible which were included as a sample for this study.
4. Discussion
Based on the results obtained in the analysis of the articles considered for this study, it was possible to observe a growth trend in the number of publications related to IoT and smart farming since 2011, with special emphasis from 2016 onwards, as shown in Figure 3 .

Classification of reviewed papers according to the year of publication.
It is possible to observe an expressive increase of 278% in the number of published papers in 2017/2018. It’s also possible to observe a very similar number of published papers in 2019/2020, until the first semester of 2020. The amount of published papers in recent years evidences the increasing in discussion and the relevancy of the topic IoT applied to smart farming.
Within the reviewed papers it was identified the main scenarios and environments of agriculture. As shown in Figure 4 , such scenarios can be divided into indoor and outdoor. Environments for indoor scenario are protected from climatic impacts, such as solar radiation, rain and wind. Examples of environments for indoor scenarios include greenhouse, hydroponics, crop beds, pots, etc. In contrast, environments for outdoor scenario are more susceptible to climatic impacts. Examples of environments for outdoor scenario are arable lands, orchards and generic outdoor plantation.

Typical scenarios in agriculture can be divided in indoor and outdoor. Indoor agriculture includes environments such as greenhouse, hydroponics and crop beds. Outdoor agriculture includes environments such as orchards and arable lands. IoT solutions that may be applied to multiple environments are referred to as “Generic”.
4.1. Application
Within the reviewed papers it was also identified that the most common applications of IoT solutions for smart farming are:
- Chemical control (e.g., pesticides and fertilizers).
- Crop monitoring.
- Disease prevention.
- Irrigation control.
- Soil management.
- Supply chain traceability
- Vehicles and machinery control.
Table 2 presents the reviewed papers, grouped by agricultural environment and application of the IoT solution. It is worth mentioning that several IoT solutions presented on the reviewed papers could be applied to multiple environments ( Figure 4 ). Thus, such IoT solutions are classified as “Generic”. Additionally, the “Others” column in Table 2 includes papers whose IoT solutions were developed for agricultural environments that were less mentioned, such as pots, crop beds, etc. It is possible noting a predominance in projects where the application is for crop monitoring, irrigation management, and disease prevention.
Smart farming, application and environments.
As shown in Table 2 , the most common application of IoT solutions for smart farming is crop monitoring. Moreover, as shown in Table 2 , these solutions have been developed for multiple agricultural environments, such as arable lands, orchards, greenhouses, etc. The fact that this type of application is so common in agriculture can be justified by the relevance that crop monitoring has for farmers. IoT solutions developed for monitoring crops focused on collecting environmental data of plantations (such as temperature, humidity, luminosity, etc.). Farmers can use these data to obtain a better insight of the plantations. For example, such data was used to determine the vigor of rice [ 47 , 58 ], alfalfa [ 30 ] and maize [ 57 ] crops and to control the environmental conditions of greenhouses [ 99 , 100 , 102 , 104 ]. Similarly, IoT solutions for irrigation control has also been developed for multiple agricultural environments, as demonstrated in Table 2 . Such IoT solutions aimed to optimize the use of water resources in agriculture in different ways, such as by simply using sensors for measuring the soil moisture and using these data for controlling the irrigation source [ 34 , 139 ] or in a more sophisticated way, by combining humidity data with datasets of weather to determine the amount of water required during the irrigation [ 140 ]. IoT solutions for disease prevention aimed to identify and prevent diseases on plantations. For this purpose, these IoT solutions collected multiple environmental and plantation data, such as images of plants [ 129 , 132 , 134 ], sounds [ 135 ], temperature, humidity, etc. [ 131 , 137 ]. These data were processed with different approaches, such as image processing [ 129 , 134 ] or artificial intelligence [ 132 , 137 ]. For example, the IoT solution developed in [ 129 ] processes images collected from a sugarcane crop and identifies diseases on the leaves of plants. In addition, [ 135 ] developed an IoT-enabled device that captures sounds produced by larvae inside trees. IoT solutions for chemical control presented in Table 2 aimed to optimize the application of fertilizers and pesticides on plantations. For this purpose, these IoT solutions collect data (such as nitrogen, salinity or PH) from the crops. Based on the collected data, such IoT solutions can identify crop areas that may require the application of fertilizers or pesticides. For example, in [ 54 ] aerial images of crops are processed to determine the nitrogen concentration in a large plantation. These images are useful to determine the specific region that requires fertilizer. In addition, [ 55 ] developed an automated robot that optimizes the application of pesticides in greenhouse cultivations. IoT solutions for soil management aimed to identify different soil attributes used for planting. For example, such IoT solutions are used to measure the soil moisture [ 163 ], to identify the water consumption pattern [ 159 , 161 ] and to identify the nutrients of the soil [ 158 ]. IoT solutions for vehicles and machinery control focused on collecting data of and managing agricultural equipment and machinery such as tractors, harvesters and trucks. For this purpose, IoT solutions had to deal with the characteristics inherent to agricultural equipment, such as mobility. Data from the equipment itself, such as implement status, engine performance, or speed are collected using sensors [ 41 ] to optimize their maintenance cycle. Additionally, due to the mobility of agricultural equipment, opportunistic computing was used to collect data from remote crop areas by using sensors coupled to tractors [ 174 ].
Each agricultural environment presented in Table 2 brings its own challenges for the projects, which includes the environment impact on the communication between sensors, either by the distance between the sensor nodes [ 25 , 105 , 180 ], by the lack of communication in the croplands [ 98 , 174 ] or even by the impact of vegetation in the signal propagation [ 70 , 175 ]. Furthermore, as indicated in [ 28 ], climatic elements—such as rain, snow or solar radiation—have influence on both the planting and the sensor nodes.
To cover these scenarios commercial electronic sensors are used by 96% of the reviewed papers. This expressive usage can be justified by the fact that such sensors are affordable, certified, ready-to-market and meet the main monitoring needs in IoT solutions for smart farming. Such sensors are used for collecting real-time data about multiple agricultural parameters, such as climatic data, substrate information, luminosity, CO2 concentration and images through cameras and multispectral sensors, as shown in Table 3 . Moreover, several papers (4%) focused on developing custom-made sensors for monitoring specific agricultural aspects, such as soil nutrients (e.g., nitrate [ 158 ]) and leaf evapotranspiration for measuring the hydric stress in tobacco crops [ 81 ].
Types of physical sensors and use in smart farming.
Different types of sensors are used in agriculture for collecting data from different aspects of agriculture such as crop monitoring, substrate monitoring and environment monitoring.
As presented in Table 3 , different types of sensors were used in IoT solutions for smart agriculture to collect data from multiple aspects of agriculture, such as the crop, substrate, environment and other. For this purpose, as shown in Table 3 , for environment monitoring electronic sensors were used in IoT solutions to collect environmental data, such as temperature, humidity and luminosity [ 104 , 109 , 114 ]. In addition, for substrate monitoring electronic sensors were used to collect data from the substrate (e.g., soil and water), such as temperature, moisture and nitrogen. Likewise, pH sensors were used for measuring the acidity or the alkalinity of the water in hydroponics cultivations. For crop monitoring, cameras and multispectral sensors were used to collect images of crops. These sensors can be installed on an UAV to obtain aerial images of large plantations [ 47 , 57 , 58 ] or used in robots to retrieve a detailed image of the leaf of a plant [ 111 ].
4.2. Perception
The choice of hardware is a very important aspect of the IoT project development because it impacts the costs and the technologies that can be used. 60% of the reviewed papers mentioned the hardware used to support the IoT solution. Furthermore, SBCs were mentioned by 40% of the reviewed papers. The use of SBCs can be justified by the fact that these devices are affordable and versatile [ 38 ], enabling the development of custom-made IoT devices. For example, some SBCs such as Arduino has an integrated development environment (IDE). This IDE enables the development of custom programs to be installed as firmware on the Arduino boards [ 184 ]. Similarly, Raspberry Pi is compatible with several operating systems, such as Raspbian, Ubuntu Core or Mozilla Web Things [ 185 ]. Some of these operating systems are open-source, which allow for the customization of its source-code. Besides, these operating systems support applications developed with programming languages such as Python [ 26 ]. Furthermore, the capabilities of SBCs can be extended by associating them with other hardware components, such as sensors or transceivers. This characteristic makes SBCs able to work as gateways or sensor nodes in IoT solutions. Among the papers that mentioned SBCs, 82% mentioned the use of Arduino, Raspberry Pi and ESP boards (such as ESP8266, ESP12 and ESP32). Table 4 presents the application of embedded system platforms and UAV devices in smart farming.
Embedded system platforms and UAV devices in smart farming.
As shown in Table 4 , IoT-enabling devices are used for multiple applications on IoT solutions for smart farming. SBCs were used both as sensor nodes and gateways. Table 4 reveals that Arduino was the most commonly used embedded system platform among the reviewed papers. The extensive use of Arduino can be justified by the fact that Arduino is open-source hardware that enables the development of different devices through the use of boards that extend their native functionality. Table 4 also shows that embedded system platforms have been more widely used in IoT solutions for crop monitoring. As sensor nodes, for example, in [ 124 ] sensors for collecting environmental data such as soil humidity, solar radiation and rain are connected to an Arduino Uno. The Arduino is, then, used to monitor the health of a vineyard. Likewise, in [ 117 ] a Raspberry Pi is used to manage the temperature and air humidity of a greenhouse. IoT devices are also used as gateways to connect short-range WSN with the internet by using long-range communication protocols. For example, in [ 127 ] a gateway is used to connect WSNs using 3 different protocols (ZigBee, Bluetooth and Wi-Fi) with a remote server by using 3G. In [ 84 ] a LoRaWAN gateway obtains data from sensor nodes using LoRa and retransmits this data to a cloud-hosted platform by using 4G. 3G and 4G are cellular network technologies that, as discussed in Section 2.3 , enable communication in long distances and with a high data transmission rate. These technologies will be discussed with more details in Section 4.3 .
In addition, Table 4 also reveals that UAV is widely used by IoT solutions for monitoring crops, disease prevention and chemical control. The use of UAV for crop monitoring is due to the fact that UAV has the potential to accelerate and reduce the cost of monitoring extensive crops. For this purpose, cameras and multispectral sensors are attached to UAV devices that are used to obtain aerial images from large crops. Such images are processed by the IoT solution to calculate agricultural parameters, such as the leaf area index (LAI). The LAI is a parameter used to determine the vegetation coverage within a specific area. LAI, combined with other parameters, can be used to evaluate the amount of nitrogen in rice crops [ 58 ], determine the vigor of rice and maize [ 47 , 57 ] crops and detect diseases in sugarcane crops [ 129 ]. Moreover, UAV devices are used in [ 46 ] to optimize the application of pesticides and fertilizers in arable lands.
4.3. Network
Data obtained with sensor nodes are usually sent to the destination (e.g., database, server, IoT platform) through a wired or wireless network. Within the reviewed papers, 60% have mentioned the network protocol used in the IoT solution. Among the mentioned network protocols, CAN and Ethernet were the most used ones for wired networks. Likewise, LoRaWAN and protocols for cellular network (e.g., GPRS, 3G, etc.) were the most used protocols for long-range wireless networks. Analogously, ZigBee, Wi-Fi and Bluetooth were the most used protocols for short and mid-range wireless networks. Table 5 shows network protocols used for the IoT solutions within the reviewed articles.
Use of network protocols in smart farming for different farming scenarios.
As shown in Table 5 , several network protocols are used in different environments of agriculture (e.g., arable land, greenhouse, orchard) to enable communication between IoT solution devices, such as sensor nodes and gateways. Such network protocols enable the creation of short or long-range networks. Table 5 reveals that for short and middle-range communication, IoT solutions of the reviewed papers used different technologies, such as Wi-Fi, ZigBee and Bluetooth. Moreover, it is possible to observe in Table 5 that Wi-Fi is the most common network technology for communication within the analyzed articles. This extensive use of Wi-Fi can be justified by the fact that Wi-Fi is a ubiquitous technology and, therefore, easy to implement. However, due to the higher energy consumption of Wi-Fi, low-energy consumption technologies, such as ZigBee or Bluetooth, are also extensively used. For example, [ 62 ] used ZigBee to send images from a plantation to a remote server and [ 188 ] developed a sensor node that uses Bluetooth to deliver monitoring information from the farm directly to an application installed on a smartphone. Table 5 also demonstrates that IoT solutions of the reviewed papers used cellular networks, Sigfox, or LoRaWAN for long-range networks. Cellular networks are prevalent in IoT solutions for Smart Farming. This can be justified by the fact that cellular networks allow the communication of IoT devices in long distances and with a high data rate. For example, [ 141 ] uses cellular network to send data collected from humidity sensors to a cloud-based platform and to control an irrigation system. Similarly, Sigfox and LoRaWAN enable communication in very long distances while requiring low energy to operate. Based on these characteristics, Sigfox and LoRaWAN were used for long-range communication, as an alternative to cellular networks or in regions where there was no cellular network coverage. Sigfox is used in [ 34 ] as the network protocol of an IoT solution used to control the irrigation of a plantation. Likewise, in [ 137 ] the LoRaWAN is used to send data from multiple sensors installed in a greenhouse to a remote platform.
Besides the distance between sensor nodes, gateways, and other network elements, the vegetation itself can be an obstacle for sensor communication, as demonstrated by [ 175 ] and [ 70 ] who analyzed the impacts on signal propagation on 433 MHz and 2.4 GHz frequencies in rice plantations and an orchard. An additional challenge for greenhouses arises from the high density of sensors, which can lead to interference in the wireless signal due to proximity [ 105 , 112 , 180 ]. To mitigate this problem wired networks, such as CAN [ 100 ] or Ethernet [ 122 ], can be used. As shown in Table 5 , these technologies have been more used in greenhouses, because usually this type of agricultural environment is more appropriated for implementing wired networks. Moreover, [ 112 ] investigated the path loss on wireless signals and concluded that the proper positioning of directional antennas can optimize the number of sensory nodes required for monitoring a greenhouse.
Network topology is another important aspect of an IoT solution. According to [ 61 ] the topology of sensor networks can be star, tree (or cluster) or mesh. The network topology impacts the distance between the sensor nodes and the destination and, consequently, the number of sensor nodes in the WSN [ 190 ]. For example, star networks are composed of a central node (coordinator) and several peripheral nodes. In such topology, peripheral nodes send data to the central node [ 93 ]. Therefore, the maximum distance between the peripheral nodes and the central node is limited by the maximum distance allowed by the physical layer communication standard. On the other hand, as discussed in Section 2.3 , in mesh networks each node has routing capability, hence extending the network coverage by allowing multi-hop communications [ 191 ]. Based on the architecture of the IoT solution and on the project description it was possible to identify the topology adopted by 61% of the reviewed papers. For example, a star topology is used in [ 34 ] for connecting sensor nodes to a central node using the LoRa protocol. This central node acts as a gateway and retransmits messages to a cloud-based application that controls an irrigation system using Sigfox. Also, in [ 107 ] the star topology is used to connect multiple sensors within a greenhouse. Such sensors use the ZigBee protocol to send messages to a central node, which acts as the network gateway. Mesh networks are considered more complex to be implemented but also more reliable due to the redundancy of communication between the sensor nodes [ 105 ]. Such topology is used in [ 105 , 107 ] for monitoring a greenhouse. Tree (or cluster) networks combine multiple star networks. Both [ 61 ] and [ 178 ] implement a cluster network for monitoring crops. In [ 61 ] sensor nodes collect information from a crop and send messages to a router node. This router node acts as the gateway of the cluster and retransmits the message to the main router node of the network. In [ 178 ] several router nodes are deployed in the crop area in order to optimize the energy consumption of sensor nodes.
Furthermore, embedded system platforms have been used to support network topologies. The chart in Figure 5 presents the distribution of embedded system platforms by network topology or device connection type. It is worth mentioning that although point to point is not a network topology, this type of device connection was used in several IoT solutions within the review articles. As shown in Figure 5 , Raspberry Pi is often used in IoT solutions implementing the star network topology. Arduino is the embedded system platform used in multiple types of network topology or device connections. Additionally, Arduino is the most frequently used embedded system platform to support star network topology and point-to-point communication. Finally, ESP-based devices include devices that use system-on-a-chip (SoC) modules such as ESP-32 and ESP8266 (Espressif Systems, Shanghai, China). ESP-based devices are often used in IoT solutions that implement star network topology or point-to-point communication.

Distribution of IoT-enabling devices by network topology or device connection type within the reviewed papers.
As mentioned in Section 4.2 , embedded system platforms can be used to build gateways or sensor nodes. As shown in Figure 5 the use of Raspberry Pi, Arduino and ESP stand out, probably because such embedded system platforms are cost-effective [ 38 ] and enable different network protocols (e.g., ZigBee, Wi-Fi and Bluetooth) with the use of transceivers. This characteristic allows such embedded system platforms to act as sub-nodes and central nodes in a star network [ 27 , 30 , 34 ] or as router nodes in mesh and cluster networks [ 106 , 178 ].
IoT devices transmit information to cloud-based platforms or applications through application protocols [ 109 ]. Such protocols can follow the publisher/subscriber architecture which, as mentioned in Section 2 , are appropriate for devices with limited computing resources. Among the application protocols used in the reviewed papers HTTP, MQTT and CoAP stand out. Such application protocols are useful to enable compatibility between non-standardized IoT devices and IoT platforms. For example, SmarFarmNet developed in [ 32 ] adopts the “bring your own IoT device” concept by implementing loosely coupled application protocols such as MQTT and CoAP. Furthermore, although HTTP is not a specific protocol for machine-to-machine (M2M) communication, its use associated with REST APIs enables low coupling between IoT devices and applications, analogous to MQTT, for example. However, as [ 109 ] concludes, the MQTT protocol is preferable for smart farming applications due to its resiliency, interoperability across different network protocols and transmission rate.
Finally, although the power consumption is not an exclusive topic within the transport layer, according to [ 178 ] the highest power consumption for IoT devices within a WSN occur during the transmission of data. This review identified several approaches for optimizing the power consumption in IoT solutions for smart farming. Among the identified solutions are the use of low energy protocols (e.g., BLE, ZigBee, Sigfox), reduction of data transmission in sensor nodes by an optimized duty cycle [ 177 , 178 , 192 ] and the use of message routing approaches that are more energy-efficient [ 72 , 193 ].
4.4. Processing
Among the analyzed papers it was possible to observe that initially, the main objective of IoT solutions was to collect and store data from sensor nodes. However, in more recent years, it is possible to observe an increasing number of IoT solutions that used supplementary techniques and technologies to treat the collected data, such as cloud computing and big data. Likewise, it is possible to observe an increasing number of works that used simultaneously two or more techniques or technologies for processing data. As shown in Figure 6 , the most cited technologies within the reviewed papers are cloud computing (34%), machine learning (15%), big data (13%), and artificial intelligence (9%).

Techniques and technologies for data-processing in smart farming identified within the reviewed papers. In recent years, the use of modern processing techniques, such as artificial intelligence and big data became more common. IoT applications relies on cloud computing for storing and processing the big data of agricultural information collected by IoT devices.
Table 6 presents IoT solutions that relied on cloud-based platforms for processing data and highlights the main data processing techniques (e.g., Artificial Intelligence, Big Data, etc.). The column “Other/Not identified” comprehends IoT solutions that have used cloud-based platforms but have either (i) used any of the data processing technologies identified by other columns on Table 6 or (ii) not explicitly mentioned the type of data processing technology that was adopted.
IoT-enabling platforms and data processing technologies used in smart farming within the reviewed papers.
Table 6 reveals that the most found cloud-based platforms in the reviewed papers are ThinkgSpeak, FIWARE, Ubidots, SmartFarmNet, AWS IoT and Thinger.io. In particular ThingSpeak is the most used cloud-based platform across all the reviewed papers, due to the fact that this platform is open-source with low infrastructure requirements [ 34 ]. In addition, Table 6 shows that AWS IoT was used with a higher number of data processing techniques. Not all cloud-based platforms offer the same set of functionalities, but in general, they have capabilities for data storage [ 30 , 94 , 102 , 122 , 132 ], processing [ 194 ] and visualization [ 102 ] and action control on farms [ 34 ]. Furthermore, Table 6 also reveals that, even though there are multiple cloud-based platforms, several reviewed papers developed their own cloud-based platform for the IoT solution.
Cloud-based platforms provide scalability for IoT solutions by relying on cloud computing to process and data. For instance, some platforms shown in Table 6 , such as Thinger.io [ 33 ], are built entirely on top of infrastructure services provided by cloud providers (e.g., Amazon AWS and Microsoft Azure). Also supported by such services, the platforms make available data analysis modules with graphics and panels that allow real-time monitoring of the information obtained or the creation of customized panels from the integration of multiple data [ 33 ].
Due to the scalability provided by these platforms, the large amount of data generated by the sensors is stored in databases to form the so-called big data, an unstructured set of information that is used to generate information about crops. According to [ 197 ] big data demands the use of technologies to optimize the processing time due to the large volume of information. For example, Hadoop—a parallel database for big data applications—proved to be efficient when analyzing the rainfall index data from several meteorological stations [ 197 ].
IoT solutions use different types of techniques and technologies for processing the collected data. Table 7 presents commonly used technologies per applications as identified in the reviewed papers. Column “Other Technologies” encompasses all the technologies that are not identified by any of the other columns in Table 7 .
Technologies and application in smart farming.
Table 7 reveals that the most commonly used technologies to support data processing are artificial intelligence, machine learning, and big data. The use of these technologies is related to their ability to process large amounts of information in a short time. In addition, Table 7 also shows that crop monitoring is the most common type of application for IoT solutions that have used data processing technologies. Moreover, crop monitoring is also the type of application that used the most different technologies for data processing. This can be understood by the fact that usually IoT solutions for monitoring crops collect a bunch of data and rely on machine learning and big data to process such data.
As demonstrated in Table 7 , bigdata was used for different applications in IoT solutions, such as crop monitoring, soil management and irrigation control. For example, supported by big data, in [ 142 , 161 , 194 ] the soil moisture data gathered by physical sensors were related to data made available in datasets, such as the NASA Prediction of Worldwide Energy Resources (POWER) [ 198 ]—which contains meteorological data—purchase and sale values of crops, information from the user and government agencies to optimize the amount of water in irrigation cycles, support the farmer in the acquisition of agricultural inputs—such as seeds and fertilizers—and generate information and perspectives about other activities related to agriculture. Big data was also used by [ 56 ] in the development of a decision support system to provide irrigation and monitoring advice to farmers from a knowledge base created with data obtained by physical sensors (e.g., temperature, soil moisture) and virtual sensors (e.g., soil type, season). Virtual sensor is a type of software that, given the available information, processes what a physical sensor otherwise would [ 199 ].
In addition, automatic management with IoT depends on the manipulation of multiple variables. Initially, the simple observation of soil humidity and temperature can be used to trigger irrigation or cooling systems, as proposed by [ 187 ]. Nevertheless, greenhouse management can be more complex. As shown in [ 104 , 107 , 120 ], greenhouse parameters like temperature and humidity are closely tied and changing one of them can affect several others.
Fuzzy logic, as indicated in Table 7 , was used in IoT solutions applications that need to handle multiple variables, such as irrigation control and monitoring crops. For this purpose, [ 120 ] uses fuzzy logic to handle multiple variables of temperature and humidity into a greenhouse and determine when a cooling system and an irrigation system should be started. Similarly, [ 73 ] uses fuzzy logic to optimize the number of sensors for monitoring soil temperature and moisture. Machine learning was also used in data processing by [ 46 ] to predict environmental conditions based on the forecast values of weather, humidity, temperature and water level and thus to control an irrigation system, by [ 47 ] to combine multiple parameters obtained from images, such as color and texture indices and by [ 48 ] to identify marks on the plants and, thus, to identify possible diseases. Similarly, in [ 58 , 125 ] it was used to detect diseases, identify growth stages and the health of plantations.
Similarly, as shown in Table 7 , IoT solutions used computer vision for applications that need to deal with image processing, such as crop monitoring and diseases prevention. It was also possible to observe in the reviewed papers the use of computer vision to identify and classify elements in images obtained by cameras, enabling the identification of fruit in an orchard [ 200 ] or the existence of diseases and pests in plantations [ 48 , 129 , 133 ]. Additionally, in [ 133 ] computer vision was used as a monitoring tool to detect the presence of insects that can cause diseases in olive groves and in [ 48 ] the same technique was employed to analyze diseases that cause morphological deformations in plants. Additionally, computer vision was used in crop management systems, for example in [ 134 ] where it was implanted in a robot equipped with a camera and other sensors, being able to obtain images of vegetation and, through computer vision, detect weeds in plantations and eliminate them. Similarly, in [ 111 ] a robot can identify a plant and interact with the environment to irrigate it, if necessary.
Finally, blockchain proved to be an opportune technology for systems that need to implement traceability of the supply chain, as shown in Table 7 . According to [ 171 ] blockchain is a global public distributed ledger that records all transactions between users. In fact, this type of control is relevant for agriculture in several aspects, such as food safety, guarantee of origin or cost reduction. To ensure information security, this technology was proposed by [ 30 , 167 , 171 ] for agricultural product traceability. For example, in [ 171 ] an IoT solution uses blockchain to record information regarding the tea production based on 5 business processes: production plan, quality inspection, sales processing, product quality inspection and order delivery. In [ 167 ] a production tracking system for agricultural cooperatives have been developed. In [ 30 ] a similar system is being proposed but still in development stage.
5. Considerations
IoT solutions for smart farming take advantage of the scalability provided by platforms and cloud computing to store large amounts of data obtained by sensors. These big data of specific information may be processed with artificial intelligence techniques—such as machine learning—to improve the management of smart farming. For example, the processing of big data may be used to obtain crop insights, optimize water resources and increase the crop quality by preventing disease and reducing the amount of chemical products employed. Crop monitoring solutions use SBC (e.g., Arduino and Raspberry Pi) or UAV (e.g., drones) together with sensors (e.g., humidity, temperature, CO 2 or image) to collect data in indoor or outdoor environments.
Different types of network connections are used for communication between IoT devices, such as wired and wireless connections. The review shows that wired networks, such as CAN and Ethernet, are used for indoor agriculture (e.g., greenhouses). The use of wired network on indoor agriculture may be justified by the fact that in this scenario the physical components of the network are less susceptible to climatic agents impacts. Likewise, generally distance between sensor nodes in indoor agriculture enables this type of connection. Wireless connection, on the other hand, is used both in indoor and outdoor agriculture. Wi-Fi is the most mentioned protocol within the analyzed projects, due to its ubiquitous utilization in the daily life. However, power consumption and signal range characteristics may limit use of Wi-Fi in larger projects or in projects with power restrictions. To overcome the power consumption issue, energy-efficient protocols such as ZigBee, BLE or LoRa are used for communication in wireless networks.
Furthermore, it is worth mentioning that this review investigated papers where the IoT solution for smart farming was applied to agriculture only. However, the use of IoT for smart farming can also be applied to other activities related to farming, such as livestock [ 201 ]. Moreover, despite the fact that power-supply in IoT solutions for smart farming does not represent a specific layer of an IoT solution architecture [ 17 , 18 ], this topic has been covered in some of the reviewed papers. For example, [ 72 , 177 ] proposed improvements in algorithms for message routing and in duty cycles in sensor nodes. These approaches contribute to the reduction of power consumption by IoT devices. Similarly, a mission-based approach was used in [ 53 ] to optimize the power consumption in UAV. This approach was used to identify the most efficient path for a set of drones. Likewise, [ 177 ] proposed an intelligent activity cycle to improve the performance of data aggregators in terms of energy efficiency on cloudy days.
6. Conclusions
This work presented a systematic review of the state-of-the-art of IoT adoption in smart agriculture and identified the main components and applicability of IoT solutions. This review reported a change in the treatment of data in recent works: while previous work showed that the majority of decision support systems used simple processing mechanisms to handle data collected in real-time, more recent work showed an increasing number of management systems that use complementary technologies that rely on cloud and big data computing for processing large amounts of data. Furthermore, it was observed in this review that in recent work the use of artificial intelligence and image processing techniques has become more common to improve the management of smart farming. From the identified applications of IoT for smart farming it was observed that the most common application is the monitoring of crops. This review also showed that different network protocols may be simultaneously used in IoT solutions for smart farming. In addition, the comparison of types of network connections used in IoT solutions for smart farming revealed that wired networks are used in indoor scenarios (e.g., greenhouse) while wireless networks are used both in indoor and outdoor scenarios (e.g., arable lands, orchards). Moreover, the review discussed in this work suggests the increasing relevance of IoT solutions for smart farming. Future work may extend this review by including other relevant articles and complementary analysis of project costs, usability and regional challenges intrinsic to IoT applications. Another important future research direction could be the analysis of the edge and fog computing usage in smart agriculture as a way to deal with challenges associated with traditional centralized cloud solutions such as high communication latencies, lack of support for real-time reaction to detected events, large bandwidths, etc.
Acknowledgments
The authors acknowledge to the Mobile Computing Laboratory of the School of Technology and Management of the Polytechnic Institute of Leiria and to the Computer Science and Communication Research Center for the facilities granted in the implementation of part of this work, in the context of the Smart IoT Ecosystems research line.
Author Contributions
All authors contributed equally to this work. Conceptualization, E.N., N.C., A.P.; data curation, E.N., N.C., A.P.; formal analysis, N.C., A.P.; funding acquisition, A.P.; investigation, E.N., N.C., A.P.; methodology, N.C., A.P.; resources, A.P.; supervision, A.P.; validation, N.C.; writing—original draft, E.N., N.C.; writing—review & editing, E.N., N.C, A.P. All authors have read and agreed to the published version of the manuscript.
This work was partially supported by Portuguese Fundação para a Ciência e a Tecnologia–FCT, I.P., under the project UIDB/04524/2020” and by Portuguese National funds through FITEC–Programa Interface, with reference CIT “INOV–INESC Inovação–Financiamento Base”.
Conflicts of Interest
The authors declare no conflict of interest.
Accessibility Links
- Skip to content
- Skip to search IOPscience
- Skip to Journals list
- Accessibility help
- Accessibility Help
Click here to close this panel.
Purpose-led Publishing is a coalition of three not-for-profit publishers in the field of physical sciences: AIP Publishing, the American Physical Society and IOP Publishing.
Together, as publishers that will always put purpose above profit, we have defined a set of industry standards that underpin high-quality, ethical scholarly communications.
We are proudly declaring that science is our only shareholder.
Smart Agriculture and Smart Farming using IoT Technology
V Dankan Gowda 1 , M Sandeep Prabhu 2 , M Ramesha 3 , Jayashree M Kudari 4 and Ansuman Samal 5
Published under licence by IOP Publishing Ltd Journal of Physics: Conference Series , Volume 2089 , 1st International Conference on Applied Mathematics, Modeling and Simulation in Engineering (AMSE) 2021 15-16 September 2021, India (Virtual) Citation V Dankan Gowda et al 2021 J. Phys.: Conf. Ser. 2089 012038 DOI 10.1088/1742-6596/2089/1/012038
Article metrics
5860 Total downloads
Share this article
Author e-mails.
Author affiliations
1 Department of Electronics and Communication Engineering, B.M.S Institute of Technology and Management, Bangalore, Karnataka, India
2 Department of Electronics and Communication Engineering, Canara Engineering College, Benjanapadavu, Bantwal, Mangalore, Karnataka, India
3 Department of Electronics and Communication Engineering, GITAM School of Technology GITAM(Deemed to be University), Bangalore, Karnataka, India
4 Department of Computer Science and Information Technology, JAIN Deemed to be University, Bangalore, Karnataka, India
5 Faculty of Hospitality and Tourism Management, Siksha O Anusandhan Deemed to be University, Bhubaneshwar, Odisha, India
Buy this article in print
It has become easier to access agriculture data in recent years as a result of a decline in digital breaches between agricultural producers and IoT technologies. These future technologies can be used to boost productivity by cultivating food more sustainably while also preserving the environment, thanks to improved water use and input and treatment optimization. The Internet of Things (IoT) enables the production of agricultural process-supporting systems. Referred to as remote monitoring systems, decision support tools, automated irrigation systems, frost protection systems, and fertilisation systems, respectively. Farmers and researchers must be provided with a detailed understanding of IoT applications in agriculture as a result of the knowledge described above. This study is about using Internet of Things (IoT) technologies and techniques to enhance agriculture. This article is meant to serve as an introduction to IoT-based applications in agriculture by identifying need for such tools and explaining how they support agriculture.
Export citation and abstract BibTeX RIS
Content from this work may be used under the terms of the Creative Commons Attribution 3.0 licence . Any further distribution of this work must maintain attribution to the author(s) and the title of the work, journal citation and DOI.
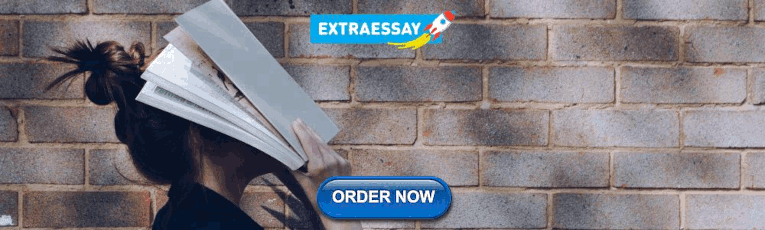
IMAGES
VIDEO
COMMENTS
Upon meticulous examination of the full-text papers, a further 119 articles from Scopus and 115 from Web of Science were ultimately excluded from the study. Consequently, 77 articles were retained for in-depth review, after accounting for duplicates across both databases. ... Smart agriculture plays a crucial role in enhancing environmental ...
This paper presents a comprehensive review of emerging technologies for the internet of things (IoT)-based smart agriculture. We begin by summarizing the existing surveys and describing emergent technologies for the agricultural IoT, such as unmanned aerial vehicles, wireless technologies, open-source IoT platforms, software defined networking (SDN), network function virtualization (NFV ...
Smart agriculture technology based on the Internet of Things (IoT) technologies has many advantages related to all agricultural processes and practices in real-time, which include irrigation and plant protection, improving product quality, fertilization process control, and disease prediction, etc.(Adamides et al., 2020).The advantages of smart agriculture can be summarized as follows: 1 ...
Abstract. The drive toward efficient yet sustainable agriculture in the contemporary world necessitates the adoption of smart farming. Smart farming refers to a set of solutions that increase agricultural efficiency, mainly through the use of information and communication technologies. We will write a custom essay on your topic.
The smart farming is a subset of precision agriculture, which is designed to control the suitable environments. for crops inside a greenhouse by using the least resources. It combines modern ...
A total of 90 papers have been identified, and content analysis was conducted to mine knowledge in the domain for 2011-2022. ... (2023): Smart agriculture: a literature revie w, Journal of ...
Smart agriculture is a rising area bringing the benefits of digitalization through big data, artificial intelligence and linked data into the agricultural domain. This chapter motivates the use and describes the rise of smart agriculture.
The applications of Smart Farming can contribute to enhancing sustainable development [197] [198] [199]. Smart Farming can provide numerous advantages to the agricultural industry, including ...
Smart farming is a development that has emphasized information and communication technology used in machinery, equipment, and sensors in network-based hi-tech farm supervision cycles. Innovative technologies, the Internet of Things (IoT), and cloud computing are anticipated to inspire growth and initiate the use of robots and artificial intelligence in farming. Such ground-breaking deviations ...
Smart farming reduces the ecological footprint of farming. Minimized or site-specific application of inputs, such as fertilizers and pesticides, in precision agriculture systems will mitigate leaching problems as well as the emission of greenhouse gases ( 6 ). With current ICT, it is possible to create a sensor network allowing for almost ...
Abstract: Despite the perception people may have regarding the agricultural process, the reality is that today's agriculture industry is data-centered, precise, and smarter than ever. The rapid emergence of the Internet-of-Things (IoT) based technologies redesigned almost every industry including "smart agriculture" which moved the industry from statistical to quantitative approaches.
The future of connectivity. Without a solid connectivity infrastructure, however, none of this is possible. If connectivity is implemented successfully in agriculture, the industry could tack on $500 billion in additional value to the global gross domestic product by 2030, according to our research. This would amount to a 7 to 9 percent ...
The growth of the global population coupled with a decline in natural resources, farmland, and the increase in unpredictable environmental conditions leads to food security is becoming a major concern for all nations worldwide. These problems are motivators that are driving the agricultural industry to transition to smart agriculture with the application of the Internet of Things (IoT) and big ...
In response to these challenges, the concept of Climate-smart Agriculture (CSA) has emerged as a holistic approach to end food security and promote sustainable development while addressing climate change issues. CSA is a set of agricultural practices and technologies which simultaneously boost productivity, enhance resilience and reduce GHG ...
The 19 papers included in this special issue examined the factors influencing the adoption of climate-smart agriculture (CSA) practices among smallholder farmers and estimated the impacts of CSA adoption on farm production, income, and well-being. Key findings from this special issue include: (1) the variables, including age, gender, education, risk perception and preferences, access to credit ...
Finally, reviewed papers published between 2010 and 2015 and presents a state-of-the-art of IoT solutions for smart farming and smart agriculture. Authors relied on an IoT architecture with three layers (perception, network and application) to analyze the application of sensor and actuator devices and communication technologies within several ...
1. Introduction. Smart technologies in agriculture will boost the production of farm crops and livestock since autonomous systems will be able to control actuators effectively, improve the utility, control resource usage, and ensure products conform to market requirements while maximizing profit and minimizing the cost of production [1].Smart farming refers to the use of technologies such as ...
It is vital that climate-smart agriculture takes the local context and cultural and social sensitivities into account, and ultimately listens to the local community about the approach that best fits their reality. Nuclear technology plays an important role in agriculture and food security, complementing conventional climate adaptation and ...
This study is about using Internet of Things (IoT) technologies and techniques to enhance agriculture. This article is meant to serve as an introduction to IoT-based applications in agriculture by identifying need for such tools and explaining how they support agriculture. Export citation and abstract BibTeX RIS. Previous article in issue.
Lack of proper knowledge and literacy regarding the scopes, mode of action, and implementation of IoT-based technology in faming can result in underutilization of the smart system in agriculture. Deployment of IoT-coupled smart sensors and accessories in rural farm areas, where the farmers are less familiar to advanced technologies could be ...
SMART AGRICUL TURE USING IOT. Kanumuri Dinesh V arma. IOT with Cyber Security. Bournemouth University. Bournemouth England. [email protected]. Abstract — Agriculture industry is ...
Development of smart agriculture practices. More productive and resilient agriculture requires a major shift in the way water, soil, nutrients and other agricultural resources are managed. Isotopic techniques can track and quantify carbon, water and nutrient movement and their dynamics under diverse agroecosystems to improve smart agricultural ...
Smart Agricultural Technology provides international coverage for practical applications and smart systems that integrate advanced computing with electronic instrumentation or controls for agricultural planning and production. This journal is a companion to the highly reputable Computers and Electronics in Agriculture, providing a forum for the broad application of technologies in: